Introduction
According to the WHO Rehabilitation Need Estimator, about 190 million people worldwide in 2019 had rehabilitation needs for mental disorders (aggregated as schizophrenia, autism spectrum disorders and developmental intellectual disability) (Institute for Health Metrics and Evaluation, 2022). Psychosocial interventions are at the core of psychiatric recovery. Their goal is to improve social integration, quality of life and overall functioning, by developing emotional, cognitive and social skills (Morin and Franck, Reference Morin and Franck2017). Studies show that psychosocial rehabilitation (PSR) programmes are effective in reducing residual symptoms, increasing everyday life activities (including employment) and reducing the likelihood of psychiatric admissions (Bighelli et al., Reference Bighelli, Rodolico, García-Mieres, Pitschel-Walz, Hansen, Schneider-Thoma, Siafis, Wu, Wang, Salanti, Furukawa, Barbui and Leucht2021; Gühne et al., Reference Gühne, Weinmann, Arnold, Becker and Riedel-Heller2015; Huxley and Baldessarini, Reference Huxley and Baldessarini2007; McGurk and Mueser, Reference McGurk and Mueser2004; Morin and Franck, Reference Morin and Franck2017).
A lot of PSR tools are available, such as psychoeducation (PE), family therapy, cognitive behavioural therapy (CBT), peer support, cognitive remediation (CR) or vocational training (VT) (Bighelli et al., Reference Bighelli, Rodolico, García-Mieres, Pitschel-Walz, Hansen, Schneider-Thoma, Siafis, Wu, Wang, Salanti, Furukawa, Barbui and Leucht2021; Kern et al., Reference Kern, Glynn, Horan and Marder2009). All these programmes should be considered as evidence-based practices (Huhn et al., Reference Huhn, Tardy, Spineli, Kissling, Förstl, Pitschel-Walz, Leucht, Samara, Dold, Davis and Leucht2014; Morin and Franck, Reference Morin and Franck2017), yet there is a paucity of evidence in the literature regarding how clinicians make decisions to refer to PSR interventions based on the needs and characteristics of patients. For instance, meta-analysis and systematic reviews of CR in schizophrenia have reported that, while there is considerable variability in individual treatment response (Biagianti et al., Reference Biagianti, Castellaro and Brambilla2021), the identification of response predictors is still an under-investigated topic (Altman et al., Reference Altman, Tan and Rossell2023). Of note, studies have been criticized for having used small datasets (Corbera et al., Reference Corbera, Wexler, Poltorak, Thime and Kurtz2017) and for having reported inconclusive findings (Barlati et al., Reference Barlati, Deste, Galluzzo, Perin, Valsecchi, Turrina and Vita2019; Medalia and Richardson, Reference Medalia and Richardson2005), with no high-quality replicated evidence (Seccomandi et al., Reference Seccomandi, Tsapekos, Newbery, Wykes and Cella2020). In schizophrenia, a recent meta-analysis concluded that heterogeneous treatment effects of PSR interventions had been explored, but no evident role for any of the moderators (e.g. sex, age, duration of illness and clinical severity) was found (Bighelli et al., Reference Bighelli, Rodolico, García-Mieres, Pitschel-Walz, Hansen, Schneider-Thoma, Siafis, Wu, Wang, Salanti, Furukawa, Barbui and Leucht2021).
However, these programmes have different clinical purposes and may not fit patients equally. For instance, the primary objective of PE is to improve insight and therapeutic alliance, while that of CR is to reduce the consequences of cognitive impairments, and that of vocational rehabilitation is to improve social functioning more directly (e.g. via increasing occupational activities). Therefore, it is likely that mental health clinicians try and tailor PSR interventions to the needs and characteristics of patients. For such a highly complex patient population, referrals to PSR interventions would be the product of complex interactions of many different factors, including socio-demographic characteristics, clinical history, current clinical issues and more in-depth psychological factors (e.g. insight, motivation for care and quality of life). Unfolding these interactions would be critical to better understand how these various factors guide clinical decisions. So far, however, studies have used traditional statistical tools that would be unable to take into account such a large number of predictors or their complex relationships with one another.
A potential solution to solve this challenge may be offered by machine learning methods. Machine learning models would typically take into account a vast number of variables and best predict an outcome based on their complex relationships with one another. Moreover, recent advances in explainable artificial intelligence methods have allowed better interpretability of machine learning models with regard to the contribution of each predictive factor (Watson et al., Reference Watson, Krutzinna, Bruce, Griffiths, McInnes, Barnes and Floridi2019). Here, we used these methods to identify major contributors of initial referrals to four PSR interventions in clinically stable patients with serious mental illness: CBT, CR, PE and VT. Specifically, our aims were to (1) fit a series of machine learning models aiming at predicting referrals to PSR interventions based on socio-demographic and clinical factors; (2) assess the models’ predictive performance and (3) determine the relative importance of the predictors. We reasoned that our approach would help assess whether decisions to refer to PSR interventions depend on socio-demographic and clinical characteristics, evaluate their relative importance when making referrals and highlight disparities in referrals (i.e. identifying populations that are more referred to certain treatment programmes). We used electronic health data from the French network of PSR centres, REHABase, and exploited a relatively large number of socio-demographic and clinical predictors.
Method
Data
Data source
Our dataset consists of patients enrolled in the REHABase cohort. The cohort includes patients with serious mental illness referred to 15 centres of a French PSR network (Franck et al., Reference Franck, Bon, Dekerle, Plasse, Massoubre, Pommier, Legros-Lafarge, Jaafari, Guillard-Bouhet, Quilès, Couhet, Verdoux, Gouache, Martin, Cervello, Demily and Dubreucq2019). Patients are referred to the centres by public mental health services, private psychiatrists and general practitioners or are self-referred. The vast majority of patients are referred by their secondary care services and continue to benefit from ongoing treatment from their treating team. Referrals are usually accepted based upon clinical assessments, provided that patients demonstrate (1) serious functional impairment resulting from their mental illness, which substantially interferes with one or more major life activities (NIMH, 2023); (2) minimal clinical stability and (3) a clear motivation to attend PSR programmes. Acceptance of referrals is based on clinical judgment and not on clinical or functional scales. Once in the service, patients undergo a standardized socio-demographic, clinical, functional and cognitive evaluation performed by a multidisciplinary team (psychiatrists, nurses, neuropsychologists, occupational therapists and social workers), collected in an electronic case report form. Patients subsequently benefit from a personalized rehabilitation care plan that may last a few months up to a year. Regular group meetings are held monthly to monitor quality control and ensure good inter-rater reliability.
REHABase is a cohort database but, as such, not a study. Therefore, the current study was not pre-planned in the REHABase cohort project. Our analysis was restricted to patients included in the REHABase cohort from January 2016 to January 2022. For the purpose of this study, we only included patients with a DSM-5 (Diagnostic and Statistical Manual of Mental Disorders, 5th edition) diagnosis of schizophrenia spectrum disorder; autism spectrum disorder; bipolar disorder; depressive disorder; anxiety disorder; post-traumatic stress disorder; obsessive compulsive disorder (the latter three disorders were further regrouped under the umbrella of anxiety disorder) or personality disorder, based upon a clinical interview performed by a psychiatrist (American Psychiatric Association, 2013). The database obtained the authorizations required under French legislation (French National Advisory Committee for the Treatment of Information in Health Research, 16.060bis; French National Computing and Freedom Committee, DR-2017-268).
Outcome variable
Our outcome variable was clinical referrals to one of the following four PSR programmes:
1. CBT. Under this treatment programme, we regrouped not only standard CBT but also mindfulness and acceptance and commitment therapy, which are often described as the 3rd wave of CBT (Hayes and Hofmann, Reference Hayes and Hofmann2021). CBT aims to help patients overcoming and/or developing awareness of, and accept or let go of, negative thoughts, feelings, physical sensations and behaviours.
2. CR. Methods compiled under this treatment programme aim to improve cognitive abilities via training of cognitive functions such as memory, attention or executive functioning, with the goal of durability and generalization. Four elements are included in CR programmes (Bowie et al., Reference Bowie, Bell, Fiszdon, Johannesen, Lindenmayer, McGurk, Medalia, Penadés, Saperstein, Twamley, Ueland and Wykes2020): (1) the presence of an active and trained therapist; (2) the repeated practice of cognitive exercises; (3) the structured development of cognitive strategies and (4) the use of techniques to improve the transfer of cognitive gains to the real world. For the purpose of this study, we included referrals to social cognition programmes in this category. Social cognition programmes focus on emotion processing, social perception, theory of mind skills and/or modifying interpretational cognitive biases.
3. PE. This consists in informing a person with a psychiatric disorder about the bio-psychosocial model of the disorder, its main symptoms, expected effects and side-effects of medication, maintenance treatment, psychotherapy and relapse prevention. This programme is specifically important to increase insight and therapeutic alliance, develop coping strategies and decrease self-stigma.
4. VT. This programme aims at increasing independent functioning and includes recreational activities, volunteering and supported employment (possibly with a period of preparation).
For the purpose of this study, we limited our analysis to the first recorded referrals. Further information is provided in the supplementary methods.
Predictors
The choice of predictors was guided by internal discussions among clinical experts of our network and aimed at generating a set of predictors that would best represent socio-demographic and clinical characteristics given the constraints of data availability. Thirty-seven predictors were included. The list of predictors can be found in Table 1. A detailed list of predictors is provided in the supplementary methods). Information on predictors was documented by assessors at baseline (i.e. before the beginning of the treatment).
Table 1. Socio-demographic and clinical characteristics of participants

Abbreviations: CBT, cognitive behavioural therapy; CR, cognitive remediation; PE, psychoeducation; VT, vocational training; CGI, clinical global impression; SQoL, subjective quality of life; WEMWBS, Warwick-Edinburgh Mental Well-being Scale; ISMI, internalized stigma of mental illness.
Missing data
Our dataset was not exempt from missing data (supplementary methods). Missing data were not omitted from the analysis, and participants were not excluded based on missing data. Rather, assuming that information available on our dataset was similarly available to clinicians, we reasoned that missingness may be an important factor when making a decision to refer to a PSR programme. Therefore, for each predictor with missing values, we added an additional ‘missingness indicator’ variable, used as a proxy for unmeasured confounding (Choi et al., Reference Choi, Dekkers and le Cessie2019). We imputed missing values with the median (for continuous variables) or mode (for categorical variables) of the corresponding variable (Berkelmans et al., Reference Berkelmans, Read, Gudbjörnsdottir, Wild, Franzen, van der Graaf, Eliasson, Visseren, Paynter and Dorresteijn2022; Gmel, Reference Gmel2001; Zhou et al., Reference Zhou, Eckert and Tierney2001).
Our final dataset included a total of N = 1146 participants (which was similar to the number of initially eligible patients). Finally, note that we did not run any complete cases analysis.
Analysis
We divided our analysis into two different steps. First, we used various machine learning algorithms to predict referrals to PSR interventions based on socio-demographic and clinical predictors: ridge regression regularization (Friedman et al., Reference Friedman, Hastie and Tibshirani2010), multinomial regression, recursive partitioning trees (Breiman et al., Reference Breiman, Friedman, Olshen and Stone1984), random forest (Breiman, Reference Breiman2001) and extreme gradient boosting (Chen and Guestrin, Reference Chen and Guestrin2016). We used a two-step 20-fold cross-validation procedure, also called nested or double cross-validation (Stone, Reference Stone1974), which resulted in two sets of performance evaluation: (1) an ‘external’ or outer cross-validation performance over 20 testing folds and (2) a range of 20 ‘internal’ or inner cross-validation performance. Predictive accuracy was assessed based on the micro-averaged area under the receiver operating curve (AUROC) and the area under the precision-recall curve (AUPRC). Details related to data preprocessing, grid search for machine learning hyperparameters, cross-validation strategy and measures of predictive accuracy are reported in the supplement (supplementary methods, Supplementary Table S2).
Second, we used explainable machine learning methods from the best performing algorithm to compute the explanatory power of each socio-demographic and clinical feature. To do so, we used the artificial intelligence-based SHAP (SHapley Additive exPlanations) method, regarded as the only model-agnostic explanation method with a solid theoretical foundation (Lundberg and Lee, Reference Lundberg and Lee2017) (supplementary methods). For each variable and each observation, a higher SHAP value corresponds to a higher likelihood of the target outcome (i.e. being referred to one of the four PSR treatment programmes). We first provided predictor-level SHAP absolute values aggregated at the level of the population in order to rank the predictors’ overall predictive ability (aka variable importance). Second, for each treatment programme, we provided one-way dependence plots showing the association between the raw values of the 10 most important predictors and their SHAP values.
To perform this analysis, we used R version 4.1 and packages such as caret, multiROC and fastshap. The Prediction model Risk Of Bias ASsessment Tool (PROBAST) guided writing of the manuscript (Wolff et al., Reference Wolff, Moons, Riley, Whiting, Westwood, Collins, Reitsma, Kleijnen and Mallett2019).
Results
The mean age of the overall cohort was 33 years (range, 16–72 years), and 449 (39%) were women. Of those, 318 (27.8%) were referred to CBT, 203 (17.7%) to CR, 236 (20.6%) to PE and 388 (33.9%) to VT. A complete description of the dataset is provided in Table 1.
Performance of machine learning models
The highest overall micro-cross-validated AUROC and AUPRC were obtained for the random forest model (AUROC: external validation, 0.672; internal validation across 20 folds: range, 0.659–0.674; PR-AUC: external validation, 0.407; internal validation across 20 folds: range, 0.381–0.410; Supplementary Figure S1). Random forest outperformed regression regularization (AUROC, external validation: 0.668); multinomial regression (0.662); extreme gradient boosting (0.653) and recursive partitioning trees (0.638) (Supplementary Tables S3 and S4).
Contribution of each predictor to initial referrals
We used the artificial intelligence-based SHAP method to further explore the explanatory power of socio-demographic and clinical variables. Aggregating the four programmes, the 10 most important contributors to the prediction on a global scale were as follows: having a diagnosis of schizophrenia; having a secondary psychiatric diagnosis; education; having a physical health diagnosis; unemployment; being a disabled worked beneficiary; having a diagnosis of personality disorder; score on the clinical global impression (CGI) scale; missing information on insight and sex (Supplementary Figure S2).
We then examined associations between the predictors and initial referrals to PSR interventions using one-way SHAP dependence plots. A higher likelihood of being referred to CBT was associated with not having a diagnosis of schizophrenia; having a relatively high level of education; having a secondary psychiatric diagnosis; not having a physical health diagnosis; not being unemployed; having a diagnosis of personality disorder; not having been referred by a clinician from the public system and having a relatively low self-esteem. Missing information on duration of illness and missing information on self-stigma were also associated with being referred to CBT (Fig. 1).
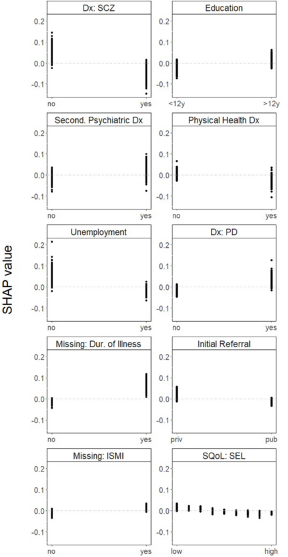
Figure 1. One-way SHAP dependence plot of the 10 most important predictors of referrals to CBT.
A higher likelihood of being referred to CR was associated with having a diagnosis of schizophrenia; not having a secondary psychiatric diagnosis; having a relatively low level of education; being a disabled worker beneficiary; having an illness duration of 5–10 years; not having an addiction diagnosis; being female; not having a diagnosis of personality disorder and not having a physical health diagnosis. Missing information on quality of life was also associated with being referred to CR (Fig. 2).
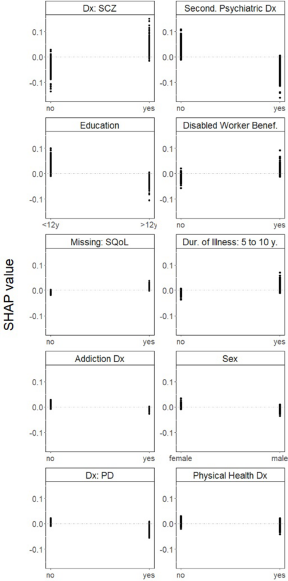
Figure 2. One-way SHAP dependence plot of the 10 most important predictors of referrals to CR.
A higher likelihood of being referred to PE was associated with having a primary diagnosis of schizophrenia; not having a secondary psychiatric diagnosis; having a relatively high level of education; not having a physical health diagnosis; not being a disabled worker beneficiary; having a primary diagnosis of autism spectrum disorder; not having a primary diagnosis of personality disorder and being male. Missing information on insight was associated with not being referred to PE, while missing information on CGI was associated with being referred to PE (Fig. 3).
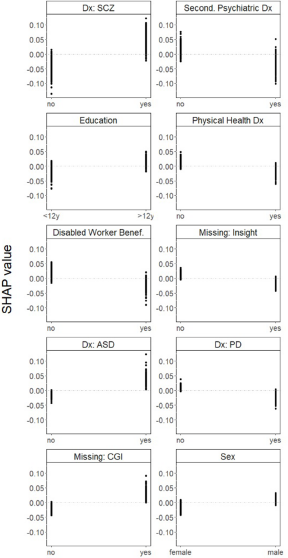
Figure 3. One-way SHAP dependence plot of the 10 most important predictors of referrals to PE.
Finally, a higher likelihood of being referred to VT was associated with having a secondary psychiatric diagnosis; not having a primary diagnosis of schizophrenia; having a physical health diagnosis; being unemployed; having a relatively low level of education; having a low score on the CGI scale; not having a primary diagnosis of bipolar disorder; having an addiction disorder and having been initially referred by a clinician from the public system. Missing information on insight was also associated with being referred to VT (Fig. 4).
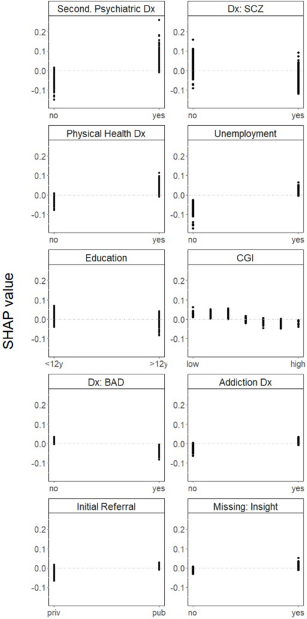
Figure 4. One-way SHAP dependence plot of the 10 most important predictors of referrals to VT.
Discussion
The current study used a large number of socio-demographic and clinical variables to predict referrals to four PSR treatment programmes in patients with serious mental illness among a network of 15 rehabilitation centres. We first show that the predictive accuracy of a random forest algorithm outperformed that of recursive partitioning trees, extended gradient boosting, and to a lesser extent, multinomial regression and regularized regression. Overall, the performance of our winning random forest algorithm (AUROC of 0.67) may be considered as either moderate, fair or acceptable or even poor, weak or low (de Hond et al., Reference de Hond, Steyerberg and van Calster2022). It is unlikely that such uncertainty indicates a poor model or lack of access to important predictors, given that we used several machine learning algorithms and that we had access to an overall large number of predictors. A more reliable hypothesis may be that available socio-demographic and clinical features are necessary but not sufficient to explain initial decisions to refer patients to PSR programmes. Referrals might also rely on other important factors in the dynamics of clinical decisions, for instance, centre characteristics (such as being a teaching hospital, having its own culture regarding treatment referral, financial resources, etc.), clinicians heuristics, provider bias (e.g. implicit or explicit bias) and patients’ preferences (Blankertz and Robinson, Reference Blankertz and Robinson1996; Carter et al., Reference Carter, Crowe, Jordan, McIntosh, Frampton and Joyce2015; Medalia and Richardson, Reference Medalia and Richardson2005). Another possibility is that lack of resources for certain treatment programmes may lead to patients being referred to interventions that would not have been chosen as a first instance. An interesting goal for future research would be to tease out the contribution of the above-mentioned factors to predict referrals to PSR interventions.
The moderate contribution of socio-demographic and clinical characteristics to predict referrals to PSR interventions is enlightened by the implementation science literature, which studies ‘methods to promote the systematic uptake of […] evidence-based practice into routine practice’ (Eccles and Mittman, Reference Eccles and Mittman2006). Within this framework, some of the non-patient variables that we mentioned above may be understood as barriers to implementing psychosocial interventions. Psychosocial and recovery interventions are indeed relatively difficult to implement, due to being too complex and time-consuming or requiring significant material, financial and human resources (van der Krieke et al., Reference van der Krieke, Bird, Leamy, Bacon, Dunn, Pesola, Janosik, Le Boutillier, Williams and Slade2015). Interestingly, blockers of PSR interventions may themselves be moderated by patients’ socio-demographic and clinical characteristics. For instance, compared to those with depressive or anxiety disorders, patients with schizophrenia may not be referred to CBT because of the beliefs that therapy may be difficult and cumbersome, especially when considering lack of resources or training, and the fact that symptoms may impede engagement (Hazell et al., Reference Hazell, Strauss, Cavanagh and Hayward2017; Ince et al., Reference Ince, Haddock and Tai2016; Prytys et al., Reference Prytys, Garety, Jolley, Onwumere and Craig2011).
Despite its moderate performance, our winning random forest model likely provided the best possible predictive accuracy with respect to the data at hand. This was further exploited using artificial intelligence-based SHAP values (Lundberg and Lee, Reference Lundberg and Lee2017) to decipher the contribution of socio-demographic and clinical factors to predict initial referrals to PSR interventions. Given the paucity of evidence regarding how patients’ needs and characteristics guide referrals, any major disparities would reflect informal, ‘eminence-based’ or circumstantial practices that would rely mostly on our clinicians’ expert opinions. Incidentally, these choices may also reflect unmet patient needs, where some categories of patients may benefit from suboptimal treatment programmes. We wish to encourage clinicians and researchers to further investigate these circumstantial practices (described below), for instance, by determining whether they are ethical and optimal from a health services perspective. Finally, because our findings may reflect unmet needs rather than good practice per se, we can only, at this point, recommend to further explore these aspects, rather than directly use our findings in clinical practice.
A first disparity may be related to psychiatric diagnosis and comorbidities. While all treatment programmes tested in the current study have been recommended in patients with schizophrenia (Crowther et al., Reference Crowther, Marshall, Bond and Huxley2001; Morin and Franck, Reference Morin and Franck2017; Twamley et al., Reference Twamley, Jeste and Lehman2003; Watzke et al., Reference Watzke, Galvao and Brieger2009), PE and CR were more likely to be chosen for patients with psychotic disorders than CBT and VT. Patients with psychosis may be seen as having higher needs for (and higher gains from) PE (de Barros Pellegrinelli et al., Reference de Barros Pellegrinelli, de O. Costa, Silval, Dias, Roso, Bandeira, Colom and Moreno2013) and CR (Li et al., Reference Li, Zhou, Zhang, Ng, Ungvari, Li and Xiang2020), due to their well-documented lack of insight (Braw et al., Reference Braw, Sitman, Sela, Erez, Bloch and Levkovitz2012; Lysaker et al., Reference Lysaker, Pattison, Leonhardt, Phelps and Vohs2018; Ramachandran et al., Reference Ramachandran, Ramanathan, Praharaj, Kanradi and Sharma2016) and cognitive difficulties (Zaytseva et al., Reference Zaytseva, Fajnerová, Dvořáček, Bourama, Stamou, Šulcová, Motýl, Horáček, Rodriguez and Španiel2018), respectively. Clinicians also preferred to refer patients without comorbidities to the former programmes, perhaps in the view of facilitating treatment delivery (Gold et al., Reference Gold, Köhler-Forsberg, Moss-Morris, Mehnert, Miranda, Bullinger, Steptoe, Whooley and Otte2020; Thornicroft et al., Reference Thornicroft, Ahuja, Barber, Chisholm, Collins, Docrat, Fairall, Lempp, Niaz, Ngo, Patel, Petersen, Prince, Semrau, Unützer, Yueqin and Zhang2019). This was in sharp contrast with referrals to VT, where comorbidities, whether from addictions, mental or physical health, would not a priori play a role in treatment completion.
We also report disparities relative to clinical characteristics. For instance, the fact that patients referred to VT were more likely to have a lower severity score on the CGI scale may reflect the need to satisfy the demands of occupational activities or supported work. By contrast, current clinical and psychological issues (in particular low self-esteem) would increase the likelihood of referrals to CBT (Carter et al., Reference Carter, Crowe, Jordan, McIntosh, Frampton and Joyce2015; Keeley et al., Reference Keeley, Storch, Merlo and Geffken2008; Maddox et al., Reference Maddox, Crabbe, Fishman, Beidas, Brookman-Frazee, Miller, Nicolaidis and Mandell2019), which primarily aims to address current negative thoughts about self and others.
Functioning and educational attainment were other sources of disparities. For instance, clinicians tend to refrain from referring patients with social dysfunctions (e.g. unemployment and being a disabled worker beneficiary) and lack of education to CBT and PE, as they may judge that these treatment programmes may require a certain level of literacy and understanding (Hu et al., Reference Hu, Xu and Mao2022). By contrast, unemployed patients were preferentially referred to VT (which provides direct occupational support), and patients who are disabled worker beneficiaries were more likely to be referred to CR (which would tackle cognitive dysfunction in relation to the disability). Likewise, the fact that a relatively low level of education was associated with referrals to CR may be a reflection that these treatment programmes aim at palliating for cognitive and social deficits (Akshaya et al., Reference Akshaya, Gopal, Pavithra and Raghavan2022) that are known to be strongly associated with lack of educational attainment (Dalsgaard et al., Reference Dalsgaard, McGrath, Østergaard, Wray, Pedersen, Mortensen and Petersen2020; Guerra-Carrillo et al., Reference Guerra-Carrillo, Katovich and Bunge2017; Lövdén et al., Reference Lövdén, Fratiglioni, Glymour, Lindenberger and Tucker-Drob2020).
Limitations
First, our results are subject to some degree of uncertainty due to the substantial amount of missing values, especially for clinical features (e.g. clinical severity, insight, quality of life, well-being and self-stigma). Missing information, however, was a significant contributor to initial referrals, where, for instance, PE was not the treatment of choice when insight – the key process addressed by this intervention – had not been evaluated.
Second, one might question the generalizability of our findings. Indeed, delivering some of the interventions analysed in the present study in low-resource countries may be unfeasible. Likewise, some of our predictors were directly linked to the French universal healthcare system and social security (e.g. being a disabled worker beneficiary), and future studies should test whether these criteria are meaningful to predict referrals to PSR programmes in other countries. Finally, our analysis may only be applicable to patients that consent to have their data recorded in our database. As we do not record patients who refuse to participate, we have no possible way of investigating whether this may have been a potential problem for our analysis. Informal discussions with mental health clinicians, however, indicate that patients who do not consent to have their data collected are rare.
Third, variable importance measures should not be interpreted as absolute indicators of the contribution of individual variables. Machine learning algorithms, such as random forest, involve non-linear transformations and interactions between variables; therefore, each variable importance measure is in essence relative to other variables.
Fourth, in theory, an analysis taking into account multiple referrals rather than initial referrals only would have been both more representative of referrals as they happen in real life and more statistically informative (i.e. with greater statistical power). Further, such an analysis would enable the investigation of socio-demographic and clinical factors that contribute to greater resource utilization. Such an analysis, however, would have been both more complex (taking into account censored data) and uncertain (due to the amount of missing observations for clinical variables after the initial referral).
Fifth, this study focused on treatment referral rather than treatment effectiveness. Choices made by experts from our network may not necessarily be associated with positive outcomes. As mentioned above, it is not impossible that they reflect, at least in part, service- or clinician-level factors, rather than evidence-based patient-level criteria. In that sense, future studies should investigate whether features that contribute to treatment referrals are also predictive of positive treatment outcomes.
Conclusion
Using a variety of machine learning models, we demonstrated that a combination of socio-demographic and clinical features was not sufficient to accurately predict initial referrals to four PSR programmes among a French network of rehabilitation centres. In addition, the explanations generated by SHAP plots provided valuable insights into the sources of referrals for our cohort of patients and, in particular, disparities in referrals with respect to diagnoses, current clinical and psychological issues, functioning and education. This, in turn, may provide potential avenues for future research aiming at investigating and resolving such disparities.
Supplementary material
The supplementary material for this article can be found at https://doi.org/10.1017/S2045796024000015.
Availability of data and materials
The datasets generated during and/or analysed during the current study are available upon reasonable request to the corresponding author, GB.
Acknowledgements
Informed consent was obtained from all individual participants included in the study.
Author contributions
Dr Barbalat had full access to all of the data in the study and takes responsibility for the integrity of the data and the accuracy of the data analysis.
- Concept and design: Barbalat and Franck.
- Responsibility for data collection: Haesebaert, Chéreau-Boudet, Gouache, Legros-Lafarge, Massoubre and Guillard-Bouhet.
- Extraction, analysis or interpretation of data: Barbalat and Plasse.
- Drafting of the manuscript: Barbalat and Franck.
- Critical revision of the manuscript for important intellectual content: Barbalat, Haesebaert and Franck.
- Statistical analysis: Barbalat and Plasse.
- Obtained funding: Franck.
- Administrative, technical or material support: Plasse.
- Supervision: Franck.
Ethical standards
All procedures performed in studies involving human participants were in accordance with the ethical standards of the institutional and/or national research committee and with the 1964 Helsinki Declaration and its later amendments or comparable ethical standards. The database obtained the authorizations required under French legislation (French National 429 Advisory Committee for the Treatment of Information in Health Research, 16.060bis; French 430 National Computing and Freedom Committee, DR-2017-268).
Competing interests
The authors declare no competing interests.