What forms does political participation take among disparate classes of society? Why do individuals overcome collective action problems? How do people’s identities, engagements, and affiliations affect their preferences? The modern development of academic survey research has enabled empirical assessments of these and other questions about political behavior that are central to the study of politics (Heath, Fisher, and Smith Reference Heath, Fisher and Smith2005). To gauge preferences, traits, or behaviors, survey researchers define a target population and then obtain a sampling frame—a list that is, ideally, accessible and comprehensive so as to represent the population. Randomly selecting elements from this frame means that each individual has a known, nonzero, and equal probability of inclusion in the sample. In turn, sound inferences can be made about the population with estimates of uncertainty; statistical theory allows us to confidently say something intelligible about a population without surveying every one of its members.
Yet political scientists are often interested in people who are not easily subjected to survey sampling. Violent extremists, first movers of protests, and undocumented migrants, for instance, are populations unlikely to be found on lists. Like right- and left-wing activists, members of nonstate armed groups, the super-rich, and refugees fleeing conflict, they are “hard to survey”: they are difficult to sample, identify, reach, persuade, or interview (Tourangeau Reference Tourangeau, Tourangeau, Edwards, Johnson, Wolter and Bates2014). Probabilistic approaches to sampling such populations are sometimes possible, but can often be infeasible, unethical, or unrepresentative. Nonprobabilistic surveys are used as well, but they present bias and limit generalizability.
I posit another approach, one that is designed for difficult populations and that approximates probability sampling—but that has been almost entirely absent from the toolbox of political research methods. Respondent-driven sampling (RDS) is primarily used in epidemiological studies of populations at high risk for HIV. Yet it can be deployed among any number of networked populations and is particularly well suited to hard-to-survey ones. RDS leverages relations of trust that are critical among hidden populations, increases representativeness, systematizes the sampling process, and supports external validity.
RDS procedures resemble or use tools that are common to political science, such as snowball sampling and statistical weights. For sampling, an RDS survey begins with a handful of nonrandomly selected participants from a population, or seeds. Seeds recruit a set number of peers, and the process repeats in waves, creating chains of participants linked to their recruiter and recruits by non-identifying IDs. The use of RDS extends to analysis: over the course of the survey, RDS collects information from respondents about the size of their personal networks, which is used as a weight to balance elements with unequal inclusion probabilities. This enables RDS to achieve its primary advantage: population inferences with estimates of uncertainty.
In the sections that follow, I consider the challenges posed by the study of hard-to-survey populations and the common probabilistic and nonprobabilistic approaches that political scientists take to resolve them. I review non-HIV-related applications of RDS and find that RDS is largely unknown or misused despite the range of populations on which it could shed light. I contend that the method is worthy of inclusion in the toolbox of political scientists and introduce its benefits, sampling and analysis procedures, and trade-offs. Given RDS’s strong assumptions and our own disciplinary standards, I suggest that it is best used within an integrative multimethod design that can bolster RDS with conceptualization, network mapping, navigating sensitivity, understanding respondent behavior, and inference. I illustrate its use through my study of activist refugees from Syria, one of the first properly administered political science applications of RDS. A brief presentation of my findings demonstrates that RDS can provide a systematic and representative account of a vulnerable population acting to effect change in the course of a brutal conflict.
Approaches to Sampling Hard-to-Survey Populations
Some of the primary questions that political scientists ask are related to political ideas or behavior. Often, however, they ask such questions of populations that challenge standard techniques for sampling and therefore surveying. Tourangeau classifies hard-to-survey populations as being one or more of the following: “hard to sample” if they cannot be found on a population list that can serve as a sampling frame or if they are rare in the general population, “hard to identify” or “hidden” if their behavior is risky or sensitive, “hard to reach” if they are mobile and difficult to contact, “hard to persuade” because they are unwilling to engage, or “hard to interview” because they lack the ability to participate (Tourangeau Reference Tourangeau, Tourangeau, Edwards, Johnson, Wolter and Bates2014). Some populations, like undocumented migrants, present multiple such challenges. They may be hard to sample because they are not documented on population lists that could constitute sampling frames; hard to identify because the visibility of their status could pose risks to their well-being; and hard to reach because, as a mobile population, they lack permanent or formal contact information. In conflict-afflicted settings, entire populations may be hard to survey because of generalized challenges of access and vulnerability (Cohen and Arieli Reference Cohen and Arieli2011; Firchow and Mac Ginty Reference Firchow and Mac Ginty2017).
Some social scientists have suggested that quantitative research in difficult settings is unreliable or even impossible because of poor data quality, political sensitivity in authoritarian or conflict-afflicted areas, and the imperative of building trust among hard-to-reach populations (Bayard de Volo and Schatz Reference Bayard de Volo and Schatz2004; Morgenbesser and Weiss Reference Morgenbesser and Weiss2018; Romano Reference Romano2006). When research does not rely on generalizability—as may be the case with process tracing and discourse analysis—qualitative methods are indeed preferable (cf. Tansey Reference Tansey2007). But if we seek to make claims about a population or analyze individual-level data, can we forgo surveys on such pressing issues as migration, conflict, and contention?
Those who wish to make generalizing claims can potentially manage these challenges using probability-based strategies that, because of the properties of random sampling, allow for population estimates. Doing so requires a sampling frame from which to randomly select potential respondents. If a single representative list is unavailable, it may be feasible to supplement it with one or more incomplete lists. If no list is available, researchers might create an original frame, perhaps using societal informants to do so. Finally, researchers can intercept the target population at a place or event (a sort of embodied list), randomly selecting respondents from among the attendees. The application of these probabilistic approaches depends on the circumstances and nature of the target population, and each, if it can be implemented, has its advantages such as coverage and efficiency, as demonstrated in table 1. Yet they can fall short when it comes to many hard-to-survey populations, for whom incomplete lists may not exist, whose exposure through the creation of lists may pose risks, and whose participation in highly visible forms is unlikely.
Table 1 Probabilistic and Nonprobabilistic Approaches to Sampling Hard-to-Survey (H2S) Populations
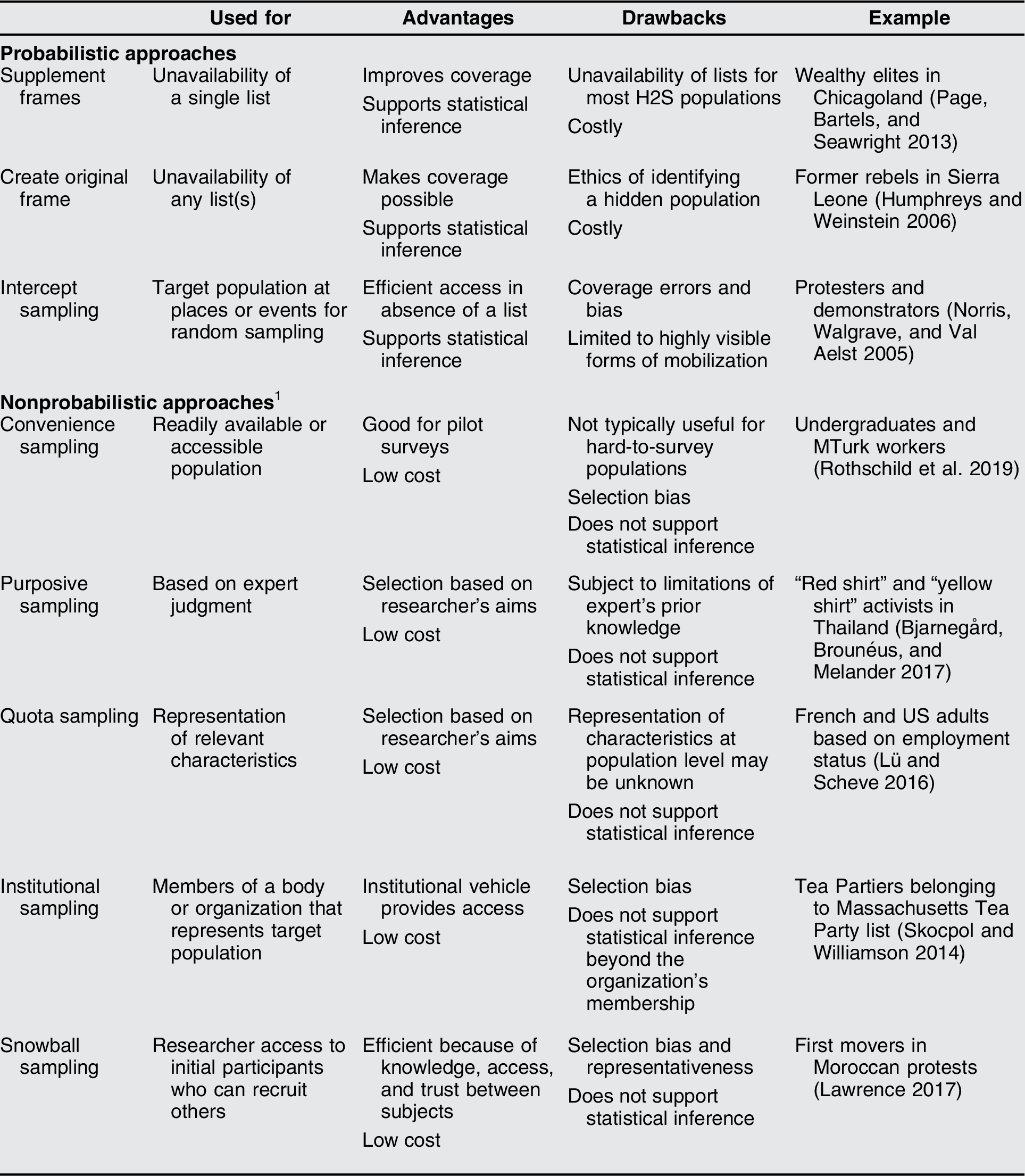
1 Nonprobability examples are of quantitative survey research only. Qualitative uses of these tools should be judged separately, because methods such as process tracing may be characterized by a distinct logic of inference.
Others rely on nonprobabilistic approaches that create study samples by means other than randomization. Analysts cannot statistically estimate the uncertainty of such data; instead, they subjectively evaluate statements about the population (Kalton Reference Kalton1983). Researchers nevertheless frequently deploy nonprobabilistic methods to reach both common and hard-to-survey populations. Convenience, purposive, quota, and institutional sampling are all nonprobabilistic sampling strategies, each of which has advantages (e.g., relatively low monetary costs), but also limitations, primarily selection bias, as seen in table 1.
A ubiquitous nonprobability sampling method is snowball sampling, wherein a researcher identifies one or more members of the population and then relies on them to identify others in the population to participate, proceeding through chains of participant referrals.Footnote 1 Snowball sampling is primarily deployed in interview and field research, in which access to a network on the basis of trustful relations is often a must. Snowball sampling is sometimes used for quantitative survey research as well. The method has distinct advantages for the study of hard-to-survey populations. Snowballing leverages relations of trust between subjects who have greater knowledge about, access to, and influence over their own community (Atkinson and Flint Reference Atkinson and Flint2001; Cohen and Arieli Reference Cohen and Arieli2011). These advantages can efficiently lead an outside researcher to a large pool of otherwise guarded subjects. For example, in a survey that intended to deploy probability sampling to reach foreign nationals in South Africa, potential respondents feared identifying themselves to the study team’s formal organizational partner; the team ultimately relied on the foreign nationals themselves to snowball them to their desired sample size (Misago and Landau 2013).
Researchers using snowball sampling make important contributions to the study of elusive populations. However, in addition to the fact that statistical inferences cannot be made to the population, the method tends to favor individuals with large networks, resulting in selection bias and a lack of representativeness that can extend through multiple waves of one prosocial individual’s acquaintances (Griffiths et al. Reference Griffiths, Gossop, Powis and Strang1993; Kaplan, Korf, and Sterk Reference Kaplan, Korf and Sterk1987; van Meter Reference Van Meter1990). Yet snowballing’s advantages to political scientists studying difficult populations in challenging contexts are so significant that its use “may make the difference between research conducted under constrained circumstances and research not conducted at all” (Cohen and Arieli Reference Cohen and Arieli2011, 433).
Respondent-Driven Sampling in the Social Sciences
I contend that there is another sampling option. RDS shares the chain-referral qualities of snowball sampling that are ideal for the study of hidden and hard-to-survey populations. Unlike snowball and other nonprobabilistic sampling strategies, however, RDS approximates a probability sample to generate population estimates. The driving force of RDS has been the epidemiological study of populations at high risk for HIV infection: men who have sex with men, female sex workers, and injection drug users. Developed by Douglas Heckathorn (Reference Heckathorn1997), RDS has been used in hundreds of surveys on HIV-risk populations conducted with the support of numerous intergovernmental and national health agencies (see table 2).Footnote 2
Table 2 Respondent-Driven Sampling for Hard-to-Survey (H2S) Populations
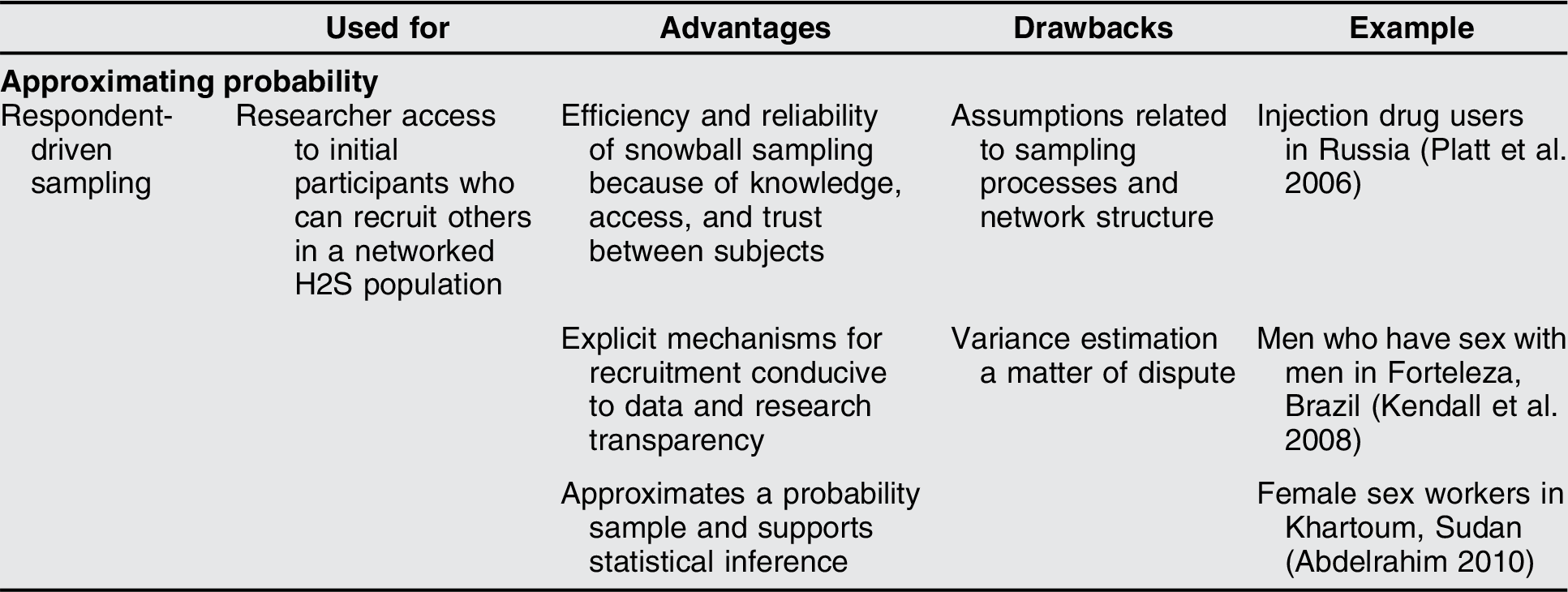
To explore the potential for RDS in political science, I began by asking if and how it has been used outside of HIV research. I conducted a systematic review of non-HIV-risk peer-reviewed social science publications that reported using RDS.Footnote 3 Twenty-seven studies qualified for inclusion in the review.Footnote 4
My impressions before conducting the review prompted me to explore whether scholars were actually deploying RDS when they said they were. Although all of them described RDS as their method, it emerged that only 10 of the 27 studies actually implemented RDS sampling (nonrandom seeds, participant recruitment, and participant linkage through IDs) and analysis (degree-based weights, population estimates) procedures. Among the majority that did not fully implement RDS were some that did not follow its protocols at all, and others used some or all of the sampling techniques but none for analysis. I suspect the method is used piecemeal because of the appeal of its systematic sampling procedures. But when methods are inappropriately labeled, the benefit of RDS’s sampling transparency is overshadowed by confusion.
RDS’s under- and misusage in the social sciences aside, the studies serve to illustrate the types of populations that RDS can capture, as shown in table 3. Some are explicitly defined by political behavior, including returned French colonial settlers, ex-combatants in Liberia, protesters in China, and Vietnam War resisters in Canada. Almost all of them, including migrants and refugees, would be of relevance to the study of politics. RDS, it seems, is an untapped resource for empirical political scientists.
Table 3 Non-HIV-Risk RDS in the Social Sciences
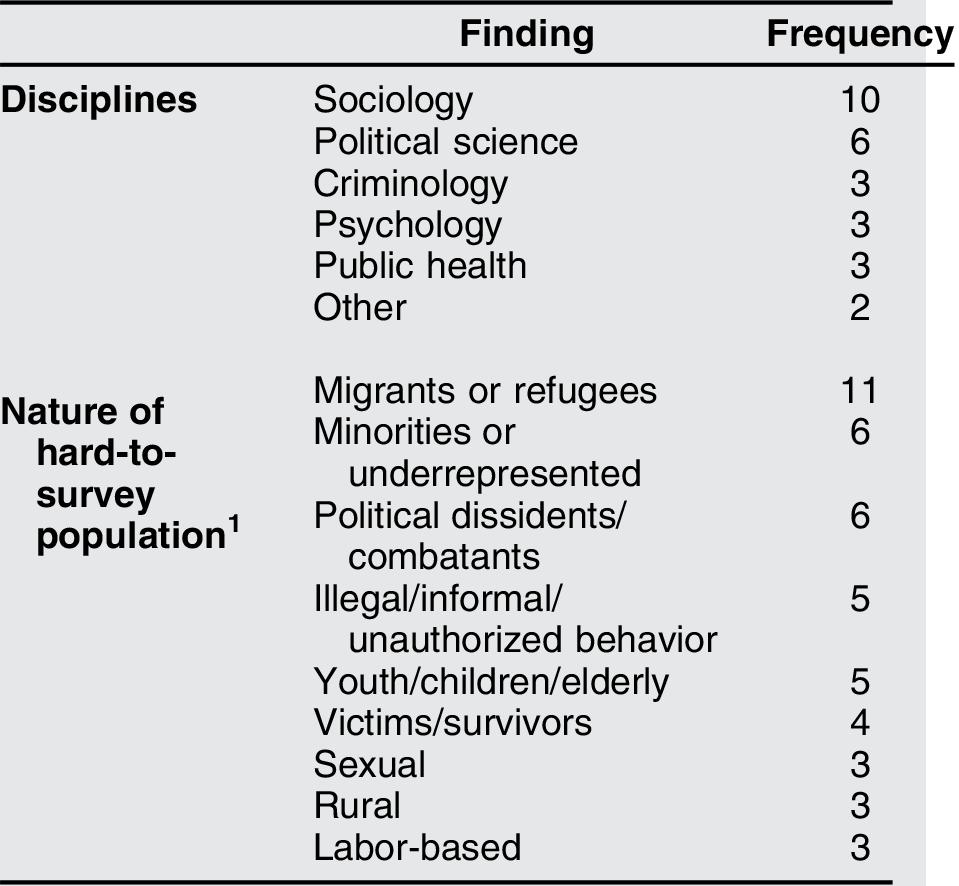
1 Each study included one population, but several are classifiable across categories
RDS can help researchers reach a large and representative group of hard-to-survey people confidentially. Like snowball sampling, RDS reaches people who are hard for an outsider to reach or persuade, via the delegation of recruitment to members of the target population who may be encouraged to participate when referred by a trusted member of their network. This trust can be maintained, because participation remains anonymous or confidential depending on the survey protocol (although a participant’s recruits can assume her participation). Further, this process can introduce a diverse set of respondents and, when balanced with statistical weights, maintain representation for those who are relatively less networked.
Additionally, RDS meets standards for data and research transparency that are increasingly emphasized in the discipline (cf. Organized Section in Comparative Politics 2016). RDS systematizes sampling designs because recruitment of survey participants proceeds through explicit mechanisms. Researchers regulate the number of recruits, systematize modes and instructions for recruitment, and link participants with sequential or random numbers that do not compromise anonymity. RDS has become standardized as it has gained the support of major institutions including the World Health Organization and the US Centers for Disease Control and Prevention. Therefore, RDS studies can achieve production transparency, “a full account of the procedures used to collect and generate data” (APSA Committee on Professional Ethics, Rights, and Freedoms 2012, 10).
Finally, and critically, the systematic collection of data about respondents’ networks allows inferences to be made about the population with estimates of uncertainty. This key quality of RDS endows a study with a reasonable degree of generalizability.
Mechanics and Trade-Offs of Respondent-Driven Sampling
Sampling and Analysis
Similar to snowball sampling, RDS is a chain-referral sampling method that moves through networks of individuals defined by relevant characteristics and eligibility criteria of the target population. Sampling begins with the selection of seeds, members of the target population whom the researcher nonrandomly selects to be the initial survey participants. Seeds then recruit a set number of their peers, usually up to three, to participate. The number is limited so as not to overrepresent individuals with large personal networks. Seeds recruit by passing information about the survey and participation, along with a unique identification number that links recruiter to recruits, creating recruitment chains. This process repeats in waves within each recruitment chain, ideally until the desired sample size is reached.
As sampling proceeds, the survey collects information from participants about the number of their own connections within the larger target population, known as their degree.Footnote 5 Eliciting accurate degree reports demands attention to three points. First, network contacts are defined by the characteristics of the target population and should meet eligibility criteria for survey participation. Second, relationships between recruits should be reciprocal: the degree reflects how many people in the target population the respondent knows, who know the respondent in turn. Third, a temporal frame for personal contact is included in the degree question(s) to further home in on an accurate degree size and ensure reciprocity of relations (Wejnert Reference Wejnert2009). Thus, a degree question generally asks (1) how many people in the target population (e.g., people characterized by a living in area b), (2) do you know (e.g., you know their name and they know yours), (3) whom you have been in contact with in the last c period of time?Footnote 6 Successive questions and probing are used to elicit accurate responses.Footnote 7
In the analysis, the degree is used to create a weight for each element as an estimate of its inverse inclusion probability. The logic of the degree-based inverse inclusion probability is that someone with a relatively large personal network has a high probability of coming into the sample, so is assigned a lower weight in the final analysis; conversely, someone with a relatively small degree is less likely to have been recruited and so is assigned more weight. In this way, the biases of snowball sampling are corrected, and population proportions can be estimated with uncertainty.
Performance and Assumptions
RDS is considered to perform well in accessing hidden populations and creating diverse and representative samples (Abdul-Quader et al. Reference Abdul-Quader, Heckathorn, McKnight, Bramson, Nemeth, Sabin, Gallagher and Des Jarlais2006; Kendall et al. Reference Kendall, Kerr, Gondim, Werneck, Macena, Pontes, Johnston, Sabin and McFarland2008; Salganik and Heckathorn Reference Salganik and Heckathorn2004; Wejnert and Heckathorn Reference Wejnert and Heckathorn2008),Footnote 8 especially in comparison with nonprobabilistic methods. Estimates produced by RDS have been found to be unbiased (Barash et al. Reference Barash, Cameron, Spiller and Heckathorn2016; Salganik and Heckathorn Reference Salganik and Heckathorn2004). But its reliability in variance estimation remains an “open question” (Heckathorn and Cameron Reference Heckathorn and Cameron2017); likely, confidence intervals are too narrow (Baraff, McCormick, and Raftery Reference Baraff, McCormick and Raftery2016; Goel and Salganik Reference Goel and Salganik2010). An additional challenge presented by the method is the absence of data on hidden populations against which estimates might be evaluated; evaluations are often based on simulated data or on data from non-hidden populations.
Unlike simple random sampling, RDS makes a number of assumptions related to sampling processes and network structure (Wejnert Reference Wejnert2009):
1. Ties between respondents are reciprocated; that is, individuals know their recruits, who know them in turn.
2. The overall network is a single component, and each respondent can be reached by any other through a series of network ties.
3. Sampling is with replacement.
4. Respondents can accurately report their personal network size, or degree.
5. Peer referral is random from among the recruiter’s peers.
All of these assumptions can be difficult to meet. For example, with regard to assumption 5, it is reasonable to think that respondents are more likely to recruit those peers to whom they are close, with whom they have most recently been in contact, or who they think are most likely to participate, rather than stochastically. Methodologists take three approaches to RDS assumptions. First, they promote preemptive study protocols; for instance, encouraging questionnaires that use language more likely to ensure reciprocity of ties and accurate degree reports (Gile, Johnston, and Salganik Reference Gile, Johnston and Salganik2015; WHO 2013).Footnote 9 Second, they develop and hone both model- and design-based estimators that account for, or in some cases, eliminate assumptions (Gile Reference Gile2011; Shi, Cameron, and Heckathorn Reference Shi, Cameron and Heckathorn2016; Volz and Heckathorn Reference Volz and Heckathorn2008). Third, they address the consequences of assumptions not being met, often finding that moderate violation of assumptions does not bias estimates (Aronow and Crawford Reference Aronow and Crawford2015; Barash et al. Reference Barash, Cameron, Spiller and Heckathorn2016), but also determining that some violations can indeed undermine the method (Gile and Handcock Reference Gile and Handcock2010; Shi, Cameron, and Heckathorn Reference Shi, Cameron and Heckathorn2016). Next I demonstrate how qualitative tools can also be used to address assumptions.
A Multimethod Approach to RDS
RDS holds promise for political scientists, but its shortcomings demand a cautious approach for a discipline that challenges its practitioners to demonstrate descriptive and causal inference. I propose that using RDS as part of a multimethod research design can mitigate its limitations and accommodate it to political studies.
Integrative multimethod research designs, according to Seawright (Reference Seawright2016), support a single inference by using distinct methods in the service of designing, testing, refining, or bolstering each other. Following this multimethod logic, I propose that qualitative methods can be used to support an RDS survey through concept formation, network mapping, navigating sensitivity, understanding respondent behavior, and evaluating causality. In turn, an RDS survey can support a larger research design by furnishing descriptive evidence that is otherwise elusive.
Conceptualization
RDS practitioners conduct “formative research” before launching a survey, often in partnership with local organizations, with the aims of ensuring the target population is networked, assessing feasibility and logistics, and selecting seeds. For political scientists, conceptualization of the target population and its defining political behavior is likely necessary before formative research because of the hiddenness that characterizes the subject. To advance their field utility and resonance among a survey population,Footnote 10 concepts can be approached empirically. Specifically, immersive field research can reveal “actually observed behaviors, insider understandings, and self-reported identities” (Singer Reference Singer, Schensul, LeCompte, Trotter, Cromley and Singer1999, 172). Additionally, interview research can allow one to reconsider or define anew notions of group identity and political behavior among underrepresented populations (Rogers Reference Rogers and Mosley2013). The resulting concept should be operationalizable with indicators (Adcock and Collier Reference Adcock and Collier2001; Goertz Reference Goertz2005), which can serve as eligibility criteria for inclusion in the survey. The overall effort of conceptualization allows our findings and inferences to be understood and assessed.
Mapping the Network
At least two of RDS’s assumptions rely on knowledge of a hard-to-survey population that is, by definition, difficult to glean. Assumption 2 posits that the network of a target population should be interconnected enough to become independent of the seeds, which is possible if there are not subgroups within it that will induce homophily by recruiting only within their subgroup. Homophily can be statistically diagnosed from survey data, but its prevalence is worth gauging in advance in order to judge RDS’s feasibility. Assumption 3 posits that sampling is with replacement. Yet in practice, participants should be surveyed only once; therefore, it is advised to maintain a sampling fraction that is small relative to the overall size of the target population (Barash et al. Reference Barash, Cameron, Spiller and Heckathorn2016).Footnote 11 Yet knowledge of the size of a hidden population is usually, at best, based on the estimates of key informants, elite sources, and records.Footnote 12 Such sources may not proffer accurate accounts of populations engaged in grassroots or illicit processes or may have interests in misstating the size of a population or the nature of its preferences and behavior (Wood Reference Wood2003). As such, these accounts should be supplemented by the researcher’s own efforts.
To do so, one can begin by translating these RDS assumptions into concepts from ethnographic network mapping: assumption 2 relates to bridges, or the interpersonal connections, bonds, and activities common to people in a group; assumption 3 relates to boundaries, or the bases of inclusion in and exclusion from a network. Interview research and close observation within a community can allow a researcher to grasp the bridges and boundaries that constitute a network, its subgroups and interconnectedness, and even approximations of its size (Trotter II 1999).
Navigating Sensitivity
RDS is designed to reach sensitive populations; gauging sensitive matters within surveys demands additional efforts. Immersive field research can help scholars navigate sensitivity in at least three ways, two of which are ably charted in Thachil’s (Reference Thachil2018) work on “ethnographic surveys”: the use of (1) context-sensitive sampling strategies and (2) sensitive questioning techniques. Thachil’s “worksite” sampling strategy differs from RDS,Footnote 13 but both are context sensitive and potentially efficient ways of gaining access to a population.Footnote 14 I contend that, as a third means of navigating sensitivity, qualitative research of difficult phenomena should supplement an RDS survey. Members of a hard-to-survey population, like those who are vulnerable or engaging in informal or illicit behavior, are especially likely to be protective against outside or seemingly impersonal (i.e., survey) research. Although sensitive questioning techniques within a survey can be helpful, deeper understandings likely stand to be gained. Observation, interviews, and immersion can access the kinds of data that can greatly bolster an RDS survey of a hidden population, including politically sensitive preferences and beliefs (Wood 2007), the role of rumors and silences in narratives of violence (Fujii Reference Fujii2010), and the use of dissimulation in repressive contexts (Wedeen Reference Wedeen1999).
Understanding Respondent Behavior
Interviews can also help address selection bias in RDS studies, which are liable to suffer from nonresponse issues that plague contemporary survey research—but at an increased intensity because of hidden populations’ wish to avoid detection (Smith Reference Smith, Tourangeau, Edwards, Johnson, Wolter and Bates2014). Unit nonresponse is difficult to detect along the respondent-driven chain of sampling: it can be unclear whether a participant chose to recruit fewer than three people, and whether and how many of those potential recruits chose not to participate. As such, many RDS surveys use secondary incentives to encourage successful recruitment. Still, social scientists may lack the capacity or imperative to conduct follow-up surveys that elicit explanations for nonresponse.Footnote 15 An alternative approach is for a researcher to remain in contact with the seeds, deepen contact with willing survey participants, and conduct interviews with them as well as with nonparticipant members of the population. These interviews can provide information about sources of nonresponse and help determine whether its causes are nonrandom (Rogers Reference Rogers and Mosley2013). In addition, this practice can create trustful relationships with key members of the population who can contribute to the survey’s success.
Evaluating Causality
RDS survey data cannot establish causality, a challenge that characterizes almost all observational quantitative data. Approaches to causal identification have become prominent in contemporary methods literature and include qualitative methods for explicating mechanisms that underlie relations between variables (Brady, Collier, and Seawright Reference Brady, Collier, Seawright, Brady and Collier2004; George and Bennett Reference George and Bennett.2005; Gerring Reference Gerring2008). I only add here that ethnographic tools (observation, immersion, and interviews) can be particularly useful for penetrating opaque circumstances and ground-level processes, where hard-to-survey populations may operate (Bayard de Volo and Schatz Reference Bayard de Volo and Schatz2004).
Studying Activist Refugees
In this section, I illustrate the potential of RDS through its application in a study of activist Syrian refugees in Jordan. I first present what we have learned about Syria(ns) since the outbreak of the civil war in 2011 and what we have not and cannot learn using standard survey techniques. I then describe the way I executed an integrative multimethod design that included an RDS survey and the findings that RDS provided to a process-tracing analysis of the relationship between activism and external assistance during conflict.
Refugees typify hard-to-survey and vulnerable populations. Unless a researcher is interested in—and has access to lists of—certain documented groups of refugees (e.g., those registered in formal camps), they can face seemingly “unassailable barriers” to random and representative sampling (Bloch Reference Bloch2007). Some contend that survey research is anyway likely to “completely miss” the defining aspects of refugee experiences (Rodgers Reference Rodgers2004). Syrians now constitute the largest population of refugees at a time that, globally, historic numbers of people are displaced from their homes.Footnote 16 So there has nevertheless been a recent expansion of political science scholarship on Syrians and the uprising-cum-war that led to their displacement.
Among field researchers, the expansion is largely attributable to the refugees themselves. With the country closed off by physical danger and authoritarian restrictions, refugees—who primarily reside in neighboring Lebanon, Turkey, and Jordan—have become conduits for the study of Syria (Corstange and York Reference Corstange and York2018; Koehler, Ohl, and Albrecht Reference Koehler, Ohl and Albrecht2016; Leenders and Mansour Reference Leenders and Mansour2018; Schon Reference Schon2016),Footnote 17 as well as subjects in their own right (Parkinson and Behrouzan Reference Parkinson and Behrouzan2015; Zeno 2017). Pearlman’s work studies refugees as conduits, illuminating Syrian experiences of authoritarianism, revolution, and war (2017), and as displaced subjects, exemplifying the value of qualitative interviews, via snowball sampling, for theory building and causal process tracing (2016a; 2016b). A small number of scholars have managed to conduct quantitative surveys of Syrians. In Lebanon, they have gained access to lists of registered refugees from the UNFootnote 18 and probed Syrians’ attitudes on sectarianism and civil war factions (Corstange Reference Corstange2018; Corstange and York Reference Corstange and York2018), and their propensities to mitigate community problems and to return to Syria (Masterson Reference Masterson2018; Masterson and Lehmann forthcoming). In Turkey, scholars gauged attitudes toward conflict resolution by purposive sampling of neighborhoods with high concentrations of Syrians and random sampling at the household level (Fabbe, Hazlett, and Sinmazdemir Reference Fabbe, Hazlett and Sinmazdemir2019). In almost all cases, these studies’ findings defy popular and scholarly expectations.
To my knowledge, the only social-science survey of Syrian refugees in Jordan was conducted by Arab Barometer (2018), which found that Syrians do not feel represented by any political party. Yet scholars have been studying Syrians in Jordan in other ways, and some are shedding light on refugees’ political mobilization. Through qualitative interviews and participant observation, they have demonstrated how women transferred their uprising activism from Syria to Jordan (Alhayek Reference Alhayek2016); how Syrian civilian associations navigate Jordanian security imperatives while undertaking varieties of activism (Montoya Reference Montoya2015); and how relations between diasporic diplomats, activists in refuge, and those inside rebel-held territory are affected by states and international agencies (Hamdan Reference Hamdan2017). These studies collected data in urban areas, where the vast majority of Syrians in Jordan reside.Footnote 19 Clarke (Reference Clarke2018), harnessing event data obtained from the UN, has demonstrated that encamped Syrians in Jordan have also mobilized contentiously. In addition to identifying diverse manifestations of nonviolent engagement, these studies have highlighted the networked nature of their study populations. Further, they fit into recent literature that has demonstrated—qualitatively and at aggregated levels of analysis—the ways in which refugees enact agency nonviolently (Holzer Reference Holzer2012; Murshid Reference Murshid2014), in contrast to the violence and victimhood often predicted of them.
Syrian activists are hard to survey quantitatively because they are not documented on population lists from which we can sample, are a small fraction of the overall Syrian refugee population, often participate in informal associations and endeavors, and are more situated in cities than in bounded camps. But the studies cited earlier illustrate a population transforming and being transformed by civil war processes. Can we assess emerging theories with new microlevel data? Probability-based approaches would not allow us to do so: any lists, if they existed—for example, of an organization’s employees—would be woefully incomplete; the creation of a list would be ethically unacceptable given the variety of state and nonstate intelligence agents operating in and around the country; and Syrians’ activism usually has not been enacted en masse in forms that could be intercepted. A multimethod research design—integrating RDS with qualitative research tools—allowed me to survey them despite those obstacles.
“To keep the Syrian issue alive”: Using RDS to study Syrian Activists in Jordan
By most accounts, the 2011 Syrian uprising was a fledgling phase of the conflict that repression crushed, armed insurgency overtook, and emergency needs rendered obsolete. Violence displaced millions of Syrians, and for most refugees, what became a protracted exile was characterized by coping, resilience, and foreclosed opportunities (Khoury Reference Khoury2015). Yet throughout the war, Syrian activists continued to engage nonviolently in a wide range of activities on behalf of the Syrian cause, both inside Syria and in refuge.Footnote 20 Their activism evolved and shifted, but it persisted and, in some cases, even became extensive. What explains trajectories of civilian activism in contexts of civil war? My research employs process tracing at the mesolevel in and around Syria to answer this question and to understand conditions for and changes in wartime activism. To bolster it, I sought evidence that would support descriptive inference at the individual level across a population that was hidden, which led me to RDS.
Conceptualizing Activism
Through observation and interviews, I found a wide repertoire of Syrians’ civil action in Jordan, including fundraising for rebels’ families, documenting wartime violations, providing humanitarian support for refugees, and journalistic reporting on the conflict. Syrians referred to acting on behalf of the Syrian cause in any of these ways as activism. In its breadth, this concept fits scholarly notions of nonroutine action aiming to engender change in people’s lives (Bayat Reference Bayat2002; Martin Reference Martin, Anderson and Herr2007),Footnote 21 is resonant among Arabs (Schwedler and Harris Reference Schwedler and Harris2016), and is useful in a field study seeking to capture a population engaged in a range of activities across the border of a civil war state. It is also operationalizable: eligible survey participants would be Syrians in the Amman Governorate who had “engaged in political, social or economic activism on behalf of the Syrian cause” since their arrival in Jordan.Footnote 22
Network Mapping
My repeated immersion over three years suggested that while the networked nature of activists was constant, the network’s boundaries were shifting: many Syrians were engaging in activism, and more were doing so from the capital Amman, rather than in northern border cities. The capital, then, would need to be the geographic area for the survey to ensure a small sampling fraction (assumption 3). And although the nature of their activities was also changing, becoming more formal and oriented toward humanitarianism, individuals seemed to move seamlessly from one action to another—for example, from citizen journalism to development assistance—embodying bridges in the network and suggesting that the network remained singular (assumption 2). Finally, in conversation with interlocutors and ordinary refugees, I gathered that activists were generally young and a small fraction of all Syrians in Jordan, allowing me to roughly gauge the size of the target population.Footnote 23
Design and Sensitivity
The Jordanian government’s restrictiveness and selectivity vis-à-vis Syrians generally, and political activists particularly, demanded circumspection. Before the 2016 survey launch, members of the target population with whom I had developed trustful relations—rather than elites or institutions—assisted me by evaluating the protocol and instrument. The mode of the survey was “phone” based and “computer” assisted: recruitment, appointments, and interviews were carried out via the encrypted messaging and calling applications WhatsApp and Viber, and we recorded responses on a tablet device. These mobile applications were widely trusted, hugely popular, and affordable; they allowed us to maintain confidentiality, emulate the social patterns of activists, and reduce physical burden.Footnote 24 Recruits received messages from their recruiter peers that included information about the survey and a unique non-identifying ID linking them to each other. On completion, we offered participants top-ups to their mobile data plans.Footnote 25 A benefit of the phone-based approach was that respondent ties were likely reciprocal, given shared contact information (addressing assumption 1). Meanwhile, I explored some lines of inquiry—such as cooperation with armed groups or proscribed political ones—in qualitative research.
Sampling
The survey began with five seeds selected for diversity in gender, age, and types of activism, with the objective of reducing their bias on the final sample (Gile and Handcock Reference Gile and Handcock2010).Footnote 26 Their engagements ranged from war-related journalism to providing psychosocial support for refugees; they worked formally or informally with Western organizations, Syrian-led ones, or independently. The survey was ultimately completed by 176 participants over the course of 20 recruitment waves in a three-month period,Footnote 27 a smaller than desired sample size but with a considerable number of sampling waves that, in theory, limits the bias of the seeds on the sample (see figure 1).Footnote 28
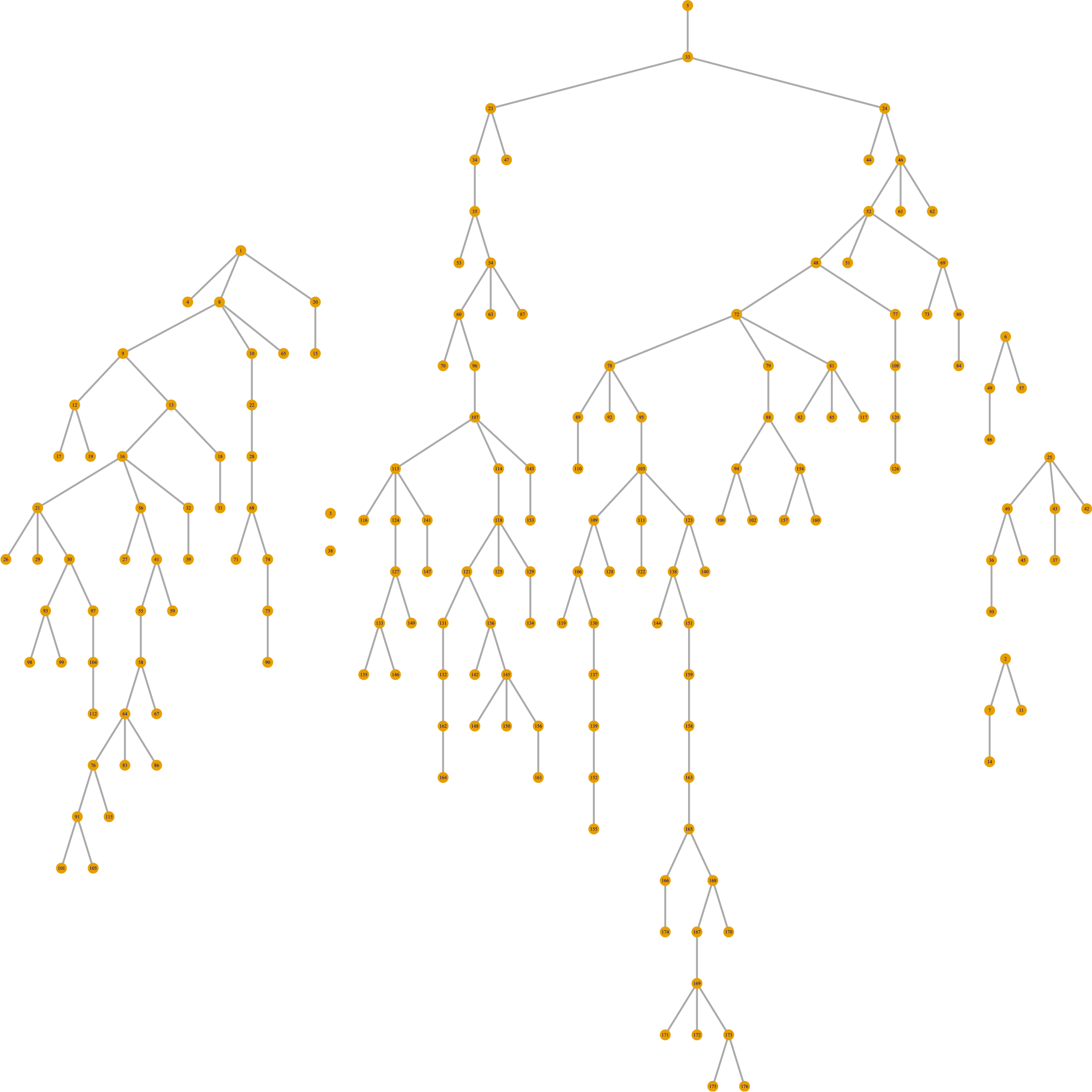
Figure 1 Recruitment tree.
Respondent Behavior
To assess participation and nonresponse bias, I carried out interviews with members of the target population. Many participants expressed enthusiasm for a study that would shed light on their civil actions. Still, others identified three causes for slow recruitment: busyness, study fatigue (refugees, often probed by humanitarian organizations, feel they are rarely rewarded), and distrust. The first two are common explanations for unit nonresponse that did not invoke serious concerns about selection bias. What about distrust? The survey itself revealed that feelings of trust are limited among activists: only 12% [95% CI: 1.4, 22] feel that most people can be trusted; the remainder feel a need to be careful about others. My qualitative research suggested that activists were cautious about perceptions and misperceptions of their engagements in a high-security context. That research, which captured sensitive and political activism extensively, could counterbalance the selection bias of the survey.
Analysis
The survey asked questions about respondents’ sociodemographic backgrounds, activism, and preferences and ambitions. Table 4 presents unadjusted and adjusted statistics on select descriptive characteristics to demonstrate inverse-inclusion probability weighting. It also shows that the population is relatively young and well educated.Footnote 29
Table 4 Descriptive Sample and Population Percentages with 95% Confidence Intervals
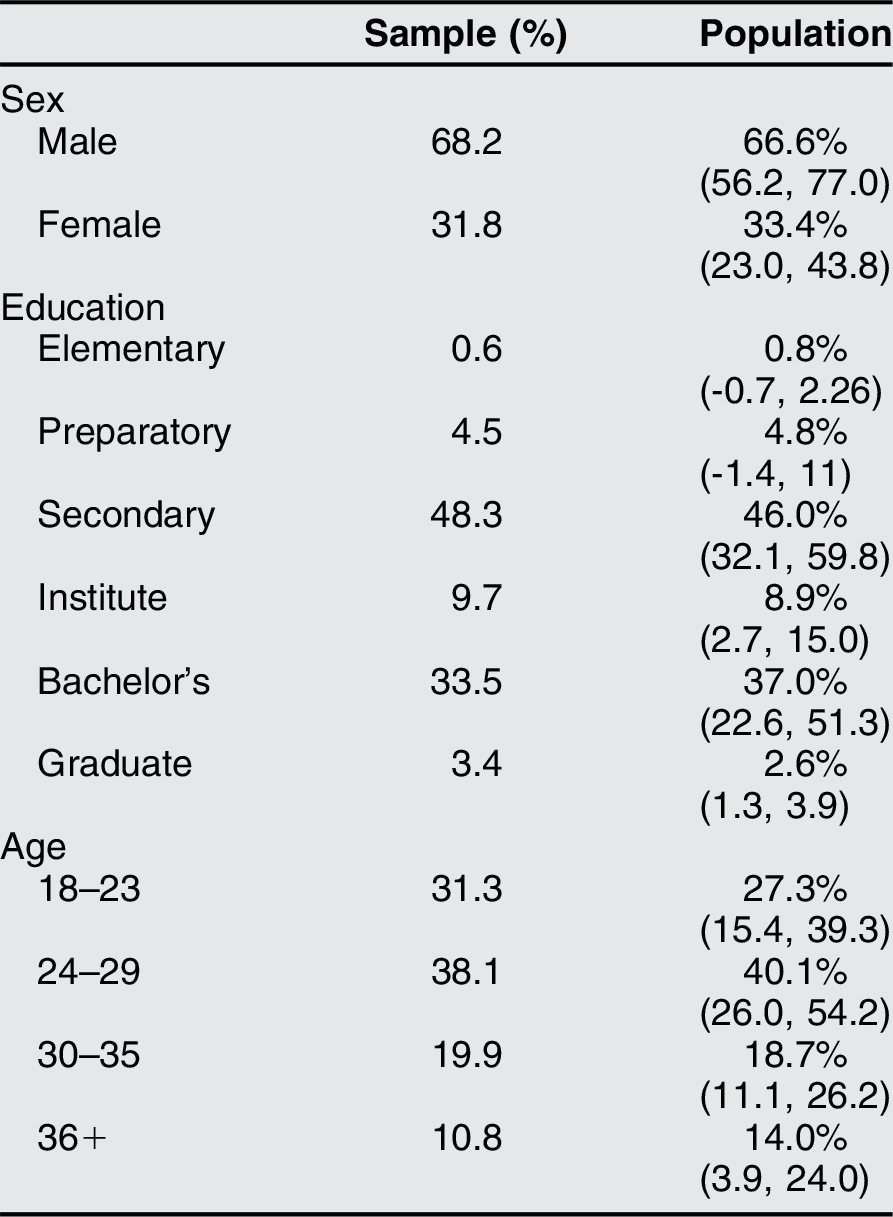
Note : n=176.
RDS proved an appropriate sampling strategy. Activists are networked: the median degree reported was 12, meaning that respondents personally knew and were in contact with 12 members of the overall target population, on average.Footnote 30 Further, these networks are central to their activism: 83% [72, 93] of respondents reported that they cooperate with Syrian activists in Jordan or in Syria to carry out their work.
RDS also offered evidence, or causal process observations, to support my exploration of a linkage between aid and activism. Scholars of civil resistance have noted that external assistance can advance a movement. Yet, although the abundant assistance targeted at Syrians seems to have mobilized them, it may also have changed the nature of their activism. Specifically, I consider how resources provided by external actors generate processes that lead to the spread and formalization of civilian activism.
By virtue of the survey design, all of the participants had engaged in activism. Surprisingly, less than half did so during the uprising in Syria. The survey thus provides evidence that many Syrians entered into activism only after arriving in Jordan, meaning there apparently exist mobilizing opportunities for activism despite Syrians’ vulnerability and the government’s political circumspection. I argue that this opportunity structure is the humanitarian and developmental response in Jordan: today’s humanitarian responses generate a process of what I call feeding activism. The response to the crisis in Syria resembles global trends in humanitarian assistance: aid has increased enormously over time, as have its purposes, the quantity and diversity of its purveyors, and its turn toward local civil society actors (Barnett and Weiss Reference Barnett, Weiss, Barnett and Weiss2008; Duffield Reference Duffield2001; Risse Reference Risse, Carlsnaes, Risse and Simmons2013; de Waal 1997), contributing to individual and organizational entry into and expansion of activism.
Another interesting finding is that two-thirds of activists worked formally with organizations (international, national, and local), apparently despite Jordan’s restrictions on the employment of Syrians. This observation fits into a process of formalizing that is, I contend, generated by humanitarian assistance. The modern provision of aid is characterized by rationalization, resulting in the bureaucratization of actors and the activities in which they are involved, including grassroots ones (Ferguson Reference Ferguson1990; Mundy Reference Mundy2015; Sending and Neumann Reference Sending and Neumann2006).
The survey also demonstrates that Syrians are converging on certain forms of activism and away from others in which they had previously participated, even while in Jordan. Figure 2 indicates that Syrians are engaged foremost in humanitarian relief, development, and media and no longer in protest, institution building, fundraising, and advocacy. This may well fit into a process whereby external aid fragments activism. In aid contracting, multiple principals shape the distribution of resources on which populations of organizations are dependent, leading them to focus more on survival than collective or transgressive action (Bush Reference Bush2015; Bush and Hadden Reference Bush and Hadden2019; Cooley and Ron Reference Cooley and Ron2002; Hoffman and Weiss Reference Hoffman and Weiss2006).
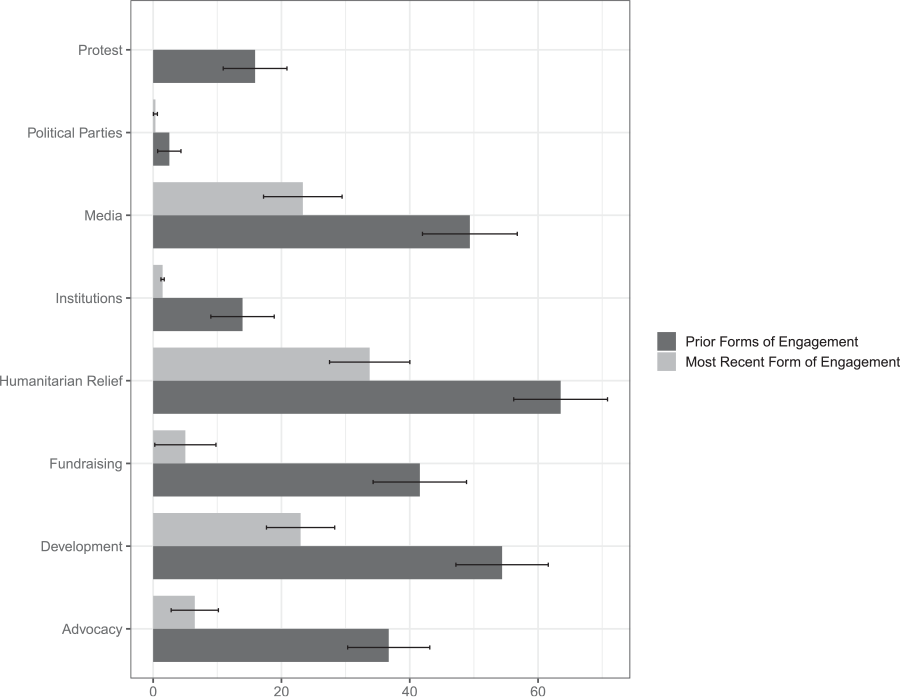
Figure 2 Percent of population previously and most recently engaged in various types of activism (95% CI)
The causal evaluation of these findings takes place within the larger process-tracing project. As part of an integrative multimethod research design, qualitative tools support RDS, and RDS supports the case study.
Evaluation
In this section I situate these substantive findings in an assessment of RDS’s mechanics and performance. For diagnostics, consider the gender variable as a simple illustration. As shown in table 4, about two-thirds of Syrian activists in Jordan identify as male. Were females sufficiently represented by RDS? Social norms that foster same-sex friendships and greater male participation in the public sphere may (1) lower women’s inclusion probability in a survey or (2) limit their participation in activism. We would not want the first issue to mask the second, as likely occurred in a snowball sample survey of the Syrian political opposition in which respondents were only 15% female (IRI 2012). RDS improves on the representativeness of such a sample, and reported network sizes reveal why: the mean network size of male respondents was 18.8, whereas that of females was 13.4—in turn, females were given, on average, slightly larger weights in the analysis. Thus, although their participation in activism is less common than males, RDS ensures that this finding is not determined by their lower inclusion probabilities.
We can use statistical tools to explore this matter further. Assumption 2 expects that the network is a single component (a priori, considered via ethnographic network mapping). Recruitment homophily is the tendency of people to enlist participation from people similar to themselves; a statistic equal to 1 indicates no homophily. On sex, homophily registers at 1.23, meaning more males were recruited than expected due to chance alone. This statistic is modest, but should nevertheless encourage further exploration of relevant differences between men and women. A diagnostic tool, a convergence plot, indicates whether estimates of a trait stabilized and became independent of the seeds. Figure 3 suggests that the gender estimate did indeed begin to stabilize. A larger sample size likely would have ensured complete convergence on the proportion of women in the population.
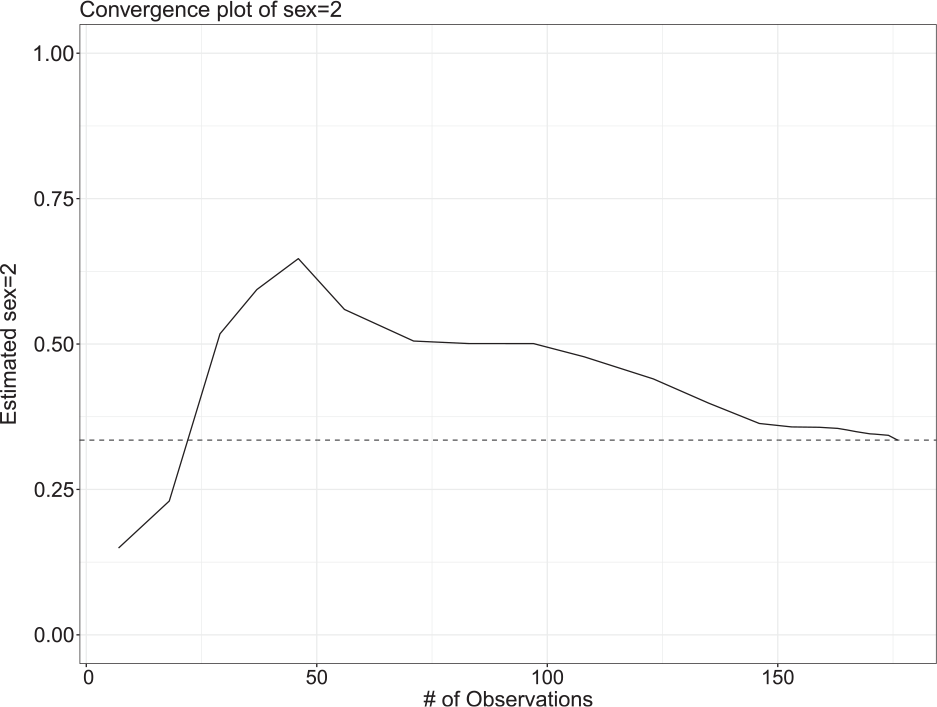
Figure 3 Convergence of sex to female (2).
How did RDS perform overall? The sample size was relatively small and confidence intervals wide. Future applications could benefit from technical and resource improvements such as a larger research team and the use of secondary incentives. Yet leveraging trustful relations between members of a hard-to-survey population did lead to a successful application of the method. The survey achieved a systematic accounting of engaged Syrians that is compatible with emerging research on Syrians’ mobilization, reflecting the diversity of manifestations found separately by previous studies. It fostered and advanced representation by capturing activism in its most high-profile forms—such as documentation of human rights violations—as well as activism that is lower in profile, like provisioning aid for young refugees, enacted by those who wished to effect change but whose networks, political propensities, and levels of experience were modest. That the results are generalizable instills confidence in their use for describing a phenomenon about which we know little, but which is, arguably, intrinsically important (Gerring Reference Gerring2012): a vulnerable population acting under extraordinary circumstances to effect change. Their political behavior is neither marginal to civil war processes nor to transnational responses to conflict.
Conclusion
Sampling frame issues affect survey research even in contexts of high data quality, where solutions like frame combination can be feasible because some record of the population exists. But when data quality is low and a population is hard to survey, understanding, and applying, alternative tools is necessary and appropriate. RDS is a method for sampling and analysis that leverages trust between members of hidden populations to produce representative samples, conclusions about which can then be inferred to the population because of weighting based on respondents’ degree-based inclusion probability. Parting ways with the purities of probability sampling but advancing statistically on nonprobability approaches, RDS may be a “good enough method” for surveying: a method that allows both for flexibility and rigorousness in difficult contexts (Firchow and Mac Ginty Reference Firchow and Mac Ginty2017). It can be better if deployed within an integrative multimethod research design. Is it then good enough for political science?
When evaluating a novel method, we should ask whether it advances our knowledge and theories. In my experience, RDS did both. The survey accessed activists whom I may not have reached on my own because their activism and propensities were low in profile; their traits may not have otherwise been accurately represented at their population levels. In so doing, RDS advanced my ability to theorize the causes and consequences of activism’s trajectories by ensuring a comprehensive understanding of individuals’ backgrounds, engagements, and preferences.
An integrative RDS research design can provide data at the microlevel that advances descriptive inference. Description is often subordinated to causal inference in mainstream political science (Gerring Reference Gerring2012). But it is an end worth pursuing, especially when we know little about a phenomenon—as is almost always the case regarding the political behavior and preferences of hard-to-survey populations—and is a crucial step toward making compelling causal inferences.
Supplementary Materials
To view supplementary material for this article, please visit https://doi.org/10.1017/S1537592719003864