Introduction
To breed super rice, Yuan Longping, an academic at the Chinese Academy of Sciences, proposed developing long-stalked rice varieties to increase biological yield. An ideal rice plant type that exhibited no decrease in the number of spikes per m2, high harvest index and enhanced lodging resistance was evaluated to transform the rice plant from having a dwarf and semi-high stature to high and even super-high stature of over 160–170 cm that would effectively improve the air and light conditions in a paddy field, increase leaf area index, biological yield and grain yield (Wu Reference Wu2000). However, increasing plant height and lodging resistance have always been in conflict in crop cultivation and breeding because the two traits seem to be mutually exclusive. Thus, developing rice varieties that are tall but resistant to lodging has only been a dream for breeders, but it remains one of the key goals in achieving the objectives of super rice breeding. Many factors affect plant lodging and various plant-type evaluation methods have been proposed from different perspectives (Yoshida, Reference Yoshida1972; Donald and Hamblin, Reference Donald and Hamblin1976; Yagi Reference Yagi1983; Tang et al., Reference Tang, Wu, Zhang and Wu1989, Reference Tang, Chen, Xu, Zhang, Xie and Yan2004; Tian and Yang, Reference Tian and Yang2005). Currently, there is an urgent need for a simple and accurate mechanism to evaluate rice plant types and predict the ideal ideotype, especially with the aim of breeding long-stalked lodging-resistant varieties.
The most common methods for constructing mathematical models of plant lodging resistance have been correlation analysis, path analysis and grey relational analysis (GRA) (Zhang et al., Reference Zhang, Chen, Yang, Hua, Gao, Gao and Zhao1999, Reference Zhang, Jin, Ma, Wan, Liu and Xu2010; Wan and Ma Reference Wan and Ma2003; Guan and Shen, Reference Guan and Shen2004; Ma et al., Reference Ma, Ma, Tian, Yang, Zhou and Zhu2004; Lang et al., Reference Lang, Yang, Wang and Zhu2011; Yang et al., Reference Yang, Zhu, Zhang, Zhao, Zhou and Wang2012; Wang et al., Reference Wang, Zhang, Han, Zheng, Wang, Feng and Wang2015a). Both correlation analysis and path analysis are based on linear regression, but in the case of a small sample size, it would be inappropriate to establish a linear relationship based simply on correlation coefficients. The core of GRA is the calculation of the relational grade, which treats each sample with equal weight and leads to poor objectivity (Wang et al., Reference Wang, Guo and Lian2005). Moreover, the classification effectiveness and non-linear fitting of these methods also need to be improved. In contrast, the artificial neural network method has been applied widely due to its capacity for strong pattern recognition and powerful non-linear fitting (Tang, Reference Tang2003; Wang and Zhang, Reference Wang and Zhang2008; Ma et al., Reference Ma, Li and Meng2009; Wang et al., Reference Wang, Wang and Deng2010; Khan Reference Khan2013) with the following distinctive features: (1) it has a strong fault tolerance and makes judgments by learning from imperfect data and graphics; (2) it can be used to approximate a non-linear system of any degree of complexity; and (3) it has good artificial intelligence including self-learning, self-adaptation and association, which provide unique advantages in pattern classification and non-linear fitting. Because of these distinctive properties, the neural network method is able to simulate complex ecological processes and behaviours that are impossible for many traditional models, and it has been applied extensively in various fields (Lek and Guégan, Reference Lek and Guégan1999). The most applied neural networks are the radial basis function (RBF) and back propagation.
In the current study, plant morphological, anatomical and histochemical methods were used to investigate the relationship between lodging resistance and stem-wall thickness, length of the third internode, plant height, stem lignin content, stem vascular bundle number and the large vascular bundle ratio using rice lines with varying degrees of lodging resistance and height. The relationship between stem structure and lodging index was investigated using the RBF neural network and multivariate regression analyses, and the optimal plant type was predicted for each of the morphological types. The current study aimed to establish a rapid and accurate mechanism for evaluating lodging and plant type and to provide a basis for breeding tall, lodging-resistant super rice varieties.
Materials and methods
Materials
Fifteen rice lines obtained from the Institute of New Rice Germplasm, Henan Normal University, China (35°19′ N, 113°54′ E, 118 m a.s.l.) were used: 14040, XF5, F121, X3, 14052, 14078, 14074, 14053, 14085, 14076, 14088, 14030, 121-1, 131-1 and 14012. The lines were planted in three experimental fields (Field 1, 113°54′ E, 35°19′ N, 118 m a.s.l., Field 2, 113°56′ E, 35°4′ N, 81.65 m a.s.l. and Field 3, 114°34′ E, 35°9′ N, 65.32 m a.s.l.) at Henan Normal University on 20 May in both 2015 and 2016, at a density of 67 500 plants/ha. The soil at the fields were all Chao soil (National Soil Census Office, 1998) with pH of 8.3 containing 15–20 g/kg organic matter, 430–560 mg nitrogen (N)/kg, available phosphorus (P) 9.1–11.0 mg/kg, exchangeable potassium (K) 145–156 mg/kg and no previous crop was grown in any of them. Cultivation and management practices were the same for each year, experimental field and treatment. Fertilizer was applied as follows, in both years: compound fertilizer (N:P:K at 15:15:15) was broadcast before ploughing with a urea topdressing broadcast on 10 June and 18 July. Alternate wetting and drying irrigation was used throughout the growing season as follows (growth stages (GS) are according to Zadoks et al., Reference Zadoks, Chang and Konzak1974): during seedling growth and tillering (GS 13–29), 3 days drying (i.e. with no irrigation) and 5 days flooded (to a depth of 2 cm); during stem elongation (GS 30–39), 3 days drying and 9 days flooded (4 cm deep); during booting, inflorescence emergence and anthesis (GS 40–69), 2 days drying and 9 days flooded (5 cm deep); during milk development (GS 70–75), 3 days drying and 7 days flooded (5 cm deep); during late milk and dough development (GS 76–90), 5 days drying and 2 days flooded (5 cm deep). Insecticides were sprayed on 14 July and 25 August each year. Manual weeding was carried out twice, on 3 July and 3 August in both years. Each line was assigned to a plot 5 m × 65 cm under a randomized block design and three replicates with five rows per plot surrounded by three guard rows. Samples (20 plants/line) were collected randomly at the inflorescence emergence stage (GS 50), milk development stage (GS 70), dough development stage (GS 80) and ripening stage (GS 90). The third internode, counting from the base of the stem, served as the material used for analysis.
Analysis of anatomical and morphological characteristics
Sections of the central portion of the third internode (20 µm thick) were cut by hand and incubated for 2 min in 2% phloroglucinol solution according to the Wiesner reaction method and mounted using 50% hydrochloric acid (Strivastava, Reference Strivastava1966). The sections were analysed under an optical microscope equipped with a digital camera (Nikon DS-Fi1c, Japan) to determine the stem radius, stem wall thickness and number of large and small vascular bundles, based on which the value of the stem wall radius was calculated and statistical analysis performed. The area of the vascular bundles was determined under a fluorescence microscope (Zeiss Axioskop 40, Germany).
Determination of the flexural strength and lodging-resistance index of rice stems
Ten representative non-lodged plants were sampled at GS 50, GS 70, GS 80 and GS 90 to analyse flexural strength (a material property, defined as the stress in a material just before it yields in a flexure test), height of the centre of gravity and fresh weight of the stem. All the measurement protocols were modified from Wei et al. (Reference Wei, Li, Wang, Qu and Shen2008).
The stem flexural strength was determined using a plant stem strength tester (SY-S03, Zhengzhou, China) consisting of a sensor rod positioned perpendicular to the surface of a table. The third internode, used as the test material, was unsheathed and placed on the table perpendicular to the sensor rod, and strength values were recorded after exerting compression. The procedure was done five times in total, and the average taken as the flexural strength value of the material. The distance (in cm) between the bottom and the balance point of the stem (including spikes, leaves and sheaths) was measured three times and the mean distance used as the height of the sample stem's centre of gravity. To determine the fresh weight of the stem, the roots were removed and the above-ground portion of the stem washed clean; the water was absorbed with filter paper. Then, the stems were weighed three times and the mean considered as the stem fresh weight of the sample (g). The stem lodging index = (height of the centre of gravity × fresh weight)/flexural strength (Chen et al., Reference Chen, Shi, Yin, Wang, Shi, Peng, Ni and Cai2011).
Determination of lignin content
Samples were collected from non-lodged plants (ten plants/plot) 0, 10, 20, 30 and 40 days after formation of the third internode. The third internodes without their sheaths were frozen in liquid nitrogen and stored at −20°C. According to Lin et al. (Reference Lin, Huang, Wang, Li and Yan1996), 0.5 g of each sample was weighed accurately and placed in a mortar; 95% ethanol was added; and the mixture was homogenized and then transferred to a centrifuge tube for centrifugation at 2600 g for 5 min. The pellet was washed twice with 95% ethanol and twice with 1:2 ethanol/n-hexane (V/V); it was then air-dried, dissolved in 25% bromoacetyl acetic acid and incubated for 30 min in a 70°C water bath. Then, 0.9 ml of NaOH (2 mol/l), 5 ml of glacial acetic acid and 0.1 ml of hydroxylamine hydrochloride (7.5 mol/l) were added, and the volume was ultimately fixed with glacial acetic acid at 15 ml. The absorbance of the supernatant at 280 nm was measured, and the value per gram of fresh sample was used to represent the lignin content (Chen et al., Reference Chen, Shi, Yin, Wang, Shi, Peng, Ni and Cai2011).
Data analysis
The test for significant differences and the linear regression analysis were performed using SPSS17.0 software. Data were standardized using the range standardization method and an artificial neural network was constructed using MATLAB.
Results
The current study was carried out for 2 years at three experimental fields at Henan Normal University in 2015 and 2016. Statistical analysis of the results found no significant differences between sites or seasons (Table 1); therefore, only results from experimental Field 1 (35°19′ N, 113°54′ E, 118 m a.s.l.) in 2016 are presented.
Table 1. Analysis of variance of the studied traits for the 15 rice lines grown at three fields for 2 years

Variations in the flexural strength and lodging index of rice stems
The flexural strength and lodging index of rice stems varied with developmental stage and rice accession (Table 2).
Table 2. The flexural strength and lodging indices of rice stems at different developmental stages

The flexural strength of the third internode tended to increase gradually from GS 50 to GS 90, but it did not change significantly from GS 80 to GS 90. Stem lodging indices for 14053 and 131-1 were significantly (P < 0.05) higher than those of the other lines and were followed by those of 121-1, 14085, X3 and 14052. Stem lodging indices for 14076 and F121 were not significantly different from those of 14040, XF5 and 14088, and the indices for 14074, 14030, 14078 and 14012 were significantly (P < 0.05) lower than those of the other lines. Within the same line, the stem lodging index varied among different GS, and there was an interaction between GS and line; e.g., X3 and 14053 had the highest lodging indices at the mid grain-filling stage, whereas 131-1 and 121-1 had the highest lodging indices at the late grain-filling stage.
Under normal conditions for growth and development, rice is most susceptible to lodging from the mid to late grain-filling stage. The results of the current study showed that the flexural strength of rice stems did not change much during this period, whereas the lodging index changed significantly. This is probably because the material in the base of the rice stem is reallocated during this period, leading to changes in the centre of gravity and the construction of tissue in the stalk, which ultimately causes the lodging index to change. Furthermore, the new starch that accumulates in the grain from flag leaf photosynthates also increases panicle weight.
Correlation between tissue building and the lodging index of rice stems
In different rice lines, the lodging index of the stem is associated not only with flexural strength but also with plant height, height of the centre of gravity and stem fresh weight. Therefore, the mechanism of rice lodging was further investigated by analysing the structural characteristics of stem tissues at different developmental stages. The relationship between those structural characteristics and the stem lodging index is shown in Table 3.
Table 3. Correlation coefficients between the structural characteristics of rice stem tissue and rice stem lodging index

Y, lodging index; X 1, cortical thickness (cm); X 2, number of vascular bundles; X 3, length of the third internode (cm); X 4, proportion of large vascular bundles; X 5, plant height (cm); X 6, area of vascular bundles (cm2); X 7, lignin content (%).
*P ⩽ 0.05; **P ⩽ 0.01.
The rice stem lodging index (Y) was highly significantly (P < 0.01) and negatively correlated with lignin content (X 7) and stem wall thickness (X 1) and significantly (P < 0.05) negatively correlated with the area of vascular bundles (X 6) and proportion of large vascular bundles per unit area (X 4). However, it was highly significantly (P < 0.01) but positively correlated with plant height (X 5), length of the third internode (X 3) and number of vascular bundles (X 2), indicating that the length of the third internode, stem wall thickness and number of vascular bundles were the major structural factors affecting the rice lodging index. Therefore, a moderate increase in stem thickness and decreasing the length of the third internode and number of vascular bundles can decrease the lodging index. Stem wall thickness (X 1) was highly significantly (P < 0.01) and positively correlated with lignin content (X 7), indicating that the level of lignification increased with the relative thickness of the stem wall. The number of vascular bundles (X 2) was significantly (P < 0.05) and negatively correlated with the area of the vascular bundles (X 6) and with the proportion of large vascular bundles per unit area (X 4). Plant height (X 5) was significantly (P < 0.01) and positively correlated with the length of the third internode (X 3); the tallest line, 131-1, which also had the longest third internode, had the smallest lodging resistance. However, 14078, one of the lines with the lowest lodging index, was taller than 180 cm but had rather a good lodging resistance because the length of its third internode was between that of XF5 (dwarf) and 131-1 (tall), and it had a thicker stem wall and larger vascular area (Figs 1a and b) dominated by large bundles. Lines with a high lodging index, represented by 14085, had a significantly (P < 0.01) higher number of predominantly small vascular bundles as well as a smaller vascular area than the other lines and exhibited traits such as a thinner stem and poorer compression resistance (Figs 1e and f). The proportion of vascular bundles in 14088, which had a moderate lodging index, was not significantly different from that of 14012, which had the lowest lodging index, but the area of vascular bundles was higher in 14088 than in 14012 (Figs 1c and d).

Fig. 1. Cross-section of rice stem vascular bundles. The structure and area of vascular bundles are shown. 14078 (a, b) has the largest area of vascular bundles followed by 14088 (c, d) and then 14085 (e, f) with the smallest.
Radial basis function neural network established between stem structure and stem lodging index
Choosing the influential factors
Based on the correlation coefficients between lodging index and the other variables, the following were chosen as the influencing factors for a model using the RBF neural network method: stem wall thickness (X 1), number of vascular bundles (X 2), length of the third internode (X 3), proportion of large vascular bundles per unit area (X 4), plant height (X 5) and area of vascular bundles (X 6).
Prediction by radial basis function neural network
The RBF neural network model to predict lodging resistance was constructed in the Matlab environment. The input and output vectors of the training samples were established by assigning X 1–X 6 as the neurons of the input layer, and the lodging resistance coefficient (Y) was assigned as the neuron of the output layer.
Determination of hidden layer basis function centres and variances
The fuzzy K-means clustering algorithm was used to determine the centre of each basis function and its corresponding variance.
Table 4 shows that six cluster centres were identified through the training and the spreading coefficient was also determined for each basis function.
Table 4. Hidden layer basis function centre and variance

Determination of the weight from the hidden layer unit to the output unit
The weight from the hidden layer to the output layer was revised using the gradient-descent algorithm to minimize the error function. The weight vector after training was [−8.4101, −3.0024, 14.5441, −5.0603, −5.6558, 7.8235]T.
Multivariate regression analysis of stem structure and stem lodging index
Multivariate linear regression (MLR) was performed on the factors described above to screen the major factors affecting the stem lodging index, giving rise to the final fitting equation:

in which three factors, i.e., the stem wall thickness, number of vascular bundles and plant height, were ultimately included in the equation with a determination coefficient (R 2) of 0.997. F- and t-tests showed that the regression equation and regression coefficients were statistically significant (P < 0.05). Above all, the fitting was ideal.
Comparison of the predictive ability of three mathematical models in evaluating the rice lodging index
Based on the correlation data, comparative analysis of the means of the prediction results regarding the built-up area was performed (Table 5), and the prediction and actual curves were also compared (Fig. 2). Figure 2 shows that the predicted curve of the RBF network and the actual curve essentially overlapped; the prediction curve of the MLR network overlapped the actual curve in the area of samples 1–5, deviated from it slightly in the area of samples 12–13 and deviated obviously in the area of samples 7–12. Table 6 shows that the mean residual and relative errors of the RBF network prediction were the lowest at 0.04 and 7.07%, respectively, whereas those of the MLR were obviously higher than those of the RBF network at 0.11 and 11.27%, respectively; approximately twice those of the RBF neural network with poorer prediction accuracy. With the same data support, the overall prediction effectiveness of RBF was better than that of MLR. The residual, which is the difference between the predicted value and the actual value of the model, is capable of portraying the accuracy of the model prediction precisely and visually. Table 5 shows that the RBF network residuals were the lowest for all the samples, whereas those of the MLR were the highest for all the samples, of which the residuals of samples 2, 6, 10 and 11 were obviously higher, and highest for samples 2 and 6, than those of the other samples, indicating that the lodging index values of these samples might also be affected by other factors. The residual data indicated that RBF exhibited better predictive ability. In addition to the overall accuracy and the residuals, another key point in comparing the predictive ability of different models is to analyse the prediction accuracy on the mutation value of the models. Figure 2 shows that the curve of the change in the rice lodging index exhibited two distinct inflection points at samples 8 and 11, generating three periods. In the period from samples 1 to 6, the lodging index increased slowly but then increased sharply in the period from samples 8 to 11. It then increased slowly again, indicating that the stem structure exerted a greater impact on lodging between samples 8 and 11. In summary, the RBF neural network demonstrated significant advantages in prediction accuracy and was suitable for complex non-linear interactions among variables.

Fig. 2. Comparison of the prediction curve and the actual curve.
Table 5. Comparison of the predictive ability between RBF neural network and multivariate regression analysis

Table 6. Comparison of prediction results from different models

Identifying the optimal rice plant type from using the radial basis function neural network
By comparing the predictive accuracies of the two models, the RBF neural network was found to have better prediction ability, so it was used to determine the lodging index of different types of rice stems and identify the optimal plant type.
Table 7 shows the parameters of the optimal rice plant types with respect to lodging resistance found in the current study.
Table 7. The optimal plant type of rice as predicted by RBF
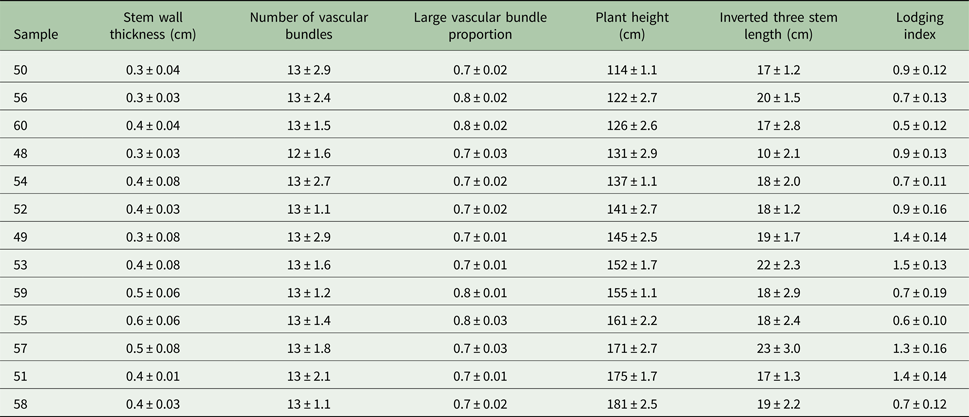
Discussion
Lodging causes poor grain filling and yield loss, reduces grain quality, and reduces mechanical harvesting efficiency (Acreche and Slafer, Reference Acreche and Slafer2011; Berry and Spink, Reference Berry and Spink2012). Much research has been carried out on the external form, internal structure, chemical composition and mechanical strength of cereal crops and how this affects lodging (Esechie, Reference Esechie1985; Luo et al., Reference Luo, Tian, Li and Lin2007; Kong et al., Reference Kong, Liu, Guo, Yang, Sun, Li, Zhan, Cui, Lin and Zhang2013; Novacek et al., Reference Novacek, Mason, Galusha and Yaseen2013; Tian et al., Reference Tian, Liu, Zhang, Song, Wang, Wu and Li2015).
The morphology of plants and internodes strongly affects the plant's ability to resist lodging. Pickett et al. (Reference Pickett, Liljedahl, Haugh and Ullstrup1969) reported that the resistance of maize to stalk lodging was correlated with plant height, stalk diameter and length of internodes below the ear. Stalk lodging in maize was positively correlated with basal internode length (Esechie, Reference Esechie1985), but negatively correlated with basal internode diameter (Martin and Russell, Reference Martin and Russell1984; Novacek et al., Reference Novacek, Mason, Galusha and Yaseen2013). The lodging coefficient of the stem in foxtail millet was associated with specific morphological properties of the culm, such as greater culm diameter, culm wall thickness (Tian et al., Reference Tian, Liu, Zhang, Song, Wang, Wu and Li2015). Wang et al. (Reference Wang, Zhu, Lin, Li, Teng, Li, Li, Zhang and Lin2006, Reference Wang, Wang, Zheng and Tao2012) reported that the most important anatomical and chemical properties for selection of lodging-resistant wheat cultivars were the ratio of culm wall thickness to the outer radius. A negative correlation between plant height and lodging resistance has been reported in some studies (Crook and Ennos, Reference Crook and Ennos1994; Navabi et al., Reference Navabi, Iqbal, Strenzke and Spaner2006). In the present study, the rice stem lodging index was highly positively correlated with plant height, length of the third internode, and negatively correlated with stem wall thickness, basically consistent with the results of previous studies.
The mechanical strength of the stem, closely related to stalk lodging, depends not only on the thickness and length of the stem, but also on the anatomical structure (vascular bundle, mechanical tissue, etc.) and chemical composition of the stem (Luo et al., Reference Luo, Tian, Li and Lin2007; Tian et al., Reference Tian, Liu, Zhang, Song, Wang, Wu and Li2015). The unit number of large vascular bundles and proportion of sclerenchyma tissue in the culm had a positive correlation with the stem's mechanical strength in wheat (Wang et al., Reference Wang, Zhu, Lin, Li, Teng, Li, Li, Zhang and Lin2006). The present study demonstrated that the rice stem lodging index was highly negatively correlated with the area of vascular bundles and proportion of large vascular bundles per unit area but positively correlated with a number of vascular bundles. This indicates that similar to the findings of other cereal crops research, the proportion of large vascular bundles per unit area was a more important trait affecting stem-based lodging. After comparing the diameter and area of the vascular bundles of wheat, barley and oats, Pinthus (Reference Pinthus1974) pointed out that the diameter and area of the vascular bundle in lodging-resistant varieties were significantly larger than those in non-resistant varieties. Zhang et al. (Reference Zhang, Wu, Wu, Ding, Li, Li, Wang, Liu, Tang, Ding and Wang2016) found in rice that a decline in the area of vascular bundles resulted in lower stem strength and a higher lodging index. However, there are different reports on the relationship between the number of vascular bundles and lodging resistance. Duan et al. (Reference Duan, Wang, Wang, Wang and Cai2004) reported that the number of vascular bundles in rice stem was significantly positively correlated with its mechanical strength. Luo (Reference Luo2005) also emphasized that the number of large and small vascular bundles in rice stems was co-developed: lodging resistance was stronger when the number of vascular bundles was higher. Therefore, the number of vascular bundles could also be used as a characteristic for breeding lodging resistant rice cultivars.
The mechanical strength of rice stem depends primarily on the cell walls of mechanical tissue (i.e. sclerenchyma cells under the epidermis and vascular bundle sheath cells) in the internodes. Lignin, the main component of secondary cell walls, is important not only to plant growth and development but also for plant mechanical support (Ma Reference Ma2009). A significant correlation was found between lignin accumulation and the mechanical strength of arabidopsis (Arabidopsis thaliana L.) shoots (Jones et al., Reference Jones, Ennos and Turner2001). In wheat, it was also found that lignin accumulation of culms in varieties susceptible to lodging was lower than in lodging-resistant varieties (Berry et al., Reference Berry, Spink, Sterling, Agarwal and Atalla2003; Chen et al., Reference Chen, Shi, Yin, Wang, Shi, Peng, Ni and Cai2011; Kong et al., Reference Kong, Liu, Guo, Yang, Sun, Li, Zhan, Cui, Lin and Zhang2013). A similar pattern was found in common buckwheat (Wang et al., Reference Wang, Hu, Liu, She, Ruan, Yang, Yi and Wu2015b) and in the present study.
In rice, the stem's mechanical strength increases gradually as the plant grows and matures, and the flexural strength increases correspondingly (Luo et al., Reference Luo, Tian, Li and Lin2007). The present study showed that from the mid grain-filling stage onwards, the flexural strength of rice no longer changed significantly, which differs from the findings in wheat (Chen et al., Reference Chen, Shi, Yin, Wang, Shi, Peng, Ni and Cai2011). Although there was no significant change in the flexural strength of rice stems in the mid grain-filling stage, changes in the structure of the rice stem tissue resulting from the reallocation of matter in the stem and in the degree of lignification, as well as the new starch accumulated from the flag leaf photosynthates, led to changes in the lodging index.
Analysis of the correlation between rice stem structure and lodging index revealed that the two factors have a rather complex relationship with various interactions among the independent variables, so a neural network approach is needed to identify the macroscopic structural factors that affect lodging index of the stalk. There were significant differences in flexural strength and lodging index between rice lines with different plant heights. Therefore, it is possible to identify the variables that have the highest impact on rice lodging by analysing the structural properties of different lines at different developmental stages and to mathematically model the relationship between these variables and lodging index.
In the current study, factors affecting lodging were identified by analysing the correlation between morphological characteristics of the stems and lodging index values of different rice lines, and optimal rice plant types were predicted through three evaluation methods, including correlation analysis, multivariate regression analysis and artificial neural networks. A comparison of the three methods showed that the prediction accuracy of artificial neural networks was approximately twice as high as that of multivariate regression analysis. Through a vertical comparison of the changes in lodging index among the lines, it was found that choosing lines with significant variation in lodging index and examining the structural differences in their stems can provide the appropriate samples for further quantitative analysis of changes in the structural construction in the stem wall and the lignification process of the vascular bundles. Ultimately, based on the predictions using the established RBF neural network, optimal rice plant types were identified, and based on their morphological structures, it was found that the tall rice varieties with strong lodging resistance have stem wall thicknesses of 4.5–6.0 cm and third internode lengths of 17.5–19.5 cm, which is between that of the super-tall, lodging-susceptible and conventional dwarf varieties. Additionally, the proportion of large vascular bundles should be 0.73–0.75 to achieve the breeding objectives of increased plant height and lodging resistance. Radial basis function modelling of the changes in flexural strength and lodging index during rice development provides a new approach for studies evaluating the mechanism of lodging resistance in rice as well as theoretical references for rice breeding.
Acknowledgement
The authors thank Dr Tingjie Wang for his valuable revision to the manuscript.
Financial support
This work was supported by the National Natural Science Foundation of China (No. 31370219, U1304317, 31300163, and U1504316), the Key Research Project Foundation of Higher Education Institutions (No. 16A180045), the Project of the Rice Industry System in Henan Province (No. Z 2012-04-02), the Important Science and Technology Specific Projects of Henan Province (No. 141100110600) and the Youth Foundation of He'nan Normal University (2014QK19).
Conflicts of interest
None.