The obesity epidemic in the USA has increased in the past 40 years, with current rates at 39·8 % in adults and 20·6 % in adolescents aged 12–19 years( Reference Hales, Carroll and Fryar 1 ). Energy-balance behaviours, including diet and physical activity, are behavioural precursors to accelerated weight gain( Reference Harrison, Bost and McBride 2 ). In the American diet, sugar-sweetened beverages (SSB) are a main contributor to added sugar consumption, total energy intake( Reference Hedrick, Davy and Myers 3 ) and obesity( Reference Bleich, Vercammen and Koma 4 ). Evidence from observational and experimental studies consistently demonstrates strong associations between SSB consumption and increased risk of weight gain, type 2 diabetes mellitus and metabolic syndrome( Reference Carwile, Willett and Spiegelman 5 – Reference Wang, Yu and Fang 13 ). In adolescents, SSB consumption is associated with weight gain, increased risk of type 2 diabetes mellitus and dental caries( Reference Malik, Schulze and Hu 14 – Reference Schulze, Manson and Ludwig 16 ).
Systematic review evidence suggests that substituting SSB with healthier alternative beverages, such as water, milk, coffee or tea, can help prevent weight gain without compromising overall beverage intake( Reference Pan, Malik and Hao 17 ). Additionally, replacing unhealthy beverages with healthier caloric and non-caloric options, especially water, is emphasized as part of the ‘healthy eating pattern’ focus of the 2015–2020 Dietary Guidelines for Americans( 18 , Reference Wang, Ludwig and Sonneville 19 ). Even so, SSB intake and healthy beverage (HB) intake are not mutually exclusive and can co-occur on a daily basis( Reference Hedrick, Davy and Duffey 20 ); therefore, it is important to examine factors affecting both SSB and HB intake.
Autonomous motivation can be defined as one’s motivation to engage in a behaviour because of personal choice, interest or value( Reference Deci and Ryan 21 ). According to Self-Determination Theory, autonomous motivation emerges from the interaction between an individual’s values for health and well-being, and social support for these values( Reference Deci and Ryan 22 ). Autonomous motivation is considered an interpersonal intrinsic trait in children and adolescents as it can be socially supported by an authority figure (e.g. parent) and vice versa( Reference Grolnick 23 ). In adults, autonomous motivation is positively associated with health-related behaviours such as increased fruit and vegetable intake, lower overall energy intake, higher physical activity, and lower rates of smoking and risky sexual behaviour( Reference Dwyer, Bolger and Laurenceau 24 , Reference Leong, Madden and Gray 25 ). In adolescents, autonomous motivation is associated with commitment to eat a healthy diet and consumption of fruits and vegetables( Reference Dwyer, Bolger and Laurenceau 24 , Reference Contento, Koch and Lee 26 ). Associations between autonomous motivation and SSB consumption, and between autonomous motivation and substitution of SSB with healthier beverages, have yet to be established.
Dietary behaviours develop in the family context( Reference Birch and Davison 27 , Reference Davison and Birch 28 ). While parents are often conceptualized as ‘agents of change’ when addressing child and adolescent health behaviours, the converse – or adolescents as agents of change – is rarely considered. Should adolescent autonomous motivation predict parent beverage intake (i.e. partner effect) beyond parents’ own motivation (i.e. actor effect), it would highlight new avenues for intervention to promote healthy behaviours in families. Adolescents’ own autonomous motivation and perceived autonomy support from parents can promote reduced adolescent consumption of SSB and increased consumption of HB( Reference Riebl, MacDougal and Hill 29 ). Parents with high levels of autonomous motivation to reduce SSB consumption may have increased HB consumption themselves, and may also have the potential to promote additional consumption of HB in their adolescent children( Reference Ng, Ntoumanis and Thøgersen‐Ntoumani 30 , Reference Powers, Koestner and Gorin 31 ).
Although multiple theories (e.g. Social Cognitive Theory, Family Systems Theory) highlight the need to consider bidirectional relationships between family members and dyads within the family unit, few studies have empirically evaluated the interdependence among family members in SSB-related health behaviours and psychosocial precursors. Dwyer et al.( Reference Dwyer, Bolger and Laurenceau 24 ) recently reported stronger effects of adolescents’ autonomous motivation on parents’ fruit and vegetable consumption (compared with effects of parents’ autonomous motivation on adolescents’ fruit and vegetable consumption). Few studies have evaluated the effects of adolescent attitudes on other health outcomes such as their healthy and unhealthy beverage consumption behaviours.
An ideal methodological approach for evaluating the complex interdependence between family members, by modelling both actor and partner effects concurrently, is called the Actor–Partner Interdependence Model (APIM). Actor–partner modelling examines how one dyad member’s behaviour predicts his/her partner’s behaviour while controlling for dyadic interdependence( Reference Dwyer, Bolger and Laurenceau 24 , Reference Gonzalez and Griffin 32 ). Drawing on APIM methodology, the aim of the present study was to examine the interdependent effects of autonomous motivation to limit SSB consumption in relation to SSB and HB intake in mother–adolescent and father–adolescent dyads.
Methods
Data source and sample
The current study adopts a dyadic cross-sectional design using publicly available data from the Family Life, Activity, Sun, Health, and Eating (FLASHE) study. The FLASHE study was conducted from April to October of 2014 with a demographically representative US sample of parents and their adolescents recruited from an already existing group of respondents of a panel research organization( Reference Nebeling, Dwyer and Oh 33 – Reference Oh, Davis and Dwyer 35 ). Each parent and adolescent completed two Internet-based surveys. A parent (mother or father) and an adolescent were sampled from each household. Parents went through a screening process, and information on all children in the household was collected via a full household roster with one eligible adolescent being randomly selected( Reference Nebeling, Dwyer and Oh 33 – Reference Oh, Davis and Dwyer 35 ). Eligible adolescents were between the ages of 12 and 17 years and lived in the parent’s household at least 50 % of the time( Reference Nebeling, Dwyer and Oh 33 – Reference Oh, Davis and Dwyer 35 ). Data used in the current study from these surveys assessed participants’ diet-related behaviours and factors that may be related to those behaviours, as well as demographic characteristics. Principal investigators received institutional review board approval and all enrolled participants signed a voluntary informed consent( Reference Nebeling, Dwyer and Oh 33 – Reference Oh, Davis and Dwyer 35 ). The FLASHE study design, implementation procedures and methods are outlined in detail elsewhere( Reference Dwyer, Bolger and Laurenceau 24 , Reference Nebeling, Dwyer and Oh 33 – Reference Oh, Davis and Dwyer 35 ).
Dependent measures
Sugar-sweetened beverage consumption
Both members of the dyad reported their SSB consumption with the Dietary Screener Questionnaire (DSQ)( 36 ). Participants reported the frequency of consuming fruit drinks, regular soda, energy drinks and sports drinks in the previous 7d. The response scale ranged from 1 (‘I did not drink [beverage] during the past 7d’) to 6 (‘I drank [beverage] 3 or more times per day during the past 7d’). To develop the composite score, we adopted an approach by Cohen et al.( Reference Cohen, Cohen and West 37 ) as well as by FLASHE investigators( Reference Bolger and Laurenceau 38 ) to ensure interpretation of findings relied upon a consistent metric used across studies within the larger FLASHE project. As such, SSB consumption frequency for each beverage was summed and then rescaled by dividing the total consumption score by the total number of possible categorical indicators and multiplied by 10 to give a composite ‘SSB consumption’ score ranging from 0 to 10.
Healthy beverage intake
HB intake was also assessed using the DSQ( 36 ). Participants reported the frequency of consuming water and milk intake in the past 7d. The response scale and the process used to calculate a composite HB consumption score mirrored those used for SSB.
Independent measures
Autonomous motivation to limit sugar-sweetened beverages
Consistent with Dwyer et al.’s approach( Reference Dwyer, Bolger and Laurenceau 24 ), autonomous motivation to limit SSB was measured using a two-item subscale adapted from the Treatment Self-Regulation Questionnaire( Reference Levesque, Williams and Elliot 39 ), in which participants have to rate the degree to which they disagree or agree with the following statements: ‘I would try to limit how much junk food and sugary drinks I have because I have thought about it and decided that I want to limit junk food and sugary drinks’ and ‘I would try to limit how much junk food and sugary drinks I have because it’s an important thing for me do’. Responses ranged from 1 (‘strongly disagree’) to 5 (‘strongly agree’) and were summed to generate a score ranging from 1 to 10. Previous studies have shown excellent inter-item correlation for these items in both parents (r=0·53) and adolescents (r=0·54)( Reference Dwyer, Bolger and Laurenceau 24 ). These indicators in the current study demonstrate good internal consistency (α=0·70) and strong inter-item correlations (r=0·55) for both parents and their adolescents.
Demographic characteristics
Parent education (‘less than a high school degree’, ‘a high school degree or GED’ (where GED=General Educational Development), ‘some college but not a college degree’ or ‘a 4-year college degree or higher’), parent and adolescent sex, and adolescent age in years were measured and considered as potential covariates in the primary analyses.
Associations between potential covariates, autonomous motivation, SSB and HB intake were assessed prior to performing the primary analyses. To ensure model parsimony, only covariates (e.g. parent education, parent gender and adolescent gender) that were significantly related to the key independent or dependent variables were included in final analyses.
Statistical analysis
APIM, which is also known as a seemingly unrelated regression, was used to test whether parent and adolescent autonomous motivation to limit SSB consumption predicted their own SSB and HB intake (actor effects) while concurrently considering effects on their partner’s beverage consumption (partner effects; i.e. effects of adolescent motivation on parent beverage consumption and parent motivation on adolescent beverage consumption). Gonzalez and Griffin( Reference Gonzalez and Griffin 32 ) advise the use of APIM as a key technique to examine interdependence of behaviour between individuals in a dyad. Dyadic analyses were conducted using structural equation modelling in the statistical software package Stata version 14. There are two key strengths of using a structural equation modelling approach for these data. First, structural equation modelling allows for all model variables to be either observed (measured) or latent (underlying, unmeasured). Second, structural equation modelling is particularly useful for APIM models designed to estimate interdependent paths, because of robust iterative estimation processes that account for both actor and partner effects( Reference MacKinnon and Luecken 40 ).
In the first APIM, autonomous motivation to limit SSB was modelled as a key predictor, and SSB consumption was modelled as a key outcome for both parents and adolescents. In the second APIM, autonomous motivation to limit SSB was modelled as a key predictor, and HB intake was modelled as a key outcome for both parents and adolescents.
In the current study, we used observed variables. Models were run to test actor and partner effects for each outcome (SSB consumption, HB intake) for mother–adolescent and father–adolescent dyads using multiple-group path analysis( Reference Marsh 41 , Reference Muthén and Muthén 42 ). Parent gender was used as the grouping variable covariate in the multiple-group path analysis performed to test the interdependent effects of autonomous motivation to limit SSB consumption in relation to SSB and HB intake in mother–adolescent and father–adolescent dyads, controlling for parent education and adolescent gender. Only dyads with complete data were used for the analysis. The original sample included 1859 parent–adolescent dyads; 1649 had complete data and therefore were included in the analyses. There were no significant demographic differences between families that had complete data and families that did not have complete data.
As suggested by Hooper et al.( Reference Hooper, Coughlan and Mullen 43 ), the following goodness-of-fit measures were used for reporting model fit: root-mean-square error of approximation (RMSEA), comparative fit index (CFI) and standardized root-mean residual (SRMR). RMSEA was used to assess adequacy in the model fit, with values of 0·08 or lower indicating adequate fit( Reference Hoyle 44 ). The CFI( Reference Bentler 45 ) was used to compare the fit of the estimated model against a more restrictive baseline model with values of 0·90 (ranging from 0 to 1) or higher indicating the estimated model demonstrated adequate fit( Reference Hu and Bentler 46 ). The SRMR is an absolute measure of fit; defined as the standardized difference between the observed correlation and the predicted correlation. Given that SRMR is an absolute measure of fit, a value of 0 indicates perfect fit. The SRMR has no penalty for model complexity. A value less than 0·08 is generally considered a good fit( Reference Hoyle 44 ). Finally, we calculated covariance explained by APIM effects as suggested by Bolger and Laurenceau( Reference Bolger and Laurenceau 38 ) and following Kenny’s path tracing rules( Reference Kenny 47 ).
Results
Participant characteristics are summarized in Table 1. Our study sample (n 1649) consisted of one parent (73·9 % mothers) and one adolescent (50·2 % girls) per household. Of the parents sampled, 1225 were mothers and 424 were fathers. Two-thirds of participants identified as White, 36 % identified as Black and 10 % identified as Latino. The majority of parents were between 35 and 59 years of age. Over half of the parent sample (53·5 %) had less than a college degree. Additional demographic characteristics are reported in Table 1.
Table 1 Demographic characteristics of the demographically representative US sample of parent–adolescent dyads (n 1649); Family Life, Activity, Sun, Health, and Eating study, April–October 2014

Descriptive statistics and bivariate relationships for the independent and dependent variables are reported in Tables 2 (mother–adolescent dyads) and 3 (father–adolescent dyads). Overall, parents reported greater autonomous traits to limit SSB (mean=8·28, sd =2·14) than adolescents (mean=7·17, sd =2·77). Parents and adolescents, on average, reported consuming SSB more than three times per week and consuming HB more than twice per day. Adolescents consumed more beverages overall, with the mean score for SSB and HB being higher in adolescents than mothers and fathers. Substantial dyadic covariance was illustrated in the correlation coefficients for mother–adolescent (r=0·50) and father–adolescent (r=0·54) dyads for SSB consumption and in mother–adolescent (r=0·51) and father–adolescent (r=0·53) dyads for HB intake.
Table 2 Pair-wise correlation matrix between predictors, outcomes and theoretically relevant control variables in the demographically representative US sample of mother–adolescent dyads (n 1225); Family Life, Activity, Sun, Health, and Eating study, April–October 2014

SSB, sugar-sweetened beverage; HB, healthy beverage.
**P<0·01.
Table 3 Pair-wise correlation matrix between predictors, outcomes and theoretically relevant control variables in the demographically representative US sample of father–adolescent dyads (n 424); Family Life, Activity, Sun, Health, and Eating study, April–October 2014

SSB, sugar-sweetened beverage; HB, healthy beverage.
**P<0·01.
Results from the APIM analyses are illustrated in Figs 1 and 2. Standardized parameter estimates are reported to allow for comparison across models. Figure 1 illustrates actor and partner effects of autonomous motivation on SSB consumption in mother–adolescent and father–adolescent dyads, controlling for parent education and adolescent gender. Regarding actor effects, there was a significant positive association between adolescents’ autonomous motivation and adolescents’ SSB consumption, but no significant associations between mothers’ or fathers’ autonomous motivations to limit SSB and their SSB consumption. Regarding partner effects, there were significant associations between maternal autonomous motivation and adolescent SSB consumption and adolescent autonomous motivation and maternal SSB consumption. There were no significant partner effects of autonomous motivation on SSB consumption in father–adolescent dyads. Overall, the mother–adolescent and father-–adolescent models predicting SSB consumption provided a modest fit to the data and explained less than 3 % of the covariance within dyad members on SSB consumption.
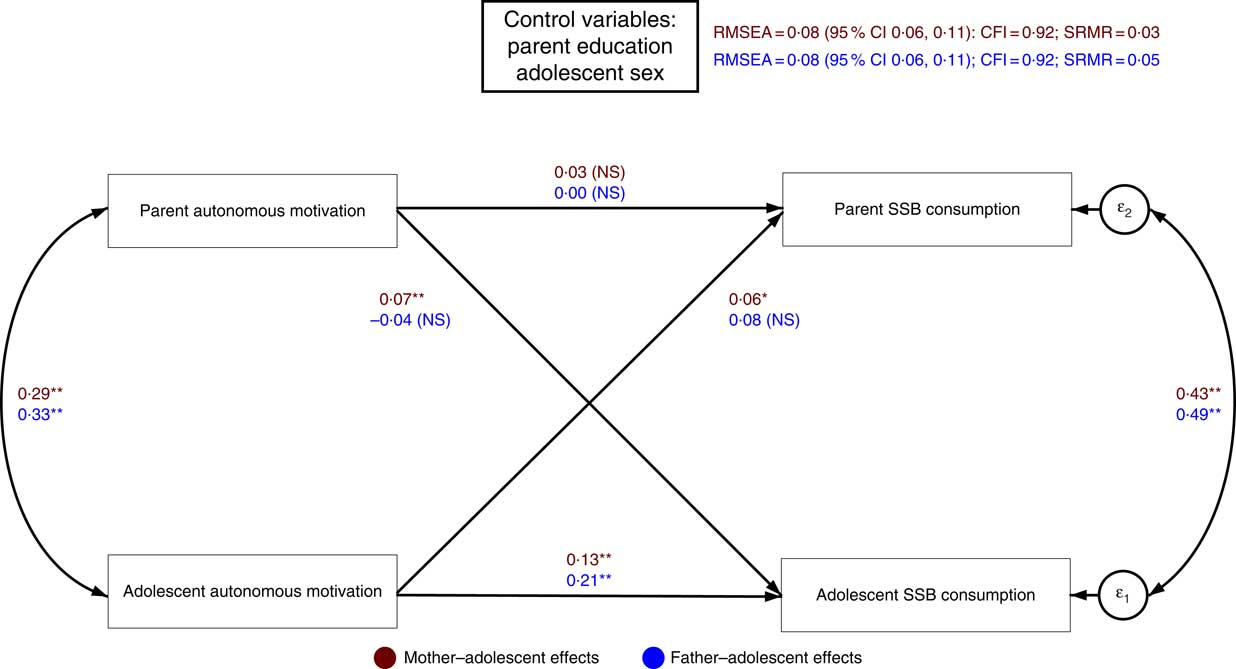
Fig. 1 (colour online) Actor and partner effects of autonomous motivation on consumption of sugar-sweetened beverages (SSB) in mother–adolescent (n 1225) and father–adolescent dyads (n 424), using the actor–partner interdependence modelling approach, in the demographically representative US sample of parent–adolescent dyads; Family Life, Activity, Sun, Health, and Eating study, April–October 2014. *P<0·05, **P<0·01 (RMSEA, root-mean-square error of approximation; CFI, comparative fit index; SRMR, standardized root-mean residual; ε1=0·98; ε2=0·94)
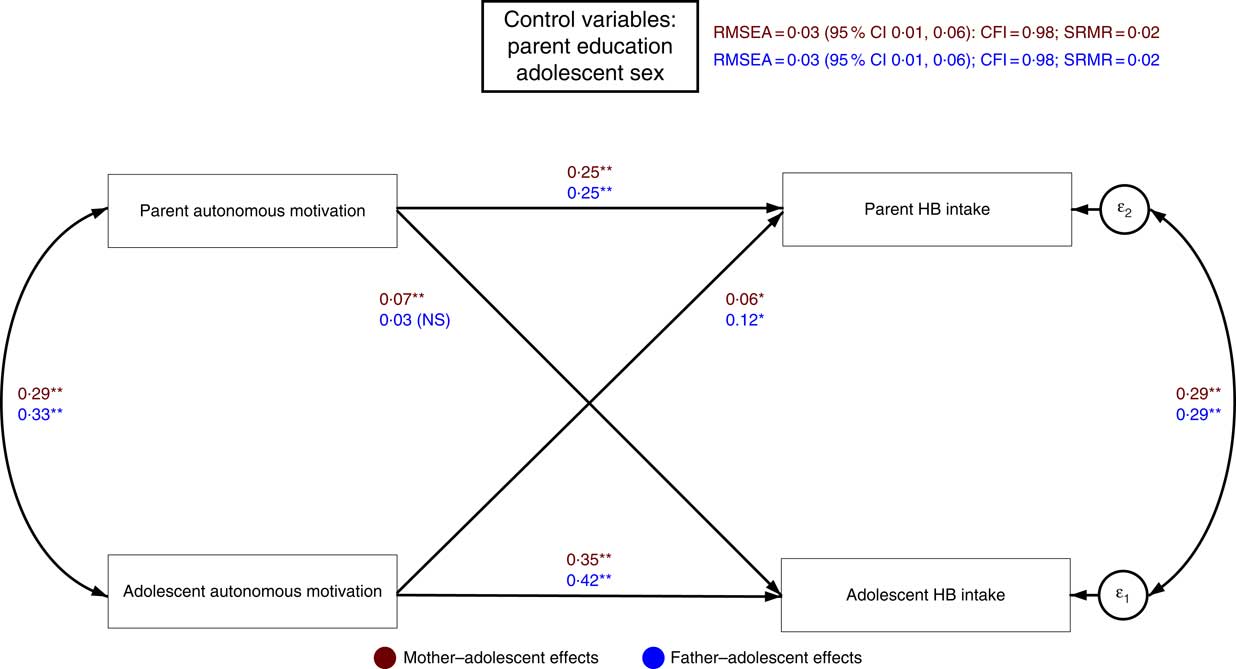
Fig. 2 (colour online) Actor and partner effects of autonomous motivation on intake of healthy beverages (HB) in mother–adolescent (n 1225) and father–adolescent dyads (n 424), using the actor–partner interdependence modelling approach, in the demographically representative US sample of parent–adolescent dyads; Family Life, Activity, Sun, Health, and Eating study, April–October 2014. *P<0·05, **P<0·01 (RMSEA, root-mean-square error of approximation; CFI, comparative fit index; SRMR, standardized root-mean residual; ε1=0·88; ε2=0·79)
Figure 2 illustrates actor and partner effects of autonomous motivation on HB intake in mother–adolescent and father–adolescent dyads. Actor effects were identified for adolescents, mothers and fathers. That is, there was a significant association between adolescents’ autonomous motivation and their HB intake and between parent (mothers and fathers) autonomous motivation and their HB intake. Regarding partner effects, there were significant partner effects for adolescent autonomous motivation on parent HB intake for both mothers and fathers. In addition, there was a significant partner effect for maternal autonomous motivation on adolescent HB intake but not for paternal autonomous motivation on adolescent HB intake. Adolescent autonomous motivation had stronger actor and partner effects on HB intake in father–adolescent dyads, as compared with mother–adolescent dyads. Models predicting HB intake provided an excellent fit to the data, with autonomous motivation to limit SSB explaining 12·78 % of the covariance in the mother–adolescent dyad associations and 17·46 % of the covariance in father–adolescent dyad associations.
Table 4 summarizes actor and partner effects for SSB and HB consumption. Overall, actor and partner effects were more frequently observed for HB than SSB consumption. For HB, all actor and partner effects were significant except for the effect of father autonomous motivation on adolescent HB intake. For SSB, actor effects were found for adolescents but not parents. In addition, partner effects were identified for adolescent–mother and mother–adolescent pathways, whereas no partner effects were identified for fathers.
Table 4 Significant standardized parameter estimates (β) from actor and partner effects, using the actor–partner interdependence modelling approach, in the demographically representative US sample of parent–adolescent dyads (n 1649); Family Life, Activity, Sun, Health, and Eating (FLASHE), April–October 2014

SSB, sugar-sweetened beverage; HB, healthy beverage.
All standardized parameter estimates presented in the table are significant at P<0·05.
Discussion
Dyadic associations between autonomous motivation to limit SSB and consumption of SSB and HB among mother–adolescent and father–adolescent dyads were examined using APIM. Given the substantial correlation between parent and adolescent beverage consumption reported in prior research, we expected that actor and partner autonomous motivation would explain this dyadic covariance. We found moderate support for this hypothesis, with significant positive effects identified for eleven out of sixteen pathways. For HB consumption, all but one of the actor and partner effects were significant. For SSB consumption, half of the anticipated actor and partner effects were significant. Looking specifically at partner effects, three out of four of the adolescent-driven partner effects on parent beverage intake were significant, both the mother-driven partner effects on adolescent beverage consumption were significant and none of the father-driven partner effects on adolescent consumption were significant.
Greater effects of autonomous motivation on beverage intake were identified for HB in comparison to SSB consumption. This difference may be explained by the possibility that family psychological traits such as autonomous motivation are one of many factors predicting adolescent and adult SSB intake( Reference Riebl, MacDougal and Hill 29 ). Additional factors could include the marketing of SSB, the availability of SSB in fast-food outlets and policies such as SSB taxation( Reference Long, Gortmaker and Ward 48 , Reference Falbe, Thompson and Becker 49 ). There may be far fewer environmental factors outside the family shaping HB consumption. In addition, parents’ awareness of the benefits of water intake( Reference Dennis, Dengo and Comber 50 , Reference Muckelbauer, Sarganas and Grüneis 51 ) may make it easier for them to positively influence their child’s HB intake( Reference Riebl, MacDougal and Hill 29 ). These findings imply that either autonomous motivation to limit SSB precludes modifications to other beverage intake behaviours, or that autonomous motivation to limit SSB may be an indicator of attitudes and planned behaviours about beverage intake in general. An example of the former possibility could be the presence of a substitution effect, in which attitudes about limiting SSB consumption lead to greater HB intake in the short term, and then slowly lower SSB intake in the long term. With regard to the latter possibility, the measure used to assess autonomous motivation of SSB was comprised of only two items in a composite score, so it is feasible that these two items are tapping an underlying latent factor comprised of other unmeasured indicators of general beverage consumption behaviours. These findings may indicate the need for longitudinal and measurement studies in this area of inquiry.
Adolescent-driven effects were greater than mother-driven or father-driven effects. In fact, adolescent-driven effects accounted for most of the covariance explained in all models tested. As evidenced by relevant literature, there may be other factors within and outside the family context that play a greater role in shaping adolescents’ health behaviours( Reference Haines, Rifas-Shiman and Horton 52 ). Other contextual factors that may be shaping adolescents’ behavioural intentions and health behaviours (e.g. peers, school, neighbourhood) should also be accounted for in future research exploring determinants of adolescents’ beverage intake( Reference Ezendam, Evans and Stigler 53 , Reference Ebbing, Feldman and Osganian 54 ). Furthermore, these findings illustrate that adolescents possess traits to influence their family members, while parents may be stepping back to promote autonomy in their adolescent children. Bolstered by rapid changes in executive functioning and developmental needs for autonomy, adolescents who develop autonomous motivation to reduce their own SSB consumption may also have the capacity to influence their family’s health behaviours( Reference Baranowski 55 ). Parents may respond by allowing the adolescent to steer or influence family SSB and HB consumption patterns. By promoting autonomous motivation to limit SSB and engage in other health behaviours, interventions may be able to mobilize adolescents as effective agents of change in the family.
More consistent partner effects on adolescent beverage consumption were identified for mothers in comparison to fathers. Although APIM effects explained low dyadic covariance when SSB consumption was the outcome, APIM effects explained significantly greater dyadic covariance in father–adolescent dyads compared with mother–adolescent dyads when HB intake was the outcome. In our sample, mothers played a greater role in shaping adolescents’ SSB consumption compared with fathers. Research suggests that fathers’ psychosocial traits are less influential to their child’s SSB consumption compared with those of mothers in general( Reference Branscum and Housely 56 ). This could mean that maternal psychosocial predictors of their practices and child’s eating are more established( Reference Mitchell, Brennan and Hayes 57 ) compared with fathers’ own psychosocial predictors of their diverse practices( Reference Khandpur, Charles and Blaine 58 ) and their child’s eating behaviours. In addition, the consistent patterns in mother–adolescent dyads compared with father–adolescent dyads could be explained by the shared time spent between mothers and adolescents being typically greater than fathers’ and adolescents’ time together( Reference Lam, McHale and Crouter 59 ). An implication of this finding may reflect the need for teasing out the independent roles that mothers and fathers play in their adolescents’ behaviours.
A few strengths in our study are worth noting. First, APIM account for all exogenous pathways and interdependent covariance, ensuring that each possible hypothesized effect is analysed in relation to the final outcome( Reference Kenny, Kashy and Cook 60 ), which added richness to the current investigation. Additionally, given the use of a large, demographically representative sample of adolescent–parent dyads in the USA, findings are likely generalizable across this population. However, it is important to note that the greatest consumers of SSB (African Americans, Latinos) are proportionally representative to their population demographics, meaning that they are under-represented in comparison to White families in the current study. An additional strength to our study was having a large enough sample of fathers to meaningfully examine the role for mothers and fathers separately( Reference Davison, Gicevic and Aftosmes-Tobio 61 ). With regard to limitations, the cross-sectional nature of the FLASHE study limits our ability to make inferences of bidirectionality in these associations, suggesting that longitudinal designs will be critical for future studies. Seasonal influences were automatically accounted for by collecting data from spring to early autumn (April to October of 2014) for beverage intake. However, other important influences such as geographic and cultural factors per region were not accounted for when the data were collected. This may inhibit our ability to make bold inferences about the findings given the diverse dietary cultures implemented across the country (for example, the southern USA may consume more sweet tea compared with other regions). Although we controlled for adolescent sex, future research should consider whether results are consistent for same-sex parent–adolescent pairs (father–son, mother–daughter) and different-sex parent–adolescent pairs.
Prior research highlights relationships between psychological traits such as autonomous motivation and adolescent( Reference Bere, Glomnes and te Velde 62 – Reference Grimm, Harnack and Story 64 ) and adult beverage intake( Reference Riebl, MacDougal and Hill 29 , Reference Haines, Rifas-Shiman and Horton 52 , Reference Bogart, Elliott and Ober 65 ). To our knowledge, the present study is the first assessing interdependence in parents’ and adolescents’ beverage intake from their autonomous motivation while reporting results separate for mothers and fathers. Accounting for the interdependence of parent–adolescent relationships in the family context is important. Our study expands on current research by taking a dyadic systems approach to address family-level public health problems. Findings from the present research may serve as a stepping stone for future studies examining how family members’ attitudes may influence one another’s health outcomes.
Acknowledgements
Acknowledgements: The authors would like to thank colleagues at the National Cancer Institute and at the home institution for providing feedback at various stages during the development of the manuscript. Portions of this article were presented at the 2018 American Association for the Advancement of Science (AAAS) Annual Meeting on 17 February 2018, in Austin, TX, USA. Financial support: R.F. is supported by a National Institutes of Health Training Grant (award no. T32DK007703). Z.B.K. is supported by a Fulbright Program Grant sponsored by the Bureau of Educational and Cultural Affairs of the US Department of State and administered by the Institute of International Education. J.A.S. is supported by the Harvard T.H. Chan School of Public Health–Dana Farber Cancer Institute Educational Program in Cancer Prevention (R25). The funders had no role in the design, analysis or writing of this article. Conflict of interest: All authors declare that they have no conflict of interest. Authorship: All authors contributed conceptually to the development of the manuscript. R.F. conducted analyses of the manuscript. J.A.S. and Z.B.K. supported in analyses conducted. R.F. and Z.B.K. prepared an initial draft of the manuscript. K.K.D. provided guidance throughout the process and reviewed and edited the manuscript. All authors contributed to the various revisions of the initial draft. All authors have read and approved this version of the manuscript. Ethics of human subject participation: This study was conducted according to the guidelines laid down in the Declaration of Helsinki and all procedures involving human subjects were approved by the NCI’s Special Studies Institutional Review Board (SSIRB) (iRIS no. 327123); the US Government’s Office of Management and Budget (OMB) (0925-0686); and Westat’s Institutional Review Board (IRB) (6053.01.01). Written informed consent was obtained from all subjects.