Chronic non-communicable diseases are the leading cause of mortality in numerous countries and various strategies to address them have been discussed( Reference Schmidt, Duncan and Azevedo e Silva 1 ). One of the main avenues is to combat obesity, in which abdominal obesity, the type most strongly associated with such diseases, has received attention from researchers owing to the greater accuracy it offers in detecting increased visceral fat, which is implicated in cardiometabolic alterations( Reference Tchernof and Després 2 ).
Traditionally, abdominal obesity has been gauged by way of waist circumference (WC), either in isolation or as a ratio to hip circumference (waist-to-hip ratio, WHR)( 3 ). However, given the more important inverse relationship between height and cardiometabolic disturbances( Reference Langenberg, Shipley and Batty 4 – Reference Sawaya, Leandro and Waitzberg 6 ), several authors have suggested adjusting WC for height to augment its diagnostic power in comparison with other anthropometric indices for abdominal and total adiposity( Reference Lee, Huxley and Wildman 7 – Reference Savva, Lamnisos and Kafatos 9 ). In Brazil, waist-to-height ratio (WHtR) is being indicated as a good predictor for arterial hypertension( Reference Fuchs, Gus and Moreira 10 , Reference Caminha, Ferreira and Costa 11 ) and coronary risk( Reference Haun, Pitanga and Lessa 12 , Reference Almeida, Almeida and Araújo 13 ).
Some studies, however, have found in favour of using BMI( Reference Liu, Tong and Tong 14 ) and WC( Reference Vasques, Rosado and Rosado 15 ), as well as WHR( Reference Pereira, Sichieri and Marins 16 ), for different cardiometabolic outcomes.
Given the lack of consensus on the measure most indicated for evaluating abdominal obesity in adults, the present study aimed to answer the following questions: with what likelihood does WHtR correctly identify adult individuals with cardiometabolic alterations? Does this index offer better discriminatory power than other measures of abdominal obesity in detecting clinical outcomes in this age group? Considering the population of the Brazilian Longitudinal Study of Adult Health (ELSA-Brasil), what is the optimal cut-off point of WHtR for predicting these outcomes? Does it perform better than the main cut-off point referenced in the literature (0·50)?
Participants and methods
The present cross-sectional study draws on the ELSA-Brasil baseline, the methodological details of which have been described elsewhere( Reference Aquino, Barreto and Bensenor 17 , Reference Schmidt, Duncan and Mill 18 ). To summarise, the population eligible for ELSA-Brasil comprised active and retired civil servants, aged 35–74 years, from teaching and research institutions in six Brazilian state capitals (Porto Alegre, São Paulo, Rio de Janeiro, Vitória, Salvador and Belo Horizonte).
For the present study, a population aged 35–54 years was selected to gain greater age group homogeneity and in view of the low predictive power of anthropometric indices with increasing age. Individuals lacking information on any of the anthropometric measures of interest (n 6) or on biochemical variables (n 8) were excluded; no values were missing for blood pressure. Participants thus totalled 9264, 4238 men and 5026 women.
Data collection procedures
At the ELSA-Brasil baseline (2008–2010), data were collected by a trained team using standardised procedures and equipment at all the ELSA-Brasil study centres( Reference Schmidt, Griep and Passos 19 ).
The anthropometric measures of weight, height and waist and hip circumferences were obtained using techniques recommended by Lohman et al.( Reference Lohman, Roche and Martorell 20 ), each measurement being taken only once. WC was measured at the mid-point between the lower edge of the costal arch and the iliac crest at the mid-axillary line.
Three measurements of blood pressure (BP) were taken, with the mean of the last two being used in the analyses( Reference Mill, Pinto and Griep 21 ).
As part of the quality control procedures, some measurements were repeated randomly in a study sub-sample. A high intraclass correlation coefficient was found for WC (0·99), for systolic BP (0·88) and for diastolic BP (0·89), indicating good reliability in taking these measurements and thus in constructing the indicators derived from them( Reference Schmidt, Griep and Passos 19 ).
The following biochemical blood measurements were used in defining the clinical indicators: glucose (mg/dl), TAG (mg/dl) and HDL cholesterol (mg/dl). Laboratory samples were collected from participants after a 12 h overnight fast( Reference Fedeli, Vidigal and Leite 22 ).
Anthropometric predictors
The anthropometric indices tested were: (i) BMI (kg/m2); (ii) WC (cm); (iii) WHR; and (iv) WHtR.
Cardiometabolic outcomes
Blood pressure and blood biochemistry served to construct the following selected cardiometabolic outcomes, which were treated dichotomously (yes/no): (i) hypertension, classified as systolic BP≥140 mmHg and/or diastolic BP≥90 mmHg and/or use of antihypertensive medication during the two weeks prior to the test; (ii) hypertriacylglycerolaemia, TAG≥150 mg/dl; and (iii) diabetes mellitus, reported as prior clinical diagnosis and/or use of medication and/or fasting glycaemia > 125 mg/dl and/or 2 h post-test glycaemia≥200 mg/dl in an oral glucose tolerance test.
The last outcome investigated was the presence of two or more components of metabolic syndrome (≥2 MetS), out of a total four possible components: (i) BP≥130 and/or 85 mmHg; (ii) TAG≥150 mg/dl; (iii) HDL cholesterol <40 mg/dl (men) or <50 mg/dl (women); (iv) fasting glycaemia≥110 mg/dl; these limits are based on the National Cholesterol Education Program Adult Treatment Panel III( 23 ). WC was excluded in this case, because its presence could lead to collinearity with the indicators used to evaluate abdominal fat, which also include waist measurement. The use of two or more positive components of metabolic syndrome is a diagnostic option already successfully employed by other authors( Reference Schneider, Klotsche and Silber 5 , Reference Liu, Tong and Tong 14 , Reference Can, Bersot and Gönen 24 ). It is at least as frequent a condition as the situations in isolation – if not more so – and, more importantly still, this set of components of metabolic syndrome together is of greater clinical significance.
Data analysis
The area under the receiver-operator characteristic curve (AUC) and the respective 95 % confidence intervals were calculated, for men and women, for each pair of anthropometric index (BMI, WC, WHR and WHtR) and metabolic outcome of interest (hypertension, diabetes, hypertriacylglycerolaemia and ≥2 MetS). Then, the differences between WHtR AUC and that for each age-adjusted anthropometric index were tested( Reference Janes and Pepe 25 ). AUC are summary measures of the performance of a predictive model. The larger the value of the AUC for a given outcome, the greater the model’s power of discrimination. The value 1·0 indicates excellent power to discriminate between the presence or absence of the outcome and 0·5 is the limit of significance. Accordingly, the lower limit of the 95 % CI should not be below 0·5( Reference Martinez, Louzada-Neto and Pereira 26 , Reference Steyerberg 27 ).
The cut-off points recommended in the literature for the study indices were examined for sensitivity and specificity in detecting two or more metabolic syndrome components, that is: (i) BMI≥25·0 kg/m2 and ≥30·0 kg/m2, for either sex( 28 ); (ii) WC≥88 cm (men)( 28 ) and ≥102 cm (women)( 28 ); (iii) WHR≥0·80( Reference Pereira, Sichieri and Marins 16 ) or ≥0·85( 28 ) (women) and ≥0·90( 28 ) or ≥0·95( Reference Pereira, Sichieri and Marins 16 ) (men); and (iv) WHtR≥0·50 and ≥0·60, for either sex( Reference Ashwell and Hsieh 29 ).
The next stage was to obtain optimal cut-off points for each anthropometric index, using two strategies. The first was to calculate the Youden index (J)=max (sensitivity+specificity – 1), from which the point vertically furthest from the non-association AUC was identified; then, the cut-off point representing the least distance squared (d 2) between the receiver-operator characteristic curve and the point closest to the ideal, represented by the upper left-hand corner of the AUC plot, which indicates maximum accuracy( Reference Perkins and Schisterman 30 ).
A more detailed analysis was made of the predictive power of two different cut-off points of WHtR: ≥0·50, for either sex( Reference Ashwell and Hsieh 29 ), and that identified in the previous analysis. The calculated statistics included sensitivity, specificity, false-positive rate, false-negative rate, test accuracy and Brier score, stratified by sex and age group.
The Brier score represents an overall measure of the model’s performance and evaluates the distance between the likelihood predicted by the index in test (in this case WHtR) and the likelihood of the outcome occurring. A value of 0 % agrees totally and the nearer values approach 50 %, the worse the model’s performance and, therefore, the less informative it is – at 50 % it offers the same probability of being right or wrong. Above 50 % it has no clinical significance. This score penalises false positives and false negatives less than R 2 does( Reference Steyerberg 27 ).
Lastly, the OR and respective 95 % CI values were calculated between the high WHtR (classified by the different cut-off points identified) and the ≥2 MetS outcome.
The data were analysed using the statistical software packages MedCalc® version 14.1 and Stata® version 10.1.
All participants signed a declaration of free and informed consent. The present paper is the product of a subproject approved by the Ethics Committee of Brazil’s Escola Nacional de Saúde Pública (CAAE No. 44106915.2.1001.5240).
Results
Women made up 54 % of the study population. Mean age (46·2 (sd 5·1) years; P=0·18) was similar in both sexes, with nearly one-third of the population in each age stratum, as shown in Table 1. Table 1 also shows that mean WC and WHR were, as expected, higher in men, while BMI and WHtR (indices formulated to include height) did not differ between the sexes. All indices relating to adiposity increased linearly with age in both sexes. The increase was more marked in measures of abdominal obesity (WC, WHR and WHtR) than in BMI. In all age groups, the prevalence of the clinical outcomes was greater among men, and the outcome ≥2 MetS was twice as prevalent in men as in women.
Table 1 Anthropometric characteristics and prevalence of metabolic disturbances, by age group, in men and women (aged 35–54 years), Brazilian Longitudinal Study of Adult Health (ELSA-Brasil) baseline, 2008–2010

WC, waist circumference; HC, hip circumference; WHR, waist-to-hip ratio; WHtR, waist-to-height ratio; ≥2 MetS, presence of two or more components of metabolic syndrome.
The values of the WHtR AUC, after adjustment for age, can be seen in Table 2. They ranged from 0·68 to 0·72 in men and from 0·69 to 0·75 in women, with the smallest AUC found for hypertriacylglycerolaemia and the largest for ≥2 MetS. The AUC obtained for WHtR were also compared with those of the other anthropometric indices for each clinical outcome. WHtR returned significantly larger AUC than BMI for practically all outcomes examined, except hypertension among men. WHtR performed significantly better than WHR for hypertension in both sexes and also displayed larger AUC than WC in predicting diabetes. When the ≥2 MetS outcome was examined, WHtR showed better discriminatory power than the other indices, although not differing from WHR in women (Table 2).
Table 2 AdjustedFootnote † area under the curve (AUC) and respective 95 % CI for anthropometric predictors of metabolic disturbances in men and women (aged 35–54 years), Brazilian Longitudinal Study of Adult Health (ELSA-Brasil) baseline, 2008–2010

WC, waist circumference; WHR, waist-to-hip ratio; WHtR, waist-to-height ratio; ≥2 MetS, presence of two or more components of metabolic syndrome.
*P<0·05, ** P<0·01, *** P<0·001 in test to differentiate between two AUC (WHtR v. other anthropometric indices).
† Adjusted for age group.
The analysis stratified by age group (data not shown) showed that, for all the anthropometric indices tested, AUC values diminished with age. However, loss of predictive power was greater for men after 50 years of age. WHtR maintained reasonably informative AUC values, i.e. good predictive power, in all age strata, with values greater than 0·69 for men and 0·74 for women (from age 50 to 54 years). Among women, WHtR and WHR performed equally well after age 50 years, while WC and BMI showed a more marked decline.
Sensitivity and specificity values at different cut-off points for the anthropometric indices were compared for the ≥2 MetS outcome (Table 3). Many of the cut-off points observed in our study were higher than those previously identified in the literature. The exceptions to this were WC in men and, in some cases, WC and WHR in women.
Table 3 Reference and optimalFootnote † cut-off points for selected anthropometric indices applied in discriminating the presence of two or more components of metabolic syndrome in men and women (aged 35–54 years), Brazilian Longitudinal Study of Adult Health (ELSA-Brasil) baseline, 2008–2010

WC, waist circumference; WHR, waist-to-hip ratio; WHtR, waist-to-height ratio.
† Optimal cut-off points corresponding to maximum value of the Youden index (J)=max (sensitivity+specificity –1) or the least distance squared (d 2) between the receiver-operating characteristic curve and the upper left-hand corner of the AUC plot.
To evaluate discriminatory characteristics, validity measures were calculated for the classic cut-off of WHtR (≥0·50) and the optimal cut-off points identified (≥0·54 for men and ≥0·55 for women), as shown in Table 4. The first cut-off achieved greater sensitivity, with substantial false-positive rate and higher Brier score.
Table 4 Validity measures of waist-to-height ratio (WHtR) cut-off points and OR and 95 % CI for presence of two or more components of metabolic syndrome (≥2 MetS), by sex and age, Brazilian Longitudinal Study of Adult Health (ELSA-Brasil) baseline, 2008–2010
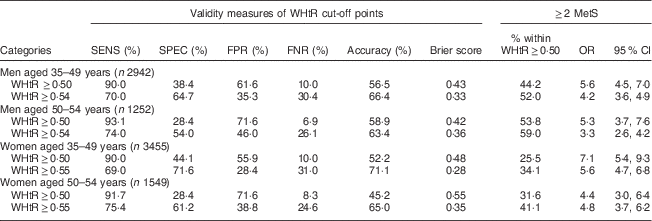
SENS, sensitivity; SPEC, specificity; FPR, false-positive rate; FNR, false-negative rate; % within WHtR≥0·50, prevalence of two or more components of metabolic syndrome in subjects with WHtR≥0·50.
Discussion
WHtR showed good predictive power to identify metabolic disturbances in isolation or jointly, returning AUC close to or greater than 0·70 for all the outcomes, particularly for predicting the presence of two or more components of metabolic syndrome (≥2 MetS).
In the present study, the WHtR AUC ranged from 0·68 to 0·72 in men and from 0·69 to 0·75 in women. AUC equal to or greater than 0·7 were identified in meta-analyses by Browning et al.( Reference Browning, Hsieh and Ashwell 31 ) and Ashwell et al.( Reference Ashwell, Gunn and Gibson 8 ), highlighting diabetes and metabolic syndrome.
For the same outcomes, similar WHtR AUC were observed for Iranian men (0·65 to 0·72), but were lower for Iranian women (0·63 to 0·69), with higher values for ≥2 MetS in both sexes( Reference Mirmiran, Esmaillzadeh and Azizi 32 ). Studies have also found smaller AUC values for these same disturbances in China, ranging from 0·57 to 0·67 for men and from 0·60 to 0·67 for women( Reference Liu, Tong and Tong 14 ). In Turkey, findings were similar to those of the present study for the different outcomes examined, especially regarding the ≥2 MetS outcome, returning AUC of 0·70 and 0·76, respectively, for men and women( Reference Can, Bersot and Gönen 24 ).
Few studies in Brazil have used receiver-operator characteristic curves to measure and compare the performance of WHtR with other anthropometric indices( Reference Caminha, Ferreira and Costa 11 – Reference Liu, Tong and Tong 14 ). Even using other outcomes, studies in Bahia State to identify coronary risk using the Framingham score found important WHtR AUC values for men (0·76)( Reference Haun, Pitanga and Lessa 12 ) and women (0·74)( Reference Almeida, Almeida and Araújo 13 ). Vasques et al.( Reference Vasques, Rosado and Rosado 15 ) examined insulin resistance in male workers at a public university in Minas Gerais and found a WHtR AUC of 0·70. In Alagoas, in a female population-based study, Caminha et al.( Reference Caminha, Ferreira and Costa 11 ) observed AUC equal to 0·73 when evaluating hypertension. Despite the different outcomes and populations, there are similarities in the values encountered for AUC, attesting to the predictive power of the WHtR indicator.
WHtR performed better than the other anthropometric indices, although not differing substantially from WHR for women.
WHtR performed better than BMI for practically all outcomes examined, in both men and women, which is corroborated by the meta-analyses of Lee et al.( Reference Lee, Huxley and Wildman 7 ), Ashwell et al.( Reference Ashwell, Gunn and Gibson 8 ) and Savva( Reference Savva, Lamnisos and Kafatos 9 ). The use of WC instead of weight to construct the indicator (WHtR) brings gains for identifying individuals with higher levels of abdominal fat, despite normal BMI levels( Reference Ashwell and Gibson 33 ). It is widely recognised that the strongest association is between visceral fat and chronic diseases, compared with overweight( Reference Tchernof and Després 2 , 3 ). In addition, it is advantageous to use two rather than three measures, and only one anthropometric indicator for the evaluation of obesity in the adult population, rather than BMI and waist, separately.
In men, WHtR was observed to outperform the other anthropometric indices in predictive power, although the differences from WC and WHR were less substantial in women. The expected presence of increased body fat in women’s thighs and buttocks makes WHR also an important tool in evaluating the extent of body fat in women.
Using the same outcome (≥2 MetS), Mirmiran et al.( Reference Mirmiran, Esmaillzadeh and Azizi 32 ) found higher AUC for WHtR compared with other anthropometric indices. Similarly, in Turkey, WHtR achieved the best discriminatory power in adults of both sexes, always better than WHR( Reference Can, Bersot and Gönen 24 ).
The predictive power of anthropometric indices generally declines with age, which can be explained by the alterations in body composition that take place in both sexes. Among the women particularly, onset of menopause entails lower oestrogen production and increased abdominal adiposity as compared with the hip region( Reference Tchernof and Després 2 ). The rather insignificant alterations in hip circumference measurements with advancing age found among participants in the ELSA-Brasil study point to a certain maintenance of subcutaneous adipose tissue, which is associated with lesser cardiovascular risk than intraabdominal or visceral adipose tissue( Reference Neamat-Allah, Wald and Husing 34 ). That fact may explain why WHR was just as valid as WHtR in predicting cardiometabolic outcomes in women even after age 50 years, with both indicators managing to predict the occurrence of ≥2 MetS accurately. Nevertheless, height would have the advantage, in addition to being self-reported, of being a more routine measurement in health research and services and less inconvenient for the individual examined.
It is noteworthy that ethnic differences among populations have more direct implications for WC, which shows quite different cut-off points by sex and ethnicity( Reference Vasques, Rosado and Rosado 35 ). In this case, WHtR offers the advantage of having very close or identical cut-off points for men and women.
The choice of a cut-off point should be based more on the objectives of health care, than on statistical criteria, properly. The optimal cut-off points originating from the ELSA-Brasil population differed from those suggested by the literature, particularly for BMI( 28 ) and WHtR( Reference Browning, Hsieh and Ashwell 31 ). The latter points were established based on the balance between sensitivity and specificity, resulting in 0·55 for females and 0·54 for males.
In Brazil, these values had already been identified by Almeida et al.( Reference Almeida, Almeida and Araújo 13 ) to discriminate high coronary risk in women, with sensitivity and specificity of 68 and 66 %, respectively. For this same outcome, Haun et al.( Reference Haun, Pitanga and Lessa 12 ) suggested slightly lower cut-off points (0·52 for men and 0·53 for women), and classified individuals with 68 % sensitivity in both sexes and specificity of 64 % for men and 58 % for women. Caminha et al.( Reference Caminha, Ferreira and Costa 11 ) found the optimal cut-off equal to 0·54 for women in Alagoas State.
For the same outcome (≥2 MetS), suggested cut-off points vary considerably for different populations. In the population of Iran( Reference Mirmiran, Esmaillzadeh and Azizi 32 ), the cut-off point for men coincides with the finding of the present study (0·54), but is much higher for women (0·59), due to high prevalence of female obesity. In China, where BMI measurements are around 23·0 kg/m2, the cut-off points are 0·51 (men) and 0·53 (women)( Reference Liu, Tong and Tong 14 ); in Turkey, where mean BMI levels are high (28·3 kg/m2 for men and 30·2 kg/m2 for women), the proposed cut-offs are 0·58 and 0·59, respectively( Reference Can, Yildiz and Samur 36 ). And in the USA, Bohr et al.( Reference Bohr, Laurson and McQueen 37 ) proposed the cut-off point of 0·58 to predict metabolic syndrome in young American adults (24 to 34 years).
High mean BMI and WC, in both sexes, were observed in ELSA-Brasil, which explains the higher optimal cut-off points of WHtR identified, since these indices are highly correlated, but with different predictive ability.
In present study, the cut-off for WHtR proposed internationally for both sexes( Reference Ashwell and Hsieh 29 , Reference Browning, Hsieh and Ashwell 31 ) (0·50) obtained high sensitivity (about 90 %). When applied in populations with high prevalence of obesity (as in the case of ELSA-Brasil( Reference Schmidt, Duncan and Mill 18 )), more sensitive cut-off points inflate the number of false positives. However, this is not a problem for actions in public health, in which we are interested in the early detection of individuals at risk or in subclinical stages of diabetes or CVD, like impaired glucose tolerance or dyslipidaemia. (Higher WHtR cut-off points reduced the prevalence of abdominal obesity in the population, in some strata by almost half – as among women up to 50 years of age – increasing by three or four times the number of false negatives, failing to detect a possible onset of metabolic disease.)
Therefore, the poor specificity presented by the 0·50 cut-off point does not compromise its use, since the cost the health care of these diseases will probably be much higher than the inclusion of false-positive individuals in new screening tests, such as BP measurement and laboratory tests( Reference Kapur 38 ), or health education promotion.
Despite the intrinsic limitations on causality in cross-sectional designs, it is valid to claim that the substantial number of individuals in the ELSA-Brasil population afforded the present study greater statistical power, contributing not just better precision in estimating AUC, but also permitting analysis adjusted for possible confounders and observation of the desired effect in different sex and age strata.
Information quality assurance and control measures were also taken at all stages of data collection( Reference Schmidt, Griep and Passos 19 ), minimising errors or flaws very common in multicentre studies involving large numbers of actors.
Although widely used in diagnostic studies to compare the power of different predictors, the AUC is an insufficient measure of discrimination to evaluate the predictive model completely. In addition, we used the Brier score, a robust methodology for analysis of validity( Reference Steyerberg, Pencina and Lingsma 39 ) and, in the present study, the values corroborated the consistency of the results, highlighting the internal validity of the anthropometric indicator tested.
In conclusion, we propose to include WHtR, which had good discriminatory power for important cardiometabolic outcomes and performed similarly to, or better than, other available indices of adiposity, as an anthropometric index in the monitoring of nutritional status of the Brazilian adult population, assuming the cut-off of 0·50 as more sensitive and indicated for actions in public health.
Acknowledgements
Acknowledgements: The authors would like to thank all ELSA-Brasil participants for their contribution to this study. Financial support: The ELSA-Brasil baseline study was supported by the Brazilian Ministry of Health (Science and Technology Department) and the Brazilian Ministry of Science and Technology (Financiadora de Estudos e Projetos and CNPq National Research Council; grant numbers 01 06 0010.00 RS, 01 06 0212.00 BA, 01 06 0300.00 ES, 01 06 0278.00 MG, 01 06 0115.00 SP, 01 06 0071.00 RJ). The Brazilian Ministry of Health and the Brazilian Ministry of Science and Technology had no role in the design, analysis or writing of this article. Conflict of interest: None. Authorship: Contributed to the conception and planning of the study: M.C., D.C., L.O.C., R.H.G., M.C.M. and M.J.M.F. Data analysis and interpretation: M.C., J.U.B. and M.J.M.F. Prepared the manuscript: M.C. Critical review of the manuscript: M.C., D.C., L.O.C., R.H.G., M.C.M. and M.J.M.F. Ethics of human subject participation: This study was conducted according to the guidelines laid down in the Declaration of Helsinki and all procedures involving human subjects/patients were approved by the Ethics Committee of Brazil’s Escola Nacional de Saúde Pública (CAAE no. 44106915.2.1001.5240). Written informed consent was obtained from all subjects/patients.