CLINICIAN'S CAPSULE
What is known about the topic?
There are no large population-based studies demonstrating the impact of changing EMS resource allocation (ambulance response) and patient outcomes.
What did this study ask?
What was the impact of implementing a new evidence-based EMS resource allocation plan on 24-hour mortality?
What did this study find?
This study found a 7% reduction in the probability of dying within 24 hours of ambulance response with the EMS response plan.
Why does this study matter to clinicians?
Clinicians involved in EMS systems and ambulance response may consider implementing a similar data linked analysis to evaluate patient outcomes.
BACKGROUND
The optimization of resource allocation for emergency medical services (EMS) systems determines appropriate response plans for patients to minimize morbidity and mortality. The resource allocation plan prescribes the paramedic qualification that should respond and at what speed, as well as the associated potential first responder attendance for a given 911 call that is EMS-system specific.Reference Bandara, Mayorga and McLay1,Reference Ball, Williams and Smith2 Most EMS systems revise the resource allocation according to dispatch codes on a regular basis in the interests of optimizing patient care.Reference Hinchey, Myers, Zalkin, Lewis and Garner3–Reference Hettinger, Cushman, Shah and Noyes5
As the sole legislated authority to provide emergency health services in the Canadian province of British Columbia, BC Emergency Health Services (BCEHS) has a responsibility to use resources safely, efficiently, and in the best interest of patient care, safety, and outcomes.6 Prior to 2012, BCEHS revised its resource allocation based on a non-structured, subjective evaluation. In 2012–2013, BCEHS adopted an evidence-informed methodology, and changes were implemented accordingly.Reference Craig, Verbeek and Schwartz7 The decision for change used clinical and operational data to validate the appropriateness of the assigned resources and speed of response.Reference Craig8 The development of an EMS response plan is based on evidence for interventions and response provided by data generated from call-taking patient-interrogation tools such as the Medical Priority Dispatch System (MPDS®). The MPDS® protocol was designed to standardize and codify the operation. Each determinant is made up of a number, ranging from 1 (abdominal pain) to 32 (unknown); a letter ranging from O (very minor) to E (immediately life-threatening), relating to the severity of the patient's condition; and a number that is a subgroup and provides more specific information about the patient's condition.Reference Clawson, Bass, Brice, Delbridge and Gunderson9–Reference Cone, Galante and MacMillan12 As a result of the 2013 response plan revision, out of approximately 1,300 MPDS® codes, 161 codes were reclassified: 68 were reclassified from requiring advanced life support to requiring basic life support, 74 were reclassified from requiring a rapid response (“lights-and-siren”) to regular speed response (“no-lights-and-siren”), and 19 were reclassified to a combination of resource and speed upgrades or downgrades. Fire first responders remained involved in the most time-sensitive incidents requiring interventions such as cardiopulmonary resuscitation (CPR) and defibrillation.
The purpose of this study was to determine the effect on 24-hour mortality of the implementation of a revised resource allocation plan in a province-wide, population-based EMS system.10,Reference Michael and Sporer13,Reference Newbold, Eyles, Birch and Spencer14 Twenty-four hour mortality was chosen because of its ability to reflect variations in health status and to tie mortality to the actual situation of 911 EMS treatment and transport versus other causation that might have occurred in the hospital contributing to 30-day mortality.Reference Strauch, Bergmans, Winkens and Roekaerts15
METHODS
Study population and setting
BCEHS serves a population of 4.5 million in an area of 944,735 km2 covering the entire province of British Columbia. The 911 dispatch call volume is over 500,000 events per annum, and there are approximately 4,000 paramedics ranging from emergency medical responders to advanced life support paramedics (excluding first responders).6 The outcomes and EMS data used for this observational study covered 36 months from 2012 to 2015 (19 months before implementation of the new response plan and 17 months after implementation). Patients under 18 years of age, obvious deaths, and MPDS® determinant O (referral to nurse line) were excluded from this evaluation. This study excluded air medical response transport and inter-facility transfers.
Data sources
BCEHS data included records from its computer-aided dispatching system from Intergraph™ (http://www.hexagonsafetyinfrastructure.com/products/command-control-and-communications/intergraph-computer-aided-dispatch) and patient care reports submitted by paramedics. Patient care reports data were housed electronically in a patient care information system built by BCEHS. The National Ambulatory Care Reporting System (https://www.cihi.ca/en/national-ambulatory-care-reporting-system-metadata) for Emergency Department (ED) admission data and the discharge abstract database (https://www.cihi.ca/en/discharge-abstract-database-metadata) for hospital separation data were also included in the analysis.16,17
Data linkage and cohort derivation
Every personal health number recorded by paramedics was validated at BCEHS given the existing billing process; therefore, the personal health number was used to perform deterministic data linkage. The personal health number completeness was 93.7% in the pre-period and 94.4% in the post-period. The increase in personal health number completeness was common in each geographical area and in each patient severity group that was classified by MPDS® determinant. The disposition result of the ambulance data was included as part of the overall calculation of mortality as the only available outcome data for patients who died before reaching the hospital. These cases were not expected to be linked with National Ambulatory Care Reporting System or discharge abstract database (132,216 cases). There were 965,346 records expected to be linked. If a patient was transported by ambulance to the hospital, the ambulance record was linked to the National Ambulatory Care Reporting System record by the personal health number and a six-hour time window between 911 phone calls received and the registration time at the ED. If a patient had multiple 911 phone calls or multiple ED registrations in the six-hour period, the records with the closest timestamps between the ambulance and ED registration were considered as the matching visit. After the ED visit, if the patient was admitted to the hospital, the discharge abstract database record was linked by personal health number and a six-hour time window between the ED disposition time and hospital admission time.Reference Sayers, Ben-Shlomo, Blom and Steele18,Reference Newgard19 Records with an exact 24-hour difference between the ED disposition time and hospital admission time were linked assuming the date was entered incorrectly in either National Ambulatory Care Reporting System or discharge abstract database. Without this step, these records would not have had outcome data available for analysis. In total, 716,963 records were linked with National Ambulatory Care Reporting System or discharge abstract database out of the 965,346 expected that equated to a 74% linkage rate. Cases that were not constantly under National Ambulatory Care Reporting System collection coverage throughout the entire period were removed to control for consistent outcome data during the study period.
Statistical analysis
All data preparation and statistical analysis were conducted using SAS® version 9.4. Descriptive statistics were performed to describe the change in the cohort during the pre- and post-study periods and to inform the inferential analysis. The 24-hour mortality was modelled using the pre- and post-resource allocation plan revision period as the targeted predicting variable and confounding variables. Adjusted odds ratios (OR) were calculated using logistic regression, and goodness of fit test was performed. Variables in the regression were selected based on their contribution to mortality.
Univariate logistic regression was first performed to exam the association between covariates and 24-hour mortality, as well as to determine the interaction between covariates and the pre- and post-periods. The final multivariate logistic regression was built with the targeted predicting variable of the pre- and post-resource allocation plan revision period and the significant confounding variables that were selected forward stepwise from the above steps.
Interrupted time series is a valuable tool for evaluating the effectiveness of population-level health interventions. The interrupted time series design was based on a regression analysis of outcomes that occurred during a continuous sequence of time and is frequently applied in population-level policy evaluations.Reference Taljaard, McKenzie, Ramsay and Grimshaw20 The interrupted time series analysis was applied by fitting a generalized least squares regression of the monthly 24-hour mortality during the study period with the time variable and the variables that represent the level and trend change after the resource allocation plan revision to exam the impact of the implementation. Autocorrelation, seasonality, and the opioid overdose population were considered because of an overdose spike started after the study period. There was no seasonality or overdose effects observed, and mortalities that were six months apart were correlated and addressed as the correlation structure of the generalized least squares regression model. The coefficients of level and trend variables imply the impact of the resource allocation plan revision after considering the pre-existing trend of 24-hour mortality that was accounted by the time variable.
Ethics approval
Ethics approval was obtained from the University of British Columbia Clinical Research Ethics Board.
RESULTS
Patient characteristics
The study population included 562,546 adult cases attended by paramedics with an evaluable primary outcome measure. Of these, 292,094 patient cases were included before the revision cohort revision was implemented (April 1, 2012, to October 31, 2013) and 270,452 patient cases were included after implementation (November 1, 2013, to March 31, 2015). The derivation of the study population is shown in Figure 1.
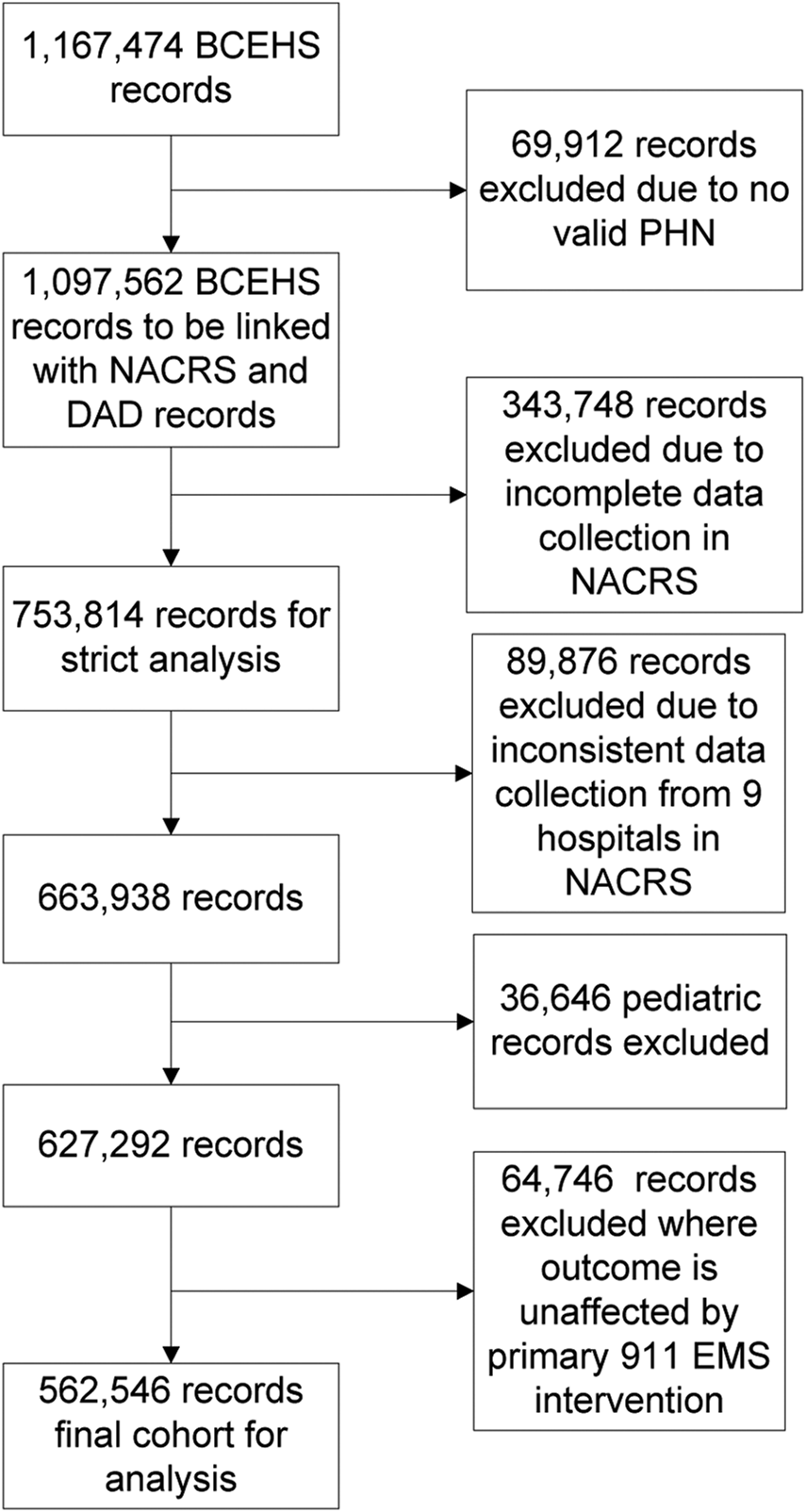
Figure 1. Derivation of the study population.
Patient characteristics for the overall cohort and the two comparison cohorts are presented in Table 1. The mean age was 59.1 (22%) years; almost one-third (30.1%) of the cases were older than 75 years, with patients being slightly older after implementation; about one- half (50.4%) were male; and over 90% were from urban and metropolitan areas. About 1% of cases were classified as immediately life-threatening (MPDS® determinant Echo), with a slightly higher proportion of immediately life-threatening after implementation. The two cohorts were similar in terms of overdose subgroup and distribution of cases across days of the week. There was a lower percentage of cases in the afternoon hours (1200–1800) and a higher percentage of cases in morning hours (0600-1200) after implementation. Because of the pre- and post-periods, there was a significantly higher proportion of winter season cases after implementation, and there was a higher proportion of cases in spring, summer, and autumn seasons before implementation. There were slightly more Charlie and Delta determinant cases after implementation and slightly more Bravo determinant cases before implementation. To better understand the factors influencing survival, we conducted several univariate logistic regression analyses to assess the effects of each variable such as age, gender, event location, acuity, opioid-related overdose, season, day of the week, hour of the day, and MPDS® determinant.
Table 1. Characteristics of study population

ILT = Immediately life-threatening; MPDS® = Medical Priority Dispatch System.
Multivariate-adjusted analysis of 24-hour mortality
Overall, the probability of dying within 24 hours of an EMS call was 7% lower after implementation (OR = 0.936, 95% CI 0.886–0.989, p = 0.018) using the multivariate-adjusted model shown in Figure 2. Patient age was significantly associated with worse outcomes: compared with 18- to 54-year-old patients, 55- to 64-year-old patients had a two-fold higher probability of 24-hour mortality; 65- to 74-year-old patients had an almost three-fold higher probability of 24-hour mortality; and 75-year-olds or older patients had more than a three-fold higher probability of 24-hour mortality. The probability of 24-hour mortality was significantly higher in males and lower in urban and metro areas. Morning calls received between 6:00 a.m. and noon had a higher probability of 24-hour mortality compared with calls received between midnight and 6:00 a.m. Compared with the MPDS® Charlie determinant cases, Alpha and Bravo determinant cases had an almost three-fold and almost one-third lower probability of 24-hour mortality, respectively, and Delta and Echo determinants had an almost six-fold and 300-fold higher probability, respectively, of 24-hour mortality. The season did not have a significant association with the primary outcome.

Figure 2. The multivariate-adjusted odds ratio of 24-hour mortality for the overall cohort.
Because of the significant difference in 24-hour mortality among the MPDS® determinants Alpha through Echo, the pre- and post-study period and MPDS® determinant interaction were tested. Although the interaction was not statistically significant (p = 0.46), the introduction of the interaction reduced the 24-hour mortality difference seen between the two study cohorts. To account for the potential interaction of the acuity, a combined MPDS® pre/post variable was developed. Of all combinations of the pre/post variable and MPDS®, Alpha and Bravo determinants had a significantly lower probability of 24-hour mortality compared with the reference group (Charlie determinant and pre-resource allocation plan revision), and Delta and Echo determinants had significantly worse outcomes. Furthermore, both Delta and Echo determinants after implementation had a lower probability of 24-hour mortality, as compared with the reference group than their combination before implementation.
The multivariate-adjusted analysis of 24-hour mortality in the immediately life-threatening group is described in Figure 3. After adjustment, the probability of 24-hour mortality of an EMS call was 11% lower after implementation (OR = 0.890, 95% CI 0.808–0.981, p = 0.019). Further analysis was undertaken to determine the impact of resource allocation revision on the immediately life-threatening group. In the post-implementation immediately life-threatening group, the proportion of patients with ineffective breathing (MPDS® determinant 06E01) was significantly higher than in the pre-implementation immediately life-threatening group. This was highly correlated with the winter season, as there were more winter months post-implementation.

Figure 3. The multivariate-adjusted odds ratio of 24-hour mortality for the immediately life-threatening subgroup.
Interrupted time series analysis
The difference between the pre-implementation trend projected forward and the observed post-implementation trend was significant (p < 0.001) in the overall cohort.
It was observed that the 24-hour mortality of the immediately life-threatening group was increasing at the rate of 0.69% per month (8.32% per year) prior to the implementation of revision; it decreased by 3.25% immediately after implementation, and the previously existing increasing trend shifted to a decreasing trend at 1.38% per month (16.56% per year). The descending change in 24-hour mortality trend was significant (p < 0.001, Figure 4).

Figure 4. An interrupted time series analysis of 24-hour mortality of immediately life-threatening subgroup pre- and post-resource allocation plan change.
DISCUSSION
In this evaluation, it was found that the overall mortality rate was lower after implementing a change in the EMS response plan to better match resources to patient needs. Without the change in ambulance resource and speed of response, there was a steady increase in mortality rate in the immediately life-threatening group. After the change, the immediately life-threatening group demonstrated a reduction in mortality of 11%, and a decreasing trend in mortality was observed. In previous literature, it was observed that a subset of MPDS® codes were associated with predicting ED mortality and called for further studies to validate the findings.Reference Hettinger, Cushman, Shah and Noyes5,Reference Sporer, Youngblood and Rodriguez21 Another study suggested there may be a small beneficial effect of rapid response time by advanced life support on survival rate. However, the study did not suggest that EMS response time was unimportant for lower acuity patients.Reference Blanchard, Doig and Hagel22 Furthermore, there was limited ability to predict which patients would require advanced life support intervention.Reference Sporer, Johnson, Yeh and Youngblood23 There have been no large observational studies conducted to evaluate the impact of changing EMS resource management according to MPDS® code and patient outcome to date.
The strengths of this study are the inclusion of all sizes of communities across a province with varying geographic area, a large number of cases, and a population-based sample. The data linkage using personal health number matched prehospital data sets, ED, and hospital outcomes. Although response time was not the primary outcome measure, the percentage responded in less than or equal to five minutes to immediately life-threatening patients in metro communities increased because of freed up advanced life support resources to respond to higher-acuity and clinically complex cases.
This was an observational study that could demonstrate associations and concomitant conclusions, but not causation. It is a pre- and post-design based on multiple data linkage points. Potential data quality issues included failure to submit a completed patient care reports resulting in missing patient characteristics or failure to record a valid personal health number resulting in inability to linkage. No effort was made to impute missing data.
The study period coincided with the implementation of an ED-level data capture system (National Ambulatory Care Reporting System) in British Columbia. This resulted in differential availability of data in the pre- and post-periods (20 v. 29 hospitals, respectively), thus the same 20 urban and rural hospitals in both the pre- and post-periods were included in the analysis to reduce bias. Furthermore, a non-sampling error of this incomplete coverage could have caused bias in the mortality estimation. Exclusion of discharge abstract database record because of an unavailable National Ambulatory Care Reporting System record from the same case was made to restrict the analysis cohort and reduced the effect of such bias of only including the survived. It is unclear how many of the excluded cases were deceased to depict the overall impact on the patient outcome because of the restricted availability of data.
Interrupted time series was used to address the data availability issue by its ability to detect the predicted value of outcome variable at a certain time point, whether the impact of the revision is significant in terms of level change and trend change. Finally, the change in fire first responders post-resource allocation plan response was not immediately implemented in fall 2013; therefore, the analysis was solely focused on the paramedic response model and its effect on mortality.
This process and findings have implications and lessons for other EMS systems using MPDS® and associated response plans in their 911 response. The data and results suggest that EMS should utilize available patient outcome data to evaluate and optimize resource management.
CONCLUSION
In a population-based EMS system, a re-alignment of resource allocation was associated with, in the post-implementation phase, a decrease in 24-hour mortality overall and a significant decrease in 24-hour mortality in an immediately life-threatening patient cohort. This process and findings have implications and lessons for other EMS systems using MPDS® and associated resource allocation and response plans in their primary 911 response.
Supplementary material
The supplementary material for this article can be found at https://doi.org/10.1017/cem.2019.429.
Acknowledgements
We would like to thank Randy Slemko and Recep Gezer for assistance with data acquisition and comments that greatly improved the manuscript; Cynthia N. Lank for editorial assistance; Douglas Andrusiek for his support; and Dr. Rob Stenstrom for his statistical expertise contribution.
Competing interests
None.