Book contents
- Frontmatter
- Dedication
- Contents
- Preface
- Acknowledgments
- Notation
- 1 Introduction
- 2 Node Domain Processing
- 3 Graph Signal Frequency – Spectral Graph Theory
- 4 Sampling
- 5 Graph Signal Representations
- 6 How to Choose a Graph
- 7 Applications
- Appendix A Linear Algebra and Signal Representations
- Appendix B GSP with Matlab: The GraSP Toolbox
- References
- Index
- References
References
Published online by Cambridge University Press: 09 May 2022
- Frontmatter
- Dedication
- Contents
- Preface
- Acknowledgments
- Notation
- 1 Introduction
- 2 Node Domain Processing
- 3 Graph Signal Frequency – Spectral Graph Theory
- 4 Sampling
- 5 Graph Signal Representations
- 6 How to Choose a Graph
- 7 Applications
- Appendix A Linear Algebra and Signal Representations
- Appendix B GSP with Matlab: The GraSP Toolbox
- References
- Index
- References
Summary
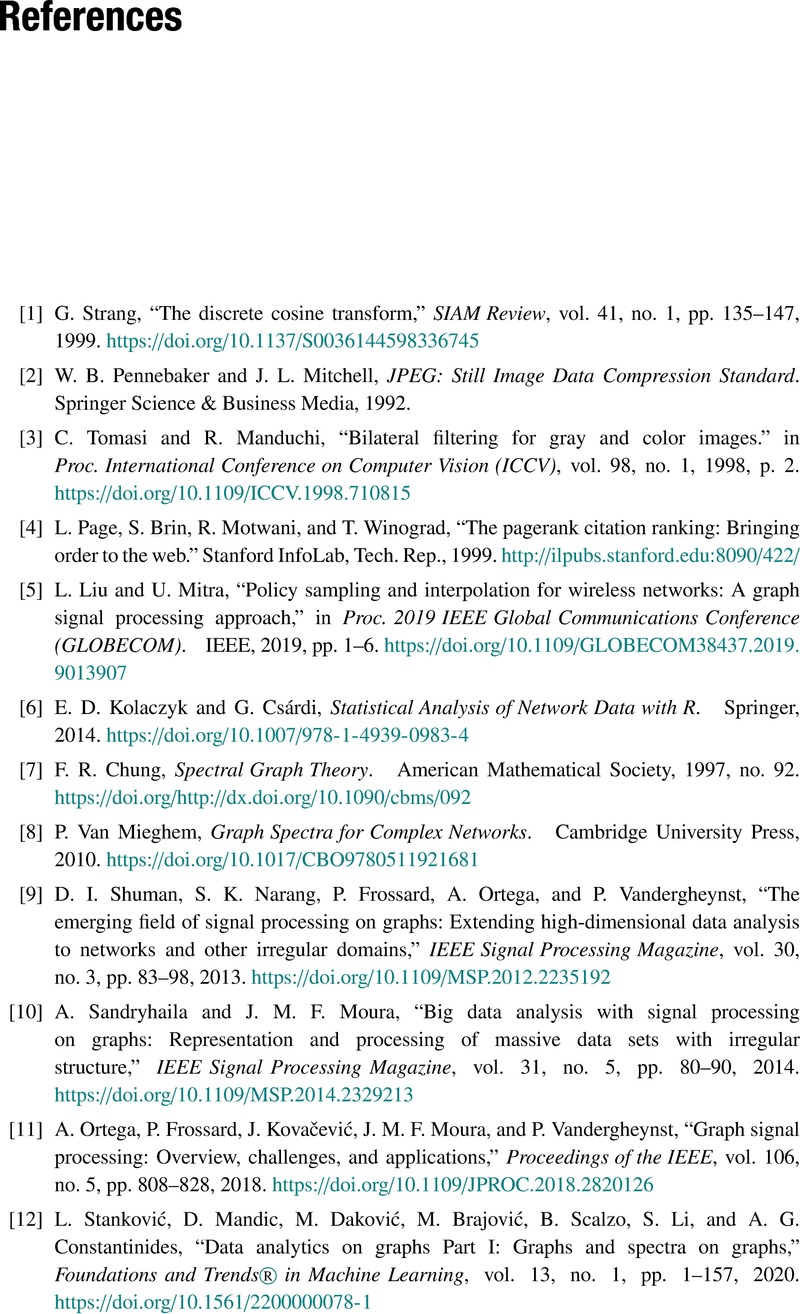
- Type
- Chapter
- Information
- Introduction to Graph Signal Processing , pp. 286 - 296Publisher: Cambridge University PressPrint publication year: 2022