No CrossRef data available.
Article contents
Towards Machine Vision-enabled STEM EELS for High-throughput Quantification of Grain Boundary Electronic Structure
Published online by Cambridge University Press: 30 July 2020
Abstract
An abstract is not available for this content so a preview has been provided. As you have access to this content, a full PDF is available via the ‘Save PDF’ action button.
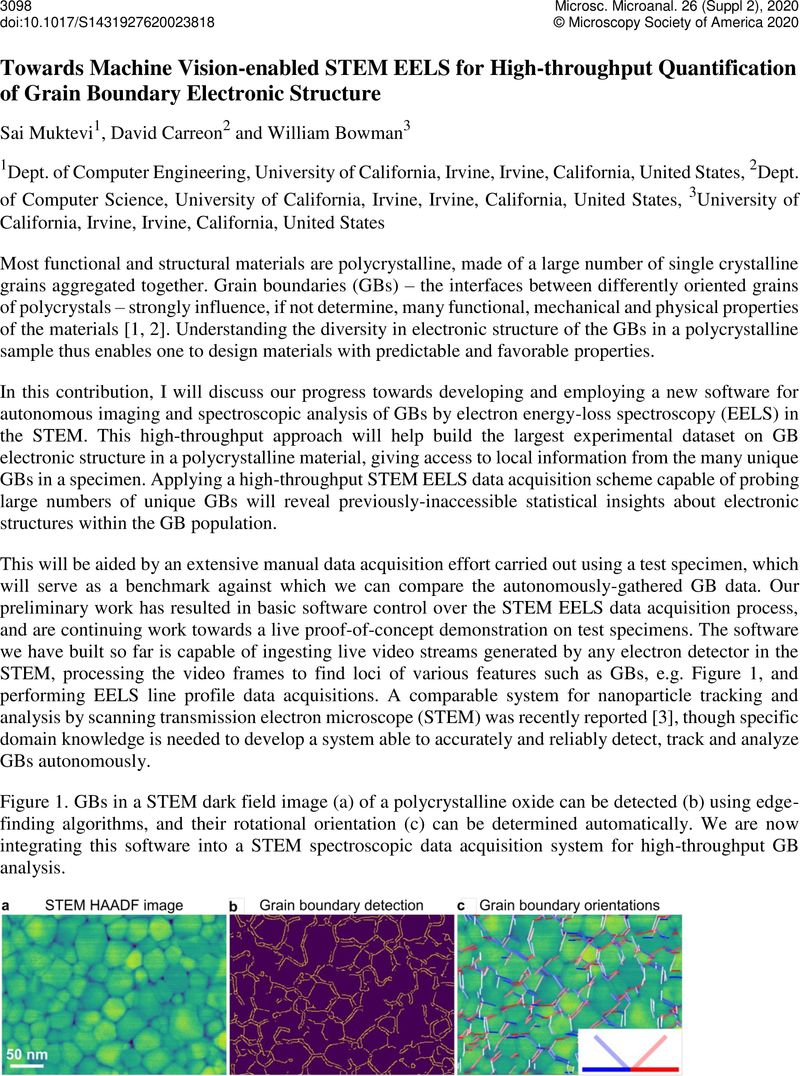
- Type
- Advances in Modeling, Simulation, and Artificial Intelligence in Microscopy and Microanalysis for Physical and Biological Systems
- Information
- Copyright
- Copyright © Microscopy Society of America 2020
References
Bowman, WJ, Kelly, MN, Rohrer, GS, Hernandez, CA, Crozier, PA (2017) Enhanced ionic conductivity in electroceramics by nanoscale enrichment of grain boundaries with high solute concentration. Nanoscale, 9:17293–17302. https://doi.org/10.1039/C7NR06941CCrossRefGoogle ScholarPubMed
Bowman, WJ, Darbal, A, Crozier, PA (2019) Linking Macroscopic and Nanoscopic Ionic Conductivity: A Semiempirical Framework for Characterizing Grain Boundary Conductivity in Polycrystalline Ceramics. ACS Applied Materials & Interfaces, https://doi.org/10.1021/acsami.9b15933CrossRefGoogle Scholar
Uusimaeki, T, Wagner, T, Lipinski, H-G, Kaegi, R (2019) AutoEM: a software for automated acquisition and analysis of nanoparticles. Journal of Nanoparticle Research, 21(6):122. https://doi.org/10.1007/s11051-019-4555-9CrossRefGoogle Scholar