Introduction
Growing evidence demonstrates that childhood trauma (CT; emotional/physical/sexual abuse, or emotional/physical neglect before the age of 18) is a potent risk factor for developing depressive and anxiety disorders across the lifespan (Lippard & Nemeroff, Reference Lippard and Nemeroff2020; Teicher & Samson, Reference Teicher and Samson2013). Studies have consistently shown CT associations with increased disease prevalence, earlier age at onset, greater illness severity, persistence, and comorbidity (Hovens, Giltay, Spinhoven, van Hemert, & Penninx, Reference Hovens, Giltay, Spinhoven, van Hemert and Penninx2015; Hovens et al., Reference Hovens, Giltay, Wiersma, Spinhoven, Penninx and Zitman2012; Nanni, Uher, & Danese, Reference Nanni, Uher and Danese2012; Nelson, Klumparendt, Doebler, & Ehring, Reference Nelson, Klumparendt, Doebler and Ehring2017). For instance, meta-analytic findings suggest that adults with a history of CT are two to three times more likely to develop depression or anxiety as well as twice more likely to develop a more persistent/chronic course of the disorder (Li, D'Arcy, & Meng, Reference Li, D'Arcy and Meng2016; Nanni et al., Reference Nanni, Uher and Danese2012; Nelson et al., Reference Nelson, Klumparendt, Doebler and Ehring2017; Norman et al., Reference Norman, Byambaa, De, Butchart, Scott and Vos2012). A recent meta-analysis of quasi-experimental studies designed to disentangle the causal effect of CT also revealed a significant causal relationship between CT and psychopathology (Baldwin et al., Reference Baldwin, Wang, Karwatowska, Schoeler, Tsaligopoulou, Munafo and Pingault2023). Hence, CT results in a substantial societal and economic burden, costing at least $428 billion annually in the USA alone (Peterson, Florence, & Klevens, Reference Peterson, Florence and Klevens2018).
Pathways through which CT adversely impacts (mental) health outcomes are complex and heterogeneous, probably involving multiple mechanisms in the brain, mind, and body (Danese & McEwen, Reference Danese and McEwen2012; Jaffee, Reference Jaffee2017; Kuzminskaite et al., Reference Kuzminskaite, Penninx, van Harmelen, Elzinga, Hovens and Vinkers2021). However, affective dysregulation could be one of the psychological CT mechanisms, at least partially explaining the vulnerability to psychopathology later in life (Dvir, Ford, Hill, & Frazier, Reference Dvir, Ford, Hill and Frazier2014; Messman-Moore & Bhuptani, Reference Messman-Moore and Bhuptani2017; Weissman et al., Reference Weissman, Bitran, Miller, Schaefer, Sheridan and McLaughlin2019). Indeed, individuals with a history of CT are more often characterized by various structural and functional brain alterations involved in emotion regulation and self-reflecting processing, higher levels of maladaptive personality characteristics such as high neuroticism, and more negative cognitive schemas and self-associations, likely leading to enhanced stress sensitivity in everyday life (Gibb, Reference Gibb2002; Hovens, Giltay, van Hemert, & Penninx, Reference Hovens, Giltay, van Hemert and Penninx2016; Kuzminskaite et al., Reference Kuzminskaite, Penninx, van Harmelen, Elzinga, Hovens and Vinkers2021; McCrory, Foulkes, & Viding, Reference McCrory, Foulkes and Viding2022; van Harmelen et al., Reference van Harmelen, de Jong, Glashouwer, Spinhoven, Penninx and Elzinga2010). In fact, adults with CT seem to respond more strongly to perceived rejection, criticism, or neglect in daily situational contexts as indicated by significantly more reports of minor (daily hassles) and major stressful life events (Gerin et al., Reference Gerin, Viding, Pingault, Puetz, Knodt, Radtke and McCrory2019; Uhrlass & Gibb, Reference Uhrlass and Gibb2007; van Harmelen et al., Reference van Harmelen, van Tol, Dalgleish, van der Wee, Veltman, Aleman and Elzinga2014).
In recent years, ecological momentary assessment (EMA) has gained popularity in measuring affective dysregulation. By repeatedly assessing current affect states, EMA reduces recall bias and provides a vast amount of data to monitor not only affect intensity (mean levels), but also its dynamics (e.g. variability, autocorrelation [commonly referred to as inertia/persistence], instability) in daily life (Ebner-Priemer & Trull, Reference Ebner-Priemer and Trull2009; Houben, van den Noortgate, & Kuppens, Reference Houben, van den Noortgate and Kuppens2015; Shiffman, Stone, & Hufford, Reference Shiffman, Stone and Hufford2008; Trull, Lane, Koval, & Ebner-Priemer, Reference Trull, Lane, Koval and Ebner-Priemer2015). In fact, emerging evidence underscores the importance of examining how various risk factors are uniquely associated with the dynamics, rather than just the mean levels, of health outcomes (Bann, Wright, & Cole, Reference Bann, Wright and Cole2022). While variability refers to affect fluctuations from a person's average affect level, commonly quantified by the within-person-variance (WPV) across time, autocorrelation refers to affect persistence over time and is quantified by lag-1 autocorrelation within affect measures (Koval, Pe, Meers, & Kuppens, Reference Koval, Pe, Meers and Kuppens2013; Trull et al., Reference Trull, Lane, Koval and Ebner-Priemer2015). Instability mainly depends on variability and/or autocorrelation and describes changes in affect from one moment to the next, typically quantified by the root mean square of successive differences (RMSSD) of affect measures (Jahng, Wood, & Trull, Reference Jahng, Wood and Trull2008; Koval et al., Reference Koval, Pe, Meers and Kuppens2013; Trull et al., Reference Trull, Lane, Koval and Ebner-Priemer2015). Increased NA levels, decreased positive affect (PA) levels, greater affect variability, autocorrelation, and instability may indicate emotional dysregulation and subsequent vulnerability to psychopathology, especially mood and anxiety disorders (Houben et al., Reference Houben, van den Noortgate and Kuppens2015; Lamers et al., Reference Lamers, Swendsen, Cui, Husky, Johns, Zipunnikov and Merikangas2018; Schoevers et al., Reference Schoevers, van Borkulo, Lamers, Servaas, Bastiaansen, Beekman and Riese2021; Thompson, Berenbaum, & Bredemeier, Reference Thompson, Berenbaum and Bredemeier2011). In line, emerging EMA evidence suggests that a history of CT is associated with decreased PA levels (Brick, Nugent, & Armey, Reference Brick, Nugent and Armey2021; Yaroslavsky, Bush, & France, Reference Yaroslavsky, Bush and France2022) and increased NA levels in the context of depression, anxiety, psychosis, somatic complaints, or suicidality (Glaser, van Os, Portegijs, & Myin-Germeys, Reference Glaser, van Os, Portegijs and Myin-Germeys2006; Paetzold et al., Reference Paetzold, Myin-Germeys, Schick, Nelson, Velthorst, Schirmbeck and Reininghaus2021; Rauschenberg et al., Reference Rauschenberg, van Os, Cremers, Goedhart, Schieveld and Reininghaus2017; Reininghaus et al., Reference Reininghaus, Gayer-Anderson, Valmaggia, Kempton, Calem, Onyejiaka and Morgan2016; Wichers et al., Reference Wichers, Schrijvers, Geschwind, Jacobs, Myin-Germeys, Thiery and van Os2009; Yaroslavsky et al., Reference Yaroslavsky, Bush and France2022). Findings on affect dynamics in individuals exposed to CT are limited, but suggest increased variability of PA and persistence of NA in young adults with and without a history of major depressive or anxiety disorders (Teicher, Ohashi, Lowen, Polcari, & Fitzmaurice, Reference Teicher, Ohashi, Lowen, Polcari and Fitzmaurice2015). Nevertheless, conflicting or null findings concerning separate momentary affect markers have also been reported (Brick et al., Reference Brick, Nugent and Armey2021; Teicher et al., Reference Teicher, Ohashi, Lowen, Polcari and Fitzmaurice2015).
Most of the inconsistencies in findings on affect dynamics could be explained by methodological heterogeneity, especially in considering mean affect levels (i.e. how individuals generally feel). Many existing studies do not consider if affect dynamics add unique value above and beyond the mean affect levels, restricting interpretation and potentially leading to incorrect conclusions. This is especially important in the context of NA, where separate NA markers are tightly interconnected due to the floor effect ̶ healthy individuals generally experience low NA, limiting the amount of variability observed (Mestdagh et al., Reference Mestdagh, Pe, Pestman, Verdonck, Kuppens and Tuerlinckx2018). In fact, a meta-analysis showed that psychological well-being indicators (life satisfaction, depressive and borderline symptoms) were not associated with affect dynamics above and beyond the mean affect levels in adulthood (Dejonckheere et al., Reference Dejonckheere, Mestdagh, Houben, Rutten, Sels, Kuppens and Tuerlinckx2019). Similarly, Brick et al. (Reference Brick, Nugent and Armey2021) found no association between CT and PA or NA WPV when adjusting for within-person mean. Some evidence is also emerging on different interpretations of dynamic affect fluctuations as being adaptive or maladaptive based on moderation by mean affect levels (Maher et al., Reference Maher, Ra, Leventhal, Hedeker, Huh, Chou and Dunton2018). For instance, for individuals with lower mean PA levels, lower PA variability might indicate a chronic affect state without momentary daily uplifts, consequently increasing the risk of depressive and anxiety symptoms (Maher et al., Reference Maher, Ra, Leventhal, Hedeker, Huh, Chou and Dunton2018). Hence, it is essential to explore the role of mean affect level to understand if affect dynamics carry unique value and function differently across individuals.
Despite mounting evidence, relatively few EMA studies comprehensively examined CT associations with momentary affect dynamics, limiting the understanding of long-lasting CT effects necessary to identify at-risk individuals and develop personalized interventions. More research is also needed to understand whether specific affect dysregulations vary based on CT severity and type. Results on CT severity generally indicate a dose-response association, with higher CT levels showing the most detrimental effects (Rauschenberg et al., Reference Rauschenberg, van Os, Cremers, Goedhart, Schieveld and Reininghaus2017; Reininghaus et al., Reference Reininghaus, Gayer-Anderson, Valmaggia, Kempton, Calem, Onyejiaka and Morgan2016; Teicher et al., Reference Teicher, Ohashi, Lowen, Polcari and Fitzmaurice2015; Wichers et al., Reference Wichers, Schrijvers, Geschwind, Jacobs, Myin-Germeys, Thiery and van Os2009). However, this has been most often examined with affect intensity and not affect dynamics measures. Findings on the effects of different CT types have been inconsistent, with some studies suggesting the largest daily NA levels for sexual abuse (Reininghaus et al., Reference Reininghaus, Gayer-Anderson, Valmaggia, Kempton, Calem, Onyejiaka and Morgan2016), but opposite findings suggesting the strongest effects for emotional and/or physical abuse have also been observed (Rauschenberg et al., Reference Rauschenberg, van Os, Cremers, Goedhart, Schieveld and Reininghaus2017; Wonderlich et al., Reference Wonderlich, Rosenfeldt, Crosby, Mitchell, Engel, Smyth and Miltenberger2007). Therefore, the current study investigated whether individuals with CT (with and without lifetime depressive and/or anxiety disorder) show dynamic affect dysregulation (greater variability, autocorrelation, and instability) above and beyond the mean affect levels compared to individuals without CT (including the differentiation among CT severity and types), using two-week follow-up EMA data from the Netherlands Study of Depression and Anxiety EMA & Actigraphy sub-study (NESDA-EMAA) (Penninx et al., Reference Penninx, Eikelenboom, Giltay, van Hemert, Riese, Schoevers and Beekman2021). In our primary analyses, we did not take psychiatric status into account because previous findings showed that the impact of CT on affect dynamics was similar in individuals with and without a history of major depression (Teicher et al., Reference Teicher, Ohashi, Lowen, Polcari and Fitzmaurice2015). Given the limited evidence on mean affect level as a moderator in the relation between CT and affect dynamics, the moderation analyses were exploratory.
Methods
Sample
Data came from a sub-study of an ongoing longitudinal cohort (The Netherlands Study of Depression and Anxiety, NESDA) (Penninx et al., Reference Penninx, Eikelenboom, Giltay, van Hemert, Riese, Schoevers and Beekman2021). Participants (n = 2981) were Dutch-fluent adults (18–65 years) with a current or remitted depressive and/or anxiety disorder (78%, Composite International Diagnostic Interview, CIDI version 2.1) (Robins et al., Reference Robins, Wing, Wittchen, Helzer, Babor, Burke and Towle1988; Wittchen, Reference Wittchen1994) and healthy controls (22%) recruited for the baseline assessment between September 2004 and February 2007 from three different settings: community, primary health care, and specialized mental health care. Interviews to determine current (past 6-month) and/or remitted (lifetime, but not past 6-month) depressive and/or anxiety disorder were conducted by trained clinical research staff. Patients with a primary diagnosis other than depressive/anxiety disorder (e.g. post-traumatic stress disorder, bipolar disorder, psychotic disorder, obsessive-compulsive disorder) were excluded. Participants were assessed on various sociodemographic, lifestyle, (mental) health, and biological factors and were followed up for the fifth time nine years after the baseline assessment (2014–2017, n = 2143, also including 367 siblings). At this time, 384 participants enrolled in the NESDA-EMAA sub-study. Specific enrollment details and flowcharts were previously described in Difrancesco et al. (Reference Difrancesco, Lamers, Riese, Merikangas, Beekman, van Hemert and Penninx2019); Schoevers et al. (Reference Schoevers, van Borkulo, Lamers, Servaas, Bastiaansen, Beekman and Riese2021). NESDA study, including the EMAA sub-study, was approved by the Medical Ethics Review Board of Amsterdam University Medical Center Vrije Universiteit location (reference number 2003/183) and by the ethical review board of each participating research center in Amsterdam, Leiden, and Groningen. All participants provided written informed consent.
After the 9-year interview, NESDA participants who were eligible and willing to participate in the NESDA-EMAA sub-study were invited to one of the research facilities within one month. For this study, NESDA participants were invited if they participated in at least two of the previous NESDA waves, consented to be approached for this sub-study, participated in the regular interview ⩽31 days prior to starting the EMA measurements, had a good mastery of the Dutch language, were familiar with smartphone use and willing to wear a wrist-worn actigraphy device. Siblings were invited if they did not have a current or past diagnosis of a depressive/anxiety disorder or another severe psychiatric disorder (e.g. psychotic or severe addiction disorder). A total of 384 participants were eligible and enrolled. Participants of the NESDA-EMAA study were asked to fill out the EMA assessments, an electronic diary on their smartphone, and to wear a wrist-worn actigraphy device (GENEActiv, Activinsights Ltd, Kimbolton, UK) daily for two weeks (see Difrancesco et al. (Reference Difrancesco, Lamers, Riese, Merikangas, Beekman, van Hemert and Penninx2019) for details). If participants did not possess a smartphone or their phone was not suitable for participation (e.g. no internet bundle), a smartphone was provided for the duration of the study (n = 107, 27.9%). Participants of the EMA assessment completed a set of 31 items (Kirtley et al., Reference Kirtley, Hiekkaranta, Kunkels, Verhoeven, van Nierop and Myin-Germeys2019, April 2) on affect states, activities, lifestyle, and context five times per day (every 3 h; fixed design). The timing of assessments was personalized to fit participants' natural wake-sleep rhythm during the week and weekend; the first daily assessment was aimed to be 1–2 h after waking up in the morning. Participants were instructed to complete questionnaires as soon as possible after receiving the text message (beep), preferably within 15 min, but at least within 60 min. A reminder was sent after 30 min.
Measures
Childhood trauma
CT assessment was based on the Childhood Trauma Interview (CTI) conducted at the baseline NESDA assessment by trained research staff (de Graaf, Bijl, ten Have, Beekman, & Vollebergh, Reference de Graaf, Bijl, ten Have, Beekman and Vollebergh2004). The CTI is a structured interview retrospectively assessing four CT types before the age of 16 years: emotional neglect, emotional abuse, physical abuse, and sexual abuse. Each CT type is answered as ‘no’ or ‘yes’ with a further frequency indication as (0) - ‘never’, (1) - ‘once or sometimes’, and (2) - ‘regularly, often, or very often’ (score range 0–2). A continuous cumulative CT severity score (range 0–8) is often calculated as a sum of the number of types and frequency of CT, with a higher score indicating more severe CT (Hovens et al., Reference Hovens, Wiersma, Giltay, van Oppen, Spinhoven, Penninx and Zitman2010; Wiersma et al., Reference Wiersma, Hovens, van Oppen, Giltay, van Schaik, Beekman and Penninx2009). In the present study, CT was analyzed dichotomously in four ways: (i) present (experienced once, sometimes, regularly, often, or very often) v. absent (no experience of CT); (ii) severe CT (CTI score 4–8) v. no CT (CTI score 0); (iii) mild CT (CTI score 1–3) v. no CT; and (iv) each CT type (emotional neglect, emotional abuse, physical abuse, or sexual abuse experienced once, sometimes, regularly, often, or very often) referenced to no experience of CT (analyzed in separate models). The CTI has been shown to have good psychometric properties (Fink, Bernstein, Handelsman, Foote, & Lovejoy, Reference Fink, Bernstein, Handelsman, Foote and Lovejoy1995; Hovens et al., Reference Hovens, Giltay, Wiersma, Spinhoven, Penninx and Zitman2012; Spinhoven et al., Reference Spinhoven, Penninx, Hickendorff, van Hemert, Bernstein and Elzinga2014).
Positive and negative momentary affect states
Momentary affect states were assessed by the 13 items previously used in the Uncovering the Positive Potential of Emotional Reactivity study (Bennik, Reference Bennik2015). The items focus on positive and negative momentary affect states as well as low and high arousal dimensions: ‘at this moment, I feel satisfied’, ‘relaxed’, ‘upset’, ‘cheerful’, ‘irritated’, ‘listless ‘, ‘down’, ‘energetic’, ‘enthusiastic’, ‘nervous’, ‘bored’, ‘calm’, and ‘anxious’. Each item is rated on a 7-point Likert scale ranging from (1) - ‘not at all’ to (7) - ‘very much’ (score range 1–7). Mean levels of PA were calculated by averaging six PA items (satisfied, relaxed, cheerful, energetic, enthusiastic, and calm), while mean levels of NA were calculated by averaging seven NA items (upset, irritated, listless/apathetic, down, nervous, bored, anxious) (Schoevers et al., Reference Schoevers, van Borkulo, Lamers, Servaas, Bastiaansen, Beekman and Riese2021) at each timepoint (Cronbach's α range = 0.87–0.92 and 0.81–0.89 for PA and NA items, respectively). Affect variability, referring to affect fluctuations from a person's average affect level over time, was quantified as the WPV across observations (var function, R stats package (R Core Team, 2020)). When variability is high, affect scores fluctuate significantly from the within-person mean. Autocorrelation, also known as affect persistence, was quantified by lag-1 autocorrelation between affect measures (acf function, R stats package (R Core Team, 2020)). High autocorrelation means that affect deviations from the mean tend to persist across consecutive measurements, yielding a higher correlation between consecutive measurements (slower return to the mean after perturbations). Instability, which largely depends on variability and/or autocorrelation and describes changes in affect from one moment to the next, was quantified by RMSSD of affect assessments (rmssd function, R varian package (Wiley & Elkhart Group Limited, 2016)). To account for nights in dynamic affect fluctuation calculations, an additional sixth observation at the end of each day was included for each participant and marked as missing in the analyses. Because affect instability was very strongly correlated with affect variability in our study (PA: r = 0.911, NA: r = 0.910, p-values <0.001) and, thus, mainly reflected variability, it was removed from further analyses (main CT associations with affect instability can be found in online Supplementary Table S1). Visualization of two examined affect components (variability and autocorrelation) based on NESDA-EMA raw PA participant data over the two-week observations is presented in Fig. 1.
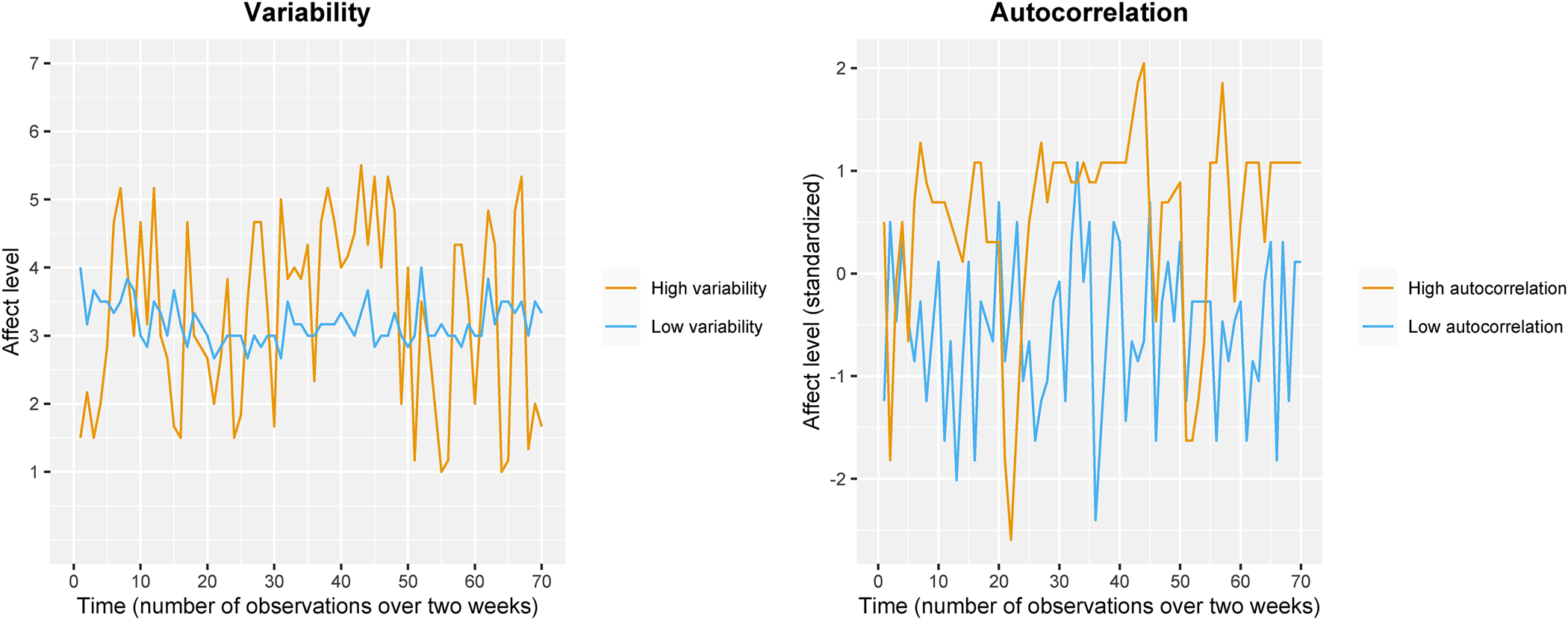
Figure 1. Visualization of two components of affect fluctuation - variability and autocorrelation, based on NESDA-EMA positive affect (PA) participant data (n = 2) over the two-week observations. Note: Individuals were selected to clearly demonstrate the concepts of variability and autocorrelation; different individuals were selected for both graphs.
Variability is quantified by within-person variance, while autocorrelation is quantified by lag-1 autocorrelation of affect measures. High variability (left side; orange line) means that affect scores fluctuate significantly from the within-person mean, whereas low variability (left side; blue line) means that affect scores tend to be close to the within-person mean. High autocorrelation (right side; orange line) means that deviations from the mean tend to persist across consecutive measurements, yielding a higher correlation between consecutive measurements, whereas low autocorrelation (right side; blue line) means that deviations from the mean tend to be resolved quickly, yielding a lower correlation between consecutive measurements.
Clinical characteristics
DSM-IV-based diagnoses of depressive (major depressive disorder, dysthymia) and anxiety disorders (social anxiety disorder, agoraphobia, generalized anxiety disorder, panic disorder with or without agoraphobia) were established with the CIDI (version 2.1) (Robins et al., Reference Robins, Wing, Wittchen, Helzer, Babor, Burke and Towle1988). Participants were divided into three groups: current disorder (past 6-month), remitted disorder (lifetime, but not past 6-month), and healthy controls (no lifetime depressive/anxiety disorder). The severity of depressive and anxiety symptomatology was assessed using well-validated self-reported scales: 30-item Inventory of Depressive Symptomatology (IDS; score range 0–84) (Rush, Gullion, Basco, Jarrett, & Trivedi, Reference Rush, Gullion, Basco, Jarrett and Trivedi1996) and 21-item Beck Anxiety Inventory (BAI; score range 0–63) (Beck, Epstein, Brown, & Steer, Reference Beck, Epstein, Brown and Steer1988). Antidepressant medication use was considered present when taken for at least one month 50% of the time and included tricyclic antidepressants (TCAs) (anatomical therapeutic chemical (ATC) code N06AA), selective serotonin reuptake inhibitors (SSRIs) (ATC code N06AB), and other types (ATC code: N06AX, N06AG, N06AF, not N06AA, not N06AB; no/yes frequent use). See supplement for correlations between antidepressant use and main study variables.
Covariates
Sociodemographic covariates included age in years, sex, and years of education at the time of NESDA-EMAA sub-study (see online Supplementary Table S2 for correlations between sociodemographic and main study variables). Because dynamic affect fluctuation is tightly linked to the mean affect level (Dejonckheere et al., Reference Dejonckheere, Mestdagh, Houben, Rutten, Sels, Kuppens and Tuerlinckx2019; Mestdagh et al., Reference Mestdagh, Pe, Pestman, Verdonck, Kuppens and Tuerlinckx2018), analyses were further adjusted for mean levels of affect throughout two weeks.
Data cleaning
EMA data were checked and excluded based on the following reasons: technical issues (e.g. server down), uploading a questionnaire after 65 min, and a response rate below 50% (see Schoevers et al. (Reference Schoevers, van Borkulo, Lamers, Servaas, Bastiaansen, Beekman and Riese2021) for details on data cleaning). With the additional exclusion of participants with missing CT data, 346 unique participants with 22 449 observations (Mtwo−week = 64.88, s.d. = 5.01; Mday = 4.66, s.d. = 0.68) were available for analysis (current n = 98, remitted n = 174, or no depressive/anxiety disorder n = 74). There was no issue with clustering participants with siblings, as no siblings had available CT data and were excluded.
Data-analyses
Sample characteristics were explored descriptively and presented as means with standard deviations (s.d.), medians with interquartile ranges (for non-normally distributed variables), or numbers with percentages across CT status groups. Independent-samples t test (for continuous variables), Wilcoxon rank test (for non-normally distributed continuous variables), and Chi-squared test (for categorical variables) were used to examine significant differences in characteristics between the groups. For further analyses, skewed outcome variables (assessed by values of skewness and kurtosis as well as QQ plots) were naturally loge-transformed (ln(x + 1) for outcomes including zero) and rechecked for normal distribution. Pearson correlation (r) was used to determine intercorrelations between affect variables (weak correlation = 0.1–0.3, moderate correlation = 0.3–0.5, strong correlation = 0.5–1.0) (Benesty, Chen, Huang, & Cohen, Reference Benesty, Chen, Huang and Cohen2009).
To examine CT associations with dynamic affect fluctuation variables, we performed multiple linear regression analyses with CT as predictor and affect as outcome variables. CT was first examined as a dichotomous variable (yes v. no), followed by categorical division based on CT severity (mild v. no; severe v. no), and the dichotomized division based on the four CT types. All CT types were examined in separate models with no CT as a reference group. Separate analyses were conducted for distinct affect variables. All analyses were adjusted for baseline sociodemographic factors (age, sex, and years of education; Model 1). To check if main findings between CT and affect fluctuations could be driven by mean affect levels, we additionally included mean PA and NA levels throughout the two weeks as covariates (Model 2). To further check if main associations could be moderated by psychiatric status, we additionally included CT × lifetime disorder (lifetime v. no lifetime disorder) interaction term. To check if main associations could be moderated by the mean level of affect, we additionally included CT × mean affect level interaction term (exploratory analysis). All interaction models included main effects of separate interaction variables. Analytical steps of the main analysis are visually presented in online Supplementary Figure S1.
Cohen's d (small effect = 0.2, medium effect = 0.5, large effect = 0.8) was calculated as a measure of between-group effect size (Cohen, Reference Cohen1988). For all analyses, the statistical significance was based on a p-value <0.05. Benjamini-Hochberg False Discovery Rate (FDR p-value <0.05) corrected for multiple analyses (Benjamini & Hochberg, Reference Benjamini and Hochberg1995). All analyses were performed in R Studio 1.3.959 software (R Core Team, 2020).
Results
Sample characteristics
The mean age of the sample (n = 346) was 49.25 (s.d. = 12.55), and the majority was female (67.0%; Table 1). Half of the sample (50.3%) had remitted depressive/anxiety disorder, 28.3% had a current (past 6-month) diagnosis, and 21.4% were healthy controls. Approximately half of the participants reported experience of at least one type of CT (45.9%; mild, 26.0%; severe, 19.9%), with emotional neglect and emotional abuse being the most common (41.0% and 31.0%, respectively). Compared to individuals without CT (n = 187), those with CT (n = 159) were significantly older, less educated, had a higher prevalence of current depressive/anxiety disorder diagnosis, increased depressive and anxiety symptom severity, were more often antidepressant medication users, and reported lower two-week mean PA as well as higher mean NA scores (p-values <0.05).
Table 1. Sample characteristics
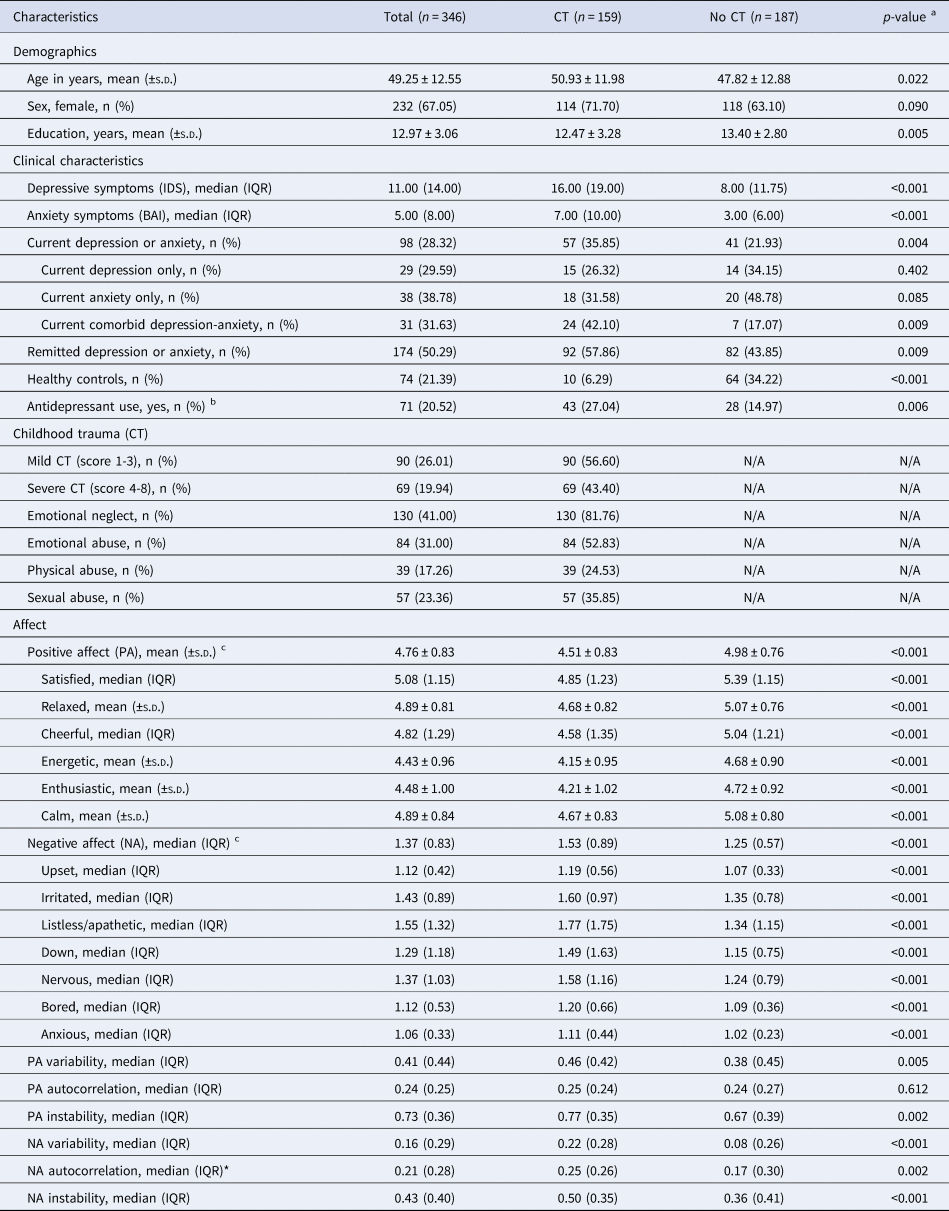
Note. BAI, Beck Anxiety Inventory; CT, childhood trauma; IDS, Inventory of Depressive Symptomatology; IQR, interquartile range; N/A, not applicable; SD, standard deviation. Bolded indicates significance at p < 0.05. All significant associations survived multiple testing (FDR p-value < 0.05) adjustment (33 tests).
a p-value for Independent-samples t test (continuous variables), Chi-squared test (categorical variables), or Wilcoxon rank sum test (non-normally distributed continuous variables).
b Frequent use of tricyclic antidepressants (TCAs; anatomical therapeutic chemical (ATC) code N06AA), selective serotonin reuptake inhibitors (SSRIs; ATC code N06AB), or other antidepressant types (ATC code: N06AX, N06AG, N06AF).
c Two-week mean.
* Data missing for 1 participant.
Correlations (Table 2) between variability and autocorrelation were positive and ranged from weak to moderate (r = 0.292 and 0.485 for PA and NA, respectively, p-values <0.001). Participants with lower PA tended to have higher PA variability (r = −0.403, p < 0.001) and PA autocorrelation (r = −0.154, p < 0.01), while participants with higher NA tended to have higher NA variability (r = 0.712, p < 0.001) and NA autocorrelation (r = 0.434, p < 0.001).
Table 2. Pearson r correlations (r) between PA and NA variables (n = 346)
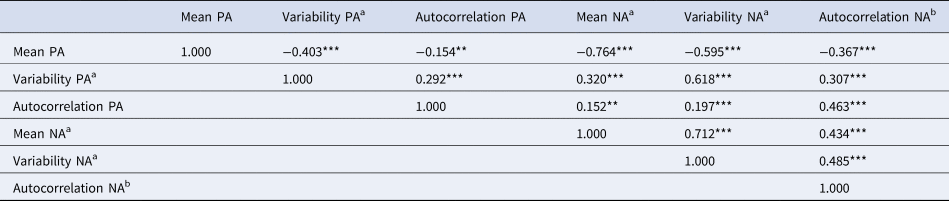
Note: ***p < 0.001, ** p < 0.01, * p < 0.05, a – loge-transformed (ln), b data missing for 1 participant; all significant associations survived multiple testing (FDR p-value < 0.05) adjustment (15 tests). PA, positive affect; NA, negative affect.
Associations between CT and affect states
Individuals with CT compared to those without CT reported significantly lower levels of PA (B = −0.490, s.e. = 0.087, p < 0.001, d = −0.620; adjusted for sociodemographic factors) and higher levels of NA (B = 0.189, s.e. = 0.034, p < 0.001, d = 0.556; adjusted for sociodemographic factors) throughout the two weeks (see online Supplementary Figure S2 for visualization of the difference between mean PA and NA levels over the two-week observations by CT status). Having a lifetime depressive and/or anxiety disorder diagnosis did not explain these associations (B = −0.311, s.e. = 0.088, p < 0.001, d = −0.383 and B = 0.117, s.e. = 0.038, p = 0.002, d = 0.337 for PA and NA, respectively; additional adjustment for psychiatric status).
Associations with dynamic affect variables (Table 3, Model 1) showed that CT was associated with significantly greater PA variability (B = 0.246, s.e. = 0.079, p = 0.002, d = 0.336), NA variability (B = 0.064, s.e. = 0.020, p = 0.001, d = 0.353), and NA autocorrelation (B = 0.060, s.e. = 0.022, p = 0.006, d = 0.308). No association with PA autocorrelation was observed (B = 0.004, s.e. = 0.020, p = 0.839, d = 0.022). Participants with mild and severe CT compared to those without CT showed higher scores of PA and NA variability as well as NA autocorrelation (B = 0.049 to 0.196 and B = 0.064 to 0.315, p-values <0.05 for mild and severe CT, respectively). All associations remained significant after multiple comparison adjustment. Standardized between-group effect sizes (Fig. 2) for significant associations ranged from small to medium (d = 0.308 to 0.353), with the most potent effects for severe CT cases (d = 0.328 to 0.471). However, after additional adjustment for mean levels of affect (Table 3, model 2), effects were vastly reduced, and all significant associations disappeared (p-values >0.05). No significant CT × lifetime disorder interactions were observed, suggesting potential consistency of results between psychiatric status groups (online Supplementary Tables S3–S5; FDR p-values >0.05).
Table 3. Linear regression results on affect fluctuations based on CT severity
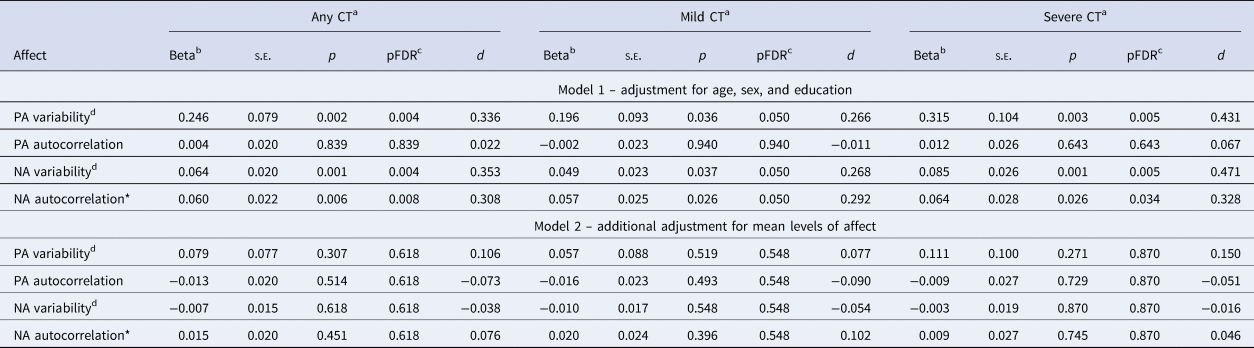
Note. CT (n = 159), mild CT (n = 90), severe CT (n = 69), no CT (n = 187).
aReference group: no CT; bUnstandardized beta; cmultiple testing (FDR p-value <0.05) adjustment for 4 tests per model; dLoge-transformed (ln); * Data missing for 1 participant. Boldface indicates statistical significance (p < 0.05). CT, childhood trauma; PA, positive affect; NA, negative affect, SE, standard error.
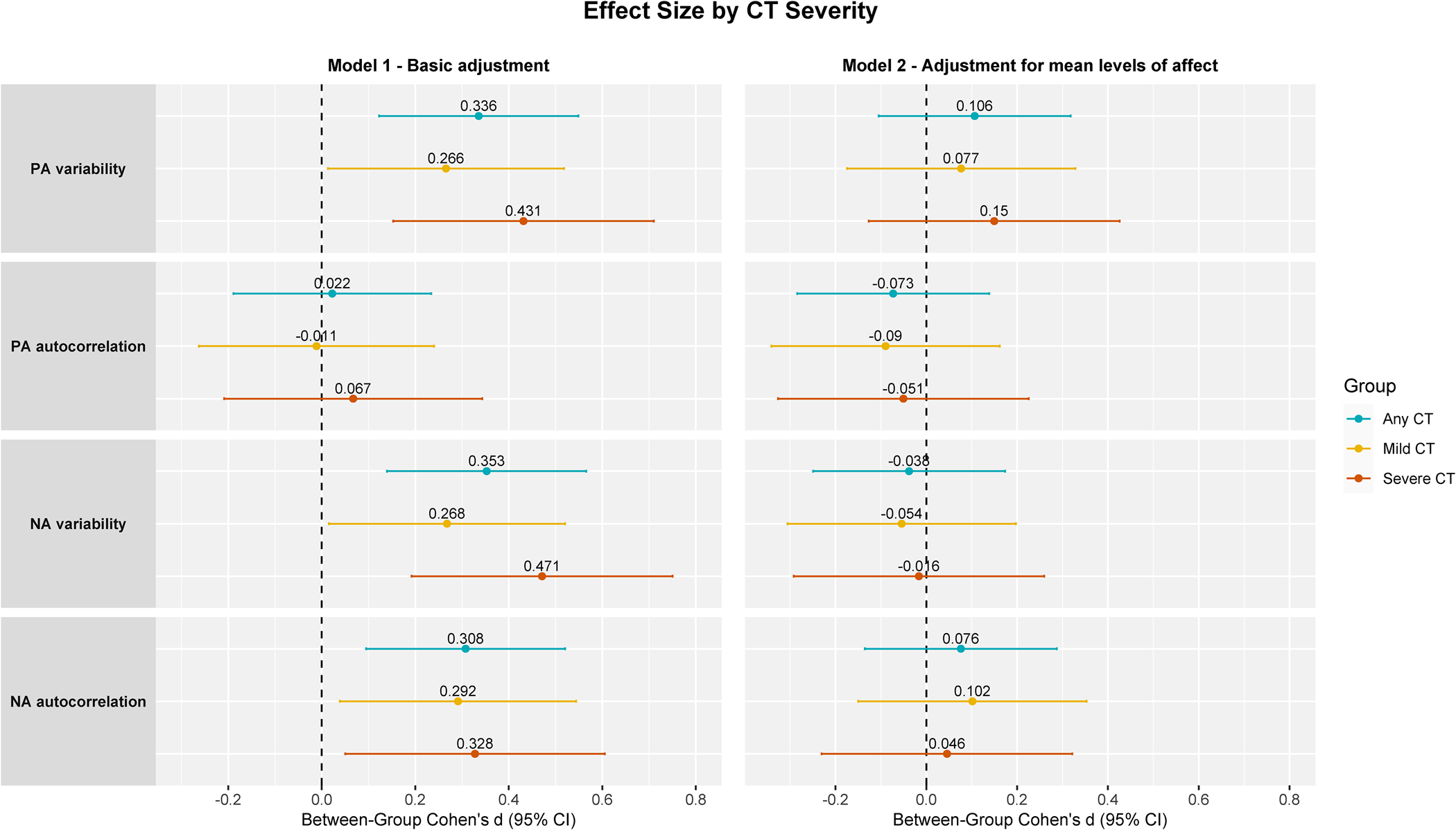
Figure 2. Forest plot of between group effect sizes with corresponding 95% confidence intervals (based on unstandardized regression coefficients) of affect fluctuations by CT severity (referenced to no CT group).
Note. Basic adjustment: age, sex, and education. CT types analyzed in separate models with no CT as reference group.
CT (n = 159), mild CT (n = 90), severe CT (n = 69), no CT (n = 187).
CT, childhood trauma; PA, positive affect; NA, negative affect
Out of CT types (online Supplementary Table S6 and Figure S3), emotional abuse and emotional neglect showed rather stronger and larger number of significant associations, with the smallest and not significant effects for sexual abuse (though results should be interpreted with caution due to the limited sample size). In line with the main findings, all significant associations disappeared after additional adjustment for the mean affect levels.
Exploratory moderation analysis by mean levels of affect
Mean levels of affect throughout the two weeks significantly moderated associations between CT and dynamic affect fluctuations (online Supplementary Table S7; online Supplementary Figure S4). Specifically, mean PA moderated the association of CT with PA variability (interaction: B = 0.304, s.e. = 0.090, p = 0.001), suggesting that among individuals with lower PA levels, CT was associated with lower PA variability and among individuals with higher PA levels, CT was associated with higher variability. Moreover, mean NA moderated the association of CT with NA variability (interaction: B = −0.151, s.e. = 0.041, p < 0.001) and NA autocorrelation (interaction: B = −0.228, s.e. = 0.056, p < 0.001), suggesting that among individuals with higher NA levels, CT was associated with lower NA variability/autocorrelation, and among individuals with lower NA levels, CT was associated with higher NA variability/autocorrelation. All significant interactions survived multiple comparison and psychiatric status adjustments. In a sensitivity analysis, the significant interactions persisted after excluding individuals without lifetime psychopathology (n = 74), who may have the largest ceiling and floor effects (online Supplementary Table S8).
Discussion
Whether the experience of CT impacts dynamic affect fluctuations during adult daily life has been limited and inconsistent. Our study comprehensively examined CT associations with two-week day-to-day affect fluctuations, consistently taking mean affect levels into account. Findings showed that compared to those without CT, individuals with CT reported lower mean PA levels and higher mean NA levels throughout the two weeks. CT was also linked to greater PA variability, NA variability, and NA autocorrelation, with small to medium effects most detrimental for severe CT cases. However, these associations largely depended on group differences in mean affect levels, suggesting that dynamic affect fluctuations in this study had no added value above the mean levels. Findings suggested consistency in adults with and without lifetime depressive/anxiety disorders, but further investigation is necessary to confirm these results. Sexual abuse showed the smallest effects, but effect sizes among CT types were rather comparable, with no relevant differences observed (95% CI around d largely overlapping). Exploratory moderation analysis suggested that associations between CT and affect dynamics varied based on mean affect levels: e.g. among those with lower PA levels, CT was associated with lower PA variability; among those with higher NA levels, CT was associated with lower NA variability and autocorrelation.
Decreased PA levels and increased NA levels in individuals with CT throughout the two weeks are largely in line with previous evidence of CT impacting mental health, e.g. in the context of depression, anxiety, psychosis, somatic complaints, or suicidality (Brick et al., Reference Brick, Nugent and Armey2021; Glaser et al., Reference Glaser, van Os, Portegijs and Myin-Germeys2006; Rauschenberg et al., Reference Rauschenberg, van Os, Cremers, Goedhart, Schieveld and Reininghaus2017; Reininghaus et al., Reference Reininghaus, Gayer-Anderson, Valmaggia, Kempton, Calem, Onyejiaka and Morgan2016; Yaroslavsky et al., Reference Yaroslavsky, Bush and France2022). CT associations with greater PA variability, NA variability, and NA (but not PA) autocorrelation are also consistent with earlier findings on CT associations with increased PA variability and NA (but not PA) persistence in young adults with and without a history of major depressive or anxiety disorders (Teicher et al., Reference Teicher, Ohashi, Lowen, Polcari and Fitzmaurice2015). Nevertheless, our findings on increased affect variability in individuals with CT contrasted with previous results showing no CT association with NA variability (Teicher et al., Reference Teicher, Ohashi, Lowen, Polcari and Fitzmaurice2015) and NA or PA WPV (Brick et al., Reference Brick, Nugent and Armey2021). These inconsistencies could be explained by methodological heterogeneity among the studies in EMA items used, samples studied, or consideration of mean affect levels. For instance, Brick et al. (Reference Brick, Nugent and Armey2021) adjusted WPV for within-person mean, while most other studies did not consider mean affect levels. In fact, after adjustment for mean affect levels, all significant associations with dynamic affect fluctuations in our study disappeared. This aligns with a meta-analysis showing that psychological well-being was unrelated to affect dynamics above and beyond the mean affect levels (Dejonckheere et al., Reference Dejonckheere, Mestdagh, Houben, Rutten, Sels, Kuppens and Tuerlinckx2019). Therefore, it is essential to consider mean affect levels when interpreting associations with affect dynamics. Although sexual abuse showed the smallest effects, we cannot conclude that there were statistically or clinically relevant differences between CT types. Instead, effects were most robust for severe CT cases, suggesting that the impact of CT likely stems from frequent recurrence and co-occurrence of CT types (Rosenman & Rodgers, Reference Rosenman and Rodgers2004). Our findings are consistent with this hypothesis and align with studies suggesting a dose-response relationship, with higher CT levels showing the most potent associations with mean affect levels or affect dynamics (Rauschenberg et al., Reference Rauschenberg, van Os, Cremers, Goedhart, Schieveld and Reininghaus2017; Reininghaus et al., Reference Reininghaus, Gayer-Anderson, Valmaggia, Kempton, Calem, Onyejiaka and Morgan2016; Teicher et al., Reference Teicher, Ohashi, Lowen, Polcari and Fitzmaurice2015; Wichers et al., Reference Wichers, Schrijvers, Geschwind, Jacobs, Myin-Germeys, Thiery and van Os2009).
Moderation analysis by psychiatric status revealed no difference in mean affect levels or affect dynamics between adults with CT with and without depressive/anxiety disorders. This is in agreement with previous research on CT-affect associations in the context of depression and anxiety (Teicher et al., Reference Teicher, Ohashi, Lowen, Polcari and Fitzmaurice2015) and also aligns with findings showing neurobiological alterations related to emotion regulation/processing in individuals with CT regardless of psychopathology (Teicher, Samson, Anderson, & Ohashi, Reference Teicher, Samson, Anderson and Ohashi2016). Nevertheless, due to low power to detect significant differences among psychiatric status groups, it is important to interpret these findings with caution. Exploratory moderation analysis by mean affect levels indicated that associations between CT and affect dynamics varied based on mean affect levels: e.g. among those with lower PA levels, CT was associated with lower PA variability; among those with higher NA levels, CT was associated with lower NA variability and autocorrelation. This aligns with emerging research that challenges the interpretation of affect dynamics and suggests that greater and lower affect dynamics could be adaptive/maladaptive depending on a person's mean affect level (Maher et al., Reference Maher, Ra, Leventhal, Hedeker, Huh, Chou and Dunton2018). For instance, as suggested by Maher et al. (Reference Maher, Ra, Leventhal, Hedeker, Huh, Chou and Dunton2018), for individuals with lower mean PA levels, lower PA variability might indicate a chronic affect state without momentary daily uplifts, consequently increasing the risk of affective symptomatology. Similarly, for individuals with higher mean NA levels, lower NA variability might indicate a lack of responsiveness to changing environments and a constant restricted maladaptive level of NA. Hence, it is tempting to speculate that for individuals with CT, generally characterized by lower PA and higher NA levels, increased rather than decreased variability and autocorrelation of momentary affect could be considered adaptive and protective from affective symptomatology. However, these interpretations should be considered preliminary and need further research to draw firm conclusions.
Strengths and limitations
The current study utilized an extensive NESDA-EMAA sample with many available observations to determine CT associations with fine-grained momentary affect dynamics. Our study expanded scarce CT-momentary affect literature by comprehensively examining the relationship between CT and affect dynamics while consistently considering the role of mean affect level. The utilization of EMA for assessing affect in individuals' daily lives also reduced the influence of recall bias (aan het Rot, Hogenelst, & Schoevers, Reference aan het Rot, Hogenelst and Schoevers2012), which has been previously observed in discrepancies between depressed patients' symptom reporting during the week and retrospectively at the end of the week (Mokros, Reference Mokros1993). Nevertheless, some limitations should be noted. First, although retrospective CT assessment is most applicable in clinical practice, it may involve recall bias. However, it has been suggested that psychiatric status does not largely impact CT reporting (Fergusson, Horwood, & Boden, Reference Fergusson, Horwood and Boden2011; Spinhoven et al., Reference Spinhoven, Penninx, Hickendorff, van Hemert, Bernstein and Elzinga2014). More recent evidence also revealed low agreement between prospective and retrospective CT reports (Baldwin, Reuben, Newbury, & Danese, Reference Baldwin, Reuben, Newbury and Danese2019; Danese & Widom, Reference Danese and Widom2020). Therefore, the generalizability of our findings is limited to retrospective CT assessment. Second, although we considered the mean affect level, we must acknowledge the strong floor effect in NA values. Indeed, we observed stronger correlations between mean and dynamic affect variables in NA rather than in PA, suggesting stronger floor effects in NA values than ceiling effects in PA values. Thus, greater NA dynamics, especially variability, in CT might have solely reflected high mean NA values while providing little additional information on variability (Mestdagh et al., Reference Mestdagh, Pe, Pestman, Verdonck, Kuppens and Tuerlinckx2018). Ongoing work in NESDA-EMAA explores an approach to managing floor effects in NA values (von Klipstein et al., Reference von Klipstein, Servaas, Lamers, Schoevers, Wardenaar and Riese2022, May 20). Nevertheless, there is currently no accepted best practice for handling floor effects in EMA data. Third, although a two-week EMA period with five daily assessments may be less burdensome to participants, thereby leading to adequate feasibility, it can be considered too short to determine the stability and generalizability of CT associations with affect dynamics over the more extended periods. A complex systems approach to psychopathology suggests that dynamic system changes may occur at varying time scales, spanning from hours to days, weeks, or even months (Bringmann, Helmich, Eronen, & Voelkle, Reference Bringmann, Helmich, Eronen, Voelkle, Krueger and Blaney2023). Examining these fine-grained affect fluctuations (e.g. increase in variance or autocorrelation) in response to stressors at the individual person-specific level is essential to gain a deeper understanding of affective patterns that might serve as indicators of the transition to psychopathology (Bos & De Jonge, Reference Bos and De Jonge2014; Wichers, Groot, & Psychosystems, Reference Wichers, Groot and Psychosystems2016; Wichers, Smit, & Snippe, Reference Wichers, Smit and Snippe2020), though current evidence is limited (Bos et al., Reference Bos, Schreuder, George, Doornbos, Bruggeman, van der Krieke and Snippe2022; Olthof et al., Reference Olthof, Hasselman, Strunk, van Rooij, Aas, Helmich and Lichtwarck-Aschoff2019; Schreuder et al., Reference Schreuder, Hartman, Groen, Smit, Wichers and Wigman2022; Smit et al., Reference Smit, Helmich, Bringmann, Oldehinkel, Wichers and Snippe2022, November 8). Employing a longer EMA period with a higher frequency of assessments may provide a more detailed person-specific understanding of changes in affect states and allow the examination of potential differences between individuals with and without CT. Last, cross-sectional data limits the examination of the temporal direction of association between CT, affect, and psychiatric status variables. Hence, a longitudinal design more proximal to the occurrence of CT is necessary.
Conclusion, implications, and future research
Our findings suggest that individuals with CT, especially severe CT, show greater affective dysregulation (increased PA variability, NA variability, and NA autocorrelation) during the two-week monitoring of emotional symptoms. However, significant differences observed between the CT and no CT groups were entirely explained by the differences in mean affect levels among these groups. Therefore, when examining CT, dynamic affect measures carry limited unique value (predictive or empirical) beyond that already captured by mean affect levels. Exploratory moderation analysis revealed that lower but not higher affect variability and autocorrelation might indicate maladaptive emotional functioning in CT individuals with generally lower PA and higher NA levels. Thus, our findings highlight the importance of considering mean affect levels when interpreting the impact of CT on affect dynamics. Future comprehensive EMA research in CT should replicate current findings to build a more robust basis for firm conclusions, examine the situational determinants (e.g. daily stressors, social interactions) of momentary affect fluctuations, and explore how different psychotherapeutic and pharmacological treatments may impact affect dynamics. Although statistically affect fluctuations do not seem to have added value beyond the mean levels in CT, they are clinically meaningful. For instance, determining the context in which emotional symptoms are triggered or dampened is helpful for patient insights and diagnostic and treatment selection purposes. Using EMA data also allows to closely evaluate treatment effects, and novel near-real-time ecological momentary interventions teaching stress management and mindfulness skills may be particularly effective in positive thinking upregulation and psychological distress downregulation for individuals with CT (Myin-Germeys et al., Reference Myin-Germeys, Kasanova, Vaessen, Vachon, Kirtley, Viechtbauer and Reininghaus2018; Nguyen-Feng, Romano, & Frazier, Reference Nguyen-Feng, Romano and Frazier2019).
Supplementary material
The supplementary material for this article can be found at https://doi.org/10.1017/S0033291723002969.
Funding statement
The infrastructure for the NESDA study (www.nesda.nl) is funded through the Geestkracht program of the Netherlands Organization for Health Research and Development (ZonMw, grant number: 10-000-1002) and financial contributions of several universities (the departments of psychiatry of Amsterdam University Medical Centers (location VUmc), University Medical Center Groningen and Leiden University Medical Center, the departments of clinical psychology of the University of Groningen and Leiden University), Mental Health care institutions (GGZ inGeest, GGZ Drenthe, Lentis, GGZ Friesland, Rivierduinen and Dimence Group) and the Rob Giel Onderzoekscentrum (RGOc). BP is supported through the European Union's Horizon 2020 research and innovation program (EARLYCAUSE grant n° 848158). CHV is supported by a Dutch Research Council (NWO) Vidi grant (09150171910042). BME is supported by a Dutch Research Council (NWO) Vici grant (435-15-006).
Competing interests
All authors declare no competing interests.