Telomeres are nucleoprotein structures that form the ends of eukaryotic chromosomes where they maintain genomic stability(Reference Blackburn1) and limit the replicative capabilities of the cell(Reference Podlevsky and Chen2–Reference Sfeir, Chai and Shay4). Telomere length at birth is an inherited trait; however, telomeres shorten approximately 50–100 bp in every round of DNA replication because of the inability of DNA polymerase to synthesise chromosomal ends completely(Reference Hodes5,Reference Olovnikov6) . Telomeres therefore shorten naturally with each cell division or replication, eventually leading to cellular senescence(Reference von Zglinicki7,Reference Campisi and d'Adda di Fagagna8) . Leucocyte telomere length (LTL) may experience accelerated attrition directly through oxidative damage, and indirectly through increased cellular replication during chronic inflammation, both of which are hypothesised to mediate the association between lifestyle and LTL(Reference von Zglinicki7). Demographic and lifestyle variables, including dietary habits, physical activity levels and substance abuse, mediate levels of chronic inflammation and oxidative stress, and thus may be associated with LTL. LTL may serve as a marker of biological, rather than chronological, age, and may also provide insight into the risk of chronic diseases associated with oxidative stress and chronic inflammation, including CVD and several cancers(Reference Finkel and Holbrook9–Reference Houben, Moonen and van Schooten15).
Telomeric DNA is rich in guanine nucleotides, which are prone to be selectively oxidised to 8-oxo-2′-deoxyguanosine and 2,6-diamino-4-oxo-5-formamidopyrimidine by reactive oxygen species(Reference Samoylenko, Hossain and Mennerich16,Reference Freitas-Simoes, Ros and Sala-Vila17) . Reactive oxygen species may also produce single-stranded DNA breaks directly, or single-stranded breaks may occur during the repair of oxidative base modifications(Reference Freitas-Simoes, Ros and Sala-Vila17). In addition to these mechanisms, single-strand breaks in telomeric DNA are less efficiently repaired by DNA repair machinery than in non-telomeric genomic DNA. It has been hypothesised that the susceptibility of telomeres to damage by reactive oxygen species is, in fact, an important mechanism whereby telomeres act as ‘sentinels’ of excessive oxidative damage, disallowing the continued proliferation of damaged and potentially oncogenic cells(Reference von Zglinicki7,Reference Zhou, Zhu and Cui18) . Lymphocyte LTL is further affected by oxidative stress because of the increased proliferation (and coinciding telomere loss) of these cells during the inflammatory response triggered by oxidative stress(Reference Hardikar, Song and Risques19).
Current research is aimed at understanding the relationship between advanced telomere attrition and anthropometric measures including increased BMI and intra-abdominal fat(Reference Mundstock, Sarria and Zatti20), as well as lifestyle factors including smoking(Reference Huzen, Wong and van Veldhuisen21), alcohol intake(Reference Weischer, Bojesen and Nordestgaard22) and suboptimal psychosocial health(Reference Stein, Levin and Uziel23), contributing through the impact of oxidative stress and chronic inflammation. Diet is an important source of oxidants and antioxidants and may affect levels of oxidative stress and inflammation in the blood and intestinal environment(Reference de Vos-Houben, Ottenheim and Kafatos24–Reference Kiecolt-Glaser, Epel and Belury27). Diets rich in fruit and vegetables high in antioxidants are associated with lower intracellular concentrations of inflammatory biomarkers and reduced risk of chronic diseases, cancer and CVD(Reference Zhou, Zhu and Cui18,Reference Marin, Delgado-Lista and Ramirez25,Reference Mendez-Lagunas, Rodriguez-Ramirez and Cruz-Gracida28,Reference Rafie, Golpour Hamedani and Barak29) . Thus, telomere length may be a biomarker of dietary oxidant/antioxidant status(Reference Freitas-Simoes, Ros and Sala-Vila17). Traditionally, nutritional epidemiology has focused on the association of specific nutrients or food groups with telomere length and other health outcomes; however, the study of dietary patterns has recently emerged as a more holistic approach at assessing these relationships(Reference Jacobs, Gross and Tapsell30,Reference Tapsell, Neale and Satija31) .
The Alternative Healthy Eating Index 2010 (AHEI-2010) is a score that provides an assessment of the diet's capacity to prevent chronic disease(Reference Chiuve, Fung and Rimm32). Designed as an alternative to the Healthy Eating Index (HEI) which quantified adherence to the US 1995 Dietary Guidelines, the AHEI-2010 was based on foods and nutrients predictive of chronic disease risk(Reference Chiuve, Fung and Rimm32). Higher AHEI-2010 scores have been associated with less weight gain over time(Reference Tobias, Zhang and Chavarro33), lower risk of acute myocardial infarction(Reference Neelakantan, Naidoo and Koh34), lower risk of chronic obstructive pulmonary disease(Reference Varraso, Chiuve and Fung35), lower long-term chronic inflammation(Reference Akbaraly, Shipley and Ferrie36), lower cancer risk(Reference Reedy, Krebs-Smith and Miller37), lower all-cause mortality(Reference Fung, Kashambwa and Sato38) and longer LTL(Reference Crous-Bou, Fung and Prescott39).
The Dietary Inflammatory Index® (DII®) is a tool for assessing diet quality based on inflammatory potential(Reference Shivappa, Steck and Hurley40). The inflammatory capacity of the diet is assessed by estimating the intake of forty-five micro- and macronutrients, multiplying each by an overall inflammatory effect score and then centring each value on global mean intake values(Reference Shivappa, Steck and Hurley40). In this study, each inflammatory effect score was estimated based on the results of a rigorous literature review (about 2000 articles read and scored)(Reference Shivappa, Steck and Hurley40). Higher DII® scores have been associated with higher incidence of cancer, CVD and all-cause mortality(Reference Shivappa, Steck and Hussey41,Reference Deng, Shivappa and Tang42) and lower LTL(Reference Garcia-Calzon, Zalba and Ruiz-Canela43,Reference Shivappa, Wirth and Hurley44) .
The aim of the present study was to assess the association between the intake of foods and nutrients predictive of chronic disease (AHEI-2010), and of the overall inflammatory potential of the diet (DII®) and LTL in postmenopausal, healthy Canadian women. While use of the DII® aligns more closely with the objectives of this study, but because relationships between AHEI-2010 scores and LTL have been extensively explored in the literature, we therefore chose to implement both. We hypothesised that AHEI-2010 scores would be positively associated with LTL and DII® scores inversely associated with LTL. The secondary objective was to assess intake of the individual components of both the AHEI-2010 and DII® for association with LTL, with the hypothesis that certain food groups or nutrients were driving any associations detected in the primary objective.
Methods
Subjects
We conducted a cross-sectional analysis using the baseline data from the Alberta Physical Activity and Breast Cancer Prevention (ALPHA) Trial(Reference Friedenreich, Woolcott and McTiernan45). Women were recruited for the ALPHA Trial through mailings to participants in the Alberta Breast Screening Programme, media campaigns and brochures and posters distributed through the Alberta Family Physicians Research Network. The main inclusion criteria included: aged 50–74 years, English speaking, residents in the Calgary or Edmonton areas, postmenopausal, no previous cancer diagnosis except non-melanoma skin cancer, no major co-morbidities, acceptable heart and lung function (as determined in baseline fitness test), BMI 22·0 to 40·0 kg/m2, breast tissue density ≥0 %, able to undertake unrestricted physical activity as determined through completion of the Physical Activity Readiness-Medical Exam (PAR-MedX) Questionnaire, inactive lifestyle, not currently planning to undertake a weight control/loss programme, not currently, previously (<12 months) or planning to take drugs related to oestrogen metabolism or breast tissue growth, not currently or planning on taking weight loss medications, not a current smoker or excessive drinker. Baseline assessments were performed from 2003 through 2007(Reference Friedenreich, Woolcott and McTiernan45).
Ethics
Research ethics boards at the Alberta Cancer Board and Universities of Calgary and Alberta approved the protocol for this ancillary study of the ALPHA Trial. The Conjoint Health Research Ethics Board at the University of Calgary and the Health Research Ethics Board of Alberta Health Services approved the LTL analysis protocol. The ALPHA Trial was conducted according to the Declaration of Helsinki, and all participants provided written informed consent. The trial was registered at ClinicalTrials.gov (identifier: NCT00522262).
Health and lifestyle assessments
Participants completed four separate questionnaires focused on their baseline health, quality of life and psychosocial factors, physical activity in the past year and dietary intake. The Baseline Health Questionnaire measured health and sociodemographic variables including menstrual and reproductive history; medical and health history; past hormone replacement therapy; medication and vitamin/supplement use; previous benign breast disease; alcohol intake and smoking history. The General Health Questionnaire captured psychosocial measures using the self-administered RAND 36-item Health Survey(Reference Hays, Sherbourne and Mazel46), which assessed overall quality of life, as well as mental and physical health. The Past Year Total Physical Activity Questionnaire(Reference Friedenreich, Courneya and Neilson47) assessed all types of physical activity done in the past 12 months. Finally, the Canadian Dietary History Questionnaire-I (C-DHQ-I) assessed dietary intake over the previous 12 months(Reference Csizmadi, Kahle and Ullman48). This questionnaire was based on the United States National Cancer Institute's Diet History Questionnaire and adapted for use in Canadian populations to reflect differences in food availability and fortification practices(Reference Csizmadi, Kahle and Ullman48). It includes 124 items and generates estimates of food and supplemental macro- and micronutrient intake.
A sub-maximal modified Balke test(Reference Balke and Ware49) was used to estimate maximum oxygen consumption from sub-maximal exercise intensities to measure cardiopulmonary fitness. Anthropometric measurements were taken by Certified Exercise Physiologists using standardised methods and equipment. These include height and weight (used to estimate BMI) and waist and hip circumferences (used to estimate waist:hip ratio). A dual-energy X-ray absorptiometry (DXA) scan was used to assess overall percentage body fat and fat distribution with a Hologic 4500 DXA Scanner (Calgary) or GE Lunar Prodigy High Speed Digital Fan Beam X-ray-based Bone Densitometer (Edmonton). A single-slice computed tomography (CT) scan was used to measure central adiposity (total abdominal fat, intra-abdominal fat and subcutaneous fat) using a Marconi PQ5000 Vision Master/Picker (Calgary) or Phillips/Marconi MX8000 multi-slice scanner (Edmonton).
Measurement of Alternative Healthy Eating Index 2010 and Dietary Inflammatory Index®
DII® and AHEI-2010 scores were estimated for each participant using data derived from the 124-item C-DHQ-I using Diet*Calc software (version 1.4.3) downloaded from the United States National Cancer Institute's Website, Division of Cancer Control and Population Sciences. The DII® was scored for all participants using methods previously described by Shivappa et al.(Reference Shivappa, Steck and Hurley40). Briefly, mean intake was standardised using the global mean and standard deviation, converted to represent a range between −1 (maximally anti-inflammatory) and +1 (maximally pro-inflammatory), multiplied by the overall inflammatory effect score, and summed to generate an overall dietary inflammatory effect score for each participant. A total of thirty-eight of the forty-five dietary parameters were available for the DII® estimation (Table 1) and included both dietary and supplemental intake. AHEI-2010 score was estimated using methods described by Chiuve et al.(Reference Chiuve, Fung and Rimm32). Intake of eleven food groups/macronutrients was used to calculate the corresponding score out of 10 and summed to obtain a score between 0 (minimally ‘healthy’) and 110 (maximally ‘healthy’)(Reference Chiuve, Fung and Rimm32).
Table 1. Association between Alternative Healthy Eating Index 2010 score and Dietary Inflammatory Index® score with leucocyte telomere length (LTL) z-score for continuous and categorised dietary indices in in Alberta Physical Activity and Breast Cancer Prevention (ALPHA) Trial participants, Alberta, Canada (2003–2006)
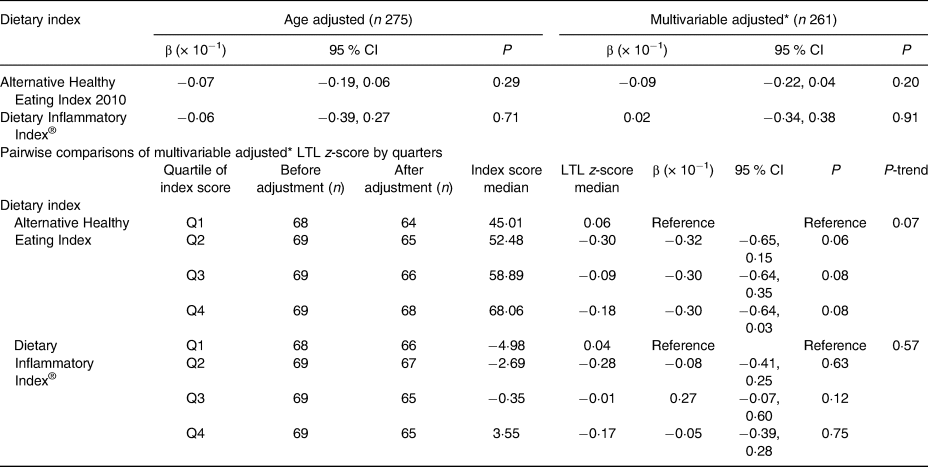
* Model adjusted for age, years since menopause, smoking history, physical activity from recreational activities, cardiopulmonary fitness (VO2max), BMI, intra-abdominal fat area, self-perceived psychosocial health, current employment status and marital status.
Measurement of telomere length
Blood samples were collected at study baseline using standardised blood collection, processing, storage and shipment protocols(Reference Friedenreich, Woolcott and McTiernan45). Nucleic acid extraction was automated on the Hamilton STARlet (Hamilton Robotics Inc.) using the Macherey-Nagel NucleoMag Blood 200 µl kit. DNA was eluted in nuclease-free water and quantified using a Qubit dsDNA HS Assay Kit (ThermoFisher Scientific Inc.).
LTL (T) relative to a standard single-copy β-globin gene (S; 36B4) reference was quantified and expressed as a ratio (T:S). Three reference samples were run on each plate: wild-type BJ (human foreskin fibroblast primary cells), BJ + hTERT (human foreskin fibroblast primary cells transformed to express hTERT), and a pooled standard DNA to estimate inter-plate variation. Pooled standard DNA was prepared by pooling thirty buffy coats selected at random into one sample, and DNA extracted using a QIAmp DNA Mini Kit. All samples were measured in triplicate, and laboratory staff was blinded to the participant ID and time point. Final telomere length measurements were normalised to correct for between-run variation using methods described previously(Reference Ruijter, Ruiz Villalba and Hellemans50).
Statistical analysis
Multivariable linear regression analysis was used to model the association between DII® and AHEI-2010 scores with relative LTL. Relative LTL was square root transformed to achieve a more Gaussian distribution and converted to z-score to simplify interpretation and comparison between studies (Fig. 1(a)), since the calculation of the T:S ratio results in the right skewed distribution inherent of relative data. The inter-plate CV from our quantitative PCR analysis were relatively large, with values for our standard pooled DNA and wild-type human foreskin fibroblast primary cells reference DNA of 39 and 27 %, respectively. After applying a previously described normalisation factor(Reference Ranstam51), inter-plate variation was reduced to 10 % for standard pooled DNA and 12 % for the wild-type BJ reference DNA. Finally, square root-transformed relative telomere lengths were normalised using z-scores for ease of interpretation and for comparison with existing literature. Relative, square root-transformed, normalised LTL is referred to simply as LTL from this point forth.

Fig. 1. Histogram of baseline data from participants in the Alberta Physical Activity and Breast Cancer Prevention (ALPHA) Trial (n 275) in Alberta, Canada (2003–2007) (a) Square root transformed relative leucocyte telomere length z-score, (b) Alternative Healthy Eating 2010 score, (c) Dietary Inflammatory Index® score and (d) anthocyanidin intake (mg/d).
Age-only and multivariable-adjusted estimates were reported. Final models were adjusted for: age, years since menopause, smoking history (<100 lifetime cigarettes; former casual smoker; former smoker), recreational physical activity (metabolic equivalent (MET)-h/week per year), cardiopulmonary fitness (VO2max), BMI, intra-abdominal fat area (cm2), self-perceived psychosocial health, current employment (dichotomous) and marital status. For the secondary analysis of the eleven individual components of the AHEI-2010 and thirty-eight available components of the DII®, a Bonferroni correction(Reference Ranstam51) was applied to adjust for multiple comparisons. Based on an extensive review of the literature, age, smoking history and past-year recreational physical activity (metabolic equivalent (MET)-h/week per year) were assessed for potential effect modification by including an interaction term in the multivariable linear regression model for each potential modifier. A P value <0·05 for the interaction term was considered statistically significant evidence for effect modification that would have resulted in stratum-specific estimates being reported. Non-linear relationships for each index and LTL were assessed using restricted cubic spline analysis with five knots at the 5th, 27·5th, 50th, 72·5th and 95th percentiles of diet score.
Sensitivity analyses were conducted to explore the effect of the DII® estimated with food only (no supplements), age-adjusted models for those for whom smoking data were available only to match the multivariable-adjusted population, and AHEI-2010 models additionally adjusted for total energy intake. Statistical analyses were performed using STATA (Intercooled (IC) version 14.0 for Mac), P values were two-sided, with α-level = 0·05.
Results
Dietary, LTL and demographic data were available for 263 women. A full project flow chart can be visualised in Fig. 2. The mean age was 60·9 (sd 5·5) years (range 50–74 years) and BMI was 29·3 (sd 4·4) kg/m2. Women were primarily Caucasian (91·6 %), non-smokers (69·2 % had smoked less than 100 lifetime cigarettes) and relatively well educated (67·6 % with college/trade diploma or university degree). Detailed population characteristics are summarised in Table 2.
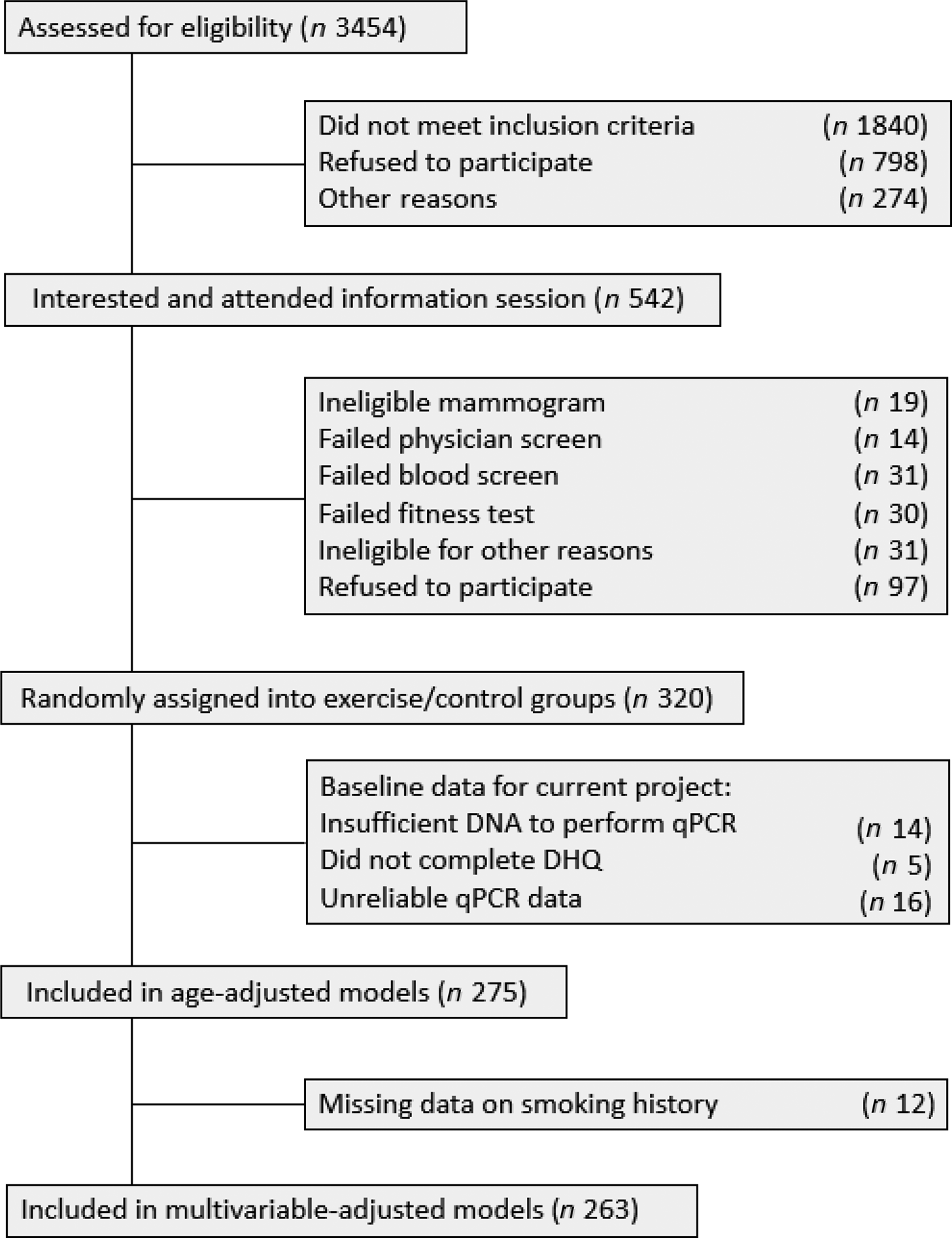
Fig. 2. Participant flow chart showing recruitment and inclusion of participants into the Alberta Physical Activity and Breast Cancer Prevention (ALPHA) Trial, including baseline participants included in the present study. Alberta, Canada (2003–2007). qPCR, quantitative PCR; DHQ, Dietary History Questionnaire.
Table 2. Baseline characteristics for participants (n 275) and univariate associations between baseline characteristics with Alternative Healthy Eating Index 2010 (AHEI-2010) score, Dietary Inflammatory Index® (DII®) score and leucocyte telomere length (LTL) in the Alberta Physical Activity and Breast Cancer Prevention (ALPHA) Trial, Alberta, Canada (2003–2006)

CRP, C-reactive protein; MET, metabolic equivalent.
Several demographic and anthropometric characteristics were statistically significantly associated with LTL including recreational physical activity (β = 0·01; P = 0·01) and intra-abdominal fat area (β < −0·01; P = 0·04) but no association was found with age (β = −0·02; P = 0·06; Table 2). The inflammatory biomarker IL-6 was inversely associated with LTL (β = −0·15; pg/ml; P < 0·01), yet not with the AHEI-2010 (β = −0·35; P = 0·49) or the DII® (β = 0·04; P = 0·81). C-reactive protein was not associated with LTL (β = −1·80; P = 0·20), the AHEI-2010 (β = −0·94; P = 0·48) or DII® (β = 0·29; P = 0·56). A complete summary of univariate associations between AHEI-2010, DII® score and LTL with baseline demographic and anthropometric characteristics are shown in Table 2.
AHEI-2010 score was approximately normally distributed, with a mean score of 56·3 (sd 9·75) (Fig. 1(b)). Results from the age-adjusted model estimated that for a one-unit increase in AHEI-2010 score, LTL decreased by approximately 0·07 (95 % CI −0·019, 0·06) standard deviations (P = 0·29). Similarly, no significant association was detected between LTL and AHEI-2010 in the fully adjusted model, where a one-unit increase in AHEI-2010 score corresponded to an estimated decrease of 0·09 (95 % CI −0·22, 0·04) standard deviations of LTL (P = 0·20). There was no statistical evidence of effect modification for age, smoking history or recreational physical activity. Primary results are summarised in Table 1. Restricted cubic spline regression analysis provided no evidence of a non-linear relationship between AHEI-2010 and LTL z-score (P = 0·37). Results of the multivariable adjusted pairwise comparisons of AHEI-2010 quartiles with LTL (Table 1) indicated no statistically significant relationship between the first and second quartiles (β = −0·32; P = 0·06), third quartile (β = −0·30; P = 0·08) or fourth quartile (β = −0·30; P = 0·08). The test for linear trend across quartiles was also non-statistically significant (P-trend = 0·07). An exploratory analysis which assessed the eleven individual parameters that comprise the index as individual exposures also found no significant association between dietary intake from any parameter and LTL in age-adjusted or multivariable adjusted models (Table 3). AHEI-2010 scores correlated inversely (r −0·44; P < 0·01) with DII® scores (Fig. 3). DII® score was approximately normally distributed as illustrated in Fig. 1(c), with a mean score of −1·01 (sd 3·58). As indicated in Table 1, the age-adjusted model estimated that for a one-unit increase in DII® score, the LTL decreased by approximately 0·06 (95 % CI −0·39, 0·27) standard deviations (P = 0·71). Similarly, no statistically significant association was detected between LTL and the DII® in the multivariable adjusted model where a one-unit increase in DII® score corresponded to an estimated increase of 0·02 (95 % CI −0·34, 0·38) standard deviations of the LTL (P = 0·91). There was no statistical evidence of effect modification by age, smoking history or recreational physical activity. Restricted cubic spline regression analysis provided no evidence of a non-linear relationship between the DII® and LTL (P = 0·56). Multivariable adjusted pairwise comparisons of DII® quartiles with LTL (Table 3) indicated no statistically significant relationships for any of the quartiles of DII® and there was no statistically significant linear trend.

Fig. 3. Scatterplot demonstrating correlation (r −0·44; P < 0·001) between Alternative Healthy Eating Index 2010 (y-axis) scores and Dietary Inflammatory Eating Index® scores (x-axis) from participants in the Alberta Physical Activity and Breast Cancer Prevention (ALPHA) Trial (n 275) in Alberta, Canada (2003–2007). ---, Fitted values.
Table 3. Associations between Alternative Healthy Eating Index 2010 component dietary intake and Dietary Inflammatory Index® component with leucocyte telomere length z-score in Alberta Physical Activity and Breast Cancer Prevention (ALPHA) Trial participants, Alberta, Canada (2003–2006)*

* Data unavailable for garlic, ginger, rosemary, saffron, thyme/oregano, turmeric and eugenol intake while calculating Dietary Inflammatory Index® score.
† Model adjusted for age, years since menopause, smoking history, physical activity from recreational activities, cardiopulmonary fitness (VO2max), BMI, intra-abdominal fat area, self-perceived psychosocial health, current employment status and marital status.
‡ Not including potatoes/French fries.
§ Not including fruit juices.
‖ EPA and DHA.
An exploratory analysis that assessed each of the thirty-eight available parameters of the DII® (Table 3) as individual potential predictors of LTL found that anthocyanidin intake was significantly associated with LTL z-score in both age-adjusted (β = 0·01; P = 0·01) and multivariable adjusted (β = 0·09; P < 0·01) models, but not in Bonferroni corrected (P = 0·23) models. All sensitivity analyses conducted had a trivial effect on the interpretation of the results and conclusions of the study (data not shown).
Discussion
This study found neither DII® nor AHEI-2010 scores to be associated with LTL in this population of healthy, postmenopausal women. Additionally, none of the eleven components of the AHEI-2010 were associated with LTL. LTL was not statistically significantly correlated with age, and neither DII® nor AHEI scores were associated with the inflammatory biomarkers C-reactive protein or IL-6 as expected.
Only one study has examined the relationship between the AHEI-2010 and LTL in 4676 disease-free women from the Nurses' Health Study (NHS), in which the authors found higher AHEI-2010 scores to be associated with longer LTL(Reference Crous-Bou, Fung and Prescott39). Results from two other studies that had previously examined the association between DII® score and LTL indicated a statistically significant inverse association between baseline DII® and LTL(Reference Garcia-Calzon, Zalba and Ruiz-Canela43,Reference Shivappa, Wirth and Hurley44) . Shivappa et al.(Reference Shivappa, Steck and Hussey41) found that higher DII® scores were associated both with shorter LTL, and with C-reactive protein (serving as a construct validation of the DII®) in a cross-sectional analysis of 7215 National Health and Nutrition Examination Survey (NHANES) participants. Garcia-Calzon et al.(Reference Garcia-Calzon, Zalba and Ruiz-Canela43) found that an increase in DII® score was associated with a two-fold increased LTL attrition over a 5-year follow-up in 520 Spanish men and women from the PREvenciόn con DIeta MEDiterránea (Prevention with Mediterranean Diet Study; PREDIMED-NAVARRA) study. Conversely, in 2018, De Meyer et al.(Reference De Meyer, Bekaert and De Buyzere52) published cross-sectional results indicating no association between DII® scores and LTL in 2509 healthy men and women, concluding an at most limited association between dietary patterns and LTL in the general population. Similarly, Meinilä et al.(Reference Meinilä, Perälä and Kautiainen53) recently reported no association between DII® scores in either cross-sectional analyses or longitudinal assessments over 10 years in their cohort of 1046 elderly men and women who participated in the Helsinki Birth Cohort Study. Our study differs in sample size (n 263 v. 4676 in the NHS) and design (cross-sectional v. prospective with change in LTL measured) to studies that reported relationships between the DII® and LTL, we could conclude that our analyses limited our ability to detect differences in LTL. Our study findings do, however, align with the findings of Meinilä et al.(Reference Meinilä, Perälä and Kautiainen53) and De Meyer et al.(Reference De Meyer, Bekaert and De Buyzere52) and therefore we must consider that the inflammatory potential of the diet does not directly or indirectly influence LTL as hypothesised.
While the statistically significant association between anthocyanidin intake and LTL was no longer statistically significant after correction for multiple testing, some discussion of this finding is warranted. Anthocyanidins are common plant pigments that reflect light in in the red to blue range and are responsible for the pigmentation in foods such as blueberries, raspberries, red onions and purple yams(Reference Juroszek, Lumpkin and Yang54,Reference Li, Deng and Zhu55) (list not exhaustive). Anthocyanidins are considered the most important subcategory of flavonoids because of their strong in vitro and in vivo antioxidant activity(Reference Mendez-Lagunas, Rodriguez-Ramirez and Cruz-Gracida28,Reference Juroszek, Lumpkin and Yang54,Reference Li, Deng and Zhu55) . While this finding may be a result of type I error from multiple testing, further investigation into this highly biologically plausible association is justified.
This study had several strengths of note. First, we were able to evaluate several well-measured potential confounders. For example, direct measurements of body size and composition were taken including a DXA scan for overall percentage body fat and fat distribution and a computed tomography (CT) scan for central adiposity (total abdominal fat, intra-abdominal fat and subcutaneous fat). Self-reported physical activity was assessed using the reliable and validated Past Year Total Physical Activity Questionnaire(Reference Friedenreich, Courneya and Neilson47). Moreover, the stringent exclusion criteria of the underlying ALPHA Trial reduced potential confounding by those restricted variables (e.g. alcohol consumption, current smoking, etc.). In addition, we had thirty-eight of the forty-five DII® parameters available to calculate the DII® score, where examples from the literature often include as few as twenty parameters(Reference Ren, Zhao and Wang56). Extensive dietary data were also available for potentially important vitamins, minerals and flavonoids.
These results are limited by using self-reported dietary measurements which may be biased to over-reporting of healthy foods and under-reporting of unhealthy foods (social desirability bias)(Reference Mossavar-Rahmani, Tinker and Huang57). There was some underreporting of total energy intake, which is common for FFQ such as the C-DHQ-I. In addition, neither dietary index was associated with the key inflammatory biomarkers C-reactive protein or IL-6, thus the DII® and AHEI-2010 are not validated by the present study. Measurement error is a common limitation when measuring telomere length, regardless of the assay chosen(Reference Aubert, Hills and Lansdorp58) and LTL did not correlate significantly with age, compromising the results of the present study. The cross-sectional study design prohibits temporality from being established, and generalisability is limited since this study was conducted on a relatively small sample of primarily Caucasian, healthy, postmenopausal women of relatively high education and socio-economic status. The inclusion of postmenopausal women only may have also limited our ability to detect associations, since the association between LTL and inflammation may be less pronounced in postmenopausal than pre-menopausal women(Reference Aviv, Valdes and Gardner59). Hypothesised mechanisms underlying this finding include differing insulin resistance and leptin levels in pre- v. postmenopausal women and decreased circulating oestrogen in postmenopausal women(Reference Aviv, Valdes and Gardner59). Oestrogen may be linked to LTL through its anti-inflammatory and oxidant properties, and its ability to stimulate telomerase, a telomere-elongating enzyme(Reference Aviv, Valdes and Gardner59). The use of a homogeneous population in terms of age (50 to 74 years), ethnicity, anthropometric characteristics, physical activity and sociodemographic factors may have limited the range of telomere length variability such that only studies with large sample sizes, or exposures that have an important impact on LTL, would be able to detect any significant changes. For the same reasons mentioned above, the results of this study have limited generalisability. Finally, given the initial design of the ALPHA Trial and its primary purpose, these analyses were exploratory in nature which we acknowledge as a limitation of this study.
This study did not provide evidence of an association between the AHEI-2010 or DII® and LTL in this cohort. However, this research alone does not discount the association between dietary patterns and reduced oxidative damage and longer telomeres. Larger studies, or longer-term cohort or randomised controlled trials, would be an ideal alternative to understand this putative relationship more fully, as detectable changes in telomere attrition rates due to environmental stressors may take several years to occur(Reference Garcia-Calzon, Gea and Razquin60). Variations or SNP in genes involved in the inflammatory response should be considered for future studies. Finally, our findings corroborate recent research which suggest that the diet's inflammatory potential may have limited impact on telomere length and telomere attrition rates(Reference De Meyer, Bekaert and De Buyzere52,Reference Meinilä, Perälä and Kautiainen53) .
Acknowledgements
The ALPHA Trial study set-up was done by Kim van der Hoek and Marla Orenstein. The Study Coordinators were Rosemary Crosby and Ame-Lia Tamburrini. Data preparation for this ancillary study was done by Qinggang Wang. The DNA extraction and quantification protocol was designed by Dr John B. McIntyre and performed by Angela Chan and Michelle Dean in the Translational Laboratory of the Tom Baker Cancer Center. Quantitative PCR telomere length analysis was performed by Nick Ting in Dr Tara Beattie's Laboratory at the University of Calgary. Dr Will King contributed to the design and conduct of the telomere length analysis. Dr Nigel Brockton provided advice on the DNA extraction protocols developed for this study.
The study was supported by research grant no. 017468 from the Canadian Breast Cancer Research Alliance (Alberta Physical Activity and Breast Cancer Prevention (ALPHA) Trial) and grant no. MOP-130238 from the Canadian Institutes of Health Research. C. M. F. was supported by an Alberta Innovates-Health Solutions Health Senior Scholar Award and by the Alberta Cancer Foundation's Weekend to End Women's Cancers Breast Cancer Chair. K. S. C. was supported by the Canada Research Chairs programme and D. R. B. by the Canadian Cancer Society Research Institute Cancer Prevention Career Development Award.
The study design was done by A. T. M., D. R. B., T. B. and C. M. F. and the study conduct was completed by A. T. M., D. R. B., T. B., K. S. C. and C. M. F.; data analysis was done by A. T. M. with guidance from D. R. B., C. M. F. and T. W.; T. B. provided essential reagents and materials and oversaw the laboratory work; A. T. M. and C. M. F. wrote the paper and have responsibility for the final content. All authors contributed to the manuscript and reviewed the final version.
The authors of this paper declare no conflicts of interest.