The 2030 Agenda for Sustainable Development is meant to build a better and sustainable future for all, as specified in 17 Sustainable Development Goals. A critical question is whether the world is on track to achieve these goals. Given the all-encompassing character of the 2030 Agenda, developing and applying research methods that can answer this question are a tremendous challenge. In this chapter, we discuss research methods to understand and explain the steering effects of the Sustainable Development Goals.
The previous chapters synthesized scholarship on the steering effects of the Sustainable Development Goals in five key areas. As defined in Chapter 1, the term ‘steering effects’ refers to the influence of the global goals on behavioural change of political, economic and societal actors. We need to know how the goals influence political discourse, institutional change and decision-making from local to global scales. Therefore, we need to include methods that aim to capture these aspects. Ultimately, however, the Sustainable Development Goals must also be evaluated in broader terms of the actual progress towards their achievement. This can be done either for the current situation and recent past or through projecting possible future impacts of current trends.
We have chosen to use a broad definition of steering effect to capture both elements: the political and societal responses to the Sustainable Development Goals and the degree to which the Sustainable Development Goals are achieved. These elements can be compared to two methods to evaluate the steering of a car: The steering effect can be measured by either changes in the steering wheel’s position or in the car’s direction. One needs both types of information: Looking at the direction of the car alone does not inform on whether it is the steering wheel that causes changes, while monitoring the steering wheel alone does not inform on whether changes are sufficient and in the right direction. Here, the steering wheel stands for the impact on institutional change, decision-making and the political discourse, and the direction of the car the overall achievement of the goals.
This chapter presents a review of the multi-faceted landscape of methods used to study the steering effects of the Sustainable Development Goals. While the other chapters in this book carried out a systematic literature review, this chapter provides an overview of the methods that are used to understand the steering effects of the goals, based on a literature review. This chapter provides a meta-level and interdisciplinary perspective on these methods. Our analysis covers methods, which we define as approaches to answer questions about the steering effects of the Sustainable Development Goals. We distinguish these approaches from tools, which build on such methods and help to bridge research, policy-making and the wider public debate. Tools often give access to data and make research on the goals more accessible. Although our analysis focuses on methods, we include tools where relevant. We include quantitative and qualitative methods and illustrate their diversity. However, we do not aim for complete coverage of all methods; we focus on those that are most widely applied.
In the next section, we first discuss the political use of science and the complex relations between science and politics. We then lay out and apply our framework for discussing the methods, organized around four dimensions relevant for understanding the methods’ contribution to studying the Sustainable Development Goals: the temporal perspective, geographical scale, topical coverage, and interactions and interlinkages. Based on this, we discuss the main purposes of the methods and their strengths and limitations. We also identify knowledge gaps and suggest potential steps forward. Finally, we summarize and compare the methods before listing some observations for further research on the steering effects of the Sustainable Development Goals.
The Political Use of Scientific Evidence
Emphasizing the need for evidence-based policy-making is constitutive for the 2030 Agenda and its implementation. The Millennium Development Goals were often criticized for being unmeasurable and that it was hard to know what was achieved (Feeny Reference Feeny and Churchill2020). When the Sustainable Development Goals were developed, more attention was devoted to elaborating a system of goals, targets and indicators to measure implementation, progress and achievements. United Nations bodies such as the Open Working Group, the Inter-agency and Expert Group on SDG Indicators, and science at large contributed to this process. As such, the Sustainable Development Goals can be seen as part of a global effort to move to transparent, evidence-based policy-making.
Although considered decisive for the steering effects of the Sustainable Development Goals, the evidence-based policy-making approach has also been challenged. First, already during the negotiations leading to the adoption of the 2030 Agenda, tensions arose around whether targets were to be derived directly from science, which was perceived as more neutral, or whether they should be more norm-based. In this context, trends towards further polarization in some societies have also led to questions about the empirical evidence for sustainable development (Gottenhuber and Mulholland Reference Gottenhuber and Mulholland2020).
Despite these challenges, the use of scientific data and evidence can serve important purposes for politics and society, such as agenda-setting, accountability, informing decision-making, and monitoring and evaluation. First, data and evidence are critical for political agenda-setting (Chimhowu, Hulme and Munro Reference Chimhowu, Hulme and Munro2019). For instance, the Fridays For Future movement uses scientific evidence for pressuring governments to change their policies towards more sustainability. Second, holding governments and other actors accountable requires data on policy processes and outcomes. For instance, governments need to be transparent and inform citizens about progress in the implementation of the Sustainable Development Goals (Breuer and Leininger Reference Breuer and Leininger2021; Laberge and Touihri Reference Laberge and Touihri2019). Third, data and evidence are needed for informing public policy-making. Evidence can feed into theories of change of policy programmes. For example, designing social cash transfers (Goal 1) to improve food security (Goal 2) requires knowledge about the mechanisms that link such social protection measures with sustainable food provision (Burchi, Scarlato and d’Agostino Reference Burchi, Scarlato and d’Agostino2018). Fourth, monitoring and evaluation are needed to measure progress of implementing the goals (monitoring) and to gain knowledge on the effectiveness of their implementation (evaluation) (Bowen et al. Reference Bowen, Cradock-Henry, Koch, Patterson, Häyhä, Vogt and Barbi2017).
Various methods have been applied for these types of political use of scientific evidence in the context of implementation of the global goals. On the one hand, studies that use prospective methods can bring out new issues on political agendas and inform policy-making. For instance, modelling future scenarios as reported by the Intergovernmental Panel on Climate Change and the Intergovernmental Science-Policy Platform on Biodiversity and Ecosystem Services has had a decisive impact on shaping environmental agendas of governments and international bodies (Beck and Mahoney Reference Beck and Mahoney2018). On the other hand, all types of political use of data and evidence require a retrospective use. For instance, societies can hold their governments accountable, and specific programmes can monitor progress by applying a set of predefined and adaptable indicators.
Although various methods are available and used to study the Sustainable Development Goals, some aspects of the 2030 Agenda require new thinking and further methodological developments. This relates to the development of a network of measurable targets and addressing the interlinkages between goals with implications for the use of specific methods. Five implications can be highlighted:
First, a lack of data to measure all 17 Sustainable Development Goals is still a challenge (Fukuda-Parr and McNeill Reference Fukuda-Parr and McNeill2019). Some of the 169 targets and the 231 unique indicators lack a reliable data basis. This is in part owing to governments agreeing on these targets and indicators even though some were not measurable in the beginning.
Second, the Sustainable Development Goals are a system of interacting components rather than just a collection of goals, targets and indictors (Pradhan Reference Pradhan2019), aiming to leave sectoral silos behind (Breuer, Leininger and Tosun Reference Breuer and Oswald Spring2019). Science needs to use and develop methods, which allow them to capture and assess integrated policy-making.
Third, there is a tension between the innovative character of the 2030 Agenda and the state of the science. Science cannot provide all information and evidence that is necessary for goal implementation (Lang et al. Reference Lang, Wiek, Bergmann, Stauffacher, Martens, Moll and Thomas2012). More transdisciplinary research, theory-building, integration of methods and generalizable evidence are needed to inform implementation of the Sustainable Development Goals.
Fourth, science–policy interfaces need to be aligned to the 2030 Agenda (Roehrl, Liu and Mukherjee Reference Roehrl, Liu and Mukherjee2020). For instance, despite broad acknowledgement of the need for evidence-based policy-making, science representatives are hardly present in national implementation (TWI2050 2020). This is important as only an informed society plays a significant role in holding governments accountable and setting public agendas (Fox Reference Fox2015).
Fifth, evidence and data are not sufficient if they are not ‘translated’ for policy-makers. Methods, including those described in this chapter, are often complex and make results less accessible for policy-makers. Policy-makers mostly care little about the methods used as long as results are reliable and robust (di Lucia et al. Reference Di Lucia, Nilsson, Khan and Slade2020). This is where tools can come in to bridge between scientific methods and policy-makers.
In discussing the methods used in the literature, we will thus consider these functions of data and evidence as well as these five implications of the particularities of the Sustainable Development Goals.
Most Common Methods
Various methods have been applied to assess the steering effects of the Sustainable Development Goals. They differ in the two fundamental aspects of the steering effects that we explained above: they assess either the impact of goals on decision-making and politics or the progress towards achieving the goals. Four dimensions are especially relevant for better understanding the main methods.
First, methods differ as to their temporal perspective. They can be useful to either examine the current situation and historical progress (that is, in a retrospective or ex-post evaluation) or to develop scenarios and project trends that help understand whether the goals can be met by 2030 (that is, a prospective or ex-ante evaluation). Second, methods can be used at different scales (global, regional, national or local), often linking local developments with national or global effects. Data availability on the Sustainable Development Goals, however, differs strongly across these scales. Third, methods can vary in topical coverage. Some assess only a single goal. Others can address multiple goals and topics and even their interrelations. Fourth, the 17 goals and their 169 targets are a set of integrated objectives that interact across scales and sustainability dimensions. A robust understanding of such interactions within and across the goals is vital for shaping policies towards achieving the goals (see also Chapter 4 of this book).
We now use these four dimensions to characterize and assess five widely used methods to study the Sustainable Development Goals. These methods are monitoring approaches, model-based scenario approaches, qualitative case studies, network analysis, and discourse and interpretative approaches. Regarding the two fundamental elements of steering effects, the first two approaches aim at measuring progress towards the goals, while the other three aim at studying the goals’ impact on decision-making and political discourses. In the following, we discuss the main purposes, strengths and limitations of the methods, also in the light of the five specific challenges that we introduced above.
Monitoring Approaches
We define monitoring approaches as methods that use data on historical and current trends to study the steering effects of the Sustainable Development Goals in terms of progress towards goal achievement. These approaches use indicators and indices to measure, monitor and rank progress in attaining some or all Sustainable Development Goals. Indicator frameworks are also used as management tools for developing implementation strategies and resource allocations for achieving the goals (Sustainable Development Solutions Network 2015). Additionally, indicators can be combined to form indices or be used as a set to understand interlinkages among Sustainable Development Goals.
To monitor progress, various indicator frameworks are used on scales from global to local. Specifically there are three indicator frameworks or databases that policy-makers, practitioners and researchers widely use (see Table 7.1):
Table 7.1. Data availability provided by three global indicator databases in mid-August 2020
Parameters and databases | United Nations (2020) | World Bank (2020) | Bertelsmann Stiftung & Sustainable Development Solutions Network (Sachs et al. Reference Sachs, Schmidt-Traub, Kroll, Lafortune, Fuller and Woelm2020) |
---|---|---|---|
Countries and areas | 258 | 215 | 193 |
Time period | 1967–2019 | 1990–2019 | 2000–2020 |
Unique indicators | 192 | — | 114*** |
Unique series codes* | 432 | 367 | — |
Disaggregation level** | 29 | 9 | — |
Covered targets | 136 | 75 | — |
* Series code is a technical abbreviation for indicators for the Sustainable Development Goals, further fragmented into individual indicators without duplications across targets.
** Disaggregation level refers to country-disaggregated data in terms of demographic factors (such as gender, age, urban and rural population), which is required to monitor the pledge of the 2030 Agenda to ‘leave no one behind’, and in terms of non-demographic factors (such as cities, type of product, or type of sector).
*** 85 global indicators and 29 OECD-only indicators.
The first is the Official Global Indicator Framework for Sustainable Development Goals of the United Nations, which defines 231 indicators that cover the multidimensional aspects of the 17 Sustainable Development Goals and their 169 targets (E/CN.3/2020/2). Based on this indicator framework, the United Nations offers historical and current data on the Sustainable Development Goals, mostly based on reports from countries or international organizations (United Nations 2020). It also provides disaggregated data, for example on gender, age group, rural–urban, cities, sectors or products, to monitor the key principle of the 2030 Agenda to ‘leave no one behind’.
For the second set, the World Bank has extracted indicators from the World Development Indicators and reorganized them according to the Sustainable Development Goals and targets (World Bank 2020). These indicators help monitor the goals; however, they do not always match the United Nations’ official indicators (United Nations 2020; World Bank 2020).
A third data set is provided by the Bertelsmann Stiftung and Sustainable Development Solutions Network, which has defined a set of indicators to measure and monitor the Sustainable Development Goals. These indicators are mapped to the Sustainable Development Goals but not to their respective targets.
In addition to the global perspective, indicators are also developed to monitor and assess progress on the goals on the regional, national and local level. Government agencies have proposed indicators to monitor progress on the Sustainable Development Goals at national and local levels based on the Global Indicator Framework (e.g., National Planning Commission 2017). For instance, in support of Germany’s Sustainable Development Strategy, Germany’s statistical authority has launched a national open online reporting platform that presents time series and metadata in an edited, interactive and downloadable way (Destatis 2020). As another case, Nepal has integrated the Sustainable Development Goals into national development frameworks. Besides Voluntary National Reviews and roadmap reports, Nepal provides a platform for the Sustainable Development Goals with data and indicator projections until 2030 (Nepal in Data 2020). Both local and national governments are committed to reporting their progress towards the Sustainable Development Goals either locally through Voluntary Local Reviews or nationally through Voluntary National Reviews (e.g., NGO Federation of Nepal 2020). These reviews are bottom-up processes based on the participation of stakeholder groups, providing insights into policy developments to achieve the goals. Thus, Voluntary National Reviews are a vehicle to understand the steering effects of the Sustainable Development Goals. Also some civil society organizations use indicators to hold governments accountable for their goal implementation. The community of civil society ‘watchdogs’ that use these indicators has been growing slowly. One example is the 2030Watch project, which focuses on high-income countries (Hege and Demailly Reference Hege and Demailly2018). At the local level, the Sustainable Development Solutions Network offers municipal indicators covering, for instance, cities in Bolivia (Andersen et al. Reference Andersen, Canelas, Gonzales and Peñaranda2020), Europe (Lafortune et al. Reference Lafortune, Zoeteman, Mulder, Dagevos and Schmidt-Traub2019), and the United States (Lynch, LoPresti and Fox Reference Lynch, LoPresti and Fox2019). These few examples show the diversity of indicator datasets for the Sustainable Development Goals and data development and adaption of the Global Indicator Framework at various levels.
Some stakeholders and scholars creating future scenarios have begun to develop methodologies for summarizing the complex data sets of Sustainable Development Goals indicators, for example, by condensing the Global Indicator Framework into fewer numbers (Bidarbakhtnia Reference Bidarbakhtnia2020) or by creating an Sustainable Development Goals index. Several studies have also tried to understand the interactions among goals and targets at global, regional and national scales based on such indicators and indices. These studies used statistical techniques to quantify correlations between two or more variables (Pradhan et al. Reference Pradhan, Costa, Rybski, Lucht and Kropp2017), to reduce complexity through factor analysis constructing composite indices of the Sustainable Development Goals (Shaker Reference Shaker2018), to identify inconsistencies and measure progress through Confirmatory and Explanatory Factor Analyses (Spaiser et al. Reference Spaiser, Ranganathan, Swain and Sumpter2017) or to understand relationships between goals through Granger causality, that is, a prediction-based statistical causality concept (Apergis, Jebli and Youssef Reference Apergis, Jebli and Youssef2018; Dörgő, Sebestyén and Abonyi Reference Dörgő, Sebestyén and Abonyi2018). However, the availability of data, the assumption of linearity in many techniques, and confounding variables in the bivariate analysis limit this type of analyses (Kroll, Warchold and Pradhan Reference Kroll, Warchold and Pradhan2019; Pradhan et al. Reference Pradhan, Costa, Rybski, Lucht and Kropp2017; Spaiser et al. Reference Spaiser, Ranganathan, Swain and Sumpter2017; Warchold, Pradhan and Kropp Reference Putra, Pradhan and Kropp2020). Finally, many examples in this section have also created tools to access information to strengthen the interface to decision-makers.
While the implementation of the Sustainable Development Goals started in 2015, data availability and quality of indicators at global, regional, national and local scales are still limited. First, we lack data for several of the 169 targets (see Table 7.1). Data coverage varies across countries and domains. Second, not all global indicators can be applied to all countries. Third, there is sometimes a mismatch between data types and scales for the same indicator (Kraak, Ricker and Engelhardt Reference Kraak, Ricker and Engelhardt2018). Fourth, disaggregated data often still lack consistency.
Importantly, the COVID-19 pandemic has radically altered the economic, social and environmental realities. The pandemic has negatively affected many Sustainable Development Goals, even though it might have also given a narrow window of opportunity for sustainable transformation (Pradhan et al. Reference Pradhan, Subedi, Khatiwada, Joshi, Kafle, Chhetri and Bhuju2021). As a result, the basis on which the indicators for the Sustainable Development Goals were built in 2015 has shifted (Naidoo and Fisher Reference Naidoo and Fisher2020; Nature Editorial 2020). A Nature Editorial (2020) thus called for a revision of the Global Indicator Framework. In such a revision, the limits of the indicator framework should also be addressed, with a priority for transformative recovery after the pandemic.
Model-based Scenario Approaches
Model-based scenario approaches are another method to study the steering effects in terms of progress towards the goals. Model-based approaches can describe the relations between societal trends and the Sustainable Development Goals. They can help understand trends retrospectively but also be used for future projections. Such projections can look at current trends and policies or what policies and measures would be needed to achieve the Sustainable Development Goals. Models may have different purposes, such as exploring different futures, supporting decision-making under uncertainty, social learning, and developing system understanding and experimentation (Kelly et al. Reference Kelly, Jakeman, Barreteau, Borsuk, ElSawah, Hamilton and Voinov2013). Different types of models have been applied studying the Sustainable Development Goals, from economic models such as general equilibrium models and (macro-)econometric models to multi-regional input–output models, system dynamics models, agent-based models and integrated assessment models (Allen, Metternicht and Wiedmann Reference Allen, Metternicht and Wiedmann2016; Bennich, Weitz and Carlsen Reference Bennich, Weitz and Carlsen2020). Many of these models can help investigate the multiple dimensions of the Sustainable Development Goals, assess the strategies to achieve multiple goals simultaneously (van Soest et al. Reference Van Soest, van Vuuren, Hilaire, Minx, Harmsen, Krey and Luderer2019), and to explore potential future changes.
Several studies have used models to investigate sets of Sustainable Development Goals, for instance the impact of climate, biodiversity and land-use policies and their interrelations (e.g., Collste, Pedercini and Cornell Reference Collste, Pedercini and Cornell2017; Humpenöder et al. Reference Humpenöder, Popp, Bodirsky, Weindl, Biewald, Lotze-Campen and Müller2018; Obersteiner et al. Reference Obersteiner, Walsh, Frank, Havlík, Cantele, Liu and van Vuuren2016; Popp et al. Reference Popp, Dietrich, Lotze-Campen, Klein, Bauer, Krause and Edenhofer2011; van Vuuren et al. Reference Van Vuuren, Kok, Lucas, Prins, Alkemade, van den Berg and Stehfest2015). One example is the Roads from Rio+20 study, which looked at a subset of the Sustainable Development Goals (PBL Netherlands Environmental Agency 2012). The study presented three pathways for achieving development and environmental goals. More recently, The World in 2050 reports (TWI2050 2018, 2019, 2020) have used multiple models to identify ambitious scenarios and their characteristics. Another approach is to translate the Sustainable Development Goals to a target space to test how a normative multidimensional objective can be met through different pathways, for example in van Vuuren and others (Reference Van Vuuren, Zimm, Busch, Kriegler, Leininger, Messner and Soergel2021), who use a limited set of indicators representative of the Sustainable Development Goals to make the targets more concrete and manageable.
Models can also be used for assessing how a specific policy goal may affect different Sustainable Development Goals. For example, several studies examined the impacts of increased use of biomass for modern energy and material purposes on the Sustainable Development Goals (Cavallett and Cherubini Reference Cavalett and Cherubini2018; Humpenöder et al. Reference Humpenöder, Popp, Bodirsky, Weindl, Biewald, Lotze-Campen and Müller2018; Wicke et al. Reference Wicke, van Leeuwen, Daioglou, de Bruin, Biermann and van Vuuren2022). They show how synergies and trade-offs can occur and how socio-economic factors or policy measures can help minimize trade-offs and boost synergies. These studies focus more on the environmental dimension than on other sustainability dimensions. This gap is a general challenge for integrated assessment models, when economic and social dimensions are less well covered (Allen, Metternicht and Wiedmann Reference Allen, Metternicht and Wiedmann2016; van Soest et al. Reference Van Soest, van Vuuren, Hilaire, Minx, Harmsen, Krey and Luderer2019; Zimm, Sperling and Busch Reference Zimm, Sperling and Busch2018). Figure 7.1 compares the importance of linkages between goals according to experts and the degree to which they are covered in models; it shows the strong coverage of models of economic growth, climate change, energy and consumption and productions, but less so on education, gender equality, health, peace and justice and governance. Still, there is some progress on covering inequality, poverty and living standards (e.g., Kikstra et al. Reference Kikstra, Mastrucci, Min, Riahi and Rao2021; Rao et al. Reference Rao, Sauer, Gidden and Riahi2019).
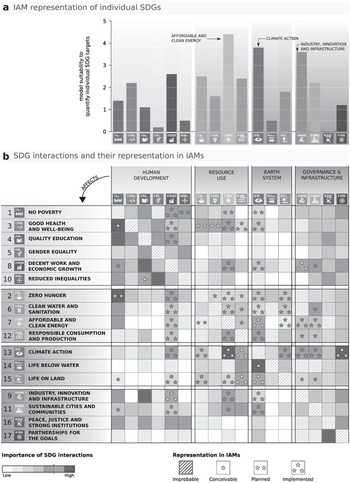
Figure 7.1 The representation of the Sustainable Development Goals by Integrated Assessment Models
The strengths of integrated assessment models and their scenarios are their projection ability, offering a consistent set of information between now and 2030 that allows to assess interactions between goals. However, the models themselves are complex and require many assumptions.
Many gaps also need to be closed by better covering the different dimensions of the goals in such models, such as human development and governance (O’Neill et al. Reference O’Neill, Carter, Ebi, Harrison, Kemp-Benedict, Kok and Pichs-Madruga2020; van Soest et al. Reference Van Soest, van Vuuren, Hilaire, Minx, Harmsen, Krey and Luderer2019; Zimm, Sperling and Busch Reference Zimm, Sperling and Busch2018). Integrating approaches from the social sciences and scenario modelling is necessary to better model the Sustainable Development Goals. Also, the level of granularity in terms of space and time and socio-economic heterogeneity of population groups is often insufficient to meet real-world challenges, and models struggle with potential disruptions and non-linear changes.
Full coverage of all interactions is impossible also owing to a lack of quantitative data. Sometimes, scenario narratives can help to provide consistency between model assumptions. Researchers have tried to make their models more accessible, transparent and replicable to facilitate uptake of the scenarios runs by different disciplines, for example in the Integrated Assessment Modelling Consortium initiative IAMC 1.5oC Scenario Explorer (Huppmann et al. Reference Huppmann, Kriegler, Krey, Riahi, Rogelj, Calvin and Zhang2019).
Promising areas for future development are the better representation of indicators to track progress and of interactions between goals to assess trickle-down effects of policies. For the latter, closer collaboration between scientists who work with other methods would be necessary. Such collaboration would also help in assessing scenario feasibility and developing scenarios that achieve multiple goals simultaneously.
Qualitative Case Studies
Qualitative case studies assess the steering effects in terms of the goals’ influence on decision-making and political discourses. This approach is widely used across the social sciences. A qualitative case study has been defined as ‘the intensive study of a particular case where the purpose of that study is – at least in part – to shed light on a larger class of cases’ (Gerring Reference Gerring2007: 20). Qualitative case studies on the steering effects of the Sustainable Development Goals look in detail at governance structures and policy processes to trace political changes in a specific jurisdiction and a particular period. In other words, with qualitative case studies, we can focus on the in-depth investigation of the implications of the adoption of the goals for an actor or institution, such as a country, city, business or civil society organization.
Numerous qualitative case studies are available on steering effects of the goals on political processes at global, national and local levels. Many are incorporated in the literature reviews conducted in the earlier chapters of this book. Some case studies examine the implementation of one or several goals in a country or region. Others take a broader perspective and explore the influence of several or all 17 goals on the behaviour of actors. Typical examples of qualitative case studies include empirical investigations of how the Sustainable Development Goals lead to changes in the institutional settings and policy landscape in countries (e.g., Breuer and Oswald Spring 2020; Forestier and Kim Reference Forestier and Kim2020; Horn and Grugel Reference Horn and Grugel2018; Tosun and Leininger Reference Tosun and Leininger2017); assessments of how the goals are locally implemented (e.g., Hickmann Reference Hickmann2021; Krellenberg et al. Reference Krellenberg, Koch, Schubert and Libbe2019) or evaluations of the emergence of public and private alliances and collaborations to attain the goals (Florini and Pauli Reference Florini and Pauli2018).
The main purpose of these case studies is to offer a detailed description and find crucial patterns of the case, using a particular analytical lens and theoretical concept. Scholars building on the qualitative case study method usually conduct content analyses of primary and secondary sources, undertake interviews or surveys, and sometimes engage in participatory observation such as at negotiations or in government agencies. By comparing cases, scholars zoom in on possible explanatory variables for implementing the Sustainable Development Goals, such as political leadership, congruence of stakeholder interests or pressure from civil society. In this way, scholars try to draw broader conclusions beyond the cases to find general enabling and constraining conditions for implementing the goals.
The case study method has several strengths. First, it is a good method to critically reflect and evaluate conceptual approaches (van Evera Reference Van Evera1997: 55–67), which contributes to the broader theoretical debate on the effectiveness of global goal-setting (Kanie and Biermann Reference Kanie and Biermann2017). Second, the case study method allows researchers to emphasize ‘substantively important cases’ (Mahoney and Goertz Reference Mahoney and Goertz2006: 242). While in quantitative studies, each case is equally important, researchers who use a case study approach often compare cases with diverse performance towards the Sustainable Development Goals. In this way, scholars can carve out key factors for the success or failure of governance interventions. Such selection strategies are based on prior knowledge about the relevant actors and institutions that deal with the Sustainable Development Goals. Third, a qualitative case study can also address broad research questions (Creswell Reference Creswell2009: 141). Compared to other methods that concentrate on specific issues and questions, qualitative case studies often start with broad questions about the wider impact of the goals. In addition, qualitative case studies look at the interlinkages of the goals and targets. A growing literature on nexus governance explores the overlap of institutions in interrelated policy domains. Scholars in this case use the Sustainable Development Goals as a point of reference and evaluate to what extent the integration of institutional responses leads to goal achievement (Hülsmann and Ardakanian Reference Hülsmann, Ardakanian, Hülsmann and Ardakanian2018; Schwindenhammer and Gonglach Reference Schwindenhammer and Gonglach2021; van Zanten and van Tulder 2020).
However, the case study method also has limitations. One problem is internal validity. Case study researchers exploring the steering effects of the Sustainable Development Goals cannot easily control the effect of alternative factors. For example, if a country has made progress on sustainability, this progress can either be owing to the adoption of the Sustainable Development Goals or to other developments, such as economic growth or broadening of social welfare. Scholars building on the case study method must try to control other factors and conditions to counter critiques that their findings are indeterminate (Collier, Seawright and Munck Reference Collier, Seawright, Munck, Collier and Brady2010: 47). A second problem of the case study method is external validity. Qualitative scholars analyse either a single or a few cases of the steering effects of the Sustainable Development Goals. Hence, to arrive at general claims that can be applied to other countries, cities or the corporate sector and civil society, qualitative case study researchers must generalize to a larger group of cases, which brings the danger that findings from one case do not apply to others (Lieberson Reference Lieberson1991; Munck Reference Munck2005).
Many scholars use the case study method to analyse the steering effects of Sustainable Development Goals. Qualitative case studies allow for an in-depth examination of the changes generated by the goals on specific institutions, policies, political programmes or implementation processes. The strength of the case study method is that it helps to evaluate theoretical approaches, focus on detailed empirical phenomena, and address broader research questions. However, internal and external validity are two shortcomings of this method. Researchers must thus apply the case study method with great care to minimize these limitations and maximize the strengths of this research. When acknowledging such limitations and strengths, qualitative case studies can produce in-depth empirical knowledge on the steering effects of goals on local or global policy-making, helping to identify enabling and constraining factors for the implementation of the goals.
Network Analysis
Network analysis is a method to assess the steering effects in terms of the goals’ influence on decision-making and political courses. Network analysis studies relations between entities. These entities are conceptualized as nodes of a network, and the relationships between them as ‘ties’ or ‘edges’. The relational pattern arising from these nodes and ties then forms the network. In principle, nodes and ties can be anything: the researcher defines what is of interest and, by extension, what the network is (Borgatti and Halgin Reference Borgatti and Halgin2011).
The main purpose of network analysis is to characterize networks as a whole or show the position of specific nodes in a network. Such analysis is valuable on its own and can be useful to find the most central actors in a field, for example in the international health aid community (Coscia et al. Reference Coscia, Hamaguchi, Pinglo and Giuffrida2018). In addition, these characteristics of networks and nodes may serve as independent or dependent variables for further analysis (Borgatti and Halgin Reference Borgatti and Halgin2011). For example, one can study whether human rights organizations that take up a central position in their network have a higher advocacy output (Murdie Reference Murdie2014); or how different network structures relate to coordination in agricultural development policy networks (Rudnick et al. Reference Rudnick, Niles, Lubell and Cramer2019).
Network analysis is applied in research on Sustainable Development Goals in two main ways.
First, it is used to assess interactions among goals and targets, which is essential for goal achievement but does not measure it directly. Instead, it can itself be seen as a steering effect of the goals that can be assessed by identifying interactions in different periods. For example, Kroll, Warchold and Pradhan (Reference Kroll, Warchold and Pradhan2019) highlighted successful transformations of trade-offs into synergies for some Sustainable Development Goals, which occurred between 2010 and 2016. Network analysis provides here insights into how goals and targets relate to each other and how interventions on one target can positively (synergies) or negatively (trade-offs) impact other targets. This analysis helps to find targets that are leverage points to intervene or hurdles in achieving the goals. Interactions between goals and targets have been conceptualized in different ways, including textual references (Le Blanc Reference Le Blanc2015), expert rating (Allen, Metternicht and Wiedmann Reference Allen, Metternicht and Wiedmann2019; Nilsson, Griggs and Visbeck Reference Nilsson, Griggs and Visbeck2016; Weitz et al. Reference Weitz, Carlsen, Nilsson and Skånberg2018), indicator data (Lusseau and Mancini Reference Lusseau and Mancini2019; Putra, Pradhan and Kropp Reference Putra, Pradhan and Kropp2020), indicator data combined with literature reviews (Zhou and Moinuddin Reference Zhou and Moinuddin2017) and expert knowledge (Anderson et al. 2021), or bibliometric data of academic publications (Ramirez et al. Reference Ramirez, Romero, Schot and Arroyave2019).
A second application of network analysis is to study the social networks of actors involved in the governance of the goals. To achieve the Sustainable Development Goals, a collective effort of a vast number of actors is required, including states and local governments, international organizations, non-governmental organizations, civil society and business (Kanie and Biermann Reference Kanie and Biermann2017; UNGA 2015). All these actors must coordinate and collaborate, and these interactions build up extensive global governance networks in which decision-making takes place (Eilstrup-Sangiovanni Reference Eilstrup-Sangiovanni, Victor, Montgomery and Lubell2016). Network analysis allows the analysis of these governance networks. For example, one can ask which actors take up the central coordinating positions in water governance networks (Angst et al. Reference Angst, Widmer, Fischer and Ingold2018); how civil society networks engage with the Southern African Development Community in regional governance (Hulse et al. Reference Hulse, Gürth, Kavsek, Stauber, Wegner and Weinreich2018); whether reciprocity is an influencer of networking patterns among international development organizations (Atouba and Shumate Reference Atouba and Shumate2010); or which international public administration has most online authority in global climate policy (Goritz et al. Reference Goritz, Schuster, Jörgens and Kolleck2020).
The main strength of network analysis is its focus on relations. The method builds on the assumption that the nodes in a network are not independent. Instead, nodes influence and are influenced by each other, not only as defined by their direct ties but also by nodes with which they connect through other nodes (Wasserman and Faust Reference Wasserman and Faust1994). Thus, a network can constrain or enable each node. Studying the nodes as separate units will thus neither lead to a complete understanding of their functioning, behaviour, or state nor an understanding of the entire network. In a world where global challenges and the actors dealing with them are increasingly interconnected, network analysis thus provides a valuable method to conceptualize, visualize and analyse those connected challenges and actors.
There are also difficulties. First, relational data is usually not readily available; obtaining it is often laborious, especially if one wants to assess changing networks over time. Moreover, network analysis is sensitive to missing data. If one of the critical nodes is missing, this may affect the entire network structure. Moreover, while network analysis can provide insights on the ‘bigger picture’ of relations in a system, it often falls short in providing details on the quality or strength of those relations.
Future network analysis applications for the study of the Sustainable Development Goals will most certainly involve more studies on goal interlinkages. Here, research on interlinkages at the local level will be significant, as relations between targets may differ based on localized context (Breuer, Janetschek and Malerba Reference Breuer, Janetschek and Malerba2019). In addition, the study of the structure, effects and effectiveness of (global) governance networks will remain important as institutions and actors are connected across the world. An understudied area here is how the Sustainable Development Goals themselves affect these networks. Given the emphasis of the goals on collaborative efforts and increased vertical and horizontal coordination (‘breaking down silos’), an important question is whether the Sustainable Development Goals are indeed changing governance or collaboration networks in any way (Bogers et al. Reference Bogers, Biermann, Kalfagianni, Kim, Treep and de Vos2021; Vijge et al. Reference Van Zanten and van Tulder2020). For example, one could ask whether there is increased collaboration between public and private actors, organizations working in different areas, national and local government, or cities globally. Answering such questions is critical to determine whether and to what extent the Sustainable Development Goals change actor relations and drive new partnerships for joint goal implementation.
Discourse Analysis and Interpretative Approaches
Discourse analysis and interpretative approaches address the steering effects in terms of the goals’ influence on decision-making and political courses. Discourse analysis is a method to study written, spoken or sign language to understand and unravel how ideas, concepts, opinions and norms become plausible social contexts (Cummings et al. Reference Cummings, Regeer, de Haan, Zweekhorst and Bunders2018; Wodak and Meyer Reference Wodak and Meyer2001). Discourse is understood as the ‘shared meaning of a phenomenon’ (Adger et al. Reference Adger, Benjaminsen, Brown and Svarstad2001: 683) that results from using a collection of ideas, concepts and categories (Hajer and Versteeg Reference Hajer and Versteeg2005: 175). The literature sometimes differentiates between ‘noncritical’ and ‘critical’ discourse analysis (Wodak and Meyer Reference Wodak and Meyer2001). While noncritical discourse analysis describes the formal characteristics of a text, critical discourse analysis seeks to analyse the ‘opaque as well as transparent structural relations of dominance, discrimination, power and control as manifested in language’ (Gee Reference Gee2011; van Dijk Reference Van Dijk, Tannen, Schiffrin and Hamilton2001; Wodak and Meyer Reference Wodak and Meyer2001: 2). To achieve that, critical discourse analysis looks at the ‘words-in-use’ and the ‘words-in-context’ in their broader socio-cultural practice, in addition to the discourse practice (Fairclough Reference Fairclough1995; Fairclough and Fairclough Reference Fairclough and Fairclough2012). In the following discussion, we draw specifically on critical discourse analysis, as it is the type of discourse analysis that best allows understanding progress in implementing the Sustainable Development Goals and what may be halting it.
Research employing critical discourse analysis in the context of the Sustainable Development Goals highlights that the goals are not necessarily transformative. Carant (Reference Carant2017), for instance, analyses the dominant and peripheral feminist discourses within the Sustainable Development Goals. She finds that some criticisms of liberal feminists (that is, feminists generally agreeing with the assumptions and solutions of liberal economic theories) concerning sexual and reproductive rights, human trafficking and a focus on women were incorporated in the Sustainable Development Goals. However, more transformative discourses of the ‘reinvention of democracy’ – that is, new ways of decision-making not limited to, or imposed by, the interests of powerful economic, patriarchal and political figures and nations – are seen as still lacking.
Similarly, Cummings and others (2018) examine the discourses of knowledge of the main policy document of the negotiations of the 2030 Agenda. They conclude that a techno–scientific–economic discourse is dominant at the level of goal implementation, thus excluding more transformational discourses. Cummings, Seferiadis and de Haan (Reference Cummings, Seferiadis and de Haan2019) take a genealogical approach to examine the presence of four discourses about the corporate sector in key texts of the Millennium Development Goals and the Sustainable Development Goals. They find that a pro-business discourse with unconditional support for the corporate sector is dominant within both sets of goals, but especially in the Sustainable Development Goals, reflecting the role of the corporate sector in their formulation. Ala-Uddin (Reference Ala-Uddin2019) shows that although the 2030 Agenda employs the language of global equality, justice and peace, the means of implementation and the proposed structure of global partnerships contradicts these principles. Similar observations have been made by Biermann and Kalfagianni (Reference Biermann and Kalfagianni2020), who show that despite an overall cosmopolitan vision of justice in the 2030 Agenda, the concrete means of implementation stated there are market-liberal, thus contradicting the aspirations of ‘leaving no one behind’.
Critical discourse analysis is a method particularly useful to study processes of inclusion and exclusion, dominance and marginalization, as these are expressed through language. In the context of the Sustainable Development Goals, this type of analysis allows identifying progress in including aspects previously excluded from global development agendas, such as in the Millennium Development Goals, but also to reveal that this progress remains superficial, as the dominant neoliberal logics prevail in the means of implementation of the Sustainable Development Goals.
There are also limitations regarding the application of this methodology, including the hermeneutic approach to text analysis, which may enter the subjectivities of the researcher into the analysis, the difficulties involved in distinguishing a discourse from what is not a discourse, as well as difficulties in showing the impact of a discourse on the objects of this discourse. For these reasons, critical discourse analysts argue that this research should be ‘intelligible in its interpretations and explanations’ (Titscher et al. Reference Titscher, Meyer, Wodak and Fetter2000: 164), that the process of collecting, analysing and explaining data should be recognizable, and that findings should be accessible and readable for the social groups under investigation (van Dijk Reference Van Dijk2006). Triangulation techniques are sometimes used here to enrich trustworthiness in the intersubjective validity of data. This means to make transparent and cross-check the openness and interchange between diverse types of data, interventions by participants and researcher interventions, interpretations and explanations (Fairclough Reference Fairclough1995; van Dijk Reference Van Dijk2006; Wodak Reference Wodak2007).
Future applications of critical discourse analysis for examining the steering effects of the Sustainable Development Goals are likely to expand beyond single goals and focus on their interlinkages. In addition, conflicting discourses and the actors, interests and power behind them need more attention to better understand the politics and diffusion mechanisms of the goals. Further, research that links discourse with practice is necessary to shed light on how the norms and ideas behind the goals are translated into their implementation.
Other Methods and Tools
There are many other methods in addition to the ones that we have just described; we now discuss some of these, without being able to be comprehensive.
Participatory research seeks to co-create knowledge with research participants by sharing the design of the research agenda, process and actions. Participatory research entails action in that the participants are not only engaged in the scientific inquiry but also in thinking of and implementing solutions for the problem at hand. In research on the Sustainable Development Goals, examples of the application of this method include the co-creation of pathways and knowledge for goal implementation at the local level (Lepore, Hall and Tandon Reference Lepore, Hall and Tandon2020; Szetey et al. Reference Szetey, Moallemi, Ashton, Butcher, Sprunt and Bryan2021), as well as the development of participatory methods to study the Sustainable Development Goals in academic curricula (Trott, Weinberg McMeeking Reference Trott, Weinberg and McMeeking2018).
Besides the modelling approaches for projecting future developments addressed earlier, other quantitative and qualitative forecasting and foresight methods are used. Trend analysis is one of the quantitative forecasting methods used to assess whether progress in achieving the goals is enough to meet the 2030 Agenda. Various tools such as the SDG Atlas (https://datatopics.worldbank.org/sdgatlas/) and SDG Dashboard (https://dashboards.sdgindex.org/) include results from trend analysis, highlighting that many countries are not on track in attaining the Sustainable Development Goals (Sachs et al. Reference Sachs, Schmidt-Traub, Kroll, Lafortune, Fuller and Woelm2020; United Nations Reference Goritz, Schuster, Jörgens and Kolleck2020). Qualitative foresight methods aim to anticipate multiple possible futures, to develop desired visions for sustainable futures or to define concrete steps for achieving a specific, selected envisioned future; this is often done in a participatory manner, engaging different stakeholders and working with different levels of participation (van den Ende et al. 2021).
Another example is computational text analysis or quantitative content analysis to identify how written or spoken text relates to the Sustainable Development Goals. Analysis methods include dictionary methods (keyword searches) and machine learning algorithms to classify large sets of texts to the Sustainable Development Goals. In some cases, researchers are interested in whether the goals and their targets are mentioned in the text, such as investigating cherry-picking of the Sustainable Development Goals (Forestier and Kim Reference Forestier and Kim2020). It is often of interest whether and to what extent the topics embedded in the Sustainable Development Goals are addressed in large bodies of text. Recent work in this area includes classifying publications of the United Nations Department of Economic and Social Affairs to the Sustainable Development Goals (LaFleur Reference LaFleur2019), mapping the EU Recovery Plan to the Sustainable Development Goals (Borchardt et al. Reference Borchardt, Barbero-Vignola, Buscaglia, Maroni and Marelli2020), identifying whether start-ups contribute to the Sustainable Development Goals (Horne et al. Reference Horne, Recker, Michelfelder, Jay and Kratzer2020) and assessing how the United Nations General Assembly resolutions mention the Sustainable Development Goals (Kim and LaFleur Reference Kim and LaFleur2020).
Besides these methods for scientific research, online tools have been developed to make the study of and support of decision-making on the Sustainable Development Goals more accessible to non-scientific audiences. For example, tools such as the SDG Tracker (https://sdg-tracker.org), the World Bank SDG dashboard (https://datatopics.worldbank.org/sdgs), and the SDG dashboards of the Sustainable Development Solutions Network (https://dashboards.sdgindex.org) present available data on indicators in an interactive manner so that process made on achieving the goals can be tracked openly. Additional to tracking the process, there are tools to understand goal interactions and interlinkages. For example, the SDG Synergies tool (https://www.sdgsynergies.org) focuses on interactions and interlinkages based on expert opinions and network analysis (Nilsson, Griggs and Visbeck Reference Nilsson, Griggs and Visbeck2016; Weitz et al. Reference Weitz, Carlsen, Nilsson and Skånberg2018); the SDG Impact Assessment Tool (https://sdgimpactassessmenttool.org) elicits the knowledge of users to qualify impacts and interactions (Johnsson et al. Reference Goritz, Schuster, Jörgens and Kolleck2020; Olfe-Kräutlein Reference Olfe-Kräutlein2020). Increasingly, commercial tools have also been developed for corporations to align their activities to achieving the Sustainable Development Goals. For example, the SDG Compass (https://sdgcompass.org) aims at instructing companies on, among other things, how to monitor and manage working towards achieving the Sustainable Development Goals. The Sustain2030 tool (https://icondu.de/sustain2030/en/) helps the private sector to make sustainable decisions in line with the Sustainable Development Goals, accounting for interlinkages and interactions. The SDG Monitor (https://www.sdgmonitor.co/) supports private sector activities to be paired with the Sustainable Development Goals by assessing their impact based on a sustainability index.
Conclusions and Future Directions
The steering effects of the Sustainable Development Goals can be assessed in terms of their influence on political decision-making and discourse, on the one hand, and on the progress towards achieving the goals on the other. The usefulness of methods to study these aspects depends on the question at hand. The strength of each method varies across different dimensions, including the main focus, the temporal perspective (retrospective or prospective); geographical scale (from local to global); the coverage of topics and areas (focus on single goals or many); and interactions and interlinkages.
We summarize the main discussion and some of the main characteristics in Table 7.2. Interactions and interlinkages have been studied with different methods by different disciplines and scientific communities, which indicates the diversity of perspectives that can be taken and the importance of interactions and interlinkages for achieving the Sustainable Development Goals.
Table 7.2. Overview of key methods, the type of steering effects of the Sustainable Development Goals that they address, their main purpose and the four key dimensions, illustrating their typical uses
Method | Monitoring approaches | Model-based scenario approaches | Qualitative case studies | Network analytical approaches | Discourse analysis and interpretative approaches | |
---|---|---|---|---|---|---|
Identification of interactions | Governance networks | |||||
Elements of effecta | Monitor progress | Monitor progress | Impact on discourse & decision-making | Not applicable d | Impact on discourse & decision-making | Impact on discourse & decision-making |
Purpose method | Monitor progress towards goal achievement over time or compare countries | Assess implications of policies; Explore possible futures & their progress; Identify preconditions for maximising benefits and minimising burdens | Investigate steering effects on political processes, including institutional settings, policy-making and implementation; Primarily looking at how goals affect actors and institutions | Assess interactions between goals and targets | Assess social networks of actors involved in governance of sustainable development | Assess progress and barriers to implementation of the Sustainable Development Goals |
Temporal scale | Retrospective | Mainly prospective | Retrospective | Retrospective | Retrospective | Retrospective |
Geographical scaleb | Local to national resolution; comparison across countries globally | Local to global; spatially specific or administrative units such as country or world regions | Local to global | Most often, global or national | Most often, global or regional | Local to global |
Coveragec | Comprehensive | Multiple goals | Single goal to comprehensive | Two or more targets or goals | Single to multiple goals | Single to comprehensive |
Interlinkages | Broad understanding of how interactions unfolded in the past | Knowledge of the impacts of future interventions across several goals | Some case studies look at interlinkages of goals and targets | Understanding of how targets or goals relate to each other and what is key for intervention | Not addressed | Not addressed in the examined literature; a potential topic of future research |
Illustrative example | United Nations (Reference Goritz, Schuster, Jörgens and Kolleck2020), World Bank (2020), Pradhan et al. (Reference Pradhan, Costa, Rybski, Lucht and Kropp2017) | Allen et al. (Reference Allen, Metternicht and Wiedmann2019), van Soest et al. (2019), Collste, Pedercini and Cornell (2017) | Horn and Grugel (Reference Horn and Grugel2018), Breuer and Oswald Spring (Reference Goritz, Schuster, Jörgens and Kolleck2020) | Lusseau and Mancini (Reference Lusseau and Mancini2019), Weitz et al. (Reference Weitz, Carlsen, Nilsson and Skånberg2018) | Goritz et al. (Reference Goritz, Schuster, Jörgens and Kolleck2020), Hulse et al. (Reference Hulse, Gürth, Kavsek, Stauber, Wegner and Weinreich2018) | Carant (Reference Carant2017), Cummings et al. (Reference Cummings, Seferiadis and de Haan2019), Ala-Uddin (Reference Ala-Uddin2019) |
a We define two elements of steering effects in this chapter: first, methods that assess how the Sustainable Development Goals affect policy-making and political discourse at local to global scales, including examining the development of policy tools or assessing the degree to which people have internalized Sustainable Development Goals in their decision-making (abbreviated here as ‘impact on discourse & decision-making’), and second, progress towards the actual achievement of the Sustainable Development Goals, or the lack thereof (abbreviated here as ‘monitor progress’).
c Coverage of topics and issues is classified as focus on single goals or sustainability topic being investigated; multiple goals or topics; comprehensive goal coverage or sustainability topics.
d Understanding interactions is essential for goal achievement but does not measure it directly. Instead, it can itself be considered a steering effect (see also section Network analysis).
As for the temporal perspective, most methods focus on retrospective analysis, with scenario-based modelling being the only method that allows assessing future scenarios and policy options and their implications for reaching the Sustainable Development Goals. While most methods could be applied from local to global scale, they are typically used on only one or two scales. For example, integrated assessment models are mainly used at the global level, and monitoring approaches at national and local levels.
Regarding coverage, some methods address all goals (for example, the SDG Index and the Global Indicator Framework for SDGs), while others are mainly used for specific groups of goals, for example in some integrated assessment models (Allen, Metternicht and Wiedmann Reference Allen, Metternicht and Wiedmann2016; van Soest et al. Reference Van Soest, van Vuuren, Hilaire, Minx, Harmsen, Krey and Luderer2019), or for only one goal (e.g., Akuraju et al. Reference Akuraju, Pradhan, Haase, Kropp and Rybski2020). Such cherry-picking of goals, however, cannot reflect the holistic and integrative nature of the 2030 Agenda. The Sustainable Development Goals are a system of interacting components, not a collection of goals, targets and indicators (Pradhan Reference Pradhan2019). Covering more goals is therefore important in future research.
The implementation of the Sustainable Development Goals can bring synergies as well as trade-offs. Sufficient understanding of how interactions within and across the goals unfold is crucial for achieving the goals, because synergies can leverage the achievement of the 2030 Agenda while trade-offs can make it impossible. Such trade-offs hence need to be tackled and made at least non-obstructive, so that progress on one goal or target does not hinder progress on another. Both quantitative and qualitative methods are therefore needed to understand synergies and trade-offs among goals. When empirical data are available, quantitative methods can generate evidence and understanding of goal interactions. In case of data limitation, qualitative methods can complement this.
Methods are often used in combination with others. For example, expert interviews, participatory research or surveys could offer insights into interlinkages between goals and targets, and these data could then be analysed through network analysis or applied to specify interlinkages in models. Indicators can assess progress but also to understand how interlinkages, discourse analysis and interviews can feed into qualitative case studies, and so on. This building on to each other of different methods makes the distinction between approaches sometimes hard and even arbitrary. Nevertheless, it is important to look at both elements of steering effects of the Sustainable Development Goals simultaneously.
Looking at the full set of the methods that we assessed, some overarching observations are evident that also point to directions for future research.
No single method can give a comprehensive overview of steering effects.
Only studies that combine methods to look at the impact on decision-making and discourse and goal achievement can provide a complete picture. Looking particularly at the two elements of steering effects identified in this chapter, various methods address only one element. However, we need a better understanding of how the influence of goals and targets on political decisions and discourses interacts with progress towards achieving the Sustainable Development Goals.
For a comprehensive overview we need to bring together the results of different methods and study the steering effects of global goals in an interdisciplinary manner. Closer cooperation within and between research communities can help close the remaining gaps. For example, while model studies can identify how much air pollutant emissions need to be reduced to meet the air quality guidelines of the World Health Organization, such studies can tell little about how these reductions can be achieved, that is, which actors need to be involved and what legislation is needed. Nor can they identify the role of the Sustainable Development Goals. In other words, interdisciplinary cooperation and mixed methods approaches are needed. Interdisciplinary research brings together different theoretical perspectives in one study and can foster methodological innovation. Some examples already exist that employ mixed methods to understand the steering effects of the Sustainable Development Goals. For example, network analysis is often employed alongside other methods to quantify relationships between sets of indicators, including statistical techniques (Mainali et al. Reference Mainali, Luukkanen, Silveira and Kaivo-oja2018), expert opinions (Weitz et al. Reference Weitz, Carlsen, Nilsson and Skånberg2018) or text analysis (Le Blanc Reference Le Blanc2015). Also, statistical techniques have been integrated with literature reviews and network analysis (Somanje et al. Reference Somanje, Mohan, Lopes, Mensah, Gordon, Zhou and Takeuchi2020) or in combination with dynamical system modelling (Spaiser et al. Reference Spaiser, Ranganathan, Swain and Sumpter2017). Finally, also integrated models have been applied in combination with different methods. Collste, Pedercini and Cornell (Reference Collste, Pedercini and Cornell2017) employed qualitative causal loop diagrams as the basis for developing a systems dynamics model.
There is a critical lack of data.
Overall, lack of quantitative and qualitative data complicates research on the Sustainable Development Goals, especially with regard to data at local levels, data of low-income countries and data collected and reported in other languages than English. This is not surprising, as data collection is difficult and expensive even for countries with advanced statistical systems (MacFeely Reference MacFeely2018). Notably, data are not systematically collected on policy changes related to the Sustainable Development Goals. Such data are collected only on a case-by-case basis and often in qualitative research. Such research thus remains restricted to the documents studied and to information from people that the research team had access to, which results in biases towards texts in the English language and generally the Global North. While this is problematic in any field, it is more so when researching goals that are be implemented globally.
Not all Sustainable Development Goals are covered equally in research.
Third, methods vary in the degree to which they cover the Sustainable Development Goals. Although many methods can address multiple goals, only few can comprehensively address all 17 goals. Given the interconnected nature of goals and targets, increased goal coverage is needed to study the interactions. Qualitative methods such as case studies and discourse analysis can comprehensively address the goals. Of the quantitative methods, only studies using indicators and indices can address all goals, but they are constrained by data availability, as discussed. Other modelling approaches are suited to address multiple goals or targets but cannot cover them comprehensively. These models selectively study specific goals and targets but miss key synergies or trade-offs. However, at the same time, adding the possibility to study more goals in modelling approaches may also not necessarily be suitable or even possible owing to increased complexity or numerical limitations.
Interlinkages among Sustainable Development Goals must be better addressed.
A critical aspect of the Sustainable Development Goals is their interactions. Accounting for the interactions allows identifying synergy and trade-offs. Some have tried to capture the interlinkages – for instance, in the use of models, but also monitoring approaches or more social science-based methods. However, as shown by van Soest and colleagues (Reference Van Soest, van Vuuren, Hilaire, Minx, Harmsen, Krey and Luderer2019), the coverage of linkages is still limited and primarily for specific clusters only.
In addition, there are interactions across scale: Advancing towards achievement of the Sustainable Development Goals in one locality affects the ability of impact-receiving places to meet their goals (Engström et al. Reference Engström, Collste, Cornell, Johnson, Carlsen, Jaramillo and Fuso-Nerini2021). Addressing spill-overs when designing sustainable development actions is imperative to connect the ‘global indivisibility’ of the agenda with local and national implementation of the Sustainable Development Goals. Recent initiatives to account for spill-overs have focused on national indexes; see SDG Index and Dashboards (Sachs et al. Reference Sachs, Schmidt-Traub, Kroll, Lafortune, Fuller and Woelm2020). However, indexes and indicators do not provide guidance on the impacts of new actions. While models describe some of the relationships, model complexity, data demands and computational time, these models may not be suitable for application at the local level (Engström et al. Reference Engström, Destouni, Howells, Ramaswamy, Rogner and Bazilian2019). Another challenge is to analyse which type of institutions and governance mechanisms facilitate the design and implementation of integrated goal achievement. If interlinkages between goals and targets cannot be ‘translated’ into a tangible policy process, integrated implementation will not be successful.
Developing science-based targets.
A critical reflection is also needed on how the Sustainable Development Goals are developed and negotiated. Compared to the Millennium Development Goals, more attention was paid to represent perspectives of stakeholders, including scientists, while setting up the Sustainable Development Goals. The set of goals, targets and indicators were negotiated in the United Nations system through an iterative process considering political preferences, science arguments and data availability. The question arises to what level these Sustainable Development Goals in the end are science-based, or whether they are purely policy targets. Global goals have the potential to steer social, environmental and economic systems towards desirable directions. Therefore, it is crucial to base these goals on science and evidence. A methodological challenge is then to develop global goals based on science-based targets that also account for sustainable governance of global and local commons to ensure societal well-being and public and planetary health.
In conclusion, no single method can comprehensively study the steering effects of global goals. All methods have strengths and weaknesses and contribute their part to the overall assessment whether we are on track with implementing the Sustainable Development Goals. Only together can the methods yield a clearer picture of reality. To adequately assess and communicate to users whether we are on track in attaining the global goals, we need to further develop our methods but also to better promote the use of this information in the science–policy interface.