Reading fluency and text comprehension are fundamental for school achievement across a variety of disciplines. Yet, international comparisons, such as PIRLS, suggest that 10 out of 50 countries, in which reading comprehension was tested with grade 4 children, show alarmingly low average scores (Mullis et al., Reference Mullis, Martin, Foy and Hooper2017). The situation seems particularly problematic in France, which ranked 34th out of 50. Indeed, 6% of the students in France versus 4% in Europe have a standard score below 400, which is taken as an indicator that they do not master elementary reading and comprehension skills (Mullis et al., Reference Mullis, Martin, Foy and Hooper2017). Since the first PIRLS evaluation in 2001, performance in reading comprehension of French students has dropped year after year, especially for complex reading comprehension skills (e.g., inference) and informative texts (e.g., scientific or documentary texts). In the recent national evaluations, in which 780,000 grade 2 students in France were tested on reading fluency and reading comprehension (Andreu et al., Reference Andreu, Cioldi, Conceicao, Etève, Fabre, Tidiane Ndiaye, Portelli, Rocher, Vourc’h and Wuillamier2019), it was also found that 22% of the students had problems in understanding a written sentence and that 30% read less than 30 words per minute. At that reading speed, it would take a child more than 9 hr to read Saint Exupery’s The Little Prince compared to a skilled reader who is able to read the novel in about 1 hr Footnote 1 . In this present article, we explore to what extent text simplification might be a viable tool for increasing reading fluency and text comprehension in normal readers who attend regular primary schools (grade 2) in France.
What does it take to understand a written text?
According to the most influential theory, the simple view of reading (Gough & Tunmer, Reference Gough and Tunmer1986; Hoover & Gough, Reference Hoover and Gough1990), reading comprehension (R) is simply the product of word identification or decoding ability (D) and oral comprehension (C), which leads to the straightforward equation R = D × C. Although it has been questioned to what extent the components are really independent (Ouellette & Beers, Reference Ouellette and Beers2010; Tunmer & Chapman, Reference Tunmer and Chapman2012) and whether one would need to take fluency (Adlof et al., Reference Adlof, Catts and Little2006; Silverman et al., Reference Silverman, Speece, Harring and Ritchey2013) or vocabulary into account (Tunmer & Chapman, Reference Tunmer and Chapman2012), this simple equation has been confirmed in a variety of languages (Johnston & Kirby, Reference Johnston and Kirby2006; Joshi & Aaron, Reference Joshi and Aaron2000; Kirby & Savage, Reference Kirby and Savage2008) and grade levels (Kershaw & Schatschneider, Reference Kershaw and Schatschneider2012; Tilstra et al., Reference Tilstra, McMaster, Van den Broek, Kendeou and Rapp2009). In a recent 5-year longitudinal study with Norwegian grade 2 readers (N = 198), Lervåg and colleagues (Reference Lervåg, Hulme and Melby-Lervåg2018) have shown that one can explain 96% of the variance in reading comprehension growth by carefully estimating decoding and oral comprehension skills (vocabulary, grammar, verbal working memory, and inference skills).
The multiplicative nature of the relationship implies that if word identification is weak (D → 0), written comprehension will be impaired even if oral comprehension were good. This is the case for children with dyslexia who exhibit difficulties in understanding a written text despite fairly normal oral comprehension (Bishop & Snowling, Reference Bishop and Snowling2004; Perry, Zorzi & Ziegler, Reference Perry, Zorzi and Ziegler2019; Ziegler, Perry & Zorzi, Reference Ziegler, Perry, Zorzi, Perfetti, Pugh and Verhoeven2019). The equation further implies that if oral comprehension is deficient (C → 0), written comprehension is impaired despite good word identification skills. This is the case for children with poor vocabulary or poor language skills, who also exhibit deficits in reading comprehension despite the fact that might correctly decode all of the words in a text (Bishop & Snowling, Reference Bishop and Snowling2004; Perry et al., Reference Perry, Zorzi and Ziegler2019; Wauters et al., Reference Wauters, Van Bon and Tellings2006; Ziegler et al., Reference Ziegler, Perry, Zorzi, Perfetti, Pugh and Verhoeven2019). Indeed, in fluent skilled readers who have fully automatized word identification (D → 1), written comprehension is almost entirely explained by oral comprehension (Gentaz et al., Reference Gentaz, Sprenger-Charolles and Theurel2015).
The second theory that is highly relevant in the context of text simplification is the lexical quality hypothesis (Perfetti, Reference Perfetti2007). Lexical quality refers to the extent to which the reader’s knowledge of a given word represents the word’s form and meaning constituents and knowledge of word use that combines meaning with pragmatic features. The lexical quality theory implies that variation in the quality of lexical representations, including both form and meaning knowledge, lead to variation in reading skill, including comprehension. The consequences of lexical quality can be seen in processing speed at the lexical level, and, especially important, in comprehension. At the extreme end, low-quality representations lead to specific word-related problems in comprehension.
This rapid review of the literature shows that in order to increase text comprehension, one can influence the ease of word identification (decoding), the quality of lexical representations, or the quality of oral comprehension. While changing oral comprehension skills and the quality of lexical representations requires early intensive intervention (Bowyer-Crane et al., Reference Bowyer-Crane, Snowling, Duff, Fieldsend, Carroll, Miles and Hulme2008), word identification can be facilitated “on the fly” by text simplification (see below). This was the approach chosen in the present study. By virtue of the equation presented above, if word identification is made easier, text comprehension should be improved. Similarly, if words with poor lexical quality are replaced by words with better lexical quality, processing speed and comprehension should be improved. Of course, simpler words are also more likely to be in the vocabulary of the child, which is an “oral-language” factor that goes beyond word identification.
Text simplification
Text simplification can operate at different linguistic levels (lexical, morphosyntactic, and discursive) in order to make texts more readable while preserving their original content (Saggion, Reference Saggion2017). Lexical simplification is concerned with providing access to the ideas with fewer words. Common strategies to carry out lexical simplification are the use of superordinate terms, approximation, synonymy, transfer, circumlocution, and paraphrasing (Blum & Levenston, Reference Blum and Levenston1978). Although simpler words are usually more frequent and shorter, one needs to consider the characteristics of the target population for the definition of simplicity (what may be simple for low-vision patients might not be simple for children with dyslexia or illiterates). Word frequency is the major variable that affects the ease of word recognition (e.g., Coltheart et al., Reference Coltheart, Rastle, Perry, Langdon and Ziegler2001). Syntactic simplification consists in reducing the complexity of syntactic structures by deleting or replacing complex constructions (Brouwers et al., Reference Brouwers, Bernhard, Ligozat and François2014), such as relative or subordinate and coordinate clauses. Discursive simplifications address phenomena, such as sentence reordering, explicitness of coreference chains, or anaphora resolution. Given that pronouns are a source of ambiguity and syntactic complexity (Bormuth, Reference Bormuth1966), the replacement of a personal pronoun by a noun reduces the amount of processing inferences a reader has to do in order to link one referring expression to its antecedents (Wilkens & Todirascu, Reference Wilkens and Todirascu2020). Other forms of simplification can also be used to increase the personalization level of texts, reorganize ideas, reduce the density of ideas, or summarize ideas (Clerc & Kavanagh, Reference Clerc and Kavanagh2006).
Trying to reduce the complexity in texts is not a new idea. For instance, publishers have offered “simplified” editions of classic literature, in which parts of the original texts have been removed to make the texts more accessible to young readers. Although this method shortens the reading material, it does not tackle the problem of simplification of the text itself. To do so, several strategies have been proposed. One possible way is to create a simplified text ex nihilo, that is, to write a text that responds to “simplicity” instructions based on linguistic and textual recommendations. In this case, there is no original text considered as complex or difficult. An example is the collection La Traversée (Weyrich Editions), a collection of short novels specifically written for adult illiterates or weak readers. To create these texts, the writers had to respect a set of specifications, which served as a guide, and they benefited from feedback on their texts from a sample of target readers. Another example is the Colibri collection (Belin Edition), which proposes books that are written for readers with dyslexia following a number of editorial rules. Each book belongs to one of four difficulty levels, which take into account the complexity of French grapheme-to-phoneme correspondences. The stories are accompanied by educational activities to facilitate their reading and check their comprehension.
The second simplification strategy is to transform an original text so that it becomes more accessible to a specific readership. The first emblematic example is Plain Language Footnote 2 which was set up by the American administration in the 70s in response to the observation that a significant part of the population was not able to understand administrative documents. The guidelines focused on avoiding complicated sentences (administrative jargon and negative sentences) and included stylistic recommendations, such as, using personal pronouns, short paragraphs, and visual layout elements (titles, lists, etc.). Another example is that of encyclopedic texts for children. For example, Vikidia proposes articles with simplified content for young readers, and the Dyscool collection (Nathan Edition) proposes 16 French books where the texts are adapted in various ways. Complex sentences are rewritten with short and simple sentences keeping the standard order (subject–verb–object). Complex or long words are explained or replaced by easier and shorter words. Stylistic figures and characters are made explicit or replaced by easier referents. Finally, for English learners, the Newsela Footnote 3 corpus is composed of 1,911 original news articles, for which professional writers created 4 equivalent versions for different reading levels.
The effects of text simplification on reading speed or comprehension have been investigated in previous studies. For instance, Margarido and colleagues (Reference Margarido, Pardo, Antonio, Fuentes, Aires, Aluísio and Fortes2008) conducted several experiments on summarization and text simplification for poor readers, more specifically, functionally illiterate readers in Brazil. They used various summaries as the basis of their simplification strategy and showed that each simplification approach had different effects depending on the level of literacy, but that all of them improved text understanding at some level. Gala and Ziegler (Reference Gala and Ziegler2016) investigated the benefits of text simplification for children with dyslexia. They asked 10 children with dyslexia to read aloud the original and manually simplified version of standardized French texts and to answer comprehension questions after each text. The results showed that the simplifications increased reading speed and reduced the number of reading errors (mainly lexical) without a loss of understanding. In a similar line of research, Rello and collaborators (Reference Rello, Baeza-Yates, Dempere-Marco and Saggion2013) found that lexical simplification (i.e., replacing complex words by simpler equivalents) improved both reading speed and comprehension for readers with dyslexia. In an eye-tracking experiment with 23 dyslexics and 23 controls, they compared texts in which words were substituted by shorter and more frequent synonyms. The use of more frequent words allowed participants with dyslexia to read faster, while the use of shorter words allowed them to better understand the text. The beneficial effects of simplification were only present for readers with dyslexia but not for the controls.
Fajardo and colleagues (Reference Fajardo, Tavares, Ávila and Ferrer2013) investigated whether adding cohesive elements of texts such as connectives (e.g., but, in contrast) and high-frequency words would improve inferential comprehension in poor readers with intellectual disability. Neither the addition of high-frequency content words nor connectives produced inferential comprehension improvements. Although the average performance of a reading level-matched control group (typically developing children) was similar to the group of poor readers with intellectual disability, the pattern of interaction between familiarity and type of connectives varied between groups.
These results by Fajardo et al. (Reference Fajardo, Tavares, Ávila and Ferrer2013) suggest that the beneficial effects of text simplification should not be taken for granted. Indeed, simplifying on the basis of overly simplistic criteria (e.g., length of sentences and words) can lead to producing texts that are less comprehensible in terms of logical links (Davison & Kantor, Reference Davison and Kantor1982) or texts whose style is neglected in terms of rhythm, variety of linguistic forms, and coordination and subordination strategies (Selzer, Reference Selzer1981). Also, removing connectives (e.g., therefore, furthermore) has been shown to have a detrimental effect on reading speed and comprehension, especially when texts have a continuous layout (Van Silfhout et al., Reference Van Silfhout, Evers-Vermeul, Mak and Sanders2014).
In sum, there is some evidence that text simplification provides a viable tool to increase reading comprehension in special-needs populations, such as illiterate or dyslexic readers (Gala & Ziegler, Reference Gala and Ziegler2016; Margarido et al., Reference Margarido, Pardo, Antonio, Fuentes, Aires, Aluísio and Fortes2008; Rello et al., Reference Rello, Baeza-Yates, Dempere-Marco and Saggion2013; but see Fajardo et al., Reference Fajardo, Tavares, Ávila and Ferrer2013). It is an open question as to whether text simplification can be useful for normally developing beginning readers and whether there is an additional benefit for those who have weak oral language skills, poor decoding skills, or weak cognitive abilities. To our knowledge, few studies investigated the effects of text simplification on different types of text (but see Fajardo et al., Reference Fajardo, Tavares, Ávila and Ferrer2013).
Goal of the present study
The overall goal of our study was to test whether text simplification could improve reading fluency and comprehension for normally developing children learning to read in French and whether the cognitive or language profile of the children would modulate the size of simplification benefits. To address these questions, we manually simplified 10 narrative and 10 scientific texts. Both genres are commonly used in primary school, but their objectives differ. On the one hand, literary texts reflect the world view and the sensitivity of its author. They are not necessarily meant to be useful or instructive. On the other hand, scientific (documentary) texts are descriptive and explanatory with a logical structure based on scientific reasoning. They tend to describe a scientific or technological causality. The variables that determine reading comprehension are quite similar for both types of texts (e.g., Best et al., Reference Best, Floyd and McNamara2008; Liebfreund & Conradi, Reference Liebfreund and Conradi2016). We were interested in comparing simplification for the two kinds of texts because the decline in comprehension scores in French primary students in the PIRLS study was most pronounced for informational texts.
In our study, all children were presented with informational and literary texts either in its original or simplified version (counterbalanced across participants). The texts were embedded in an iBook application for iPads, which allowed us to present texts sentence by sentence Footnote 4 . It could be argued that presenting texts sentence by sentence is a simplification on its own. Indeed, it has been shown that presenting texts line by line on electronic devices can improve reading speed and comprehension for struggling readers (Schneps et al., Reference Schneps, Thomson, Chen, Sonnert and Pomplun2013). However, we presented both types of texts (normal and simplified) line by line on an electronic device. Therefore, any difference between normal and simplified texts cannot be explained by this presentation mode.
After reading each sentence, the child had to press an on-screen spacebar to receive the following sentence, which made it possible to estimate an approximate fluency measure by dividing the total reading time (RT) of the sentence by the number of words or characters that made up the sentence. Finally, after the silent reading of each text, children received a set of multiple-choice comprehension questions, which were identical in the original and simplified version of the text. We hypothesized that simplified texts should be read more fluently than original texts. We further hypothesized that the simplified version of a text should obtain higher comprehension scores than the original version. We finally hypothesized that simplification should be more beneficial for poor readers and children with weak oral language skills.
Methods
Participants
A total of 165 grade 2 students (81 girls and 84 boys) from 9 classes were recruited in 4 different schools located in 3 different towns in the south of France (Sanary sur Mer, Saint Cyr, La Cadière d’Azur). These towns host a suburban population with little immigration and average-to-high socioeconomic status. The departmental school authorities pseudo-randomly selected the schools on the basis of them being fully equipped with connected iPads and the school principals agreeing to participate in the study.
All participants were native speakers of French. Three of them were bilingual (Portuguese, Arabic, and Bulgarian), but there was no reason to discard them from the study. The average age of the students was 92.9 months (7.7 years). Ethics approval was obtained from the institutional review board of Aix-Marseille University. The departmental school authorities authorized the study. Parents gave informed written consent.
Procedure
The study comprised two phases: (a) an individual testing phase made up of four sessions of 30 min (see below) whose purpose was to obtain a variety of linguistic, cognitive, and psycholinguistic measures for each participant and (b) a classroom phase, in which children silently and autonomously read the texts on an iPad (from March to June 2017). Each student read 20 texts, 10 were literary texts (5 original and 5 simplified) and 10 were scientific texts (5 original and 5 simplified). Each text was only read once by the same student, either in its original or simplified version. Thus, simplification and nature of the text were within-subject manipulations.
After reading a given text, the subjects had to answer a multiple-choice questionnaire (MCQ) designed to measure their comprehension of the text. The MCQ comprised five comprehension questions, each of which had three alternatives (correct, related to the text but incorrect, and unrelated to the text and incorrect). The questions were constructed to avoid reliance on cultural background knowledge. Importantly, the questions were identical in the original and in the simplified versions, and the students could not go back to the text in order to answer them. The order of questions and alternatives was randomized between participants. In order to motivate the students, we implemented the reading tests as a game, in which they could win rewards (stars) proportionally to the amount of correct answers provided. In case of hesitation, the student could change his/her answer before validating it and moving on to the next question.
We implemented the reading tests and the comprehension task on iPads using an iBook template. In general, the texts were presented sentence by sentence. This allowed us to collect RTs per sentence and hence estimate the average reading speed per word. Occasionally, we presented more than one sentence at once if a given sentence was meaningless without the one preceding or following it or in a dialog situation, in which the same character “pronounced” two sentences before taking turns. Importantly, when a long sentence from the original text was cut into two sentences in the simplified version of the same text, we also presented the two sentences at once to keep the amount of semantic information that is presented similar between the original and simplified versions of the same text.
Materials
We selected 10 narrative (tales, stories) and 10 scientific texts (documentaries), which were in line with the national school program for that grade level. The scientific texts were extracted from open-access scientific resources for children in primary school (Wapiti, Bibliothèque de Travail Junior, or Images DOC). For literary texts, we used extracts from traditional children’s literature used in schools, such as Antoon Krings’ collection Drôles de petites bêtes [Funny little creatures], J’aime lire [I like reading], or Réné Goscinny and Jean-Jacques Sempé's novel Le Petit Nicolas [The little Nicolas]. The average length of the overall original texts was 243 words (range: 202–252 words). The texts used in the experiment are part of the Alector corpus (see Appendix A, Gala et al., Reference Gala, Tack, Javourey-Drevet, François and Ziegler2020a).
Text simplifications were carried out manually by a group of researchers from education, cognitive psychology, linguistics, and speech and language therapy. The simplifications mainly focused on the lexical and syntactic level and mainly consisted of substituting long and infrequent words by shorter and more frequent synonyms and by changing complex syntactic structures (Gala et al., Reference Gala, François, Javourey-Drevet and Ziegler2018). Long words were either (a) deleted, such as adverbs like particulièrement [particularly, especially] or rare adjectives like amphibie [amphibious], or (b) replaced by shorter words, such as mousquetaires [musketeers] replaced by soldats [soldiers], au bout de [at the end of] by après [after]. Shorter words tend to be more frequent and are, therefore, more likely to be known by grade 2. Although the lexical substitutions were easy to perform for literary texts, they were more difficult to make for scientific texts, because specific vocabulary is crucial in the scientific domain. In most cases, we thus decided not to change specialized or technical terms, such as calcaire [calcareous] or atmosphère [atmosphere] unless this was possible (e.g., infiltrer [infiltrate] was replaced by passer [pass through]). Very few simplifications concerned discursive changes, such as when pronouns were changed by their referent to increase the explicitness of coreference chains, for example, “elle rencontra le loup (…) il [he] lui demanda” simplified by “elle rencontra le loup (…) le loup [the wolf] lui demanda”).
For the purpose of lexical simplification, we selected lexical substitutes (synonyms) from the Manulex database (Lété et al., Reference Lété, Sprenger-Charolles and Colé2004,) and from ReSyf (Billami et al., Reference Billami, François and Gala2018). The latter is an online lexicon that provides semantically disambiguated synonyms, which are ranked according to their readability. The readability ranking was obtained automatically by using statistical information (word frequencies and morpheme frequencies), lexical information (grapheme-to-phoneme distances, number of spelling neighbors, etc.), and semantics (polysemy) (François et al., Reference François, Billami, Gala and Bernhard2016). For syntactic simplification, we split sentences that contained subordinated or coordinated clauses. We also transformed long verbal phrases and modified passive to active mode. Simplified texts thus present sentences with only one inflected verb and apart from a few exceptions they follow the standard subject–verb–object structure (e.g., “Jamais elle ne rate son but” transformed into “Elle ne rate jamais son but”, where the adverb jamais [never] is moved after the verb). A detail of all the transformations can be found in the guidelines (Gala et al. Reference Gala, Todirascu, Javourey-Drevet, Bernhard and Wilkens2020b). An example of a normal and simplified text from the Alector corpus (Gala et al., Reference Gala, Tack, Javourey-Drevet, François and Ziegler2020a) is provided in Appendix A.
Text readability analysis of the material
To better understand and describe how our simplified versions differed from the original versions of the same text (e.g., Crossley, Louwerse, McCarthy, & McNamara, Reference Crossley, Louwerse, McCarthy and McNamara2007), we made use of some of the standard variables that are typically used in readability formulae (e.g., Flesch, Reference Flesch1948; Kandel & Moles, Reference Kandel and Moles1958). Within the field of readability, it is common to extract a large number of variables from texts (for review see François, Reference François2015). They belong to various feature families, within which all related variables are generally highly redundant (e.g., different kinds of frequency measures). In this research, we started out with a set of 406 variables gathered by François (Reference François2011). Note that a large number of the variables are variants of each other and thus measure similar text properties. Following the guidelines of Guyon and Elisseeff (Reference Guyon and Elisseeff2003), we chose the most important ones through a two-step process. That is, we first used domain knowledge to select the most relevant variables and we calculated their values for each of our simplified and original texts. We then used a Wilcoxon–Pratt signed-rank test to evaluate whether a variable significantly differentiated a simplified from an original text. We then checked the distributions of all significant variables and selected the most significant variables that had acceptable normal distributions. This procedure resulted in a selection of 27 variables (see Table 1) that can be organized into three families: 1) lexical, 2) morphological, and 3) syntactic.
Table 1. Selected readability variables for simplified and original versions of the texts. Standard deviations in parenthesis
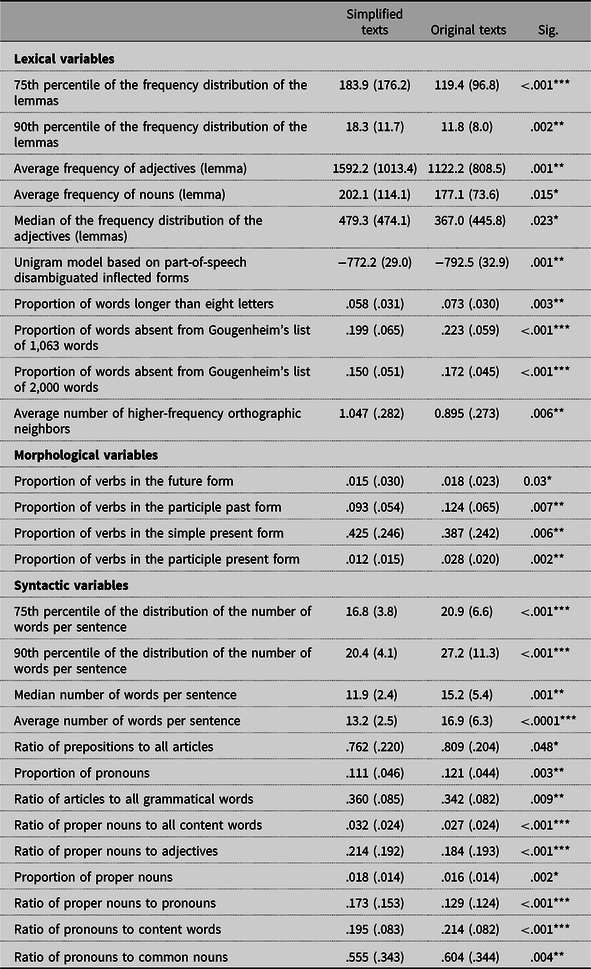
Lexical variables
The first set of 10 variables reflect lexical properties related to word frequency, word length, and orthographic neighborhood. Frequency variables were calculated using Lexique3 (New et al., Reference New, Brysbaert, Veronis and Pallier2007). We found significant differences for the average frequency of nouns and adjectives but also for the frequency of the 75th and 90th percentiles of the frequency distribution (all words). We found differences for a unigram model, which corresponds to the geometric mean of the log frequencies. Simplified texts also contained less words that were absent from a reference list of the most frequent French words (Gougenheim et al., Reference Gougenheim, Michéa, Rivenc and Sauvageot1964). In terms of word length, average word length failed to reach significance, but simplified texts had a significantly lower proportion of long words (we used > 8 letters but we had significant effects also for > 7 and > 9 letters). Finally, simplified texts had words with somewhat denser and especially more frequent orthographic neighborhoods (Huntsman & Lima, Reference Huntsman and Lima1996).
Morphological variables
We selected four significant variables that captured the inflectional complexity relative to verbal tenses and moods. Simplified texts had a significantly smaller proportion of verbs in the future form, verbs in the participle past form, and verbs in the participle present. In contrast, the proportion of verbs in the simple present form was increased in simplified texts.
Syntactic variables
In terms of syntactic difficulty, we selected a number of variables related to the number of words per sentence, such as the mean, the median, and the 75th or 90th percentiles. We also found that nine part-of-speech (POS) ratios differentiated simplified from original texts. POS ratios are often taken as a proxy for the syntactic complexity of sentences (Bormuth, Reference Bormuth1966).
Comprehension questions
After each text, participants received five multiple-choice comprehension questions. Internal reliability of this measure was high with a Cronbach’s alpha of 0.954. The percentage of correct answers was 84.2% for participants (quartiles: 75.3% and 90.0%; N = 149 participants) and 85.5% for questions (quartiles: 75.3% and 91.3%; N = 100 questions). Two types of questions were used: retrieval-type questions and questions that required participants to make inferences (e.g., Cain et al., Reference Cain, Oakhill, Barnes and Bryant2001). Because the texts were very short, there were more retrieval-type than inference-type questions (82% vs. 18%). The questions were identical for simplified and original texts.
Cognitive and language tests
Ten tests were used to assess the individual cognitive and language profile of each participant (see Table 2). They were administered in the same order for each participant.
Table 2. Descriptive statistics for the 11 cognitive and language tasks along with measures of skewness, kurtosis, and reliability (Cronbach’s alpha). Reliability for reading aloud and RAN is based on a split-half method

Phonological awareness
A computerized test (Evalec) was used to assess phonological awareness skills (Sprenger-Charolles et al., Reference Sprenger-Charolles, Colé, Béchennec and Kipffer-Piquard2005; Reference Sprenger-Charolles, Colé, Piquard-Kipffer and Leloup2010). We used the phoneme deletion subtest, in which children were presented with Consonant-Consonant-Vowel (CCV) spoken pseudowords and had to delete the first phoneme. They responded by pronouncing the remaining CV sequence. The test had 12 items.
Reading aloud
We used the French version of the Test of Word Reading Efficiency (TOWRE) adapted to French by Gentaz and colleagues (Reference Gentaz, Sprenger-Charolles and Theurel2015). This test contained a list of familiar words which had to be read aloud in 1 min (60 words, five per line) and a list of pseudowords (60 words, five per line). The words were among the 1000 most frequent words in grade 1 textbooks (Lété et al., Reference Lété, Sprenger-Charolles and Colé2004). The pseudowords were matched to the words in length, syllabic structure, and spelling. Twenty of the pseudowords had a grapheme whose pronunciation depended on the context (s = /s/ or /z/…). For the purpose of our analyses, we collapsed words and pseudowords into a single reading speed measure. To assess the reliability of the test, we calculated the correlation between words and pseudowords, which was .847.
Vocabulary
Vocabulary was assessed using the Test de Vocabulaire Actif/Passif (TVAP), a standardized vocabulary test for children aged between 5 and 8 years (Deltour & Hupkens, Reference Deltour and Hupkens1980). In this test, the student had to select one image out of six that corresponded to the word provided by the examiner. The test consisted of 30 words, 2 points per correct answer and 1 point for an approximate answer.
Oral comprehension
To assess the comprehension of syntactic and semantic information, we used a standardized French test called Epreuve de Compréhension Syntaxico-Sémantique Etalonnée (ECOSSE) developed by Lecocq (Reference Lecocq1996). In this test, a spoken sentence is given to the student, who must select the corresponding image among four possibilities, two of which contain lexical or grammatical traps. The test is designed to use a variety of syntactic structures, the complexity of which increases during the test. Because quite a few items were at ceiling, which produced a highly non-normal distribution, we eliminated all items with an accuracy rate above 95%. This left us with eight items (M11, M24, M42, N21, N44, P22, P33, and RNouv33) for which we obtained excellent psychometric properties (see Table 2). The test was computerized, such that each student was tested in the same conditions.
Working memory
We used the standard forward digit span from the Wechsler intelligence scale for children (Wechsler, Reference Wechsler2004). The experimenter pronounced a series of digits and children were asked to orally recall the series in the same order. The test started with two 2-digit series, followed by two 3-digit series, and so on up to two 9-digit series. Therefore, the maximum number of correct responses was 16. When children made two consecutive errors, the sequence was stopped.
Nonverbal intelligence
We used the Progressive Raven Matrices test (Raven Reference Raven1998), which consists of 36 items divided into 3 sets of 12 items (set A, set AB, and set B). Each item represents a geometric figure with a missing part and the participant had to choose one out of six pieces to complete the figure.
Morphology
We used a sentence completion task to measure morphological sensitivity (Casalis et al., Reference Casalis, Colé and Sopo2004). In this test, children had to complete a sentence using a derived form of a word whose root was provided in the first part of the sentence (e.g., “someone who hunts is a…”…“hunter”). We used the suffixed form which contains 12 items.
Visual word recognition
We used a standardized visual word recognition test, the Test d’identification de mots écrits (Timé 3) developed by Écalle (Reference Écalle2006). In this test, a total of 40 visually presented words must be identified in 2 tasks, a picture–word matching and a word association task. The score obtained is the total number of correct responses across both tasks.
Spelling
We used a standardized French spelling test, the Langage oral - Langage écrit - Mémoire, Attention test (L2MA, 2nd edition; Chevrie-Muller et al., Reference Chevrie-Muller, Maillart, Simon and Fournier2010). In this test, seven sentences were dictated to the student and accuracy was coded on two dimensions, morphology (45 points) and grammar (31 points). Spelling errors that did not fall into these dimensions were not taken into account.
Rapid automatized naming
The children were presented with four lines of nine digits on a computer screen (same order for all participants). They had to name them as quickly as possible. We measured the total time to name the 36 digits, which was converted into a speed measure (digits per second). Split-half reliability was .472.
Results
As concerns the digital text reading, we calculated the RT by dividing the on-screen display duration of each sentence by the number of words of each sentence (resulting in a time per word) and by the number of characters of each sentence (resulting in a time per character, used to remove aberrant values). As shown in Appendix B, the two RT distributions exhibited a bimodal distribution, reflecting the fact some responses were abnormally short probably due to rapid skipping of a sentence (lack of interest and inability to read the text) or involuntary anticipatory responses. We therefore applied a rigorous data trimming procedure to exclude such artifactual data points. We also removed participants and texts that did not have a sufficient number of valid data points. The procedure is fully described in Appendix B. It is important to note that such a rigorous trimming procedure was necessary because the reading data came from self-paced reading in fairly young children in real classroom conditions. Thus, children could skip several lines or an entire text if they did not feel like reading and there was no external monitoring that would have prevented them from doing so. As shown in Appendix B, the final analysis included data from 2,600 texts (out of 3,300 initially) and 149 participants (out of 165 initially).
RTs were log-transformed to normalize RT distributions (see Appendix B). RTs and accuracies were analyzed using mixed effect regression modeling using the lme4 package (Bates et al., Reference Bates, Mächler, Bolker and Walker2014) and the lmerTest package (Kuznetsova, Brockhoff, & Christensen, Reference Kuznetsova, Brockhoff and Christensen2017) in the R environment (RCoreTeam, 2019). Participants and items (i.e., sentences or questions) were used as random variables. The models are specified at the beginning of each of the following sections. Data, R scripts, model specifications, and summaries are available on an open science repository Footnote 5 . Additional R libraries were used for aggregating and transforming data (Wickham et al., Reference Wickham, Averick, Bryan, Chang, McGowan, François, Grolemund, Hayes, Henry, Hester, Kuhn, Pedersen, Miller, Bache, Müller, Ooms, Robinson, Seidel, Spinu and Yutani2019), plotting model results (sjPlot; Lüdecke, Reference Lüdecke2021), estimating model means (emmeans; Lenth, Reference Lenth2021), and computing principal component analyses (PCAs) (psych; Revelle, Reference Revelle2021).
Effects of simplification on reading speed and comprehension
The effects of simplification on RTs (milliseconds per word) are presented in Figure 1 for both literary and scientific texts. These effects were analyzed using a linear mixed effect model with Simplification (original vs. simplified) and Type of text (literary vs. scientific) as fixed factors and child and sentence as random factors [formula: lmer(RT ∼ Simplification x TypeOfText + (1|Sentence) + (1 + Simplification x TypeOfText |Child)]. The analysis revealed a significant effect of Simplification (b = −0.03, SD = 0.009, t = −3.5) and Type of text (b = 0.02, SD = 0.008, t = 2.73) on RTs, with no significant interaction between these two factors (t = 1.16). These effects reflect the fact that simplified texts were read faster than original texts (estimated marginal effect size of 44 ms per word) and that scientific texts were read slower than literary texts (estimated marginal effect size of 57 ms). Despite a small tendency for smaller simplification gains in scientific than literary texts (cf. Figure 1), the critical interaction failed to reach significance.

Figure 1. Reading times (in milliseconds, ms) per word for original and simplified versions of literary and scientific texts. Error bars are standard errors.
The results for comprehension (MCQ) are presented in Figure 2A. We had both retrieval-type and inference-type questions, and the results of simplification on the type of question are presented in Figure 2B. The results were analyzed using two logistic mixed effect model with Simplification (original vs. simplified) and Type of text (literary vs. scientific) for the first model, and Simplification and Type of question (retrieval vs. inference) for the second model as fixed factors [model 1 formula: glmer(Accuracy ∼ Simplification × TypeOfText + (1|Question) + (1+ Simplification + TypeOfText|Child); model 2 formula: glmer(Accuracy ∼ Simplification × TypeOfQuestion + (1|Question) + (1+ Simplification + TypeOfQuestion|Child)]. For the first model, we obtained a significant effect of Simplification (b = 0.55, SD = 0.09, z = 6.23), which reflected the fact that comprehension was better for simplified than for original texts (estimated marginal effect size of 3.97%). Type of text did not reach significance, but the interaction between Type of text and Simplification was significant (b = −0.36, SD = 0.11, z = −3.28), which reflects the fact that simplification yielded stronger effects for literary than scientific texts. Yet, the contrast analysis showed that simplification was still significant for both type of texts (z = −6.23 and z = −2.33 for literary and scientific text, respectively). Concerning the second model, the effect of simplification was significant (b = 0.36, SD = 0.13, z = 2.86) and produced a marginal effect of 4.11 % in favor of simplified texts. The analyses did not reveal an effect of Type of question neither as a simple effect nor as an interaction. This suggests that simplification worked equally well for both retrieval- and inference-type questions.

Figure 2. Results from the comprehension test. A. Proportion of correct responses (in percent) for simplified and original literacy and scientific texts. B. Proportion of correct responses for the simplified and original texts as a function of the type of question (retrieval-type vs. inference-type). Error bars are standard errors.
Effects of cognitive and language variables on simplification gains
We were interested in finding out whether simplification was more or less efficient as a function of the cognitive and language profile of the child. For instance, did poor readers or children with poor oral language skills benefit more from simplification than good readers or children with good oral language skills? To address this question, we investigated whether simplification gains in reading speed and comprehension would vary as a function of the performance of the children on our 11 language and cognitive variables. Thus, the idea was to add each cognitive and language variable to the mixed effect model and see whether it had an effect on reading speed and comprehension and whether it interacted with simplification. To reduce the number of multiple comparisons, we first conducted a PCA on the 11 variables. The PCA resulted in a four-component solution and Table 3 shows the loadings of the variables on the four components after a varimax rotation. The first component (C1) reflected reading ability, the second component (C2) oral language and vocabulary skills, the third component (C3) spelling ability, and the fourth component (C4) nonverbal intelligence and working memory.
Table 3. Loadings of cognitive and language variables on principal components (C1 to C4) computed from a varimax-rotated principal component analysis. Figures in bold are greater than 0.5
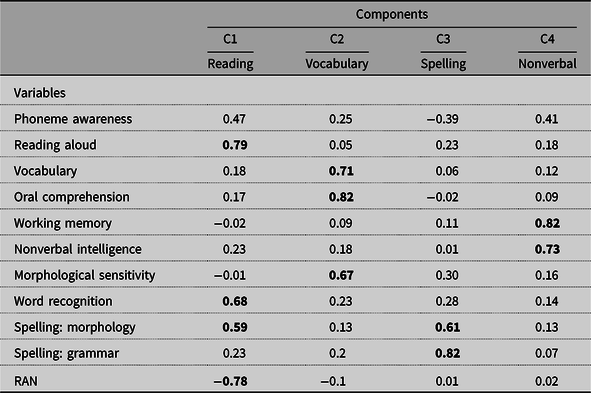
RAN, Rapid automatized naming.
We then introduced the factor scores on these four components and their interaction with simplification in the linear mixed effect models that predicted reading speed or comprehension scores. A significant effect of a component would mean that the component affects reading speed or comprehension. A significant interaction of the component with simplification would mean that the component modulates the effects of simplification. The R formula for reading speed was the following: lmer(RT ∼ Simplification + Simplification × C1 + Simplification × C2 + Simplification x C3 + Simplification x C4 + (1|Sentence) + (1|Child). The results are presented in Table 4 (upper part).
Table 4. Effects of the four principal components reflecting the children’s cognitive and language profiles on reading speed and comprehension (simple effect) and their interaction with the effects of simplification (interaction). Coefficients result from mixed effect models described in the text

Note.* p < .05; ** p < .01; *** p < .001.
As can be seen in Table 4, apart from C2 (oral language and vocabulary), all other components were associated with reading speed. Importantly, there was a significant interaction between the effects of simplification and a participant’s reading competence (C1). This interaction is plotted in Figure 3 using the R libraries Emmeans and sjPlots (Lenth, Reference Lenth2021; Lüdecke, Reference Lüdecke2021). As can be seen, simplification gains (difference between simplified and original texts) were larger for poor readers (Normalized Units < 0) than for good readers (Normalized Units > 0).

Figure 3. Modulation of reading speed (milliseconds per word, log10, y-axis) as a function of reading ability (PCA1, normalized units, x-axis). Solid and dashed lines are the estimated reading times for original and simplified texts at different levels of reading ability. Semitransparent areas are confidence intervals.
The same analysis was performed for comprehension scores. However, to take into account the fact that in the main analysis presented above, the effects of simplification on comprehension were different for scientific versus literacy texts (i.e., we obtained a significant Simplification x TypeOfText interaction), we conducted two separate analyses, one for literary and one for scientific texts. The R formula for the analyses of the comprehension scores was glmer(Accuracy ∼ Simplification + Simplification × C1 + Simplification × C2 + Simplification × C3 + Simplification × C4 + (1|Question) + (1|Child)). The results are presented in Table 4. We found that all components were associated with comprehension. For scientific texts only, C4 (nonverbal intelligence and working memory) showed a significant interaction with comprehension reflecting the fact that simplification gains were stronger for children with poor nonverbal intelligence and weak working memory. C1 showed a marginally significant interaction (p = .051). Both interactions are plotted in Figure 4.
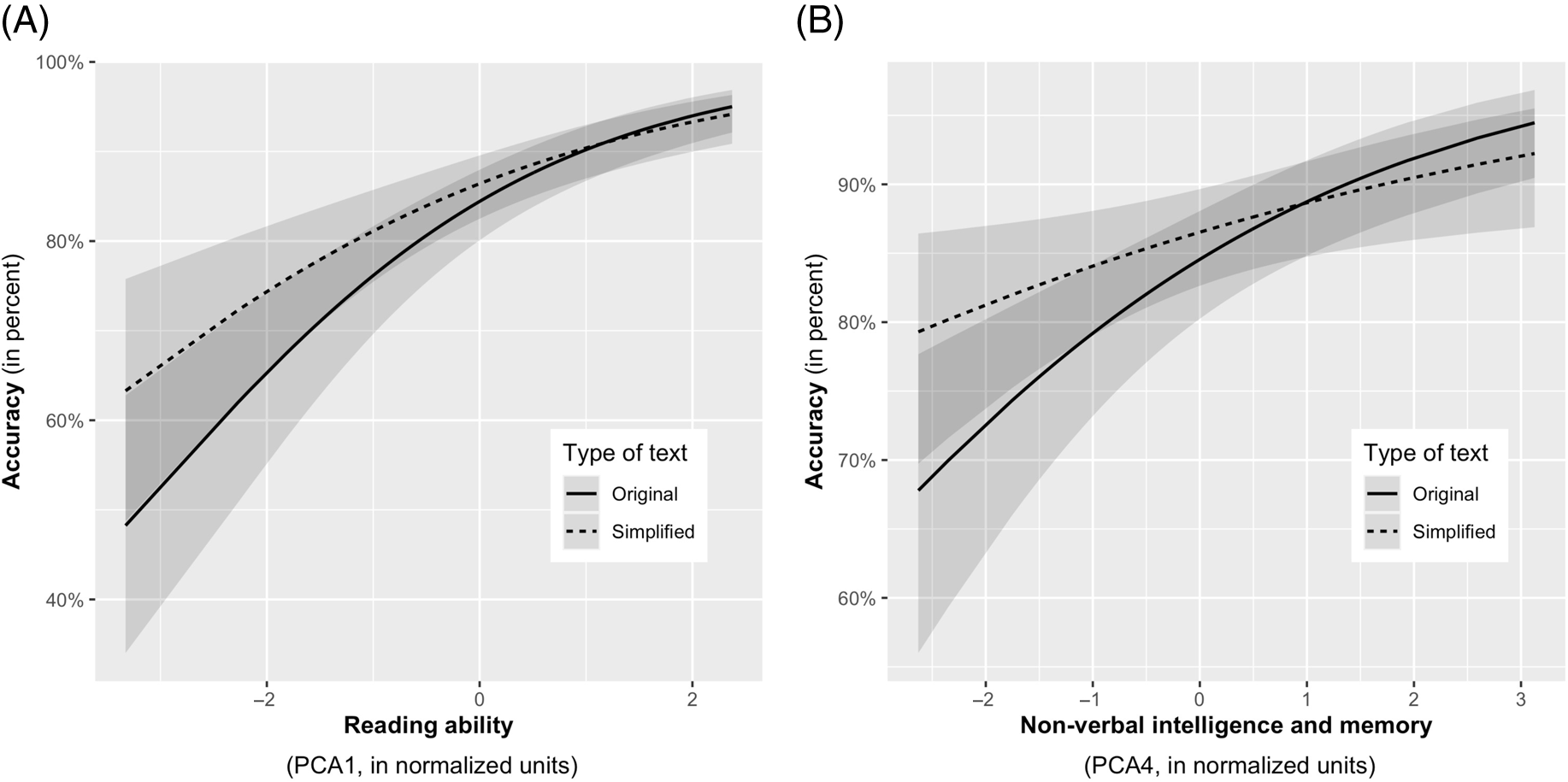
Figure 4. Text comprehension accuracy (percent correct, Y-axis) as a function of reading ability (A) and nonverbal intelligence (B). Solid and dashed curves are the estimated accuracies at different levels of the component variable (in standardized units, X-axis). Semi-transparent areas are confidence intervals.
As can be seen in Figure 4, simplification gains for comprehension (i.e., the difference between simplified and original texts) were greater for participants with weaker reading performance (Normalized Units < 0, Panel A) and weaker nonverbal intelligence (Normalized Units < 0, Panel B). This was true for scientific texts only. The logarithmic curvature of the slopes suggests that comprehension benefits were amplified for children with very poor reading or nonverbal intelligence.
Discussion
The general aim of our study was to investigate whether text simplification could improve reading fluency and comprehension in primary school. Previous studies have mainly looked at the effects of text simplification in special-needs populations, such as illiterates or readers with dyslexia (Gala & Ziegler, Reference Gala and Ziegler2016; Margarido et al., Reference Margarido, Pardo, Antonio, Fuentes, Aires, Aluísio and Fortes2008; Rello et al., Reference Rello, Baeza-Yates, Dempere-Marco and Saggion2013). In the present study, we investigated whether text simplification could be beneficial for normally developing beginning readers in a regular classroom context. Indeed, recent national evaluations suggest that 40% of 15-year-old pupils are not able to identify the theme or the main subject of a text, do not comprehend implicit information, and are unable to link two separate explicit information in a text (CEDRE, 2015). In addition, it is well known that children from socially disadvantaged backgrounds tend to show greater reading comprehension problems (Fluss et al., Reference Fluss, Ziegler, Warszawski, Ducot, Richard and Billard2009). Thus, finding ways to improve reading fluency and comprehension for children who struggle with learning to read is of outmost importance.
Our results showed that text simplification clearly improved RTs. Note that RTs were calculated per word, which controls for the fact that simplified texts tended to contain fewer words. With respect to comprehension, we found similar text simplification benefits, that is, better comprehension for simplified than original texts. Importantly, children answered the exact same comprehension questions for simplified and normal texts, which makes this a well-controlled comparison. It is interesting to note that our text simplification did not result in a speed–comprehension trade-off that is typically found in studies that focus on improving reading speed (for a detailed discussion, see Rayner et al., Reference Rayner, Schotter, Masson, Potter and Treiman2016). Instead, we clearly show that text simplification improves both comprehension and reading speed in beginning readers.
Do some readers benefit more than others from simplification?
Concerning the question as to whether some readers would benefit more than others from simplification, we obtained two interesting interactions between the cognitive and language profiles of our children and the effects of simplification on fluency and comprehension (see Table 4). As illustrated in Figures 3 and 4 for fluency and comprehension, respectively, children with poor reading skills (first PCA component) showed the greatest simplification benefits in reading fluency and to a smaller extent in comprehension (marginally significant effect). This result confirms our prediction that text simplification should be particularly beneficial for poor readers. This was expected because we changed infrequent and long words with more frequent and shorter words, which should have been easier to read for children with poor reading skills. As noted previously, beginning readers rely to a great extent on decoding (Ziegler, Perry & Zorzi, Reference Ziegler, Perry and Zorzi2020), but the impact of decoding on reading comprehension seems to decrease as children become more proficient readers (e.g., Verhoeven & Van Leeuwe, Reference Verhoeven and Van Leeuwe2008). Thus, it is an open question to what extent simplification is useful for more proficient readers.
In contrast to our predictions, however, we did not find significant interaction between the effect of simplification and vocabulary/oral language skills (second PCA component), although this component was clearly related to overall reading speed and comprehension (see the effects in Table 4). While it is important not to overinterpret null effects, the absence of an interaction between simplification and the oral language component seems to suggest that simplification was beneficial for beginning readers regardless of whether they had good or poor vocabulary or oral comprehension. We should note, however, that the vocabulary and oral language tests were quite short and might not have been sufficiently sensitive to fully assess the oral language skills of our sample.
We also found a significant interaction between the effects of simplification and nonverbal intelligence/memory (fourth component) on comprehension. The effect of nonverbal intelligence/memory on comprehension was positive: children with higher nonverbal intelligence and better memory were better comprehenders (Colé et al., Reference Colé, Duncan and Blaye2014). Yet, the interaction with the effects of simplification was negative, which suggests that children with weaker nonverbal intelligence and/or weaker memory skills benefit to a greater extent from simplification.
However, it should be acknowledged that potential ceiling effects in comprehension could undermine the conclusion that simplification does not benefit good readers as much as poor readers. More skilled readers may already be too fluent and comprehend the passages too well for simplification to improve their scores. When performance is over 90% on the original texts for “good to very good” readers (see Figure 4, zreading ability > 1), there might be little room left for simplification to improve things.
Taken together, with this caveat in mind, the overall results seem to suggest that poor readers and children with weaker cognitive abilities seem to benefit to a greater extent from text simplification. This supports the hypothesis that the major effect of simplification is that it renders word recognition and decoding more efficient, which has direct effects on reading fluency and text comprehension as predicted by the simple model of reading (Gough & Tunmer, Reference Gough and Tunmer1986; Hoover & Gough, Reference Hoover and Gough1990).
Does simplification work better on literary than on scientific texts?
With respect to comprehension, our results showed that simplification yielded stronger effects for literary than for scientific texts. A similar trend was observed for reading speed, although the interaction failed to reach significance. This might be somewhat surprising because scientific texts are typically longer, contain more infrequent words, and are conceptually more complex (Hiebert & Cervetti, Reference Hiebert and Cervetti2012). Thus, they should have benefitted to a greater extent from simplification than literary texts. However, it was much more difficult to simplify scientific texts because they tended to use a very specific low-frequency vocabulary, for which it was not always possible to find more frequent synonyms. For example, in our sentence, Algae produce more than half of the oxygen in our air, neither algae nor oxygen can be replaced by more frequent synonyms. In addition, we selected informational texts that were used in the school curriculum. They were written in a rather simplified journalistic style using somewhat shorter sentences (14.2 vs. 16.1 words per sentence, for scientific vs. narrative texts, respectively).
The overall difficulty with scientific texts highlights the importance of specifically using such reading material in classroom interventions. Indeed, Kim et al. (Reference Kim, Burkhauser, Mesite, Asher, Relyea, Fitzgerald and Elmore2021) showed that having young children read science documentaries with content literacy instruction can help them develop concept-related vocabulary while fostering domain knowledge in science and increasing general reading interest.
Limitations and future directions
There are a few limitations of our study. First, given that we simplified at three linguistic levels (lexical, morphosyntactic, and discursive) and although lexical simplifications were by far more frequent than the two other types, it is currently not possible to precisely dissociate the effects at each level. Future work is needed to pull apart the effects at each level. Second, all simplifications were made manually. Although we used rigorous rules and computational tools to help us make the simplifications, such as an online lexicon that provides semantically disambiguated synonyms ranked for their readability (ReSyf, Billami et al., Reference Billami, François and Gala2018), we fully acknowledge a subjective part of making some of the simplifications. We are currently working on a fully automatic simplification system for French Footnote 6 , which should solve this limitation in the future (see Saggion, Reference Saggion2017). Third, we did not manage to use the same number of inference- and retrieval-type questions, which was partially due to the fact that the texts were short making it difficult to come up with inference-type questions.
There are two general limitations of text simplification that are worth discussing. This first is that changing words in a text might cause a loss of a text’s authenticity. Indeed, authors do not choose words at random but they use words that best fit their communicative purpose or serve a poetic function. Researchers in text simplification are well aware of this problem, which is why the focus is on “retaining the original information and meaning” (Siddharthan, Reference Siddharthan2014, p. 259) rather than preserving the original style. As a matter of fact, synonyms rarely carry the exact same meaning and some semantic nuances might be lost when replacing complex words by simpler words. The second limitation is that simplified texts are not always available in every situation in the reading journey of a poor comprehender. Thus, even if text simplification is beneficial for poor readers, it remains of outmost importance to develop general intervention programs that bring poor comprehenders to the level of typically developing readers (see Bianco et al., Reference Bianco, Bressoux, Doyen, Lambert, Lima, Pellenq and Zorman2010, Reference Bianco, Pellenq, Lambert, Bressoux, Lima and Doyen2012).
In terms of future directions, it would be important to test whether benefits of simplification can be obtained beyond grade 2. We are currently undertaking a longitudinal study to address this issue. Furthermore, it would be important to find out whether the effects of simplification have a long-lasting general effect on improving reading skills. That is, if we were to give reading-level appropriate simplified material to poor readers for some period of time, would we be able to show that they improved general reading comprehension skills compared to a group of students who did not receive reading-level adapted simplified material? Finally, given that our iBook application measures reading speed and comprehension for each text online, one can easily imagine that the system automatically chooses the “right” level of difficulty. If reading is slow and comprehension is poor, the child would receive easier or simplified texts, if reading is fast and comprehension is good, readers would receive more difficult texts that are not simplified. Thus, such a system would be able to track students’ performance and propose adapted texts as a function of the student’s reading and comprehension level. With the rise of artificial intelligence and deep-learning tools in education, such a system could use the online player data to optimize the selection and simplification of texts with respect to the needs of the students.
Acknowledgments
Our friend and colleague Jacques Ginestié passed away in September 2020; this article is dedicated to his memory. This work has been supported by the pilot center on teacher training and research for education (AMPIRIC), funded by the Future Investment Programme (PIA3), and the center of excellence on Language, Communication and Brain (ILCB), funded by the French National Agency for Research (ANR, ANR-16-CONV-0002) and the Excellence Initiative of Aix-Marseille University A*MIDEX (ANR-11-IDEX-0001-02). The research was directly funded through grants from the ANR, ALECTOR (ANR-16-CE28-0005) and MORPHEME (ANR-15-FRAL-0003-01) and the ERC (Advanced grant 742141). The authors thank all the participants, the teachers who accepted to use the iBooks in their classrooms, and the school authorities who supported this study, especially Olivier Millangue, Nathalie Greppo-Chaignon, and Géraldine Gaudino. We also thank the Communauté d’Agglomération Sud Sainte Baume for providing access to the iPads used in the schools and technical support. We finally thank Marco Bressan for his support regarding the statistical analyses, the research assistants for their help in the data collection of the cognitive tasks, and the children of the co-authors who helped improve the iBook interface.
Example of a normal text and its corresponding simplified version taken from the Alector corpus (Gala et al., Reference Gala, Tack, Javourey-Drevet, François and Ziegler2020a). The Alector corpus is an open-access data database (see https://corpusalector.huma-num.fr/) that allows one to choose a text as a function of different criteria (length, age, type of text, and text difficulty). The texts are available in normal and simplified versions and the comprehension questionnaires are identical for both versions. The whole set is downloadable in pdf.

Appendix B Data preprocessing
Removal of atypical reading times . Raw RTs (sentence reading times; ms) were first transformed to reading times per word (RTW) and reading times per character (RTC) by dividing raw RTs with the associated number of words and number of characters of each sentence. Out of the initial 48,180 RTs (Original and Simplified), we identified those that did not fall into a central tendency, namely RTs that showed were too short. Figure A1 displays the histograms for both the RTWs and RTCs. One can see that the distributions show two tendencies, one count peak on the left side of the histograms and one ex-Gaussian-type pattern on the right side. This dual shape is symptomatic of a dual process, the ex-Gaussian-type RTW values being associated with a regular reading behavior while fast RTWs (typically < 150 ms) corresponding to a fast click on the “Next Sentence” button. In our self-paced reading task, this corresponds to a sentence skipping behavior. To eliminate these responses, which caused non-normal distributions, we applied a minimal cutoff of 150 ms per word (RTW) and 40 ms per character (RTC). To eliminate exceptionally long responses, we also applied a maximum cutoff of 5000 ms per word (RTW) and 1000 ms per character (RTC). This procedure removed 7,212 RTs (14.97% of the initial dataset).

Figure A1. Histograms of response times per word (raw RT divided by the number of words per sentence; left panel) and response times per character (raw RT divided by the number of characters in the sentence; right panel). RTs superior to 2000 ms per word and 700 ms per character are not plotted.
Removal of outliers . Regular outliers within the ex-Gaussian central tendency were then removed using the interquartile rule: RTWs smaller than Q1 - 1.5*(Q3 - Q1) and greater than Q3 + 1.5 * (Q3 - Q1) were discarded, Q1 being the first quartile and Q3 the third quartile of the RTW distribution. The interquartile rule removed 2,650 RTWs (5.50% of the initial number of RTWs).
Text selection . To ensure that we analyzed data only for texts that were properly read, for each child and each text, we only kept texts that had at least 75% of valid RTWs. This procedure also ensured that questions related to the texts were not randomly answered. Out of an initial number of 3330 texts (20 texts read by 165 participants), this procedure resulted in the selection of 2600 texts for further analysis.
Participant selection . To be included in the statistical analysis, participants had to have at least one valid text per design cell (Original, Simplified) x (Literary, Documentary). This led to the inclusion of 149 participants (out of 165 initially; 9.7% excluded).
RTW were then log10 transformed. Figure A2 shows the corresponding histogram.

Figure A2. Histogram of the final log10 response time distribution RTs per word (raw RT divided by the number of words of each sentence) after having applied the outlier exclusion and trimming procedure described above.