Introduction
In this article we propose a new theory of human cognitive evolution, which we argue lies at the core of explaining the exceptional adaptiveness of our species. In particular, we propose that members of our species are individually specialized in different but complementary neurocognitive search strategies and that consequently we regulate search for adaptive information at the group level, adapting cooperatively. The theory is grounded in Complex Systems theory (e.g. Mitchell Reference Mitchell2011) and the framework of Search (e.g. (Hills et al. Reference Hills, Todd, Lazer, Redish and Couzin2015). We call this emergent system of collective cognitive search Complementary Cognition.
Cross-cultural patterns that occur in human cognition suggest specialization in cognitive search, with large portions of the population having noticeable cognitive search biases. Looking at these complementary patterns of specialization comprehensively, we begin to see that they belong to a greater complex adaptive system (Taylor & Lockett Reference Taylor and Lockettforthcoming).
To reveal the significance of Complementary Cognition, we begin by defining search and show that Complementary Cognition can be contextualized as part of a hierarchy of systems of search, at the level of the genome; cognition; and collective cognition. These systems of search are significant: results of adaptive search can be inherited and so evolve over time.
Neurocognitive specialization in search predicts that our species evolved in a highly variable environment, since uncertainty is a key driver in the selection of search capability, and variability makes search optimization at the individual level difficult. We outline how such conditions prevailed during the evolutionary history of our ancestors (Potts Reference Potts1998) and must have created strong selection pressures for efficiency and capability in search.
We propose that such selection pressures, coupled with fundamental constraints in individual cognition, induced division and specialization in neurocognitive capabilities; that is, selection pressures acting at the individual level resulted in the evolution of individual hominins with different but complementary neurocognitive search strategies, resulting in the emergence of a new system of search at the collective level.
Specialization in search would only be possible in the context of appropriate means of collaboration. We propose that Complementary Cognition co-evolved in a positively reinforcing feedback loop with aspects of communication and cooperation. This has implications for an evolutionary theory of language, providing two key reasons for its evolution: first, as a mechanism to facilitate collaborative search between cognitively specialized individuals; secondly, as a new inheritance channel to share the results of cognitive search. Particular features of human language conform with this theory. For example, the integration of information from different search strategies creates information of unbounded complexity, and so communicating the results of complementary cognitive search requires an open system, a key feature of human language.
We suggest that the evolution of Complementary Cognition can be characterized as a Major Transition (Maynard Smith & Szathmáry Reference Maynard Smith and Szathmáry1995) and represents a significant transition in evolvability, enabling substantially greater capacity, speed and flexibility to adapt than adaptation at evolutionary or cognitive scales.
We propose that the emergence of Complementary Cognition plays a central role in explaining our species’ remarkable adaptiveness. Humans have come to thrive in nearly every terrestrial environment on the planet. From tropical rainforests, savannah to tundra, our phenotypic adaptations are almost identical. Adaptation to such a range of habitats has primarily been achieved through extra-somatic or cultural adaptations—including behavioural and technological adaptations (Binford Reference Binford1962; Richerson & Boyd Reference Richerson and Boyd2005). The evolution of these cultural adaptations is open-ended and cumulative. We propose that the reason for this adaptive capability is Complementary Cognition and that it lies at the heart of explaining our cumulative cultural evolution.
Definition of Complementary Cognition
Complementary Cognition is the theory that our species cooperatively adapts and evolves through a system of collective cognitive search.
It is proposed that Complementary Cognition emerged as a consequence of individual neurocognitive specialization in search and co-evolution with language and aspects of cooperation.
Cooperative search between specialized individuals enables the co-creation of adaptations of higher fitness value. It does not imply that individuals carry out exploratory or exploitative activities exclusively, but rather are specialized so that they differ with regard to the neurocognitive capabilities that support search, and how information search is balanced. Complementary Cognition can be contextualized as part of a hierarchy of systems through which our species adapts and evolves which includes genetic evolution.
Complementary Cognition evolved due to high environmental variability during our species’ evolution, which created strong selection pressures for cognitive search capacity and efficiency.
Complementary Cognition contributes to our understanding of behavioural modernity and the emergence of cumulative cultural evolution.
Search, adaptation and evolution
Although many systems of adaptation exist within an organism (McGlade & Allen Reference McGlade and Allen1986), the adaptive systems of genetic search and cognitive search differ in that the results can be stored, inherited and updated through further search. This leads to evolution of adaptations over time. Complementary cognitive search also has this property.Footnote 1
What do we mean by search?
Living systems need to acquire and update adaptive information: information that is of most adaptive value will vary over time and space, for example as predators or prey move, or as resources are depleted or change with seasons and environmental variability, creating uncertainty that necessitates search (Hills et al. Reference Hills, Todd, Lazer, Redish and Couzin2015). Search in some form is thus fundamental to adaptation across species.
The optimal search strategy will involve a mixture of exploration and exploitation. This can be viewed as a continuum. At extremes, all resources are fully allocated to either exploration or exploitation of existing information. Consider a simple search for food in physical space. An organism can exploit the known area where it stands, exploiting a known patch of resources, or it could search more globally, exploring unknown areas for new patches of resources, or mix these behaviours.
Search is not restricted to physical domains. Abstract search can involve information domains, such as the social domain or abstract design ideas, or searching memories for a relevant piece of information. Abstract searches occur over information landscapes instead of physical ones.
Navigating this ubiquitous ‘exploration-exploitation’ trade-off is a search optimization problem (Črepinšek et al. Reference Črepinšek, Liu and Mernik2013). Organisms continuously face the dilemma of whether to pursue actions that exploit existing but possibly suboptimal information, or explore uncertain but potentially more profitable solutions. While exploration may lead to better resources, exploring to the exclusion of exploitation might result in too many undeveloped new ideas and a lack of refined skills and expertise. By contrast, focusing too much on exploitation risks being trapped in a local optimum or failure to adapt to environmental change (March Reference March1991).
The most adaptive strategy depends on context and factors such as environmental familiarity, resource richness and level of variability. The relative value of exploiting known resources versus the cost of reducing uncertainty through exploration will also guide the appropriate strategy. The optimal strategy may vary in time and space, as well as across domains of search relevant to survival. Finding a generally optimal solution to this optimization problem is extremely challenging, and probably impossible (Cohen et al. Reference Cohen, McClure and Yu2007).
Regulating the balance between exploration and exploitation in search is fundamental to adaptive success (Cohen et al. Reference Cohen, McClure and Yu2007). This trade-off arises in many fields, often under a different name. In organizational research, exploration encompasses things such as search, variation, risk-taking, flexibility, experimentation, discovery and innovation. Exploitation includes aspects such as refinement, choice, production, efficiency, selection and implementation (March Reference March1991). In foraging, the contrast is between extensive versus intensive search (Benhamou Reference Benhamou2007); in artificial intelligence, breadth versus depth-first search (Korf Reference Korf1985); in time, long- versus short-term; in visual attention, diffuse versus focused (Rivière et al. Reference Rivière, Cordonnier and Fouasse2017); in memory, global versus local (Todd et al. Reference Todd, Hills, Robbins, Todd, Hills and Robbins2012). This disparity in terminology has made it harder for specialists in different areas to recognize these varied facets as part of the same underlying pattern.
Genetic search
Darwin's theory of evolution through natural selection can be interpreted as a search process (e.g. see Watson & Szathmáry Reference Watson and Szathmáry2016) by which successful adaptations are inherited and updated over time. Every organism's genotype can be regarded as a balance between inherited information that existed in the previous generation and variation arising for example from mutation or recombination.
Inheritance enables the results of previously successful searches to be exploited. Further search or variation allows the possibility of increased adaptation or adaptation to a changing environment. The degree to which search is explorative may depend upon the processes that produced the variation. For example, asexual reproduction may be less explorative than sexual reproduction as the variation stems only from mutation and not recombination as well (Page Reference Page2011). Sexual reproduction appears to be very effective at balancing the trade-off between exploitation of alleles that were fit on average in the past, and sampling alleles in new combinations (Chastain et al. Reference Chastain, Livnat, Papadimitriou and Vazirani2014; see Watson & Szathmáry Reference Watson and Szathmáry2016).
The resulting combination of inherited and new information will play a major role in determining an organism's fitness. Natural selection in effect searches for organisms that are adapted to their environment, each the result of a balance between exploitation and exploration. Thus genetic search drives phenotypic evolution.
Variability, uncertainty and the evolution of cognitive search
Genetic search has obvious limitations. It does not enable an organism to adapt to environmental changes during its lifetime. If changes occur faster than can be adapted for genetically, adaptation must occur through other mechanisms for the organism to survive and reproduce. Many species have evolved the capacity for cognitive search, enabling behavioural adaptation during an organism's lifespan. In effect, the rate of change in the environment relative to the frequency of genetic evolution contributes to the need for other means of adapting to change. The notion that cognitive search is selected for by high rates of variability is supported by models in the field of cultural evolution which show that capacities for learning are selected for over hard-wired behaviours in the context of environmental variability (see Henrich & McElreath Reference Henrich and McElreath2003).
Cognitive search
Cognition is not generally viewed from the perspective of search in the field of cultural evolution. Emphasis has been on how cultural adaptations are inherited and maintained between generations, with importance placed on mechanisms such as social learning (Henrich Reference Henrich2017; Laland Reference Laland2017; Richerson & Boyd Reference Richerson and Boyd2005) as well as other aspects which support high fidelity and bandwidth of inheritance such as niche construction (Sterelny Reference Sterelny2011). In other words, how past adaptive knowledge is exploited.
Notable exceptions include Jablonka and Lamb (Reference Jablonka and Lamb2005), who argue that focusing on replication and selection rather than on generation of variants and reconstruction is counterproductive to understanding cultural evolution. Using the framework of cognitive search to understand how behavioural adaptations are inherited, and adaptive knowledge updated, helps to address these concerns.
Hills et al. (Reference Hills, Todd, Lazer, Redish and Couzin2015) propose that cognition can be envisioned as a search process, characterized by the exploration–exploitation trade-off. Search can be used as a common framework for understanding cognitive behaviour and the function of cognitive control across domains (Hills et al. Reference Hills, Todd, Lazer, Redish and Couzin2015). Regulating search is so central to adaptive success that optimally balancing exploration and exploitation is believed to be one of the most important selective forces operating in the evolution of cognition (Cohen et al. Reference Cohen, McClure and Yu2007; Hills et al. Reference Hills, Todd, Lazer, Redish and Couzin2015).
Search for food, water, mates or any other resource is not a straightforward matter of simply searching in physical space for a particular goal: it is a complex and multi-layered process. One way of breaking this down is to consider search at the perceptual level, search at the level of causal structure, the level of goal selection and the level of action selection (Winstanley et al. Reference Winstanley, Robbins, Balleine, Todd, Hills and Robbins2012, 128). In combination, these different aspects of search enable an organism to construct models of the world so that it can more successfully navigate its environment and locate resources necessary for survival and reproduction.
Search at any level, will involve navigating the trade-off between exploration and exploitation. With regard to external search, an individual's sensory organs can usually only capture a small proportion of that information which is relevant to adaptation, and even this exceeds the brain's rate of information processing (Hills & Dukas Reference Hills, Dukas, Todd, Hills and Robbins2012). Thus limited attentional resources must be allocated to that portion of relevant information that has the greatest effect on fitness (Dukas & Ellner Reference Dukas and Ellner1993; Hills & Dukas Reference Hills, Dukas, Todd, Hills and Robbins2012) in a similar way to how resources must be allocated during search in physical space.
Beyond its external perceptual landscape, an organism may also search an internal information space to retrieve relevant information from memory. Internal search involves navigating the exploration-exploitation trade-off similarly to search in physical or external space (Hills & Dukas Reference Hills, Dukas, Todd, Hills and Robbins2012). It is thought that the same molecular machinery that first evolved for goal-directed search in physical space was gradually co-opted for regulating goal-directed cognition (Hills Reference Hills2006). So to complement physical search for tangible resources, organisms evolved cognitive abilities to search for information related to those resources in internal and external information spaces (Hills Reference Hills2006).
Minimizing uncertainty is one of the key computational principles that drives the search process. Such uncertainty may relate to all aspects of an organism's world, from how relevant features are represented to the nature of its interaction with the world. The degree of uncertainty plays a key role in both the initiation and termination of cognitive search (Winstanley et al. Reference Winstanley, Robbins, Balleine, Todd, Hills and Robbins2012). An organism familiar with an environment can use its knowledge and internal models to make predictions about the consequences of cues, events and actions. If observations differ from expectations to a degree that is not expected, i.e. unexpected uncertainty, this is likely to motivate search to update the understanding of the environment's properties (Winstanley et al. Reference Winstanley, Robbins, Balleine, Todd, Hills and Robbins2012). Even more fundamental is estimation uncertainty—referring to the degree of uncertainty in the estimations an organism makes based on previous experiences (Winstanley et al. Reference Winstanley, Robbins, Balleine, Todd, Hills and Robbins2012). High levels of environmental variability will cause both unexpected uncertainty and estimation uncertainty to be high, potentially making search necessary at multiple levels of inference (Winstanley et al. Reference Winstanley, Robbins, Balleine, Todd, Hills and Robbins2012).
By being driven by environmental uncertainty, cognitive search enables an organism to identify and characterize discrepancies between existing knowledge and observations, enabling knowledge to be efficiently updated or improved to enable better adaptation.
Variability and uncertainty in hominin evolution
It is well established that hominin evolution occurred in the context of extremely high levels of variability and thus uncertainty. Environmental evidence, such as oxygen isotope measurements, reveals that the period of human evolutionary history over the past six million years corresponds with one of the most dramatic periods of climate oscillation of the past 65 million years (Potts Reference Potts1998). Hominid evolution coincides with longer and even more extreme climatic oscillations (Potts Reference Potts1998). The rate of change, degree of variability or predictability as well as the level of resource richness or scarcity will all have been subject to significant variation, with variability occurring at all time scales from daily to millennial over which longer-term evolution occurs (Potts & Faith Reference Potts and Faith2015). Adapting to these conditions would have required exceptional versatility.
Local climatic conditions for early hominins also show exceptional variability. African climate is especially influenced by variation in solar radiation (Pearson Reference Pearson2013)—an important factor in explaining the dynamics of the earth's climatic systems, particularly air circulation and rainfall. Hydroclimate extremes and habitat variability are thought to be key environmental drivers of African hominin evolution (Potts et al. Reference Potts, Behrensmeyer and Faith2018). East African climate shows periods of significant instability with drastic shifts between arid and moist conditions amongst periods of greater stability (Potts & Faith Reference Potts and Faith2015). In some periods, major shifts occurred rapidly due to factors such as volcanic or tectonic activity, while in other periods change was slower, with vegetation, water and other resources changing over spans extending beyond individual lifetimes (Potts Reference Potts1998). Thus the rate as well as the range of change differed in time and space.
Potts and Faith (Reference Potts and Faith2015) identify 32 periods of high climate variability over the past five million years. They found that nearly all key technological and biogeographic milestones in eastern Africa, as well as the first appearances of new hominin species, coincided with periods of high variability. Of these 32 periods, they identify six, which represent the most prolonged and intense periods of variability. Our own species, Homo sapiens, emerged during the sixth period which lasted from c. 358,000 to 50,000 bp (Potts & Faith Reference Potts and Faith2015, table 1) and included a hyper-arid phase during the period 186,000–127,000 bp (Pearson Reference Pearson2013).
Optimizing search is difficult to achieve at the individual level, particularly when there is variability in the rate of change. Further, variability may favour selection of those organisms that have a varied diet, with this and other adaptive behaviours such as tool use necessitating search over a broader range of domains. Corresponding cognitive search capabilities might need to co-evolve to support this. The need to search across a broader range of domains, related to food acquisition, social search, technology creation and so on, also creates further difficulties in search optimization as different domains may require different search strategies.
Increased search capacity is also likely to have been under strong selection. Survival during hyper-arid periods of resource scarcity would have depended on the accurate replication of existing survival strategies, as well as further refinement to optimize adaptive knowledge. This concurs with patch exploitation theory, which predicts that organisms will stay longer and exploit the same patch when resources are scarce (Stephens et al. Reference Stephens, Couzin, Giraldeau, Todd, Hills and Robbins2012). Resource-scarce environments are therefore likely to exert a particularly strong selection pressure for high-fidelity exploitation and local or depth-first search. Concurrently, adaptation to a variable environment relies on allocating resources towards exploration. The strength of the selection pressure for exploratory cognitive search correlates directly to the degree of uncertainty and variability in domains relevant to survival.
Selection for behavioural flexibility and adaptability to complex changing and unpredictable conditions has long been argued to be one of the key drivers in hominid encephalization (Potts Reference Potts1998; Trauth et al. Reference Trauth, Maslin and Deino2010). However, capacity in cognitive search cannot increase indefinitely. Eventually, improvements meet impassable functional and efficiency constraints. Due to strong selection pressures for greater effectiveness and efficiency in cognitive search, as well as difficulties in optimizing this process, we propose that environmental selection pressures induced a division and specialization in hominin cognition.
The evolution of complementary cognitive search
Division and specialization in cognitive search
While differences in human search behaviours have been observed (e.g. Hutchinson et al. Reference Hutchinson, Stephens, Bateson, Todd, Hills and Robbins2012), the notion that the individual members of our species are neurocognitively specialized in complementary cognitive search strategies has not been previously proposed. Division and specialization are common throughout nature. In the context of an already cooperating group, within-species division and specialization are favoured when features that confer fitness benefits are functionally incompatible (Rueffler et al. Reference Rueffler, Hermisson and Wagner2012) or when efficiency benefits to reduction of task-switching costs or specialization reach a certain threshold (Cooper & West Reference Cooper and West2018; West et al. Reference West, Fisher, Gardner and Kiers2015). These criteria correspond well to the conditions outlined above and characteristics of human cognition.
Selection pressures to reduce task-switching costs
Given the level of variability, efficiency gains from avoiding task-switching costs are likely to have been an important factor. The role of task-switching costs in division of labour is well recognized. In human economies, Adam Smith (Reference Smith1776) identified that avoidance of task-switching costs through division of labour led to greater efficiency and productive gains ‘saving of the time which is commonly lost in passing from one species of work to another’.
Similarly, organisms may need to change activities or move locations. Switching between any tasks will involve costs in time and energy, but higher costs are incurred when switching between tasks that are very different or incompatible, such as exploration and exploitation. Beyond avoiding extrinsic costs which occur due to the context in which the task is carried out, the avoidance of internal metabolic costs associated with cognitive task-switching may also be a factor in division of labour (Chittka et al. Reference Chittka, Gumbert and Kunze1997; Goldsby et al. Reference Goldsby, Dornhaus, Kerr and Ofria2012).
Specifically, different large-scale networks within the brain are involved in different aspects of cognitive search. For example, the default network is involved in the exploration of new behavioural patterns, whereas the dorsal attention network is broadly associated with high-order processing, such as evaluation, revision and exploitation (Beaty et al. Reference Beaty, Kenett and Christensen2018; Mittner et al. Reference Mittner, Hawkins, Boekel and Forstmann2016). Such networks are inhibitory of one another and switching between them incurs a measurable energy cost. Increasing uncertainty has been found to be associated with less efficient (slower and less accurate) cognitive task-switching performance (Cooper et al. Reference Cooper, Garrett, Rennie and Karayanidis2015). In the context of strong selection pressures for different search behaviours, the avoidance of cognitive switching costs alone might therefore account for some level of division and specialization in cognitive search between different individuals.
Functional constraints
Another contributing factor to division and specialization is functional constraints of the brain itself. At a certain threshold, a generalized brain reaches limits on its search capacity and efficiency. Beyond those limits, the only way to increase capacity is through specialization.
In the brain, there are many examples where increased capability in global versus local search must be traded off (see Taylor & Lockett). Such constraints are observable in evidence from very different areas of cognitive psychology and neuroscience. With regard to perceptual search, for example, various studies indicate that increased capability in global and local visual search are mutually antagonistic (Geiger & Lettvin Reference Geiger and Lettvin1987; Schneps et al. Reference Schneps, Brockmole, Sonnert and Pomplun2012). This trade-off appears to be due to physical constraints that at least partly relate to the location and density of certain (magnocellular) cells in the brain (Schneps et al. Reference Schneps, Brockmole, Sonnert and Pomplun2012).
An example of functional incompatibility also relates to the way information is stored in memory—this will affect the availability of information and how it can be used for internal cognitive search. For example, procedural memory enables skills to be automatized, supporting efficient exploitation of information (Lum et al. Reference Lum, Ullman and Conti-Ramsden2013; Nicolson & Fawcett Reference Nicolson and Fawcett2000). Conversely, if an individual has difficulty acquiring automaticity of a skill, they may still have declarative (conscious) awareness of the process. This way of processing information is less efficient with regard to exploitation, but the trade-off is that it enables exploratory search to continue, so that the process might be improved or integrated with other declarative information (Nicolson Reference Nicolson2014).
Another fundamental example of physical constraints relates to the structure of minicolumns in the brain. Minicolumns are an elementary unit in the neocortex of all mammalian brains and are essential in cortical information processing (Buxhoeveden & Casanova Reference Buxhoeveden and Casanova2002). Differences in connectivity within and between modular cortical circuits result in differences in how information is processed (Casanova & Tillquist Reference Casanova and Tillquist2008; Williams & Casanova Reference Williams and Casanova2010). The width, density and connectivity of minicolumns all affect their function, and at a certain threshold cannot be further optimized for global and local information processing simultaneously (Williams & Casanova Reference Williams and Casanova2010). Optimization in one direction must come at the cost of a deficiency in the complementary area as a result of physical trade-offs (Williams & Casanova Reference Williams and Casanova2010).
It is worth noting more generally that studies of brain evolution indicate that any significant enhancement of brain power would require a simultaneous improvement in neural organization, signal processing and thermodynamic efficiency, with such a scenario being unrealistic due to trade-offs that exist between these factors (Hofman Reference Hofman, Falk and Gibson2001). In other words, due to physical constraints, and despite their plasticity (La Rosa et al. Reference La Rosa, Parolisi and Bonfanti2020) there is little scope for individual human brains fundamentally to increase in capability (Hofman Reference Hofman, Falk and Gibson2001).
We postulate that selection to reduce task-switching costs may initially have induced division of labour in hominin cognition. It is possible that some level of division of labour in cognitive search may have evolved fairly early on in hominin evolution. Once some degree of specialization in an area of search had evolved, an individual may have become predisposed to further specialization in a similar search strategy in other domains due to shared mechanisms. For example, specialization in more exploratory search in physical space might predispose an individual to specialization in aspects of exploratory internal search.
We propose that due to functional constraints the only way that search capability could increase beyond a certain threshold was through the evolution of neurocognitive specialization: that is, individuals were specialized to navigate the search process in complementary ways, with neurocognitive specializations that supported search at different scales from more global to local or exploitation (Taylor & Lockett Reference Taylor and Lockettforthcoming). Some differences in neural structures might also have evolved so that further specialization exists regarding capability in different domains of search, but this remains speculative.
We do not suggest specialization would preclude an individual specialized more in explorative cognitive search from exploiting information, or vice versa; however, since specialization comes at a trade-off in complementary realms, they will be less efficient and have less aptitude than an individual with a more depth-first search or exploitative strategy.
Such specialization in cognitive search has significant implications for our understanding of why many aspects of cooperation and communication also evolved.
Co-evolution with lowered coordination costs
Our species’ unique ability to collaborate in complex ways at scale (Melis & Semmann Reference Melis and Semmann2010) has been difficult to explain from an evolutionary perspective. For example, there remains significant debate as to why language evolved (see Szathmáry Reference Szathmáry, Nolfi and Mirolli2010, fig. 1, for an overview of theories). Complementary Cognition provides a new explanatory framework for understanding why such sophisticated levels of cooperation and means of communication such as language evolved.
As established in economic theory, division of labour and specialization can only be beneficial in the context of lowered coordination costs. Coordination costs are incurred in coordinating work or combining the output from agents performing complementary tasks (Becker & Murphy Reference Becker and Murphy1992). Time and energy may be lost due to misinformation, conflict and mistrust and poor coordination of tasks (Becker & Murphy Reference Becker and Murphy1992). The difficulty of coordination in realistic, noisy environments limits division of labour and specialization. Given the importance of coordination costs in specialization, mechanisms for reducing them can confer strong fitness benefits.
Many examples of adaptations to reduce coordination costs exist in nature, for example the coordination mechanisms evolved by eusocial insects, such as pheromone deposition in ants (Wilson Reference Wilson1962), or the waggle dance in honeybees (Frisch Reference Frisch1967). Other factors can reduce the cost of coordination. Mechanisms evolved in our own lineage to help with coordination, such as: gaze following (Brooks & Meltzoff Reference Brooks and Meltzoff2005), iconic gesturing (Tomasello & Call Reference Tomasello and Call2019), second-order theory of mind (Gowlett et al. Reference Gowlett, Gamble and Dunbar2012) and, of course, language.
Division of labour and specialization must have occurred in the context of an initially cooperative group (West et al. Reference West, Fisher, Gardner and Kiers2015). Specialization creates a secondary selection pressure for further lowering coordination costs. We propose that many features of cooperation, particularly language, may have emerged as part of a coevolutionary process with specialization in cognitive search.
Specialization typically comes at a trade-off to reduced complementary abilities. When high effectiveness is required in multiple domains, specialization is only possible if the loss of functionality in complementary domains are compensated through other means (also called ‘compensated trait loss’). Notably, compensated trait loss can involve essential traits and can occur at various gradations, ranging from complete loss of a trait to vestigialization (Ellers et al. Reference Ellers, Kiers, Currie, McDonald and Visser2012). Compensated trait loss transforms facultative relationships into obligatory ones: that is, it makes individuals inter-dependent for survival, tightening the ecological relationship (Ellers et al. Reference Ellers, Kiers, Currie, McDonald and Visser2012). In the case of Complementary Cognition, trait loss must be mitigated through interactions with other individuals that have complementary cognitive search abilities. We propose that specialization in cognitive search created a secondary selection pressure for aspects of cooperation necessary for combining these different search capabilities.
The interaction between specialization and lowered coordination costs can be viewed as a positively reinforcing feedback loop. Environmental selection pressures induce a division of labour and specialization in cognitive search, which in turn creates secondary selection pressures for lowered coordination costs as individuals become increasingly interdependent for survival. The evolution of traits that lower coordination costs then enables further specialization to occur; thus cognitive specialization and lowered coordination costs co-evolve in a positively reinforcing feedback loop.
The evolution of cognitive specialization is further complicated by the interplay between different search strategies. For example, specialization in explorative global search and consequent trait loss may result in fitness benefits to other individuals who have a local, exploitative search bias. There is likely to be a complex interplay between direct environmental selection pressures and secondary selection pressures, dependent upon the complementary search strategies of other members of the social group. Different selection pressures acting at the individual level lead to the emergence of collectives with complementary cognitive search abilities.
The evolution of aspects of cooperation and communication in humans due to interdependence has previously been theorized by Tomasello et al. (Reference Tomasello, Carpenter, Call, Behne and Moll2005). Tomasello and colleagues propose that humans exhibit ‘shared intentionality’, that is, collaboration with others towards shared goals, requiring a unique motivation to share psychological states (Tomasello & Rakoczy Reference Tomasello and Rakoczy2003; Tomasello et al. Reference Tomasello, Carpenter, Call, Behne and Moll2005). This aligns with Complementary Cognition and the proposal that our species searches cooperatively towards shared goals.
Complementary Cognition also may contribute to our understanding of personality traits, since we can expect specialization to influence how individuals best collaborate. Different kinds of social aptitudes for information sharing and collaboration will align with different cognitive specializations. We speculate that there is some measurable correlation between personality traits and cognitive search bias.
Specialization in cognitive search and co-evolution with language
Specialization will affect which cooperative traits are selected for. For example, in physical or perceptual space, forms of gestural communication such as pointing, or shared intentionality, may have been adequate for cooperative search towards shared goals. As capability and specialization evolved in other search domains, other forms of information sharing were probably required. It becomes almost impossible to search certain domains cooperatively, especially abstract domains, without means of sharing complex abstract information. Language enables such sharing. Human language differs qualitatively from that of other animal communication systems in the use of recursion, which endows human language with a uniquely open-ended capacity to communicate abstract concepts (Hauser et al. Reference Hauser, Chomsky and Fitch2002) Complementary Cognition provides an explanatory framework for language by outlining the selection pressures that could have led to its evolution, and why a new channel of information transfer and inheritance with these properties was required.
Two justifications stand out in support of the idea that language emerged as part of a co-evolutionary process with division and specialization in cognitive search. Firstly, the requirements on the channel of information sharing differ between cooperation of cognitively similar individuals and cooperation of cognitively specialized individuals as envisaged by Complementary Cognition. The results of complementary search strategies mean that information from different search strategies must be combined.
We view language as evolving firstly as a means of facilitating co-operative search, particularly of more abstract domains. Through language, individuals with complementary search strategies can search a common information landscape and so co-create models of the world and behavioural adaptations that are beyond the capability of any one individual, or even cognitively similar individuals. Environmental variability and antagonistic selection pressures for search capability (exploratory versus exploitative, different domains, etc.) made cooperative search critical to successful adaptation.
Secondly, we follow Maynard Smith and Szathmáry (Reference Maynard Smith and Szathmáry1995) in their proposal that language can be viewed as a new channel of inheritance for information as part of a Major Transition. However, Complementary Cognition provides a more comprehensive explanation of why such a system was needed: namely, as well as facilitating co-operative search, creating composite information from results of different scales of search and different domains of search would lead to a marked increase in the complexity of the resulting information. Passing on this complex information requires a mechanism of inheritance capable of transferring information with greater bandwidth and unbounded complexity, a key feature of human language (Hauser et al. Reference Hauser, Chomsky and Fitch2002).
We therefore view language as an integral part of the system of Complementary Cognition, and suggest that the evolution of language and probably other cooperative abilities may be better understood when contextualized as part of the evolution of Complementary Cognition.
Emergent benefits of Complementary Cognition
Navigating search at the group level confers a number of important benefits such as: significant efficiency savings; globally increased capacity in search; risk mitigation at the individual and group level; and recombination of different search strategies. Overall, the combination of these benefits significantly increases the robustness of the group to environmental variability. It can be regarded as a meta-adaptation which represents a substantial qualitative improvement in evolvability.
Efficiency and capacity
Specialization confers efficiency gains through self-organization as a result of reduced time and energy cost in negotiation of task allocation.Footnote 2 Specialized individuals also have greater efficiency and effectiveness in their specialist domain compared to a generalist at the cost of complementary capabilities. However, a group of specialists will have significantly improved effectiveness and efficiency overall compared to a group of generalists, as their individual deficiencies are compensated for by other specialists. This leads to a considerable group capacity advantage.
Risk mitigation
Collaborative search amongst specialist individuals mitigates risks better than collaborative search amongst generalists, particularly in a variable environment. Simple cooperation through risk pooling is quite common. A group of hunters using the same hunting strategy may mitigate risks by pooling the resources acquired and sharing the rewards of their hunt. This preserves them from going hungry when the likelihood of catching prey is less than 100 per cent.
However, simply pooling risks still exposes collaborative groups to the risk of their common hunting methods becoming inadequate due to changes in their environment. In this case, all hunters would go hungry. They are in essence pooling the risks of a single strategy amongst themselves. If each hunter used a different hunting strategy, or perhaps mixed this with foraging, fishing, agriculture, etc., then the risk is pooled across all strategies, making the entire group more robust to a strategic failure.
This effect extends to cognitive search and search in abstract domains. Distributing risks among different strategies allows a failure in one to be absorbed by the payoffs from the others. Notably, this requires pre-existing collaboration. If individuals do not already pool risks, division of labour in different strategies will yield no benefits, and a strategy that can potentially have large payoffs with a low probability may be detrimental to individual survival.
Combining information from multiple search strategies also increases the search space and the ‘quality’ of search, avoiding the risk of being trapped in a local optimum or missing the global optimum through too diffuse a search.
Modularity
Modularity in search strategies enables reconfiguration to adapt search activities to different circumstances in time, space and across domains. Modular systems (i.e. those composed of specialized, cooperating subunits) tend to be more adaptive in general. Specialized subunits are more easily re-composed and rearranged than tightly coupled systems, enabling the reconfiguration of a system to match a new functional requirement. Computer simulations of biological evolution show that unchanging environments usually lead to non-modular networks that are slower to adapt to new environments (Clune et al. Reference Clune, Mouret and Lipson2013; Kashtan & Alon Reference Kashtan and Alon2005). Modular networks evolve when a rapidly changing environment has different overall problems made up of common subproblems (Kashtan & Alon Reference Kashtan and Alon2005). When the overall goal changes, the connections between modules can rapidly rearrange in order to adapt. Simulations show that modular separation is logarithmically proportional to the rate of variation (Lipson et al. Reference Lipson, Pollack, Suh and Wainwright2002); studies in nature also find that modularity correlates with frequency of environmental change (Parter et al. Reference Parter, Kashtan and Alon2007).
If search capabilities are modularized, retargeting the search to a different goal, or shifting the strategy to adapt to new environmental conditions, becomes a matter of changing how individuals communicate, rather than requiring any fundamental change in cognitive capability. This changes the structure of the network of collaborating individuals, without fundamentally changing the cognitive task each individual carries out, leading to adaptation at the group level, while greatly reducing the cost to adapt.
Synergistic effects
In addition to an increase in total capacity, modularity/specialization and coordination grant an increase in capability—the ability to perform qualitatively different tasks. This has been demonstrated in digital organisms, able to complete tasks that could not be computed by any single individual by sharing partial task results with each other (Goldsby et al. Reference Goldsby, Dornhaus, Kerr and Ofria2012). This qualitative difference in tasks that can be performed confers a fitness advantage to individuals who are part of collaborating groups. For Complementary Cognition, this would manifest in an ability to co-create adaptations qualitatively superior to those possible for a single individual or a cooperating group of cognitively similar individuals.
Robustness can be defined as ‘the ability of a system to maintain functionality in the face of some change or disturbance, which could be internal or external to the system’ (Jen Reference Jen2005). All the factors discussed here, risk mitigation, modularity, overall increased capacity, provide the flexibility to respond and adapt to shifting selection pressures at the group level, leading to an overall significant increase in robustness.
Interplay between search strategies
Modularity in search enables search strategies to be recombined in a variety of ways to increase search capacity and capability. Explorative search captures less detail but enhances ability to identify deeper global patterns—these can guide individuals with more local search strategies who then avoid being trapped in a local maximum. As such, global search can improve the quality and efficiency of local search. In the domain of physical space, for example, a global search of an area may identify a promising area of food that a local search would have not reached, but the local search can then harvest it more efficiently. Global search may also guide search across different domains. For example, a global search may identify a broader variety of cues in an ecosystem, enabling more accurate, longer-term predictions regarding environmental change. This can in turn guide local search in relevant domains, for example to exploit different resources not so affected by the predicted changes.
Conversely, local search will be more effective at identifying local patterns potentially missed by global search. Local search can further refine the search outcomes of individuals with a global search bias. Considering a technological adaptation as an example, an exploratory search might recombine the use of a wooden tool and a stone hand-axe in a shafted axe. This would require a creative leap, but will better succeed in the context of detailed knowledge of which tree at what time gives the appropriate wood with sufficient strength. Essential in the success of the final shafted axe is the outcome of global search making the leap to recombine wood and stone in a novel manner, but also detailed in-depth understanding of wood and stone as materials, and the local depth-first search of these domains.
Creating a common information landscape from different search strategies using language leads to information that is more adaptive than is possible through individual cognition, or with populations sharing common cognitive search strategies. These collaborations may be possible with protolanguage, or without language, but many domains of search, especially abstract domains, become almost impossible to search cooperatively without means of sharing complex abstract information.
Cognitive search from global/exploratory to local/exploitative may build on the results of previous searches. These may be sourced from memory or acquired from other individuals. Through social learning mechanisms, individuals can ‘inherit’ information gathered through previous searches from parents (vertical inheritance) or peers (horizontal inheritance). Information may be passed down through multiple generations, potentially updated and modified through multiple searches across generations.
Implications for cultural evolution
Neurocognitive specialization in search has not previously been considered, nor has its potential effect on cultural evolution. With regard to inheritance of adaptive knowledge (i.e. the results of previous searches), one aspect to consider is that individuals with different cognitive specializations may be more reliable sources for different kinds of socially learned information. For example, specialists with an exploitative bias may be better at faithfully copying and recalling detailed or procedural information passed down through generations, such as the correct sequence and way of making a tool. Conversely, those with an explorative search will be better able to identify global patterns in inherited information, enabling generalizations and predictions about unknown or ambiguous situations. In this way, cognitive specialization may also increase the bandwidth and fidelity of inherited adaptive information as well as increasing capability to update shared knowledge.
Cooperative search enables group knowledge to undergo a process of ‘quality control’. A prediction error between knowledge held in the social group (i.e. the results of previous searches) and observations, or misalignment between different kinds of knowledge in the social group, can target further search to resolve those discrepancies specifically. Expending resources to targeted search confers significant efficiency benefits, continuously improving the adaptiveness of inherited information. Different search strategies enable the creation of different models of the world and will identify different kinds of prediction errors. Cooperative search therefore enables individuals to co-create adaptations of much greater adaptive value. As a consequence of this process of inheritance, search through different strategies to update adaptive knowledge and co-creation of adaptive information, we see evolution in those adaptations over time, that is, cultural evolution.
Discussion: relation to existing research and areas for future research
Alignment with variability selection
Complementary Cognition aligns strongly with Potts’ ‘Variability Selection’ hypothesis, which proposes that, rather than being adapted to a particular environment, humans are adaptive with respect to environmental variability, that is, inconsistency of selective conditions (Potts Reference Potts1998; Potts & Faith Reference Potts and Faith2015; Potts et al. Reference Potts, Behrensmeyer and Faith2018). Complementary Cognition enables precisely this—significantly, it increases the capacity to adapt not to a particular environment, but to any kind of environmental context—any information landscape.
Cultural evolution
Cultural innovation and transmission have long been seen as analogous to genetic mutation and transmission (Boyd & Richerson Reference Boyd and Richerson1985; Cavalli-Sforza & Feldman Reference Cavalli-Sforza and Feldman1981). The framework of search highlights the similarity between these processes. Genetic search drives phenotypic adaptation and cognitive search drives behavioural adaptation. In a similar manner, we propose that Complementary Cognition (cooperative cognitive search) lies at the heart of explaining the exceptional level of cultural adaptation in our species.
As highlighted by Page (Reference Page2011, 160), ‘for any type of entity, the appropriate level of variation [search] will eventually emerge from the system. Moreover, that level will tend to track the rate at which the system churns’. In this manner, the evolution of Complementary Cognition enabled us to adapt to the high levels of variability found in our species’ evolutionary history.
When variability or scarcity diminishes, however, the increased collective search capacity represents an over-adaptation relative to the environment in which it evolved. Information is still created and updated, but becomes obsolete much more slowly. This would lead to an accumulation of adaptive information: cumulative cultural evolution. The mechanisms of accumulation described by Complementary Cognition may be tested and help improve our existing models of cultural evolution.
Apparent gap between behaviourally and anatomically modern humans
Complementary Cognition could contribute to understanding the apparent delay of around 100,000 years between anatomically modern humans and typically modern human cultural behaviour (Sterelny Reference Sterelny2011). It seems that behavioural flexibility increased without any sign of concomitant change in physical adaptations, including increase in brain size—a phenomenon that Renfrew dubbed the ‘sapient paradox’ (see e.g. Renfrew Reference Renfrew2008). Potts and Faith (Reference Potts and Faith2015) identified that the period from 358,000 to 50,000 bp was among the most prolonged and intense periods of variability in our evolutionary history. These observations concur with the theory of Complementary Cognition. We expect increase in behavioural flexibility and adaptiveness to have been selected for during the period of variability, but more clearly manifested once that variability had subsided (no longer running to stand still). However, we would not necessarily expect concurrent encephalization, since we propose that increased capability emerged through a process of specialization and compensated trait loss. Trait loss is hidden at the functional level as long as the ecological interaction is maintained and so its prevalence tends to be grossly underestimated (Ellers et al. Reference Ellers, Kiers, Currie, McDonald and Visser2012). We suggest that its visual as well as functional invisibility explains why specialization in cognitive search has hitherto gone unrecognized.
Evolutionary theory of language and cooperation
Several different theories have be proposed to explain the evolution of language (Hauser et al. Reference Hauser, Yang and Berwick2014; Szathmáry Reference Szathmáry, Nolfi and Mirolli2010, fig. 1). Complementary Cognition provides a new theory of how language evolved as a means of enabling cooperative search and as an inheritance mechanism for the more complex adaptive information that resulted.
Beyond language, certain cooperative traits may also have co-evolved with cognitive specialization. It is beyond the scope of this paper to provide an adequately in-depth exploration of these possibilities. Some examples might include second- or third-order theory of mind (Gowlett et al. Reference Gowlett, Gamble and Dunbar2012), which would enable convergence to a common understanding faster than first-order theory of mind, and if so would facilitate co-operative search towards shared goals. Complementary Cognition may also provide insights into why humans are characterized by such extensive non-kin cooperation.
Major transition
We propose that the evolution of Complementary Cognition can be characterized as a major transition (Taylor & Fernandes Reference Taylor and Fernandesforthcoming). These rare evolutionary events occur when evolution favours cooperation, division of labour, specialization and interdependence to such a degree that there is a loss of individuality (Maynard Smith & Szathmáry Reference Maynard Smith and Szathmáry1995; West et al. Reference West, Fisher, Gardner and Kiers2015). The transition brings about a new level of complexity that enables behaviour not previously possible (Suki Reference Suki2012).
The Major Transitions framework was first proposed by Maynard Smith and Szathmary (Reference Maynard Smith and Szathmáry1995). They regarded the transition from societies with protolanguage to modern societies with language as the last of the major evolutionary transitions in their framework (Maynard Smith & Szathmáry Reference Maynard Smith and Szathmáry1995; Szathmáry Reference Szathmáry, Nolfi and Mirolli2010). The notion that a major transition occurred with regard to our own species has remained controversial, however (see Calcott et al. Reference Calcott, Sterelny and Szathmáry2011). We suggest that the proposed theory on the evolution of Complementary Cognition supports Maynard Smith and Szathmary's argument that language evolved as part of a major transition, but provides a new explanation of this transition that aligns much more closely with their own theoretical framework than previously posited descriptions (Taylor & Fernandes Reference Taylor and Fernandesforthcoming).
Implications and significance
Summary of Complementary Cognition
We have proposed that humans primarily adapt and evolve via a hitherto unrecognized system of complementary cognitive search, which we call Complementary Cognition.
High levels of variability prevailed throughout our species’ evolutionary history. These created strong selection pressures for greater effectiveness and efficiency in cognitive search, as well as difficulties in optimizing this process. These selection pressures induced a division and specialization in human cognitive search in different but complementary cognitive search strategies.
This division and specialization created secondary selection pressures, co-evolving with language and other aspects of cooperation, enabling cooperative search and more effective adaptation across multiple domains. Significantly, a consequence of this adaptation is that our species navigates cognitive search at the group level.
We propose a new evolutionary theory of language, namely that as well as enabling greater cooperative search, it also evolved as a new inheritance channel to enable sharing of the more complex adaptive information that resulted from recombining information from multiple search strategies. Language can thus be contextualized as an integral part of this system of Complementary Cognition.
Through specialization in complementary search strategies, as well as new mechanisms of inheritance, this system enabled higher-fidelity inheritance of adaptive information. It also provided a system of quality control for adaptive information—search across different scales from more global to local as well as across domains (e.g. time, social space, aspects of the natural world) to identify errors with pre-existing adaptations that could be updated to improve their fitness value. Through searching cooperatively and combining the results of different strategies, it also enabled the co-creation of cultural adaptations of much higher adaptive value.
Given the meta-adaptive nature of Complementary Cognition, its emergence represents a significant transition in evolvability, enabling substantially greater capability, speed and flexibility to adapt, than search at the level of genetic evolution, individual cognition, or collaboration of neurocognitively non-specialized individuals alone.
The shift in the topology and nature of information sharing is sufficient that we propose Complementary Cognition can be characterized as a Major Transition. We suggest that the evolution of this system reached its greatest extent during the last of the intense periods of variability highlighted by Potts and Faith (Reference Potts and Faith2015) (c. 358,000 to 50,000 bp) with the transition in adaptive capability it afforded being manifested in the origins of behavioural modernity.
Finally, we propose that Complementary Cognition lies at the heart of explaining the exceptional levels of cumulative cultural evolution that characterize our species. We suggest that accumulation of adaptive information occurred at times when our evolved ability to search—which evolved due to high levels of variability and scarcity—became hyper-adaptive during periods of relative stability and abundance.
Complementary Cognition within a hierarchy of evolutionary systems
Complementary Cognition can be contextualized as part of a hierarchy of self-similar systems, each of which contributes to the adaptation and evolution of our species. This hierarchy includes phenotypic adaptation and evolution, which is driven by genetic search and natural selection and behavioural adaptation, driven by cognitive search (Fig. 1). The levels of search, adaptation and evolution identified here correspond to those proposed by Eva Jablonka and Marion Lamb in their book Evolution in Four Dimensions (Reference Jablonka and Lamb2005). While we do not discuss epigenetic evolution, this can also be viewed from the perspective of search (Stolfi & Alba Reference Stolfi and Alba2017).
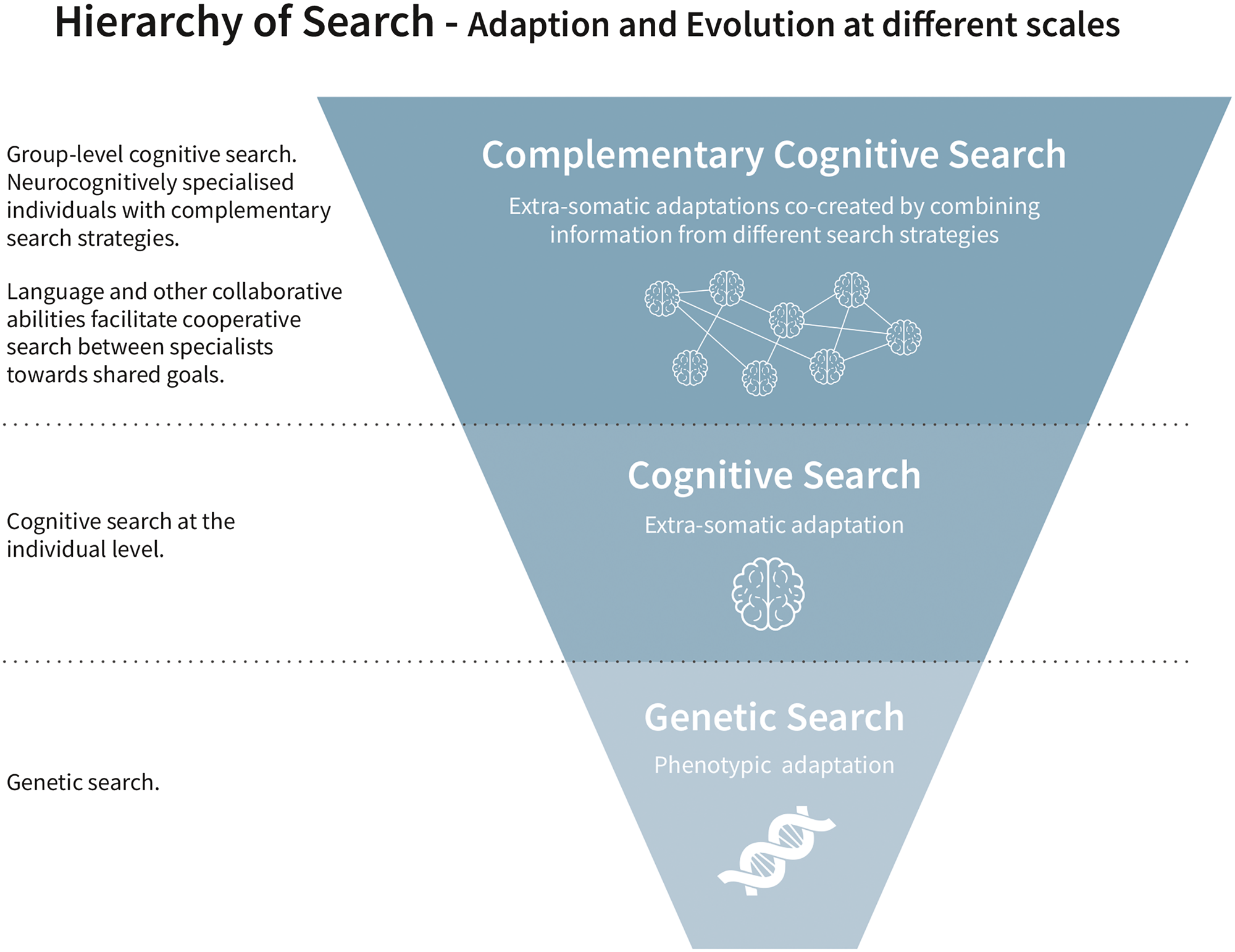
Figure 1. Hierarchy of search.
Although evolution and cognition are clearly established areas of scholarship, they are not always considered from the perspective of search in archaeology. The framework of search enables us to see how adaptation and evolution at the genetic, cognitive and complementary cognitive scales are analogous. All three identified systems have mechanisms by which previous search results (adaptive information) can be selected, stored, updated and inherited. Because new searches can build on the results of past searches, we see evolution over time, be that manifested in phenotypic or extra-somatic (including behavioural and cultural) adaptations. At each scale, variability and uncertainty create the selection pressure for improved search capabilities, leading to the emergence of a new level of search to adapt.
Limitations
It is important to not over-simplify our discussion regarding Complementary Cognition. Whilst grounded in the theory of complex adaptive systems, this article should be seen as an overview to contextualize further development and publication of specific forthcoming research. We have provided a broad overview of the evolution of cooperative cognitive search, but it is by no means a comprehensive overview of cognitive evolution in humans. For the sake of brevity, for example, many details were omitted regarding the cognitive evidence which are presented elsewhere (Taylor & Lockett Reference Taylor and Lockettforthcoming) and more in-depth treatments of the complex interactions between search biases and domains of search were left undiscussed. We also speculate that domains of search play an important role in specialization and affect the evolutionary trajectory of our species, but these have not been categorized and explored. It is likely there exists some level of sub-specialization regarding preferred domains of search in addition to general search tendencies.
Complementary Cognition should be seen as a starting point in exploring a rich area of human evolution, rather than an end. There remains a great deal of research to be carried out to understand the implications of Complementary Cognition for the modern population and systems, an area of particular practical interest. We hope Complementary Cognition can be used as a lens to explore these areas fruitfully.
Closing remarks
The fact is that no species has ever had such wholesale control over everything on earth, living or dead, as we now have. That lays upon us, whether we like it or not, an awesome responsibility. In our hands now lies not only our own future, but that of all other living creatures with whom we share the earth.
(Attenborough Reference Attenborough1979, 308)Our species’ evolutionary history was shaped by climate change and substantial environmental variability. We are now facing a period of dramatic climate change, in which our environment will vary at a rate hitherto unforeseen in human history. However, this time, human activity is the cause of variability. We have in a sense come full circle. This may be more connected than we realize.
This paper has proposed that our species adapts and evolves via a system of group level cognition, that is analogous to evolution at the genetic level. Hierarchical systems evolve from the bottom up—the reason the upper level evolves is to serve the purposes of the lower levels (Meadows & Wright Reference Meadows and Wright2015). That is, if adaption at the level of Complementary Cognition fails, adaptation will eventually fail more generally.
Successful adaptation relies on a careful balance of cognitive specializations and effective collaboration. Not balancing this well can lead directly to maladaptive behaviours and culture. Given the scale at which we now operate, such maladaptive behaviour can have a rapid and significant impact. Complementary Cognition has enabled us to adapt to different environments, and may be at the heart of our species’ success, enabling us to adapt much faster and more effectively than any other highly complex organism. However, our specialization and reliance on the appropriate balance of capabilities may also be our species’ greatest vulnerability.
The impact of human activity on the environment is the most pressing and stark example of this. Ultimately, climate change is caused by a failure to update our behaviour and adapt.
We are facing severe negative consequences as a result of not acting on information we have known about for decades, with the scope and scale of those consequences increasing month on month. How did the most adaptive complex organism on earth reach this point?
The challenge of collaborating and co-adapting at scale creates many difficulties. We believe that we have also unwittingly put in place a number of cultural systems and practices that may be undermining our ability to adapt (see Taylor & Lockett Reference Taylor and Lockettforthcoming). These self-imposed limitations disrupt our complementary cognitive search capability and may restrict our capacity to find innovative and creative solutions to the problems that we face.
Through evolutionary chance, we have been placed in a privileged position to shape not only the destiny of our own species, but that of the rest of the planet. We face a period of unprecedented environmental and cultural changes brought about by human activity, and the rate of change itself is continually increasing.
It is critical that we both understand and harness this system of Complementary Cognition. We believe that only through such understanding, and an understanding of the evolution of cognitive search, can we harness our full potential and design systems, institutions and policies that enable us to create an adaptive and sustainable society.
Acknowledgements
Thank you to Mona Khatibshahidi and Dr Adrian Wallbank for advice and help with writing and editing and Dr Brett Calcott for reading drafts and very helpful suggestions. The research was supported by Professor Nigel Lockett and Dr Cameron Petrie.