INTRODUCTION
Morpheme studies refers to the series of studies that have investigated the acquisition order of grammatical morphemes by first language (L1) and second language (L2) learners. The central question of these studies was whether learners show a universal pattern in the acquisition order of morphemes. If present, a universal pattern could indicate the existence of a universal mechanism necessary to acquire language (Dulay & Burt, Reference Dulay and Burt1973). A series of studies established that L2 learners follow a universal order in the acquisition of L2 English morphemes, a view that remains dominant to this day. However, in the 40 years since the first morpheme studies, SLA research has established the influence of the L1 on L2 acquisition (e.g., Ellis, Reference Ellis2006; Ionin & Montrul, Reference Ionin and Montrul2010; Jarvis & Pavlenko, Reference Jarvis and Pavlenko2007; Odlin, Reference Odlin1989). It is natural to expect the L1 to also influence the order of morpheme acquisition. Importantly, a recent survey of morpheme studies by Luk and Shirai (Reference Luk and Shirai2009) strongly suggests L1 influence. We therefore revisit the morpheme studies to investigate L1 influence, at the same time addressing some of the methodological shortcomings of previous studies. In particular, we explore the Cambridge Learner Corpus, a rich empirical resource that allows us to investigate the question by drawing from a rich data set of learner data from seven L1 groups across five proficiency levels.
LITERATURE REVIEW
Morpheme Studies
Brown (Reference Brown1973) examined the L1 English acquisition of 14 grammatical morphemes by three children and found that the developmental patterns were similar across the three children. Following Brown’s study, similar investigations emerged in SLA research to establish whether L1 and L2 acquisition show similar patterns. Dulay and Burt (Reference Dulay and Burt1973) investigated the acquisition order of eight grammatical morphemes (present progressive -ing, plural -s, irregular past tense, possessive ’s, articles, third-person -s, copula be, and auxiliary be) by three groups of children learning English as a L2: 95 Mexican-Americans, 26 Spanish, and 30 Puerto Ricans. The researchers predicted that the three groups would yield the same order, but that the order would be different from the order observed in L1 acquisition, because L2 learners already possess semantic distinctions that would affect L2 acquisition of mappings of semantic functions to morphemes.
Central to morpheme studies is their focus on accuracy of use as a measure of acquisition. Footnote 1 To measure accuracy, Dulay and Burt (Reference Dulay and Burt1973) calculated the percentage of correct forms in the contexts in which each morpheme was obligatory. Many subsequent studies followed this practice and adopted the related assumption that accuracy reflects the degree of acquisition (cf. de Villiers & de Villiers, Reference De Villiers and de Villiers1973). Dulay and Burt (Reference Dulay and Burt1973) found that the L2 order of acquisition was different from the acquisition order reported in Brown (Reference Brown1973) for L1 learners. But at the same time they found that all the three groups of L2 learners they investigated showed the same order, a result that led Dulay and Burt to conclude that L2 acquisition is guided by universal strategies giving rise to a universal order of L2 morpheme acquisition.
To confirm the hypothesis of the universal order of morpheme acquisition, Dulay and Burt (Reference Dulay and Burt1974) investigated L1 influence on acquisition order. They compared 60 six- to eight-year-old L1 Spanish children with 55 L1 Chinese children of the same age learning English, and they tested the acquisition order of 11 morphemes. They found a high correlation between the two groups of learners, from which they concluded that there is a consistent acquisition order of grammatical morphemes across L2 learners, despite different L1 backgrounds. Subsequent studies further confirmed the existence of a universal order of acquisition in adult L2 learners (Bailey, Madden, & Krashen, Reference Bailey, Madden and Krashen1974), in both ESL (English as a second language) and EFL (English as a foreign language) learners (Pica, Reference Pica1983a), and in both instructed and noninstructed learners (Larsen-Freeman, Reference Larsen-Freeman1975).
An early criticism of morpheme studies was that the order of acquisition conceals the relative distance in accuracy. A 1% difference in accuracy between two morphemes can result in the same ranking as a difference of 50%. To address this criticism, Krashen (Reference Krashen, Brown, Yorio and Crymes1977) reviewed the literature and grouped up in a single rank morphemes with similar accuracy scores. Based on his analysis, he proposed the “natural order” shown in Figure 1, which is believed to be universally followed by L2 learners of English. The universality of a fixed natural order has since been widely accepted (Luk & Shirai, Reference Luk and Shirai2009) among researchers of varying theoretical perspectives (Meisel, Reference Meisel2011; Ortega, Reference Ortega2009) and is presented as a basic finding in SLA textbooks (Luk & Shirai, Reference Luk and Shirai2009). Footnote 2

Figure 1. The natural order of acquisition proposed by Krashen (Reference Krashen, Brown, Yorio and Crymes1977).
In the 1980s, focus shifted to explanation, and a number of factors (e.g., complexity and frequency) were proposed to account for the observed order (Larsen-Freeman, Reference Larsen-Freeman1976; VanPatten, Reference VanPatten, Eckman, Bell and Nelson1984; Zobl & Liceras, Reference Zobl and Liceras1994). Given the belief in a universal natural order, L1 has been neglected as a determinant of the order of acquisition. This view, however, is beginning to change.
L1 Influence in Morpheme Studies
The universality of the natural order was consistent with the view that morphological crosslinguistic influence is weak, at least in comparison to phonology or lexis (Jarvis & Pavlenko, Reference Jarvis and Pavlenko2007). At the same time though, research on the acquisition of the English article has shown clear L1 effects (e.g., J. A. Hawkins & Buttery, Reference Hawkins and Buttery2010; Jarvis, Castañeda Jiménez, & Nielsen, Reference Jarvis, Castañeda Jiménez, Nielsen, Jarvis and Crossley2012; Snape, Reference Snape2005, Reference Snape2008; Zdorenko & Paradis, Reference Zdorenko and Paradis2012).
Luk and Shirai (Reference Luk and Shirai2009) reviewed the literature to investigate whether Japanese, Korean, Chinese, and Spanish learners of English acquire grammatical morphemes following the natural order. They found some clear L1 effects. For instance, Japanese, Korean, and Chinese learners acquire the possessive ’s earlier than predicted by the natural order and show later acquisition of the plural -s. However, Luk and Shirai (Reference Luk and Shirai2009) is a review paper drawing from a very diverse set of studies varying in length (longitudinal vs. cross-sectional), criteria of acquisition (accuracy order vs. the threshold of 80% or 90% correct), and mode (oral vs. written production), while its scope is limited to three grammatical morphemes.
Building on Luk and Shirai’s findings, the goal of our article is to investigate L1 effects in a more systematic way. First, we extend our investigation to six morphemes. We hypothesize that the lack of the equivalent feature in the L1 leads to low accuracy, a hypothesis confirmed for the acquisition of the article (e.g., J. A. Hawkins & Buttery, Reference Hawkins and Buttery2010; Ionin & Montrul, Reference Ionin and Montrul2010) but not investigated in a comparative way for six morphemes.
The second question we investigate is whether all of the six morphemes are equally vulnerable to L1 influence—whether, for example, the acquisition of the article and plural -s is equally affected when there are no corresponding morphemes in the L1. This question has not yet been investigated empirically within morpheme studies, and to the best of our knowledge no theoretical account makes explicit predictions regarding this question for the set of morphemes investigated here. We can, however, extract some predictions from existing proposals discussing related issues. Some SLA researchers signal the mapping of semantic/functional features to their morphological realization in the L2 as particularly challenging for learners (Slabakova, Reference Slabakova2014), with the article and aspectual distinctions among the hardest such mappings (DeKeyser, Reference DeKeyser2005). At the same time, other theoretical accounts predict that it is the morphological features generally lacking semantic content (i.e., “uninterpretable” features) that are harder to acquire in the L2 (this is known as the “interpretability hypothesis”; see Tsimpli & Dimitrakopoulou, Reference Tsimpli and Dimitrakopoulou2007).
Slobin (Reference Slobin, Gumperz and Levinson1996) offers a more explicit discussion of the question of which kind of morphemes are more susceptible to L1 influence. He draws a distinction between grammatical categories that correspond to language-specific concepts—categories of “thinking for speaking”—and grammatical categories corresponding to general semantic concepts—categories of thought. Definiteness and aspect are examples of language-specific concepts, because neither definiteness nor perfect or progressive aspect are properties of the world that can be experienced independently of language, through our senses and perceptual system. Rather, they are language-specific concepts that are learned through language use. Plurality, on the other hand, is a language-independent concept, a category of thought (notwithstanding the typological variation in the way languages express plurality and number crosslinguistically). He then predicts that language-specific categories of thinking for speaking like definiteness, aspect, and voice will be more vulnerable to L1 influence, whereas language-independent concepts like plurality will be less influenced.
With the caveat that neither Slobin’s proposal nor the interpretability hypothesis have explicitly discussed the question of L1 vulnerability for the six morphemes of our study, we can attempt to extract some predictions for our study by combining insights from the two approaches. Slobin predicts differential vulnerability to the L1—in particular, that articles and aspectual morphemes ought to be more sensitive to L1 influence than, for instance, plural -s. No specific predictions can be made for an agreement morpheme like third-person -s that does not require acquisition of a new concept (e.g., definiteness) and does not correspond to a language-independent concept, as number marking does. However, if the interpretability hypothesis is correct, we would expect this purely syntactic feature to be harder to acquire than all other features encoding some semantic function. We would therefore expect the third-person agreement -s to be most vulnerable to the L1, followed by the articles and aspectual/tense morphemes, followed by number morphemes.
The Methodological Challenges of Transfer Studies
Jarvis (Reference Jarvis2000) proposes three empirical criteria for demonstrating L1 influence or transfer on L2 acquisition: (a) intragroup homogeneity, (b) intergroup heterogeneity, and (c) crosslinguistic performance congruity. Learners with the same L1 should show the same acquisition order among them (intragroup homogeneity), whereas learners from different L1 backgrounds should exhibit different acquisition orders (intergroup heterogeneity). Intergroup heterogeneity then needs to be linked to the existence of a corresponding morpheme in the L1 performing in a similar manner with the L2. For instance, early acquisition of possessive ’s by Japanese learners of English can be linked to the Japanese particle -no, which expresses possession. It is crucial that intragroup homogeneity and intergroup heterogeneity are investigated in a single research design because they are inherently relative, relying on a comparison of how similar or dissimilar groups of learners are from each other (Jarvis and Pavlenko, Reference Jarvis and Pavlenko2007). However, there are few such studies, a gap addressed in this article.
Aims and Organization of the Article
Adopting the empirical criteria set by Jarvis (Reference Jarvis2000), our main goal is to investigate L1 influence on morpheme acquisition. Our research questions are summarized below.
-
1. Does L1 affect the accuracy order of L2 English grammatical morphemes?
-
a. Is the accuracy order consistent within each L1 group?
-
b. Is the accuracy order different among L1 groups?
-
c. Can we attribute differences in accuracy order among L1s to specific properties of the L1?
-
-
2. How strong is L1 influence in determining the accuracy of English grammatical morphemes compared to other factors such as general proficiency?
-
3. Are grammatical morphemes equally or differentially affected by the L1?
-
4. Can we link L1 influence to the absence or presence of congruent morphemes in the L1 in a systematic way?
An important feature of our investigation is that it involves learner groups across proficiency levels, therefore allowing us to ask if, within a given L1 group, the accuracy order remains the same across proficiency. If accuracy order varies as learners progress, then the idea of operationalizing the order of acquisition as the order of accuracy is in doubt.
The structure of the article is as follows. The next section introduces the Cambridge Learner Corpus, the data source of our study, and elaborates on the specific subset of data used in the study and the way information was extracted from the data. We then analyze the data to answer the preceding research questions. As we will see, strong L1 influence was observed in the acquisition order. We then grouped L1s into those with corresponding morphemes and those without and modeled accuracy based on the typological difference in L1 and general proficiency so as to investigate the strength of L1 influence and morpheme-specific L1 effect. We finally discuss the findings with reference to previous morpheme studies and to the predictions extracted from Slobin’s approach and the interpretability hypothesis.
METHOD
Target Morphemes
We targeted the six most frequently studied morphemes, which were also included in the meta-analysis by Goldschneider and DeKeyser (Reference Goldschneider and DeKeyser2001): articles, past tense -ed, plural -s, possessive ’s, progressive -ing, and third-person -s. Articles included both indefinite (a, an) and definite (the) forms. We targeted only regular past tense forms ending in -ed. Following Dulay and Burt (Reference Dulay and Burt1974), we excluded irregular forms (e.g., went, ate) and modal verbs (e.g., would, could). We also excluded all other forms ending in -ed, such as passives or participles (e.g., Do you know someone called George?). For plural -s we included both -s and -es but not irregular forms (e.g., teeth, children). Regarding possessive ’s, we included all possessive markers with an apostrophe (’s, s’). For progressive -ing we targeted all progressive uses of -ing regardless of tense and aspect (e.g., present progressive, past progressive, present perfect progressive). However, we did not target other uses of -ing, such as gerund or participial forms (e.g., I know the tall man standing over there.). Regarding third-person -s, we included -s, -es, and has. Don’t/doesn’t as a negative marker was not counted, but have/has as an auxiliary verb, including the negative form (haven’t/hasn’t) or the modal use (have/has to), was counted. The reason for excluding don’t/doesn’t was to avoid doubly counting third-person -s errors in sentences such as He don’t eats breakfast. Here, if we count don’t as an omission error and eats as an overgeneralization error, we tally two errors for one main verb, and this can unfairly lower the accuracy score of third-person -s. Note that if a verb with third-person -s was wrongly supplied instead of that with past tense -ed or a participial form (e.g., plays instead of played, wakes instead of woken), we did not count the instance as an error of third-person -s. We excluded the third-person be form, is, from counts of third-person agreement. Be is exceptional in showing three person contrasts (am/was, is/was, are/were) and for this reason has usually been treated separately in morpheme studies (e.g., Dulay & Burt, Reference Dulay and Burt1974).
Target L1 Groups
The choice of L1s was partially determined by the structure of the corpus, in that we selected L1s with sufficient amounts of data across the proficiency spectrum. We then selected L1s that could provide a typologically diverse set for comparison. We thus included L1 Japanese, Korean, Spanish, Russian, Turkish, German, and French learners of English.
Corpus
The Cambridge Learner Corpus (CLC) consists of English language learners’ exam scripts from the Cambridge English Language Assessment exams. It has been collaboratively developed by Cambridge English Language Assessment and Cambridge University Press. The corpus currently contains 45 million words from 135,000 exam scripts. More than one third of the corpus has been manually error-tagged by linguists at Cambridge University Press (J. A. Hawkins & Buttery, Reference Hawkins and Buttery2010; J. A. Hawkins & Filipović, Reference Hawkins and Filipović2012; Nicholls, Reference Nicholls2003). The CLC contains exam scripts from lower to advanced proficiency levels, aligned to the Common European Framework of Reference (CEFR), and is, thus, suitable for developmental analysis. The writing tasks cover a range of text types, including an article, an essay, a letter, and a story. Learner metadata include L1 background, collected through a short questionnaire administered to candidates when they take their exam. In sum, the CLC is a large learner corpus allowing us to investigate L1 effects for multiple L1s across proficiency.
The corpus contains parts of speech and grammatical relations annotations provided by the Robust Accurate Statistical Parser (RASP; Briscoe, Carroll, & Watson, Reference Briscoe, Carroll and Watson2006), using the CLAWS2 tagset. Each script, thus, has four versions: raw text, error-tagged text, corrected text, and part-of-speech-tagged text. The combination of error tags and part-of-speech tags enables investigation of morpheme acquisition. Part of the corpus with 1,244 exam scripts is publicly available at the following URL (Yannakoudakis, Briscoe, & Medlock, Reference Yannakoudakis, Briscoe and Medlock2011): http://ilexir.co.uk/applications/clc-fce-dataset/.
Subcorpus
In our study we used the exam scripts from the Main Suite Examinations. Our subcorpus consists of five proficiency levels corresponding to A2–C2 from the CEFR: KET (A2), PET (B1), FCE (B2), CAE (C1), and CPE (C2). It includes exam scripts of passing grades, A–C, as well as failing grades D and E. Technically, candidates with failing scripts have not reached the proficiency (CEFR) level of the corresponding exam. For our purposes, though, it is reasonable to assume that, overall, the proficiency of candidates is higher for higher exams, irrespective of whether they pass or fail the exam. We demonstrate this point in the next section.
Turning to the content of the exams, Footnote 3 in KET and PET, writing sections are combined with reading sections, but the subcorpus includes only the answers to the free writing tasks. The levels FCE through CPE have independent writing sections with two questions, and the responses to the two questions were combined and included. The writing questions involve a range of task types and elicit a variety of functions. At the FCE level, for example, test takers are asked to write a letter or an e-mail for the first question and an article, an essay, a letter, a report, a review, or a story for the second. The former involves such functions as advising, apologizing, and comparing, whereas the latter involves describing, explaining, and giving information.
The length of the scripts and the time limit vary across exam levels and from year to year. More time is typically available for longer scripts at higher exam levels. At present, the time limit for the writing section in FCE is 1 hr and 20 min for two tasks, whereas that for CAE and CPE is 1 hr and 30 min. The length of the scripts is given in Table 1. For further information on the exams and sample papers, see Hawkey (Reference Hawkey2009), Weir and Milanovic (Reference Weir and Milanovic2003), and Shaw and Weir (Reference Shaw and Weir2007), as well as the aforementioned handbooks.
Table 1. Number of scripts and words in the subcorpus used in the study

Table 1 shows the number of scripts and words as well as the average length of a script in each L1 and proficiency level in the subcorpus used in our study. Footnote 4 As expected, average script length increases in higher exam levels. Our subcorpus is made up of 11,893 scripts, a total of 4 million words.
Comparing the MTLD across the Five Levels
To evaluate how exam scripts at different levels relate to proficiency, we employed the measure of textual lexical diversity (MTLD) as an index of learner proficiency. The MTLD measures lexical diversity. It is relatively unaffected by text length and therefore suitable for our data, given the variation in text length across exams from different exam levels (Koizumi & In’nami, Reference Koizumi and In’nami2012; McCarthy & Jarvis, Reference McCarthy and Jarvis2010). Footnote 5 The measure represents the mean number of words that are necessary to satisfy the prespecified value of type-token ratio (TTR; the number of types, or unique words, divided by the number of tokens). In line with the literature, we set the TTR value to 0.72. Intuitively, the MLTD provides insight on the vocabulary and lexical diversity growth of learners’ language (for the technical details, see McCarthy & Jarvis, Reference McCarthy and Jarvis2010).
An R script was written to compute the MTLD in each exam script. Figure 2 visualizes the MTLD values across L1 groups and exam levels (excluding some KET writings because their MTLD values were higher than 200). Footnote 6 The horizontal dashed line in each panel represents the mean MTLD across the L1 groups in the exam level. The value tends to be relatively stable within each exam level and gradually rises from low to high levels, indicating lexical development. The exception to this pattern is the unexplained decrease from CAE to CPE. Nevertheless, overall the figure indicates that candidates for the same exam are of roughly equal proficiency and that those sitting different exams differ in their proficiency. In using the MLTD, we follow a long tradition of using lexical diversity measures to evaluate proficiency (e.g., Michel, Kuiken, & Vedder, Reference Michel, Kuiken and Vedder2007; Robinson, Reference Robinson2001; see also Bulté & Housen, Reference Bulté, Housen, Housen, Kuiken and Vedder2012). Because our purpose is only to confirm that exams from different levels are linked to proficiency, we have not explored a wider range of proficiency measures (cf. Lu, Reference Lu2010).

Figure 2. The MTLD for each L1 and exam level.
Scoring Method
We adopted accuracy of use to measure order of acquisition, following common practice in previous morpheme studies. We used the target-like use (TLU) score as calculated by the following formula (Pica, Reference Pica1983b):

The TLU score takes into account not only omission errors (as does the suppliance in obligatory context score, discussed subsequently) but also overgeneralization errors.
Because the majority of morpheme studies use the suppliance in obligatory context (SOC) score, we also calculated the SOC for comparisons with earlier work. The key difference between the TLU and SOC is that the latter does not take overgeneralization errors into account. To measure the SOC, all instances in which the target morpheme is obligatory are identified, and then the number of correctly supplied morphemes is divided by the number of obligatory contexts. When a morpheme is supplied in an inaccurate form (e.g., He’s eats instead of He’s eating for progressive -ing), half a point is given. Thus the SOC is calculated by the following formula:

Data Extraction
We used the corrected versions of the CLC to identify obligatory contexts, adding up all instances of a morpheme in the corrected text. Using the error-tagged exams, we subtracted the number of errors from the number of obligatory contexts to obtain the number of correct suppliances. Perl scripts were used to extract this information. See the Appendix for the further details on data retrieval.
Table 2 reports the accuracy of the scripts used to extract errors. Here, a hundred errors for each morpheme were manually identified according to the proportion of the total number of words in each L1 and the proficiency level out of the whole subcorpus. We used the error tags of the CLC to identify errors and evaluated our scripts against the CLC error annotations. We evaluated the performance of our scripts by measuring their accuracy in precision and recall of error retrieval. Precision refers to the degree to which what the script captures accurately includes what it is intended to capture, and recall refers to the degree to which the script captures what it is intended to capture. For instance, if a script to count the frequency of past tense -ed errors identified 80 of 100 instances of the errors and 70 of those 80 correctly included the target errors, then the precision is 87.5% (70/80) and the recall is 70% (70/100). F1 is the harmonic mean of precision and recall and represents the total accuracy. It is calculated as follows:

Table 2. Precision and recall of the scripts used in the study

The script accuracy is generally high, and it is safe to say that no strong bias is introduced by the script in the comparison between L1 groups or across proficiency levels.
DATA ANALYSIS AND RESULTS
Our first goal was to identify similarity in the order of accuracy across proficiency and L1 groups. For this comparison we used Spearman’s rank-order correlation; we present the analysis in the section “Similarity in the Accuracy Order within and across L1 Groups.” We then turn to specific differences in the accuracy order within and across L1 group, where we clustered morphemes with similar accuracy; we present the analysis in the section “Specific Differences in the Accuracy Order.” Finally, we quantify L1 influence and its varying strengths on morpheme accuracy through a regression analysis presented in the section “Quantifying L1 Influence and Examining Its Varying Effects across Morphemes.”
Descriptive Data
We begin with the SOC and TLU scores of the target grammatical morphemes, shown in Figure 3. To facilitate graph inspection, we only show SOC and TLU scores between 0.60 and 1.00. The first observation is that the scores are high overall; some groups achieve a TLU score above 0.90 from the KET level on (e.g., past tense -ed in L1 Japanese or plural -s in L1 Spanish). This suggests that we must watch for ceiling effects in the analysis and interpretation of data. The second observation is the striking differences in the TLU scores of individual morphemes among different L1s, despite an overall trend for TLU scores to increase with proficiency. For instance, articles are consistently the least accurate morpheme among L1 Japanese learners but exhibit high accuracy among L1 Spanish learners. By contrast, past tense -ed exhibits high accuracy across proficiency in L1 Japanese but is a low-accuracy morpheme in L1 Spanish learners. This simple graph then suggests differences in the accuracy order among different L1 groups.

Figure 3. Accuracy of each morpheme in each L1 group.
The number of obligatory contexts was small in some cases, leading to unreliable data points (e.g., five in the case of the possessive ’s in L1 Korean KET or nine in the case of the past tense -ed in L1 German KET). These data points were, nevertheless, included in the correlation analysis to allow comparisons with previous morpheme studies that did not always have a large number of obligatory contexts. Data points unreliable due to size were explicitly marked in the clustering approach and were excluded from the regression analysis.
Similarity in the Accuracy Order within and across L1 Groups
Let us begin by looking at how similar the acquisition order is across the different L1 groups, investigating the consistency of the accuracy order within each L1 group across proficiency and the differences among L1 groups. We replicate prior morpheme studies and adopt Spearman’s rank order, a commonly adopted technique for comparing acquisition orders. The Spearman’s rank-order correlation was based on the SOC scores for each L1 and proficiency level. If two orders were significantly correlated, then the groups from which the orders were obtained were assumed to show the same accuracy order. Following Jarvis’s empirical criteria (Jarvis, Reference Jarvis2000), we compared within-L1 orders (i.e., accuracy orders of learners with the same L1 but different proficiency levels) to between-L1 orders (i.e., accuracy orders of different L1 groups). If within-L1 orders are more consistent (i.e., within-L1 pairs show more similarities in the order) than between-L1 orders, then L1 is likely to affect accuracy order.
One accuracy order per L1 group per proficiency level was obtained from the SOC scores. The total number of orders per L1 per proficiency level was 35 (7 L1s × 5 proficiency levels). The total number of possible comparisons between two orders among them was 595 (35C2 =
${{35!} \over {2!\, \times \left( {35 - 2} \right)!}}$
= 595). Of the 595 order pairs, 70 were within-L1 pairs (10 within-L1, between-proficiency pairs × 7 L1s), and the rest (525) were between-L1 pairs. The Spearman’s rank correlation coefficient was calculated for each. The result showed that 269 out of the 525 between-L1 pairs (51.2%) were significantly correlated at p < .05, whereas 57 of 70 within-L1 correlations (81.4%) were significant. The difference between the two (51.2% vs. 81.4%) was statistically significant (χ
2(1) = 22.727, p < .001, φ = 0.195), indicating that within-L1 pairs show more similar accuracy orders than between-L1 pairs. This means that accuracy orders are more similar within L1 groups than between them, satisfying the first two requirements for the identification of L1 transfer by Jarvis (Reference Jarvis2000).
Specific Differences in the Accuracy Order
One weakness of the correlation analysis presented in the preceding section is that small differences in accuracy count as heavily as large differences. The analysis also does not reveal which morpheme differs in order between groups. To address these shortcomings, we clustered together morphemes with similar TLU scores within each L1 proficiency group. Our approach is similar in spirit to that of Krashen (Reference Krashen, Brown, Yorio and Crymes1977) in that it aims to group similar morphemes in one rank in the acquisition order but deviates in the technical implementation. In particular, we defined as “similar” morphemes with TLU scores that do not differ significantly from one another. Thus, similar morphemes were grouped together in one rank, whereas only morphemes with TLU scores reaching statistically significant difference were treated in different ranks in the acquisition order. One complication of testing statistical differences among TLU scores for individual morphemes is that each morpheme in a given proficiency level in an L1 group only has one TLU score. To overcome this difficulty we employed a statistical technique called bootstrapping (Larson-Hall & Herrington, Reference Larson-Hall and Herrington2010; Mooney & Duval, Reference Mooney and Duval1993; Reference Plonsky, Egbert and LaflairPlonsky, Egbert, & Laflair, in press). Bootstrapping is a resampling technique used to gain insights into the population of a given sample. Intuitively, it increases the number of data points by repeatedly drawing random exam scripts and calculating a TLU score from each sample. The following list describes the technique using L1 Japanese CPE data as an example.
-
1. One hundred and sixty-three scripts were randomly selected from the Japanese CPE data. Single scripts could be selected multiple times.
-
2. A TLU score was calculated from the sample obtained in the first step.
-
3. Steps 1 and 2 were repeated 10,000 times, resulting in 10,000 TLU scores.
After bootstrapping, we identified all the morpheme pairs with a statistical difference in the TLU score of the morphemes of the pair. For each pair, the 10,000 TLU scores of one morpheme were subtracted from the 10,000 TLU scores of the other morpheme, resulting in 10,000 differences in the TLU scores between the two morphemes. We then tested whether the 95% range of the differences included zero. If it did, the accuracy difference between the two morphemes was considered nonsignificant, and they were clustered together.
Intuitively, bootstrapping allows us to evaluate if the difference between two TLU scores is due to sampling variability or it is likely to reflect a pattern. To test this, we obtained 10,000 differences from assumedly independent samples and analyzed whether the difference is likely to be non-zero. If the great majority of the differences have the same sign (positive or negative), then the difference in the population is unlikely to be zero.
The orders obtained through clustering morphemes after bootstrapping are shown in Table 3. The morphemes in Cluster 1 marked higher TLU scores than those in Cluster 2, which in turn scored higher than those in Cluster 3, and so forth. For example, at the L1 French PET level, articles received the highest TLU score, followed by three morphemes (past tense -ed, plural -s, and progressive -ing) with similar accuracy levels. These were followed by third-person -s, with possessive ’s as the least accurate morpheme.
Table 3. Clustered order of morphemes based on TLU scores

We clustered in the highest rank all morphemes with TLU scores above 0.90 at p < .05 (marked with asterisks (*)) and any morphemes with lower scores but not statistically significantly lower based on the bootstrapping. The 0.90 threshold has been common in morpheme studies, albeit in those that use SOC scores (Hakuta, Reference Hakuta1976). Crossed-out morphemes are unreliable due to their small sample size (number of obligatory contexts < 100). Underlined morphemes did not show a significant difference with any other morpheme. Such morphemes were clustered together with morphemes of closest accuracy. Table 4 shows the natural order of acquisition (Krashen, Reference Krashen, Brown, Yorio and Crymes1977) limited to the target morphemes of the present study.
Table 4. Clustered natural order of acquisition of English grammatical morphemes

Summary of the Between-L1 Differences
Table 5 summarizes cross-L1 differences in the accuracy order. The L1 groups on the left marked a higher accuracy rank of the morpheme than those on the right. These differences were determined using the following method: If it was certain that a morpheme marked a higher accuracy rank in an L1 group than in another, then the L1 group was placed on the left. For instance, in the case of the FCE level, it was considered that there was no difference in the order of plural -s between the L1 German and L1 Turkish groups because in L1 German the accuracy of plural -s was the first, second, or third from the top, whereas in L1 Turkish it was either the third or fourth. In other words, there is a possibility that in both L1 groups plural -s was the third most accurate morpheme, in which case there is no difference in the order of accuracy. In L1 Spanish or Russian, however, plural -s was either the most or the second most accurate morpheme; thus, there could not be an overlap with its order in the L1 Turkish group. Therefore, the accuracy order of plural -s was considered higher in L1 Spanish and Russian than in L1 Turkish. The KET level was removed from the analysis because the data were mostly too small and were thus unreliable.
Table 5. Between-L1 differences in clustered orders

Note. J = L1 Japanese; K = L1 Korean; S = L1 Spanish; R = L1 Russian; T = L1 Turkish; G = L1 German; and F = L1 French. L1 groups on the left mark higher accuracy ranks on the concerned morpheme than those on the right.
The accuracy order varies across L1 groups with respect to all the concerned morphemes. We can make the following observations for the data shown in Tables 3 and 5:
-
• Articles consistently rank low in Japanese, Korean, Russian, and Turkish learners, a finding that partially confirms the results of Luk and Shirai’s (Reference Luk and Shirai2009) survey while also running counter to the natural order. In the other L1 groups (Spanish, German, and French), articles tend to be in the upper half.
-
• Past tense -ed is consistently in the highest cluster in Korean and Turkish learners, with Japanese, Russian, German, and French learners showing a similar trend. This again does not support the natural order, in which this morpheme is ranked low.
-
• Plural -s tends to appear in higher clusters in Spanish, Russian, and German learners, whereas it tends to be located somewhere in the middle in the other L1 groups.
-
• Possessive ’s tends to mark a lower accuracy rank in Spanish, Turkish, German, and French learners than in Japanese and Korean learners. Overall, however, it typically ranks low and is in this sense consistent with the natural order.
-
• Progressive -ing is in the highest cluster in all L1 groups except for German and French learners. Interestingly, in these two groups it is one of the two morphemes that fail to reach 90% of the TLU score even at the highest proficiency level, CPE.
-
• Third-person -s fluctuates even within L1s over proficiency but tends to stay in the lower half for Spanish learners of English.
Summary of the Within-L1 Differences
We have established that the order of acquisition differs across L1 groups. Let us now consider to what extent it is consistent within L1 groups across the proficiency spectrum. Table 6 shows within-L1 differences in the accuracy order, formatted in the same manner as in Table 5. Few within-L1 differences could be observed in the accuracy order, indicating a consistent order across proficiency levels. There was no within-L1, between-proficiency difference in past tense -ed, plural -s, and possessive ’s, and only one in articles. From these two tables, it is clear that the order of accuracy tends to differ among L1 groups but not within them. The clustering analysis therefore confirms both intragroup homogeneity and intergroup heterogeneity.
Table 6. Within-L1 differences in clustered orders

Note. Cp = CPE; Ca = CAE; F = FCE; and P = PET. Test takers mark higher accuracy order on the exam on the left than that on the right.
Comparison with the Natural Order
To directly analyze whether the idea of the natural order is tenable, the orders in the present data were compared with the natural order, shown in Table 7. As in Table 5, when L1 groups are on the left of the natural order (NO), this indicates that the accuracy rank of the morpheme was higher in the L1 groups than the expectation of the natural order. It is clear that our orders often deviate from the natural order. It is worth noting the absence of difference between the natural order and the order of L1 Spanish learners.
Table 7. Differences between the observed order and the natural order based on clustered data

Note. J = L1 Japanese; K = L1 Korean; R = L1 Russian; T = L1 Turkish; G = L1 German; F = L1 French; and NO = natural order. L1 groups on the left show that they mark higher accuracy order on the morpheme concerned than predicted by the NO. L1 groups on the right show the opposite.
Quantifying L1 Influence and Examining Its Varying Effects across Morphemes
Overview of the Approach
So far we have established that accuracy order varies across L1 groups but is consistent within each group across proficiency. We still need to obtain evidence for Jarvis’s last criterion—crosslinguistic performance congruity—in order to demonstrate L1 influence. More specific questions regarding L1 influence also arise: How strong is L1 influence (Research Question 2)? Does its strength vary across individual morphemes (Research Question 3)? To answer these questions we adopted regression modeling. In particular, we regressed accuracy (TLU score) against L1 influence, L1, exam level, morpheme, and their significant two-way interactions. If L1 influence is significant in determining accuracy, then Jarvis’s crosslinguistic performance congruity criterion is satisfied because it indicates that L1 features are related to L2 use. But how can we capture L1 influence? Jarvis suggests comparing the use of L2 features with that of corresponding L1 features to investigate performance congruity. Due to the range of L1s and morphemes considered here, we take a simpler approach—namely, we consider only presence vs. absence of the L2 feature in the L1 and investigate whether such variation in the L1 can affect accuracy in the L2.
We implemented the idea in the regression model through a dichotomous variable coding the L1 effect as 0 if the morpheme is absent or optional in the L1 and as 1 if it is obligatory. For example, the article in L1 Japanese was coded as 0 because Japanese is generally considered an article-less language. On the other hand, a 1 was assigned to past tense -ed in L1 Japanese because a Japanese morpheme, -ta, roughly corresponds to past tense -ed in English, and it is difficult to express pastness without the use of the morpheme in Japanese. Hence, the past tense marker (-ta) was considered obligatory in Japanese, and a 1 was assigned. Although past tense -ed encodes both tense and aspect, the decision was made on the basis of tense. In the remainder, we refer to this dichotomous variable as L1 type.
Table 8 shows all the values of the variable used in the study, (some of) the corresponding features if the value is 1, and the references that support the decision. Admittedly, this is a rather crude and oversimplified modeling of L1 influence. A corresponding morpheme in the L1, for instance, may have multiple meanings, some of which are not covered by the English morpheme (e.g., the Japanese tei, which also refers to a resultative state; Shirai, Reference Shirai1998b). As we shall see, however, it is a useful way of capturing the effect of the L1.
Table 8. L1 type in target L1s × Morphemes
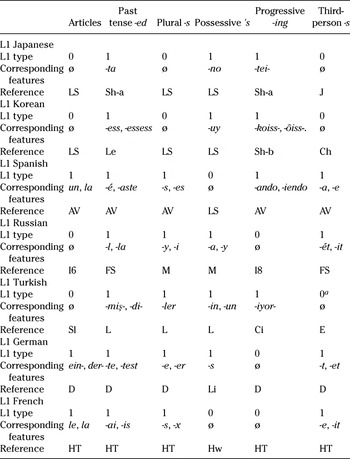
Note. If the morpheme is obligatorily marked in the language, it is given a score of 1; otherwise 0 is given. ø = no corresponding feature. AV = Azoulay & Vicente (Reference Azoulay and Vicente1996); Ch = Choi (Reference Choi2005); Ci = Cinque (Reference Crawley2001); D = Durrell (Reference Durrell2011); E = Ekmekci (Reference Ekmekci1982); FS = Filosofova & Spöring (Reference Filosofova and Spöring2012); HT = R. Hawkins & Towell (Reference Hawkins and Towell2001); Hw = R. Hawkins (Reference Hawkins1981); I6 = Ionin (Reference Ionin2006); I8 = Ionin (Reference Ionin, Haznedar and Gavruseva2008); J = Jelinek (Reference Jelinek1984); L = Lewis (Reference Lewis2000); Le = Lee (Reference Lee2006); Li = Lindauer (Reference Lindauer, Alexiadou and Wilder1998); LS = Luk & Shirai (Reference Luk and Shirai2009); M = Müller (Reference Müller, Arnaudova, Browne, Rivero and Stojanovic2004); Sh-a = Shirai (Reference Shirai1998a); Sh-b = Shirai (Reference Shirai1998b); and Sl = Slobin (Reference Slobin, Gumperz and Levinson1996).
a Turkish verbs inflect for person with the third-person form as the base form. We coded third-person -s as 0 for Turkish, following Ekmekci (Reference Ekmekci1982). The coding of third-person -s, however, makes little difference in our findings because it turns out to be the morpheme that is fairly immune to L1 influence.
Graphical Analysis
Figure 4 contrasts the TLU scores of the morphemes with and without equivalent forms in learners’ L1s. Unreliable data points (number of obligatory contexts < 100) were removed (34/210 = 16.2%) from the subsequent analysis. In addition, the L1 French KET progressive -ing TLU score was excluded from the graphical representation (but was included in the mean calculation and other statistical analyses), as it was much lower than other reliable data (0.415).

Figure 4. Distribution of TLU scores × L1 type.
The data points in Figure 4 were plotted according to three factors: L1 type, exam level, and morpheme. The scores of the morphemes with no equivalent form in the learners’ L1 (hereafter ABSENT) are shown on the left half, whereas the TLU scores of the morphemes with congruent forms in the learners’ L1 (PRESENT) are shown on the right. Solid lines and plus signs (+) show the mean TLU scores aggregated over morphemes, and the dashed line indicates the threshold TLU score (0.90). Standard deviations of the TLU scores of each group are shown inside parentheses below the exam level, with mean TLU scores for PRESENT and ABSENT in square brackets at the bottom of the figure.
First, we observe that the PRESENT morphemes are used more accurately than the ABSENT ones. The difference in the average TLU scores is 0.084, a large value considering that the overall performance is approaching the ceiling. Footnote 7 More strikingly, the (mean) TLU scores of the ABSENT morphemes at the CPE level are only as high as those of the PRESENT morphemes at the KET level, the difference being a mere 0.007. Few ABSENT morphemes scored above 0.90 in TLU.
Second, we observe that morphemes vary in how much they are affected by L1 influence. The emerging picture, summarized in the following list, further confirms the findings of the correlation and clustering analysis while highlighting the differential L1 influence on individual morphemes. Footnote 8
-
• Articles receive low scores in the ABSENT group but above-average scores in the PRESENT group.
-
• Plural -s does not show strong L1 influence until the CAE level, when the difference between ABSENT and PRESENT increases.
-
• Possessive ’s shows weak L1 influence, with scores generally low for both ABSENT and PRESENT groups.
-
• Progressive -ing shows a clear influence from the L1, with scores generally high in both groups.
-
• Third-person -s shows L1 influence at various proficiency levels, but the direction of influence is not consistent across proficiency. The advantage for the PRESENT group only shows up at the advanced levels, whereas it is the ABSENT group that has an advantage at lower levels.
The Logistic Regression Model
To isolate the effect of L1 type from other factors and examine its strength, a logistic regression model was fit to TLU scores.
Model specification and model selection
The number of correct suppliances was entered as the number of successes, and that of errors (i.e., obligatory contexts − correct suppliances + overgeneralization errors) was entered as the number of failures. The predictors included L1Type (two levels: ABSENT or PRESENT), L1 (originally seven levels, one for each L1 group, but see below), ExamLevel (the FCE-centered continuous variable for exam levels), and Morpheme (six levels, one for each target morpheme). The quadratic and cubic terms of ExamLevel were also entered to capture its potentially nonlinear effect. The model further included two-way interactions among the variables. Dummy variables with treatment contrasts were employed for factors. Although two-way interactions were generally put in the model initially, the L1-Morpheme interaction was not entered because (a) it consumes a large number of degrees of freedom and makes the resulting model unstable and (b) the interaction, which tests whether any L1 effect is observed in addition to the effect of L1Type (PRESENT/ABSENT), is not our primary concern. Furthermore, the interactions between L1 and L1Type and those among ExamLevel variables (e.g., the interaction between ExamLevel and ExamLevel Footnote 2 ) were also excluded because their substantial interpretation is difficult.
The interaction terms were reduced in a backward-elimination manner, dropping the one with the highest p value calculated with the Type II sum of squares and comparing the model with and without the interaction by F-tests until the model fit became significantly worse at p < 0.05. With respect to individual L1s, several levels were conflated for statistical parsimony (Crawley, Reference Crawley2007). We performed this step by looking at the estimates of each level, collapsing the two L1 groups with the closest estimates and inspecting whether the resulting model was significantly worse than that without the conflation. The procedure was repeated until the model became significantly worse than the previous model. The model presented subsequently is the one with as few L1 groups as possible without losing fit. Consequently, the L1 Japanese, Korean, and Spanish groups were clustered together, and so were the L1 Turkish and French groups. This deletion procedure was not applied to Morpheme because conflating its levels would make it difficult to interpret the interaction terms it participates in. The reference level of L1Type was the ABSENT group, that of the L1 was the L1 Turkish/L1 French group, and that of Morpheme was articles. Due to overdispersion (residual deviance = 1,463.6 on 120 d.f.), quasibinomial rather than binomial distribution was assumed (Crawley, Reference Crawley2007).
Summary of the model
Table 9 displays the estimates of each parameter and their 95% confidence intervals. Due to space limitations, we focus on the variable of our interest, L1 type. The variable is highly significant: Learners whose L1s have articles (reference-level morpheme) achieved higher accuracy in their use than those whose L1s do not. The significant impact of L1Type on articles is also reflected in the relatively large estimate, the size (1.152) of which is much larger than the difference between the highest and lowest exam levels (CPE − KET = (0.032 × 22 + 0.179 × 2) − (0.032 × (−2)2 + 0.179 × (−2)) = 0.714). Thus, although the effect varies across morphemes, as is demonstrated subsequently, L1 influence can outweigh general proficiency differences in morpheme accuracy. Crucially, this analysis satisfies crosslinguistic performance congruity (Jarvis, Reference Jarvis2000), as it shows an association between the presence or absence of an equivalent form in the L1 (L1 performance) and the accuracy of the morpheme in the L2 (L2 performance).
Table 9. Summary of the logistic regression model fitted to TLU scores (n = 176)
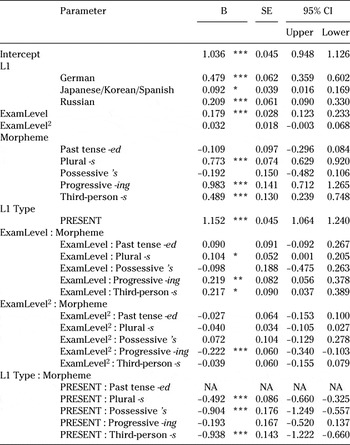
Note. CI = confidence interval; UL = upper limit; and LL = lower limit. Asterisks indicate p value: *** = p < .001; ** = p < .01; * = p < .05; and no asterisks = p < .1.
The varying strength of L1 influence across morphemes
The model also confirms that the strength of L1 influence on different morphemes varies, as the model with the interaction between Morpheme and L1Type had a significantly better fit than that without the interaction term [F(5, 150) = 18.173, p < 0.001]. Judging from the size of the coefficients, L1Type was the strongest in articles (interaction estimate of 0), followed by progressive -ing (−0.193), plural -s (−0.492), possessive ’s (−0.904), and third-person -s (−0.938), which was least affected by the L1. For third-person -s, the accuracy difference between the ABSENT and PRESENT groups became smaller than the difference stemming from one exam level (PRESENT-ABSENT difference = 1.152 − 0.938 = 0.214; one exam level difference = (0.032 − 0.039) × 12 + (0.217 + 0.179) × 1 = 0.389).
DISCUSSION
Early morpheme studies provided evidence for the view that L2 acquisition of English morphemes follows a universal natural order. Building on recent work questioning this specific hypothesis (Luk & Shirai, Reference Luk and Shirai2009) but also motivated by the large body of SLA work that has established strong L1 effects in morpheme acquisition (Ellis, Reference Ellis2006; Ionin & Montrul, Reference Ionin and Montrul2010; Slabakova, Reference Slabakova2014), we revisited the universality of the morpheme acquisition order in L2 English.
A key feature of our empirical investigation was to draw from learner corpus research; in particular, we exploited the Cambridge Learner Corpus. Adopting a learner corpus approach allowed us to address gaps in previous designs primarily related to the richness or scope of the datasets in terms of variety of L1s and proficiency levels covered. The CLC enabled investigation of a set of seven L1s across five proficiency levels. The error and part-of-speech annotation provided the basis for extracting crucial information for the analysis. The richness of our CLC subcorpus also made it possible for us to apply formal statistical analysis to provide rigorous evidence for Jarvis’s criteria for L1 influence. To this end, this study has demonstrated the relevance of large learner corpora for investigating SLA hypotheses, in particular enabling investigation of combinations of variables (L1, proficiency) of considerable scope.
Our core question was whether there is L1 influence on the acquisition order. We sought to answer this question by taking into account Jarvis’s empirical criteria of intragroup homogeneity, intergroup heterogeneity, and crosslinguistic performance congruity.
Using accuracy scores and replicating earlier correlation analyses (Spearman’s correlation), we identified clear L1 effects. Our clustering analysis showed clear between-L1 difference in the accuracy order with respect to all the target morphemes and at the same time the within-L1 stability of the order across proficiency levels, a finding that straightforwardly satisfies Jarvis’s intragroup homogeneity and intergroup heterogeneity. We also found that there was no difference between the accuracy order of Spanish learners of English and the natural order. This supports Luk and Shirai’s (Reference Luk and Shirai2009) hypothesis that the natural order is merely the reflection of the order of acquisition by Spanish learners of English.
To demonstrate L1 influence, intergroup heterogeneity regarding individual morphemes needs to be linked to corresponding morphemes in the L1. To capture this element, we assumed two L1 types, PRESENT and ABSENT, and labeled each individual morpheme in our seven L1 groups according to this crude typology. Our regression modeling established that L1 type is a strong predictor of accuracy, even stronger than proficiency in some morphemes.
A crucial finding is that the absence of the equivalent form in the L1 nearly always leads to an accuracy below 90% in all morphemes. When Tables 3 and 8 are compared, we can see that the morphemes on which learners achieved over 90% accuracy at the CPE level were almost exclusively the morphemes that are present in the learners’ L1s, with the only exception being progressive -ing in L1 Russian. Among the 19 cases of morphemes failing a 90% score at the CPE level, only 5 had equivalent forms in learners’ L1s. These were possessive ’s in L1 Japanese, L1 Korean, L1 Turkish, and L1 German and plural -s in L1 Turkish. Thus, even at the CPE level, it is difficult for learners to achieve 90% accuracy if the relevant morpheme is absent in their L1. However, when their L1 assists them, they have a good chance of success, with the exception of possessive ’s.
Regression modeling allowed us to address a question not previously investigated in morpheme studies—namely, the strength of L1 influence for individual morphemes. Our key finding was that different morphemes are differentially sensitive to L1 influence. The morphemes can be split according to their sensitivity into three groups: (1) the TLU scores of articles and progressive -ing are drastically affected by L1 type (ABSENT/ PRESENT); (2) plural -s is mildly affected by L1 influence; and (3) possessive ’s and third-person -s appear relatively immune to L1 influence.
In sum, our study established strong L1 influence that affects different morphemes in different ways. Despite the dominant view of a universal order, L1 influence is not a surprising result in view of the wide range of L1 effects that have been identified in the acquisition of L2 morphemes since the early morpheme studies. Regarding the effect of L1 type (PRESENT/ABSENT), there is a variety of theoretical proposals addressing the question of L2 acquisition of morphemes absent in the L1 (Ellis & Sagarra, Reference Ellis and Sagarra2010; Lardiere, Reference Lardiere2007; Slabakova, Reference Slabakova2014). However, what has been less discussed in the literature is the differential sensitivity to L1 influence.
Let us reconsider the differential L1 influence we have found in the context of the interpretability hypothesis and Slobin’s distinction between morphemes that encode language-specific concepts and those that encode language-independent cognitive concepts, repeating the caveat that none of these proposals have made explicit predictions for our study case. The apparent immunity of third-person singular agreement to L1 influence seems to go against the interpretability hypothesis’s tenet that morphemes lacking semantic content (uninterpretable) are harder to acquire than ones with semantic content (interpretable). Our data suggest the opposite. At the same time, our findings lend support to Slobin’s approach. It is the two morphemes encoding language-specific concepts like definiteness and progressive aspect—namely, the article and progressive -ing—that are most strongly affected by L1 type. Plural -s, which encodes a universal cognitive concept, is easier to acquire.
Although this approach is plausible, it would need to be developed further, to incorporate more of the linguistic variation in the L1s involved as well as factors like complexity of form-meaning mappings.
For instance, the acquisition of plural marking is dependent on the acquisition of the count-mass distinction, which Slobin does not seem to take into account. Jarvis and Pavlenko (Reference Jarvis and Pavlenko2007)—citing Hiki (Reference Hiki1991) and Yoon (Reference Yoon1993)—claim that the speakers of classifier languages such as Japanese perceive noun countability differently from the speakers of noun class languages, an aspect that can lead to potential L1 influence on the acquisition of plurality marking (see also Butler, Reference Butler2002). Crucially, the count-mass distinction is a language-specific distinction that may moderate the acquisition of mapping a general cognitive concept such as plurality to its morphological realization.
The complexity of the mappings is another dimension that can potentially explain our findings. As pointed out by one anonymous reviewer, there are more distinctions in definiteness or aspect than in plurality, thus making the form-meaning mappings for the article and -ing more complex than form-meaning mappings for plurality. Exploring this dimension takes us beyond the scope of the present study, as it would require additional empirical evidence to reach definitive conclusions. In view of the clear L1 influence found in this study, it seems to us, though, that the inherent complexity of individual morphemes is an orthogonal and additional dimension to L1 type.
The two morphemes least sensitive to L1 influence are possessive ’s and third-person -s. Possession is linguistically marked even in the L1s of the ABSENT group, typically by the form of postnominal modification. This means that even the learners in the ABSENT group can rely on a language-independent concept of possession. As for third-person -s, although the L1s in the ABSENT group do not have a corresponding morpheme, the morpheme is immune to L1 influence because most languages have lexical items referring to the first, second, and third person, which familiarizes both ABSENT and PRESENT learners with the concept, and some languages even encode person distinctions in their grammatical systems. This may facilitate the acquisition of this feature in an indirect way. The latter, for instance, is the case for Turkish, which does not have a corresponding morpheme but nevertheless does have grammatical person distinctions. If this line of thinking is correct, it would suggest that L1 influence is restricted not to corresponding morphemes but to more general aspects of the L1 that may not necessarily surface in the morphemes in question.
One limitation of this study, inherited from previous morpheme studies, is that it investigates the accuracy of morphemes but not their acquisition (at least not directly). However, the fact that there were few within-L1 differences in the accuracy order indicates that the order does not change along the developmental path. Therefore, no matter what criterion is used for acquisition, it is unlikely that the accuracy order observed in this study will be far different from the acquisition order.
Another limitation is that the data used in the study consisted of exam scripts; as a result, learners might have been form focused in their writings and might have exhibited different orders than if they were meaning focused. The present study, however, is generally in line with findings from previous studies, which were conducted in a more naturalistic environment. A case in point is the uniformity between the natural order and the accuracy order of L1 Spanish learners. As Luk and Shirai (Reference Luk and Shirai2009) show, the similarity between the natural order and the acquisition order of L1 Spanish learners can be taken as evidence of the resemblance between the accuracy orders observed under exam situations and those in more naturalistic settings.
CONCLUSION
L1 influence is pervasive in all areas of L2 acquisition (Jarvis & Pavlenko, Reference Jarvis and Pavlenko2007; Odlin, Reference Odlin1989). Despite the numerous claims for the “natural” order of L2 acquisition of English grammatical morphemes, the present study has demonstrated that they are not an exception to L1 influence. A correlation analysis based on SOC scores indicated crosslinguistic influence on the order by comparing within-L1 correlations and between-L1 correlations. Clustering of morphemes based on TLU scores directly falsified the concept of a universal accuracy order of morphemes across L1s. Regression analysis illustrated that the morphemes with equivalent forms in the L1 mark higher accuracy. These analyses provided strong evidence that the accuracy order is influenced by the L1. The L1 influence manifests in two ways: If a morpheme is absent from the L1, accuracy is generally lower than when the morpheme is present in the L1. This influence interacts with morphemes. The methodological approach we adopted allowed us to quantify the relative sensitivity of individual morphemes to L1 influence. We found that morphemes encoding language-specific concepts (e.g., definiteness) are more severely affected by the L1 than morphemes encoding more universal concepts. These findings suggest that acquiring a new concept is much harder than acquiring a new mapping of a concept to a form. These results not only demonstrate the influence of the L1 on morpheme acquisition but also indicate that it can be due to different sources (e.g., absence of the morpheme, acquisition of a new concept, or new concept-to-form mapping). Large corpora allow us to investigate the different interactions and gain deeper insights.
APPENDIX
IDENTIFICATION OF ERRORS AND OBLIGATORY CONTEXTS
The following discussion illustrates how we identified the errors and obligatory contexts of morphemes in the CLC, using past tense -ed as an example. The CLC error coding offers a rich set of error tags with the following format: <NS type=“(error classification)”>erroneous form|correct form</NS> (e.g., She was one of the five daughters of a farmer who<NS type=“TV”>live|lived</NS>in a small village.). An error was counted as a non-overgeneralization error if all of the following four conditions were satisfied: (a) The error classification was either IV (incorrect verb inflection) or TV (incorrect verb tense). (b) Neither the erroneous form nor the correct form included be verbs, modals, or have and their inflected forms. This excluded voice errors, errors related to modal verbs, and aspectual errors. (c) The erroneous form did not include a word ending in -ed. This excluded voice errors, errors related to modal verbs, and aspectual errors. (d) The two words immediately preceding the error tag did not include be verbs, have, get, make, let, and their inflected forms. This excluded passive voice and participial use linked to causative verbs.
Similarly, an error was counted as an overgeneralization error if the following five conditions were met: (a) The error classification was IV, TV, or FV (wrong verb form). We added FV here because overgeneralization errors were occasionally classified as FV. (b) The correct form did not include a word ending in -ed. This excluded omission errors. (c) The erroneous form did not include have and its inflected forms. This excluded aspectual errors. (d) The correct form did not include be verbs, which excluded voice errors. (e) The two words preceding the error tag did not include be verbs, have, get, make, let, and their inflected forms. This excluded participial use of -ed, including passive voice.
Finally, obligatory contexts were identified in the following way: The corrected texts have the format of |(word+morpheme):(word number starting at 1 at the beginning of the sentence)_(POS tag)| (e.g., |we:5_PPIS2| |tend+ed:6_VVD| |to:7_TO| |respect:8_VV0| |some:9_DD| |particular:10_JJ| |job+s:11_NN2|). Obligatory contexts of past tense -ed were identified as those words that have the VVD tag (past tense form of lexical verbs) and have the word form of (word)+ed, where (word) is a regular verb.