Introduction
Over the past few decades, rapid developments in agriculture have highlighted the need to convert from traditional farming to more environmentally friendly practices. During this transition, organic agricultural production has been proliferating worldwide. According to the Research Institute of Organic Agriculture (FiBL)Footnote 1 statistics, the area of organic farmland worldwide has increased from 58.0 million hectares in 2016 to 74.9 million hectares in 2020Footnote 2. This increase has caused the ratio of organic arable land to total agricultural land to increase from 1.0% in 2015 to 1.5% in 2019. Within 10 years (2009–2019), Asian countries have the second-highest growth rate of organic agricultural land in the world (approximately 140.5%). Up to 51% of organic agricultural producers came from Asian countries in 2019. The above rapid transformation has shown the need to shift from traditional to organic agriculture.
Substantial evidence has shown that organic agriculture benefits humans and the environment (Roitner-Schobesberger et al., Reference Roitner-Schobesberger, Darnhofer, Somsook and Vogl2008; Suthar et al., Reference Suthar, Ma, Thomas, Lund, Zhang, Daffis and Gale2010; Karipidis and Karypidou, Reference Karipidis and Karypidou2021). From an economic benefit perspective, organic farming helps farmers increase their income more than traditional one. According to Doanh et al. (Reference Doanh, Thuong and Heo2018), households converting to organic farming have higher incomes than those following traditional practices. Specifically, organic tea adopters earned a higher income than non-adopters, from 1038.8 to 1059.0 thousand Vietnamese Dong per hectare of cultivation plot. Similarly, Qiao et al. (Reference Qiao, Halberg, Vaheesan and Scott2015) concluded that the price premium farmers received from organic tea compensates for higher labor input and lower yield, resulting in a net profit. Besides, this farming practice contributes to higher biodiversity (Haldar and Damodaran, Reference Haldar and Damodaran2021), protecting farmers from economic disasters caused by crop damage or falling prices.
Regarding non-economic benefits, organic farming produces a smaller carbon footprint (Knudsen et al., Reference Knudsen, Meyer-Aurich, Olesen, Chirinda and Hermansen2014; Feng and Zhao, Reference Feng and Zhao2020), reduces soil erosion (Arnhold et al., Reference Arnhold, Lindner, Lee, Martin, Kettering, Nguyen, Koellner, Ok and Huwe2014; Seitz et al., Reference Seitz, Goebes, Puerta, Pereira, Wittwer, Six and Scholten2018), lowers greenhouse gas emissions (Horrillo et al., Reference Horrillo, Gaspar and Escribano2020) and minimizes global nitrogen pollution (Cattell Noll et al., Reference Cattell Noll, Leach, Seufert, Galloway, Atwell, Erisman and Shade2020). These factors preserve healthy soils and increase sustainability (Seufert and Ramankutty, Reference Seufert and Ramankutty2017; Tscharntke et al., Reference Tscharntke, Grass, Wanger, Westphal and Batary2021). Because no harmful chemicals are used (Lairon, Reference Lairon2010; Seufert and Ramankutty, Reference Seufert and Ramankutty2017), this farming method provides safe working conditions for farmers (Ahmed et al., Reference Ahmed, Thompson and Glaser2018), enhances biodiversity (Aivazidou and Tsolakis, Reference Aivazidou and Tsolakis2021) and improves human health (Mie et al., Reference Mie, Andersen, Gunnarsson, Kahl, Kesse-Guyot, Rembialkowska and Grandjean2017; Yanakittkul and Aungvaravong, Reference Yanakittkul and Aungvaravong2020), including producers, their families and neighboring residents.
Recognizing the importance of organic agriculture, economic scholars have attempted to identify many factors influencing farmers' decisions to convert from conventional to organic farming. They include farmers' demographic characteristics (Swenson et al., Reference Swenson, Ketron, Akonde, Yeboah, Owu, Slaughter and Vallabhaneni2021), market aspects (Sutherland, Reference Sutherland2013), price (Sutherland and Darnhofer, Reference Sutherland and Darnhofer2012; Jouzi et al., Reference Jouzi, Azadi, Taheri, Zarafshani, Gebrehiwot, Van Passel and Lebailly2017), policy-related factors (Kuminoff and Wossink, Reference Kuminoff and Wossink2010; Sutherland and Darnhofer, Reference Sutherland and Darnhofer2012), knowledge transfer (Sutherland and Darnhofer, Reference Sutherland and Darnhofer2012), available technology, peer networks, willingness to preserve the environment, observable economic benefit and health consciousness (Karki et al., Reference Karki, Schleenbecker and Hamm2011), the use of agriculture information systems (Doanh et al., Reference Doanh, Quynh and Pham2022b) and psycho-behavioral and psychosocial factors (Sapbamrer and Thammachai, Reference Sapbamrer and Thammachai2021)Footnote 3.
The above factors play an important role in proposing policies to promote the transition from traditional to organic agriculture. However, we think neighborhood effects (NE) are also crucial to the farmer's converting intention. The inclusion of the NE is justified on empirical and practical grounds. From the empirical perspective, Wollni and Andersson (Reference Wollni and Andersson2014) found that farmers who believe in acting in accordance with their neighbors' expectations and with greater availability of information in their neighborhood network are more likely to adopt organic agriculture. Similarly, Schmidtner et al. (Reference Schmidtner, Lippert, Engler, Häring, Aurbacher and Dabbert2012) confirmed the importance of agglomeration effects in the organic farming sector. From a practical point of view, people living in these areas, especially ethnic minorities, worship the culture and beliefs of the community (Nam, Reference Nam2010). A community relationship is a dominant relationship in society. Communalism emphasizes and governs the processes of cultural creation, dissemination and consumptionFootnote 4.
The idea of herd behavior was introduced by Banerjee (Reference Banerjee1992), whereby an individual tends to do what other individuals are doing, even when his or her private information suggests doing something quite different. Manski (Reference Manski1993) gave a similar definition, according to which an individual's behavior could change according to the behavior of the social group, also known as neighborhood effects (NE). This effect can be explained through the theory of herd behavior (HB). The individual decides to follow others and imitate group behaviors rather than deciding independently based on his or her own information (Baddeley, Reference Baddeley2010). According to Sun (Reference Sun2013), HB can occur when the individual is uncertain about his or her decision. In this case, individuals will imitate the group's behavior because they think it is the safest behavior.
To the best of our knowledge, the studies by(Bjørkhaug and Blekesaune (Reference Bjørkhaug and Blekesaune2013), Lapple and Kelley (Reference Lapple and Kelley2014), Wollni and Andersson (Reference Wollni and Andersson2014) and Allaire et al. (Reference Allaire, Poméon, Maigné, Cahuzac, Simioni and Desjeux2015) are a few related to this field. These studies form the foundation for estimating NE and spatial dependence on organic adoption. However, as stated by Lapple and Kelley (Reference Lapple and Kelley2014), a potential limitation is related to the dynamic effects. For example, pioneering organic farmers have a greater influence on the diffusion of organic farming than later adopters. Besides, spatial dependence does not fully reflect the influence of NE. For instance, the members of a social group significantly influence the subject's decisions even though these members do not live near the subject. Given the knowledge gap in the existing literature, this study aims to analyze the influence of NE on tea farmers' intention to convert from traditional to organic farming in the mountainous areas of northern Vietnam.
The novelty of this study is reflected in two main aspects. First, we expand the theory of planned behavior (TPB) by incorporating the theory of HB to explain the impacts of NE on tea farmers' converting intentions. Although the TPB is appropriate for studying organic converting behavior (Yanakittkul and Aungvaravong, Reference Yanakittkul and Aungvaravong2020), Hong et al. (Reference Hong, Chan, Thong, Chasalow and Dhillon2014) recommended that research studies incorporate novel constructs during the contextualization of a given theory. Secondly, previous studies measured NE or HB through geographic distance or surveys (Shen et al., Reference Shen, Zhang and Zhao2016). In this study, to overcome the limitations of Lapple and Kelley (Reference Lapple and Kelley2014), we use a combination of questionnaires and the method of measuring HB employed by McCartney and Shah (Reference McCartney and Shah2022). Besides using the questionnaire adopted in previous studies, we measure the NE across three cases: (1) the converting status of farming households living close to the subject under study (the individual) within a radius of 500 m; (2) the converting status of farming households that have close relationships (relatives, close friends) with the subject under study (the individual) and (3) the converting status of farming households living close to the subject under study (the individual) within a radius of 500 m and having close relationships (relatives, close friends) with the subject under study (the individual). The simultaneous application of two measurement methods makes our research results more robust.
Theories and hypotheses
The theory of planned behavior
Most previous related research has been carried out in the framework of the TPB (Yanakittkul and Aungvaravong, Reference Yanakittkul and Aungvaravong2020). Several later studies extended the TPB and combined it with the diffusion of innovation theory (Doanh et al., Reference Doanh, Quynh and Pham2022b) and the norm activation model (Nguyen et al., Reference Nguyen, Doan, Nguyen and Nguyen2021). Proposed by Ajzen (Reference Ajzen1991), TPB explains behavioral intention through three factors, including attitude (ATT), subjective norm (SN) and perceived behavior control (PBC). Accordingly, ATT reflects the degree to which a person rates a behavior favorably or unfavorably. The more favorable the individual's assessment is, the more likely he or she is to perform the behavior (Borriello et al., Reference Borriello, Massey and Rose2022). Many studies have confirmed the positive influence of ATT on intention. For example, Yanakittkul and Aungvaravong (Reference Yanakittkul and Aungvaravong2020) found that ATT promotes farmers' intention to grow organic rice. Likewise, Lapple and Kelley (Reference Lapple and Kelley2014) also documented that ATT influences Irish farmers' intention to convert from traditional to organic farming. Based on the above evidence, we hypothesize that the better the farmer's assessment of the conversion to organic farming, the more likely he or she is to convert from traditional to organic farming.
H1: ATT positively affects INTFootnote 5.
SN describes an individual's perception of external pressures (e.g., pressure from society, relatives and neighbors) when performing a particular behavior (Issa and Hamm, Reference Issa and Hamm2017). According to Yanakittkul and Aungvaravong (Reference Yanakittkul and Aungvaravong2020), the more favorable an individual's perception of pressure is, the more likely he or she will carry out his or her intention. Therefore, the higher the SN, the more farmers intend to convert from traditional farming to organic farming. Nguyen et al. (Reference Nguyen, Doan, Nguyen and Nguyen2021) showed that SN positively influences farmers' intention toward organic farming. A positive correlation between SN and intention was also found in the study of Govindharaj et al. (Reference Govindharaj, Gowda, Sendhil, Adak, Raghu, Patil and Damalas2021), who showed that SN plays a vital role in shaping farmers' behavior. Therefore, this study hypothesizes that the higher the SN, the more farmers intend to convert from traditional farming to organic farming.
H2: SN positively affects INT.
The PBC reflects the perceived difficulty of performing a particular behavior (Ajzen and Madden, Reference Ajzen and Madden1986). According to Shukri et al. (Reference Shukri, Jones and Conner2022), PBC is a multi-structural factor that consists of two main parts: perception of ease (e.g., the individual's perception of how difficult or easy it is when he or she performs the intention) and self-efficacy (e.g., the perception of the degree of confidence when he or she implements the intention). Yanakittkul and Aungvaravong (Reference Yanakittkul and Aungvaravong2020) indicated that PBC plays a vital role in shaping farmers' organic conversion. The positive impact of PBC on intention was also found in the study of Jones et al. (Reference Jones, Sok, Tranter, Blanco-Penedo, Fall, Fourichon and Sundrum2016) and Yanakittkul and Aungvaravong (Reference Yanakittkul and Aungvaravong2020). Therefore, this study hypothesizes that the higher the PBC, the more farmers intend to convert from traditional to organic farming.
H3: PBC positively affects INT.
The theory of HB and the NE
Herd behavior can be defined as the phenomenon in which the individual decides to follow others and imitate the group's behaviors rather than deciding independently based on his or her own information (Baddeley, Reference Baddeley2010). In society, philosophers consider HB the practice of individuals mimicking the behavior of crowds because they consider it the safest behavior. This imitation is reflexive and does not require the intervention of reason (Sun, Reference Sun2013).
There are two main conditions under which HB can occur: uncertainty about the decision and observing others' actions (Sun, Reference Sun2013; Youssef, Reference Youssef2020). In terms of the first condition, people tend to follow the crowd's behavior when uncertain about the decision they will make due to a lack of information or asymmetric information (Pavlović-Höck, Reference Pavlović-Höck2022). Regarding the second condition, observing many people making the same decisions is also a condition that motivates individuals to perform HB. Here, there are two important factors involved in influencing such observations. The first factor is the number of people who have performed the behavior before. According to Sun (Reference Sun2013), the number of people who have performed a specific behavior plays an essential role in forming the intention and behavior of the individual. Accordingly, the individual will tend to perform any behavior if many others have performed the same behavior before. The second factor is the identity of those who performed the previous act. An individual is likely to perform the same behavior as his predecessor if he considers his predecessor to be successful (Bandura, Reference Bandura1986) or reputable (Abrahamson, Reference Abrahamson1991).
Thus, the NE may affect tea farmers' intention to convert to organic practice. Specifically, the traditional tea farmer (the individual) observes other people (other farmers) who have converted to organic tea production. Based on these observations, traditional tea farmers decide to convert to organic tea production even if the information obtained suggests that they should not convert to organic tea production. In other words, if more neighbors and people who have a close relationship with traditional tea farmers have converted to organic tea production, the more traditional tea farmers intend to convert to organic tea production.
Here it is essential to distinguish SN and NE because they have similarities and differences. SN and NE are similar in that they both refer to the influence of intimate relationships (such as family, neighbors and close friends) on the subject's intentions. However, SN and NE differ in the following points. First, SN focuses on the influence of the thoughts of intimate people on the subject's intentions (e.g., my closest family members think that I should develop organic farming, my closest friends think that I should develop organic farming and people who are important to me think that I should develop organic farming). In this case, the subject's intentions appear to be passive. In contrast, in the case of NE, the subject's intention comes from the subject's self-observation of its intimate people. In this case, the subject's intention seems proactive (the subject actively observes and follows its intimate people). Secondly, in the case of SN, the subjects' intimate people are not necessarily those who have converted to organic tea or have succeeded in organic tea farming. In contrast, in the case of NE, the subjects' intimate people are those who have intended or have successfully converted from traditional to organic tea farming.
H4: NE positively affects INT.
Several previous studies have shown that individuals can change their beliefs by observing the actions of others. Accordingly, individuals can ignore their initial beliefs and form new beliefs about usefulness after observing the actions of their predecessors (Sun, Reference Sun2013). Basically, all three factors (ATT, SN and PBC) are formed based on the perception and beliefs of the individual (Issa and Hamm, Reference Issa and Hamm2017; Romero-Colmenares and Reyes-Rodríguez, Reference Romero-Colmenares and Reyes-Rodríguez2022). Therefore, this study hypothesizes that the NE can positively affect ATT, SN and PBC.
H5: NE positively affects ATT.
H6: NE positively affects SN.
H7: NE positively affects PBC.
Besides such factors as ATT, SN, PBC and NE, we also incorporate the demographic characteristics of tea farmers, including gender, age, education level and culture.
Methodology
Model of estimation
According to Figure 1, our research model consists of two main structures. The first structure is the direct impact of ATT, SN, PBC and NE on INT. The second structure is the indirect impact of NE on INT through ATT, SN and PBC. Previous studies often used structural equation modeling (SEM) in this case. The strength of this model is its powerful flexibility to jointly test a variety of hypotheses that involve different types of complicated cause–effect relationships (Katebi et al., Reference Katebi, Homami and Najmeddin2022). However, the SEM's assumption (all responses are continuous) exposes users to severe biases, especially if the dependent variable is a dummy variable (Hieu et al., Reference Hieu, Van and Doanh2021). Therefore, following Hieu et al. (Reference Hieu, Van and Doanh2021) and Katebi et al. (Reference Katebi, Homami and Najmeddin2022), we apply generalized SEM (GSEM), which is a combination of the generalized linear model and SEM (Zhang and Zhang, Reference Zhang and Zhang2018). Combining the two models makes it possible to handle multiple structures with different types of response variables (ATT, SN and PBC are continuous response variables, while INT is a non-continuous response variable). Basically, the GSEM model has the following form:


where y is the dependent variable, X is the explanatory variable(s), β is the coefficient of X, g(.) is called the link and F is called the family.

Fig. 1. Factors affecting farmers' intention to convert to organic tea farming.
Regarding the first structure, we will use the Bernoulli family with a logit link function to evaluate the direct impact of ATT, SN, PBC and NE on INT. Specifically, the mathematical equation is as follows:
Bernoulli family:

and Logit link:


where INT takes the value of 1 if the farmer intends to convert to organic farming and 0 if he or she does not. μ is the probability that a farmer intends to convert from traditional to organic farming.
Our second structural model estimates the impact of NE on ATT, SN and PBC. We use the Gaussian family with an identity link for this structure. Specifically, the mathematical equations are as follows:
Gaussian family and identity link of response are:
ATT

SN

PBC

where μ ATT, μ SN and μ PBC are the means of ATT, SN and PBC, respectively. d ATT, d SN and d PBC are the dimensions of the observed response vectors ATT, SN and PBC, respectively. Σ indicates the variance matrix of unexplained errors. In which, ΣATT is the variance matrix of ATT's unexplained errors, ΣSN is the variance matrix of SN's unexplained errors and ΣPBC is the variance matrix of PBC's unexplained errors.
Following Hieu et al. (Reference Hieu, Van and Doanh2021) and Katebi et al. (Reference Katebi, Homami and Najmeddin2022), we use Akaike's information criterion (AIC) and the Bayesian information criterion (BIC) to confirm the effectiveness of the GSEM. Two indexes are calculated as follows:


where L is the likelihood function, p is the number of parameters and n is the size of the sample.
In summary, we take the following steps to estimate the impact of variables on farmers' intention to convert to organic farming. First, following Doanh et al. (Reference Doanh, Do Dinh and Quynh2022a), we use exploratory factor analysis (EFA) to check the factor structure and confirmatory factor analysis (CFA) to check the reliability, validity and measurement model fit. Here, we use the goodness of fit (GOF) indices to check the efficiency of the model, including model fit chi-squared test (χ2), comparative fit index (CFI), Tucker–Lewis index (TLI) and root mean squared error of approximation (RMSEA). Secondly, we use STATA-17 software to simultaneously estimate the structures in the model (see Fig. 1) with the GSEM command. GSEM estimates the impact of control variables on INT, and hypotheses H1, H2, H3 and H4 with Bernoulli family and logit link; and hypotheses H5, H6 and H7 with Gaussian family with an identity link.
Variables and measurement
Under the framework of the TPB, farmers' intention to convert from traditional to organic farming was measured by the question: ‘Do you want to convert to organic farming?’ (1 = I want to convert; 0 = I don't want to convert to organic farming). This study measures the structures of the TPB based on the work of Hieu et al. (Reference Hieu, Van and Doanh2021) and Solesvik (Reference Solesvik2013). ATT is measured through five questions, SN is measured through six questions and PBC is measured through four questions. Details of items are given in Table A1Footnote 6. Items are accompanied by a response option ranging from 1 (strongly disagree) to 5 (strongly agree). Higher scores reflect higher levels in the measured constructs.
Concerning NE, this study measures it in two ways, including (1) using a 6-item questionnaire (Shen et al., Reference Shen, Zhang and Zhao2016) and (2) measuring by cases. According to Manski (Reference Manski1993) and McCartney and Shah (Reference McCartney and Shah2022), there are two problems in measuring NE: endogenous and correlated. In this study, these two problems stem from the following reasons. First, farming households often choose to live and participate in activities near households with similar lifestyles, incomes or bloodlines. Because of these similarities, they may have made the same decision to convert to organic farming. Secondly, even though they do not live close to each other, farmers can still communicate with their relatives (e.g., blood relatives and friends) in other areas, thanks to the development of technology. Therefore, they may face similar farming problems.
Based on the study by McCartney and Shah (Reference McCartney and Shah2022), we randomly selected farm households adjacent to the subject under study to solve the above problem. In addition, this study determines the NE across three cases, including:
(1) The converting status of farming households living close to the subject under study (the individual) within a radius of 500 m;
(2) The converting status of farming households that have close relationships (relatives, close friends) with the subject under study (the individual);
(3) The converting status of farming households living close to the subject under study (the individual) within a radius of 500 m and having close relationships (relatives, close friends) with the subject under study (the individual).
Data collection
Our data are collected through questionnaires and provided by local authorities in Thai Nguyen province, Vietnam (see Fig. 2). We chose this place because it is known as the tea land of Vietnam. According to the General Statistics Office of Vietnam, in 2020, the total tea growing area in this area was 22,399 hectares, of which the harvested area was 19,754 hectares (reaching 88.19%). Currently, Thai Nguyen province supports and encourages farmers to convert to organic tea farming. In 2020, Thai Nguyen province implemented an organic tea production model according to Vietnamese standards on a scale of 60 hectares with 186 households (Thanh, Reference Thanh2020).
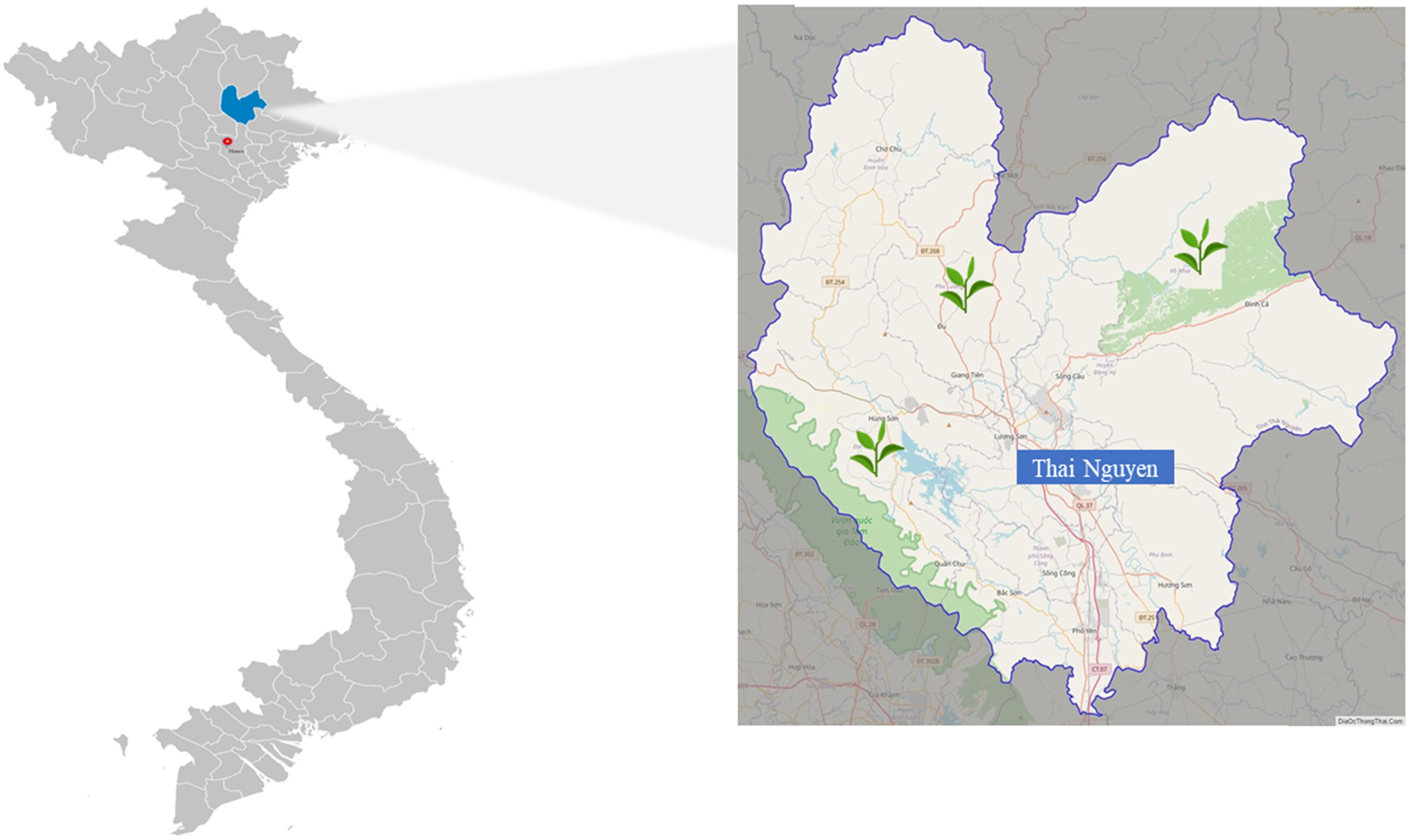
Fig. 2. Study location.
Basically, after completing the process of organic tea cultivation, the yield of organic tea equals 75–85% when compared with traditional tea (approximately 12.2 tons per ha)Footnote 7. However, when growing organic tea, farmers need 2–3 years to improve the soil and plant trees. As a result, in the early years of conversion, the yield of organic tea decreases (equivalent to 60% of that of traditional tea). This problem makes farmers afraid to convert to organic tea farming. Some other farmers have converted to organic tea production but abandoned it due to concerns about the economic development potential of this farming method.
From the list of farming households provided by the local government, we randomly selected 300 households to participate in the survey. The only requirement for survey participants is that the farmer is growing tea using traditional methods. To ensure that all data collected from farmers was accurate and unbiased, we selected interviewers from another locality. Before conducting the interviews, the interviewers were briefed on the survey content, equipped with data collection skills and gained informed consent from the interviewees. In addition, following Tseng et al. (Reference Tseng, Pham, Cheng and Teng2018), we test farmers' attention during the interview process by using one item and asking them to check the ‘very disagreeable’ response option. If farmers are not focused, they will skip this question, and their answer sheets will be discarded. At the end of the interview, 263 farmers met our criteria. In total, 21 respondents were not eligible because they did not provide enough information, and 16 respondents did not pass the attention checking.
The demographic characteristics of 263 farming households participating in the study are presented in Table 1. In total, 106 farmers intend to convert to organic farming (40.30%), and 157 do not intend to convert to organic farming (59.70%). We noticed a few similarities between the converting and non-converting groups. The majority of farmers in both groups are from the Kinh ethnic group, have a high school education and have 5 up to 10 years of tea growing experience. Besides, there are a few differences between these two groups of farmers. Most of the farmers interviewed were male and aged 36 up to 45, which implies that farmers who intended to convert are younger than those who did not intend to convert. The majority of farmers in the group intending to convert are 36 up to 45 years old (accounting for 53.77%), while the age group in the non-converting group only accounts for about 38.85%.
Table 1. Summary of statistics

Note: Switcher means the group of farmers who intend to switch from traditional to organic farming. Non-switcher indicates the group of farmers who do not intend to switch from traditional to organic farming.
Empirical results
Psychometric properties
According to the results of EFA in Table A2, the factor loadings of items in each factor are higher than 0.720. It implies that the structure of the factors is appropriate for this study. The results of CFA are presented in Table A3. Items evaluated for each construct have composite reliability values exceeding 0.921 and average variance extracted values exceeding 0.693. The entire results indicate adequate reliability. In addition, the indices of GOF, including CFI = 0.960, TLI = 0.947 and RMSEA = 0.075, imply that our data show a very good fit to the hypothetical model, and all the constructs can be used for further analysis.
The impacts of HB on farmers' converting intention
This study uses GSEM to analyze the structural relationship between the factors of the TPB and the NE on tea farmers' intention to convert from traditional to organic farming. In terms of control variables, the estimated coefficients of age and gender show a negative effect on INT but are insignificant. In contrast, the estimated coefficients of education and experience show a positive and statistically significant effect on INT. Meanwhile, culture is a factor that has a negative effect on INT but is not statistically significant. Our research results agree with Doanh et al. (Reference Doanh, Quynh and Pham2022b), who indicate that young, highly educated and experienced farmers tend to convert to organic farming.
In terms of TPB, all three structural variables in TPB impact farmers' converting intention positively. Specifically, the estimated coefficient of ATT (β ATT = 0.612*) show a positiveeffect on INT and is statistically significant at 0.05, supporting H1. SN (β SN = 0.712**) and PBC (β PBC = 0.786**) have positive and statistically significant effects on INT, supporting H2 and H3, respectively (see Fig. 3 and Table 2).

Fig. 3. Impact of HB on farmers' intention to convert to organic tea farming.
Table 2. NE and farmer's switching intention

Regarding the NE, NE was found to have a direct positive effect on INT but was not statistically significant. However, NE has a positive and statistically significant indirect effect on INT through ATT, SN and PBC. According to our estimation, a 1 one-unit increase in NE leads to an increase of 0.736, 0.633 and 0.404 units in ATT, SN and PBC, respectively. These results support H5, H6 and H7.
To ascertain the impact of the NE on tea farmers' intention to convert from conventional to organic farming, we performed a three-case assessment: the effects of the neighborhood (Nei), relatives (Rel) and neighborhood and relatives (Nei&Rel). The estimated results are displayed in Table 3.
Table 3. Impact of the NE on farmers' switching intention according to three groups of the neighborhood (Nei), relatives (Rel) and neighborhood and relatives (Nei&Rel)

Regarding direct effects, Nei (β Nei = 0.132) and Rel (β Rel = 0.299) positively affect INT but are not statistically significant. Meanwhile, the Nei&Rel (β Nei&Rel = 1090*) positively affects INT and is statistically significant at 0.05.
Regarding indirect effects, all three effects, Nei, Rel and Nei&Rel, have an indirect and statistically significant positive impact on INT through ATT, SN and PBC.
In short, empirical results indicate that the factors of TPB (ATT, SN and PBC) positively and significantly affect farmers' intention to switch to organic tea farming. Among these factors, PBC has the most impact on INT, followed by SN and ATT. These findings imply that farmers' perceptions play an important role in shaping their conversion intentions. Besides, the direct and indirect effects of NE on tea farmers' intention to convert from traditional to organic farming confirm that our hypothesis is grounded and consistent with reality.
Discussion
Besides using control variables, we combine the TPB and HB to explain tea farmers' intention to convert from traditional to organic farming. Regarding TPB factors, the positive impact of ATT on INT shows that farmers' perception of the benefits of organic farming positively affects their converting intention. Accordingly, the higher the farmer's assessment of the economic and non-economic benefits of organic farming, the more confident they are in the success of this farming method. Our argument concurs with Yanakittkul and Aungvaravong (Reference Yanakittkul and Aungvaravong2020), who indicate that the better the farmer's assessment of the conversion to organic farming, the more likely they are to convert from conventional to organic farming. The results also show that SN positively affect farmers' intention to convert to organic farming. The results of this study are compatible with Tama et al. (Reference Tama, Ying, Yu, Hoque, Adnan and Sarker2021). They suggested that SN positively impacts the intention of farmers to continue Conservation Agriculture in the northern part of Bangladesh. They argue that the closer an individual is to his neighbors, the more influence these neighbors have on the individual's decision to perform the behavior. In other words, a farmer may intend to convert to organic farming if their family, friends and neighbors think the farmer should do so. In line with the research of Läpple and Kelley (Reference Läpple and Kelley2013) about the decisions of Irish farmers to convert to organic farming, our result shows that PBC positively affects farmers' converting intentions. Accordingly, the higher farmers' awareness of the ease, convenience and capacity of their farming, the more confident they will decide to convert from traditional to organic farming.
The estimation of the influence of the NE on tea farmers' intention to convert from traditional to organic farming in Tables 2 and 3 suggests two issues. First, the NE directly affects a farmer’s converting intention when these neighbors are both neighbors and relatives of the farmer and have converted to organic farming. The results of our study have a slight difference compared to the study of Lapple and Kelley (Reference Lapple and Kelley2014), who show that the NE has a direct and statistically significant impact on farmers' converting intention. Our results support the views of Sun (Reference Sun2013) and Abrahamson (Reference Abrahamson1991), who show that individuals tend to follow the behavior of other people they trust and who have a reputation. In fact, family members, neighbors and close friends significantly influence the individual's decision (Borges et al., Reference Borges, Oude Lansink, Marques Ribeiro and Lutke2014), especially in the case where the individual's neighbor is also a relative of the individual performing the act. Therefore, a large number of Nei&Rel people converting to organic farming can strongly influence farmers' converting intention.
Secondly, the NE indirectly impacts farmers' converting intention through three factors: ATT, SN and PBC. When observing neighbors and relatives living in the surrounding area converting, farmers may ignore self-gathered information and follow the group's converting behavior. There are three possible reasons for this. First, when neighbors and relatives have converted to organic farming, farmers under study will have more confidence in the economic and non-economic benefits they will receive from the organic conversion. Secondly, many relatives and neighbors moving to organic farming can create converting pressure on farmers under study. In a study on farmers' intention to diversify agricultural production, Hansson et al. (Reference Hansson, Ferguson, Olofsson and Rantamäki-Lahtinen2013) and Senger et al. (Reference Senger, Borges and Machado2017) showed that the closer people are to farmers, the more influence these people have on farmers' decisions. Thirdly, imitating the group's behavior can help the imitator learn knowledge and experience faster, without wasting time, accumulating knowledge and verifying the learned experience. These factors help them increase their confidence in and perceived risk control of organic farming. Such a learning process increases farmers' confidence in their converting practices and increases awareness of the risk control of organic farming. Based on the positive correlation between the NE and TPB's elements, policy-makers and extension workers can take advantage of the NE to increase farmers' confidence about the benefits and the possibility of success in organic farming. Of course, the information disseminated through the neighbors must be correct, as Allsopp and Hey (Reference Allsopp and Hey2000) indicated.
Many empirical studies show that organic agriculture brings both economic and non-economic benefits (including health and the environment). However, economic benefits are visible in the short term, while non-economic benefits are often tangible in the long run. There are many differences between farmers' situations in developed and developing countries concerning the conversion to organic farming. In many developed countries, farmers receive subsidies for the conversion. They also have easier access to markets with high demand for organic products. At the same time, price differences between organic and conventional products are high (Karki et al., Reference Karki, Schleenbecker and Hamm2011). Therefore, they do not have to struggle with economic difficulties too much. In developing countries, people are more concerned with economic benefits than health and environmental ones. They are not unaware of the harmful effects of chemicals used in traditional farming, but they must struggle with economic difficulties, at least in the short run. The conversion to organic farming may present several short-term economic risks, such as possible yield reductions, increased pest and disease outbreaks and uncertainty about market prices. That is one of the reasons why farmers are hesitant to convert to organic farming.
The results of this study show that NE increases farmers' intention to convert to organic farming. Our study site is similar to mountainous areas in many other developing countries. First, farmers have a low standard of living. Therefore, they are more concerned with economic benefits, at least in the short term. Secondly, they prefer traditions and are reluctant to change, partly because their cultural identity is deeply rooted and partly because they fear economic risks (many of them are inherently in difficult economic conditions). Thirdly, farmers in these areas have a high sense of community, so they often follow each other. These features are essential in promoting the NE in the conversion to organic agriculture. Therefore, our research results can be applied outside of our study area. Specifically, to encourage farmers to convert to organic farming, it is necessary to encourage a certain number of farming households to take the lead in converting to organic agriculture. The next step is supporting these farmers to convert to organic farming successfully. Such success must be the economic performance that is visible to everyone. Of course, this success also includes non-economic benefits, which are invisible. Once the supported households have successfully converted to organic farming, the remaining farmers in the village will also convert without hesitance. Our argument is entirely consistent with Niu et al. (Reference Niu, Chen, Gao, Wang, Chen and Zhao2022), who conducted their research in five provinces on the North China Plain. They found that the peer effects of the farmers' adoption behavior of green control techniques mainly came from the learning imitation mechanism.
Conclusions
This study aims to analyze the influence of NE on tea farmers' intention to convert from traditional to organic farming in the mountainous areas of northern Vietnam. To do so, we combine the TPB and the HB, focusing on the impact of the NE. Using the GSEM and data collected from 263 tea farmers in Thai Nguyen, our empirical results show that farmers who intend to convert to organic farming are usually younger and have better education and more experience. Consistent with the theory, ATT, SN and PBC directly influence their converting intention. Besides, the NE has a significant direct influence on farmers' converting intention in the case where the neighbors are also relatives of the farmer and have already converted to organic farming. Furthermore, farmers' converting intention is indirectly affected by NE. These results provide critical insight into farmers' intention to convert from traditional to organic farming.
Based on the positive correlation between the NE and TPB's elements, policy-makers and extension workers can take advantage of the NE to increase farmers' confidence about the benefits and the possibility of success in organic farming. Doing so will spur farmers to convert from traditional to organic farming. For example, in each community, the local authorities should select some typical farmers. These selected farmers must be those who dare to take the lead in converting to organic farming, have good production and business capacity and have much influence on the rest of the community. Then, local governments should focus on supporting these farmers to successfully convert to organic farming, including support in the capital, farming techniques, risk prevention and product commercialization. With the success of these forerunners, the remaining farmers in each region will easily see economic benefits (stable sales and higher prices) and non-economic benefits (healthy living environment and safe working conditions). Along with the socio-economic development, consumer demand will be for clean products. Farmers will gradually realize that the competitiveness of organic tea is getting stronger, and that of traditional tea is increasingly weak. These factors will motivate the remaining farmers in each community to imitate the conversion to organic agriculture.
However, in some cases, the NE alone does not lead to a conversion to organic agriculture. Although farmers see their neighbors as economically successful in converting to organic tea, they do not dare to follow their neighbors. The reason may be that there is no one to inspire the farmers at the moment when they have to decide whether to convert to organic tea or not. Policy-makers and advisors must combine the neighborhood and crowd effects in this case. In our study area, we found that the NE works best when many farmers simultaneously harvest tea near each other. At this time, the local government came to meet and let the farmers register to grow tea according to the organic method. When one or a few households register to convert to organic tea, the remaining households immediately follow. The reason is that the crowd effect fuels the NE.
Despite our intensive efforts, we cannot avoid technical limitations. In this study, GSEM helps us to minimize the biases encountered by SEM when solving structural models with different types of dependent variables. However, GSEM cannot calculate NE's total impact (including total indirect and direct impact) on INT. The cause of this problem is due to the difference in measuring the dependent variables (e.g., the variable INT is not continuous while the variables ATT, SN and PBC are continuous). This limitation suggests future research directions. For example, overcoming the above limitation will allow future studies to calculate the total impact this study has been unable to make.
Acknowledgments
The authors would like to acknowledge and thank the representatives of tea growing households, researchers and extension workers who contributed information to this study.
Financial support
This research is not funded by a specific project grant.
Conflict of interest
The authors declare that they have no known competing financial interests or personal relationships that could have appeared to influence the work reported in this paper.
Appendix
Table A1. Study concepts and items

Table A2. Results of EFA

Table A3. Results of CFA
