Book contents
- Applications of Data Assimilation and Inverse Problems in the Earth Sciences
- Series page
- Applications of Data Assimilation and Inverse Problems in the Earth Sciences
- Copyright page
- Contents
- Contributors
- Preface
- Acknowledgements
- Part I Introduction
- Part II ‘Fluid’ Earth Applications: From the Surface to the Space
- Part III ‘Solid’ Earth Applications: From the Surface to the Core
- Index
- References
Part II - ‘Fluid’ Earth Applications: From the Surface to the Space
Published online by Cambridge University Press: 20 June 2023
- Applications of Data Assimilation and Inverse Problems in the Earth Sciences
- Series page
- Applications of Data Assimilation and Inverse Problems in the Earth Sciences
- Copyright page
- Contents
- Contributors
- Preface
- Acknowledgements
- Part I Introduction
- Part II ‘Fluid’ Earth Applications: From the Surface to the Space
- Part III ‘Solid’ Earth Applications: From the Surface to the Core
- Index
- References
Summary
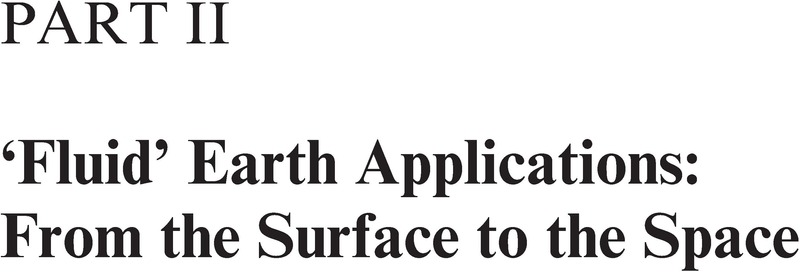
- Type
- Chapter
- Information
- Publisher: Cambridge University PressPrint publication year: 2023