Book contents
- Frontmatter
- Dedication
- Contents
- Preface
- 1 Introduction
- 2 Markov-Chain Monte Carlo
- 3 Ensemble Kalman Filters
- 4 Stochastic Spectral Methods
- 5 Karhunen–Loève Expansion
- 6 Diffusion Forecast
- Appendix A Elementary Probability Theory
- Appendix B Stochastic Processes
- Appendix C Elementary Differential Geometry
- References
- Index
- References
References
Published online by Cambridge University Press: 06 July 2018
- Frontmatter
- Dedication
- Contents
- Preface
- 1 Introduction
- 2 Markov-Chain Monte Carlo
- 3 Ensemble Kalman Filters
- 4 Stochastic Spectral Methods
- 5 Karhunen–Loève Expansion
- 6 Diffusion Forecast
- Appendix A Elementary Probability Theory
- Appendix B Stochastic Processes
- Appendix C Elementary Differential Geometry
- References
- Index
- References
Summary
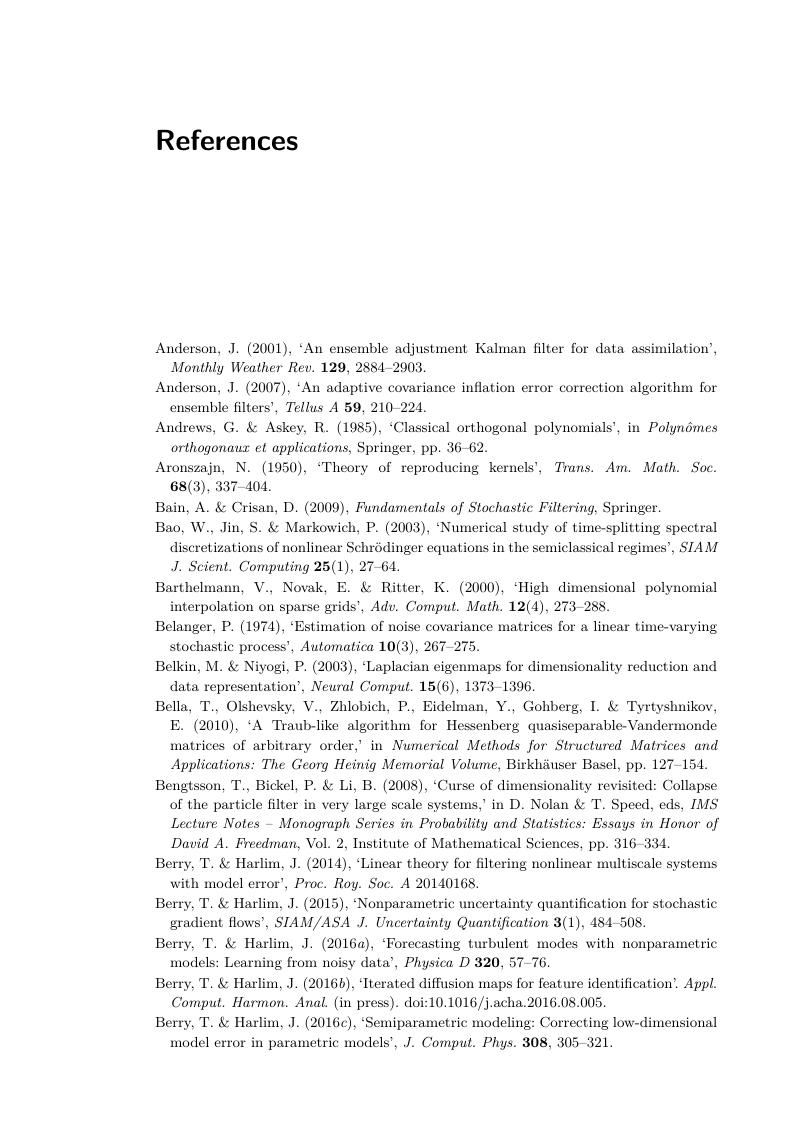
- Type
- Chapter
- Information
- Data-Driven Computational MethodsParameter and Operator Estimations, pp. 149 - 156Publisher: Cambridge University PressPrint publication year: 2018