Book contents
- Applied Mixed Model Analysis
- Practical Guides to Biostatistics and Epidemiology
- Applied Mixed Model Analysis
- Copyright page
- Dedication
- Contents
- Preface
- 1 Introduction
- 2 Basic Principles of Mixed Model Analysis
- 3 What Is Gained by Using Mixed Model Analysis?
- 4 Logistic Mixed Model Analysis
- 5 Mixed Model Analysis with Different Outcome Variables
- 6 Explaining Differences between Groups
- 7 Multivariable Modelling
- 8 Predictions Based on Mixed Model Analysis
- 9 Mixed Model Analysis in Longitudinal Studies
- 10 Multivariate Mixed Model Analysis
- 11 Meta-Analysis on Individual Participant Data
- 12 Sample-Size Calculations
- 13 Some Loose Ends …
- References
- Index
- References
References
Published online by Cambridge University Press: 15 May 2019
- Applied Mixed Model Analysis
- Practical Guides to Biostatistics and Epidemiology
- Applied Mixed Model Analysis
- Copyright page
- Dedication
- Contents
- Preface
- 1 Introduction
- 2 Basic Principles of Mixed Model Analysis
- 3 What Is Gained by Using Mixed Model Analysis?
- 4 Logistic Mixed Model Analysis
- 5 Mixed Model Analysis with Different Outcome Variables
- 6 Explaining Differences between Groups
- 7 Multivariable Modelling
- 8 Predictions Based on Mixed Model Analysis
- 9 Mixed Model Analysis in Longitudinal Studies
- 10 Multivariate Mixed Model Analysis
- 11 Meta-Analysis on Individual Participant Data
- 12 Sample-Size Calculations
- 13 Some Loose Ends …
- References
- Index
- References
Summary
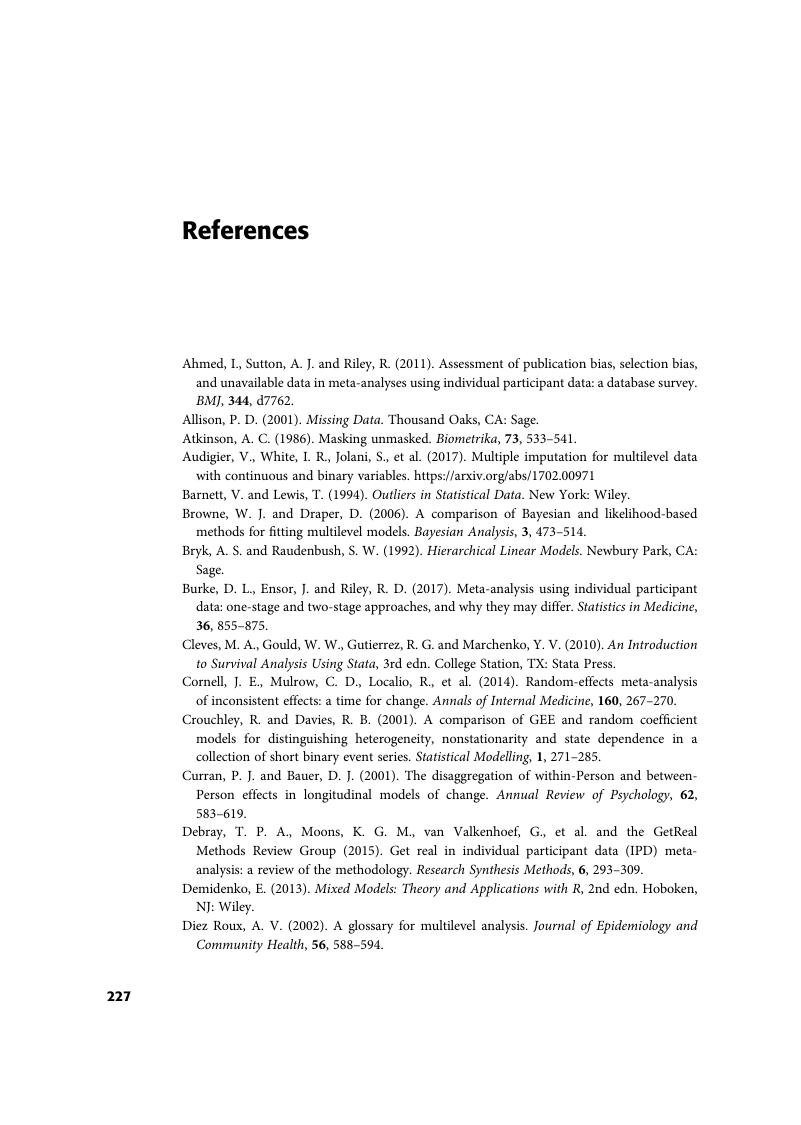
- Type
- Chapter
- Information
- Applied Mixed Model AnalysisA Practical Guide, pp. 227 - 233Publisher: Cambridge University PressPrint publication year: 2019