References
Published online by Cambridge University Press: 05 November 2012
Summary
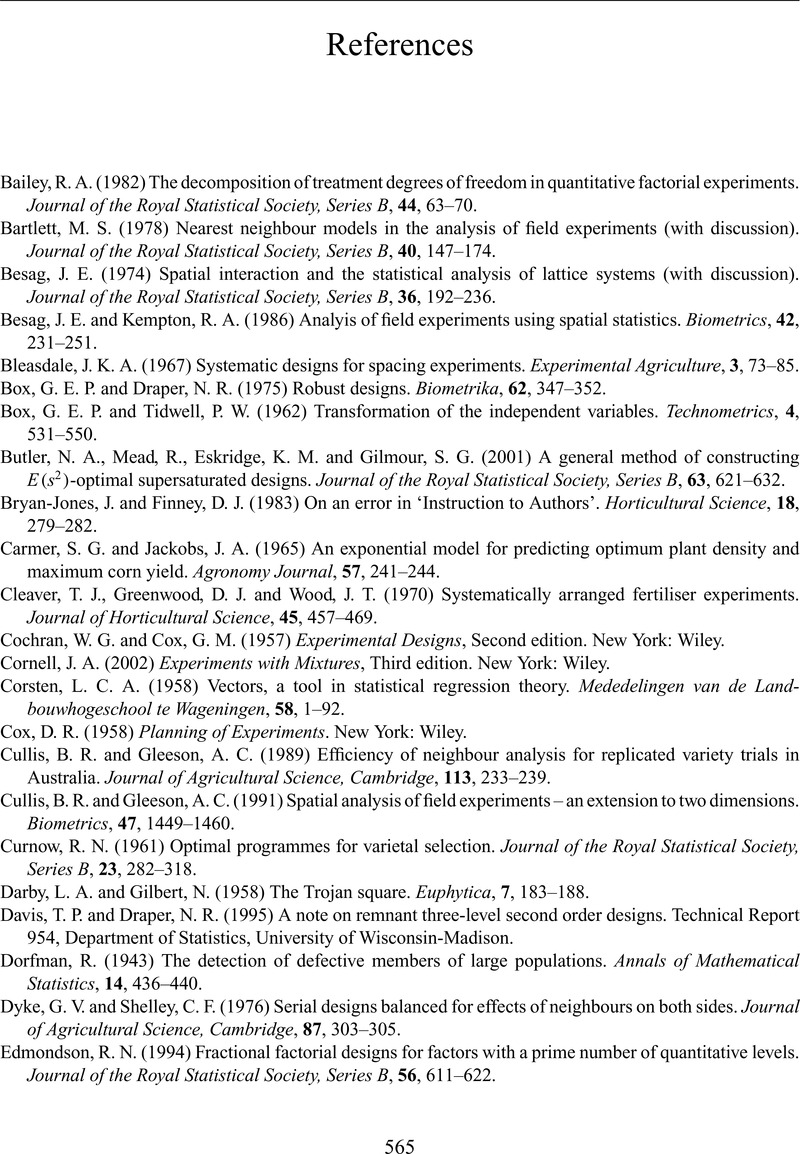
- Type
- Chapter
- Information
- Statistical Principles for the Design of ExperimentsApplications to Real Experiments, pp. 565 - 567Publisher: Cambridge University PressPrint publication year: 2012