149 results
Racial and ethnic differences in the degree of participation and retention in a decentralized cohort study of COVID-19 immunization in patients with inflammatory bowel diseases
-
- Journal:
- Journal of Clinical and Translational Science / Volume 8 / Issue 1 / 2024
- Published online by Cambridge University Press:
- 18 January 2024, e31
-
- Article
-
- You have access
- Open access
- HTML
- Export citation
42 Cognitive and Neuroanatomic Correlates of Olfactory Function in Cognitively Unimpaired and Impaired Older Adults
-
- Journal:
- Journal of the International Neuropsychological Society / Volume 29 / Issue s1 / November 2023
- Published online by Cambridge University Press:
- 21 December 2023, pp. 250-251
-
- Article
-
- You have access
- Export citation
Figures
-
- Book:
- Applying Benford's Law for Assessing the Validity of Social Science Data
- Published online:
- 09 November 2023
- Print publication:
- 23 November 2023, pp vii-viii
-
- Chapter
- Export citation
Acknowledgments
-
- Book:
- Applying Benford's Law for Assessing the Validity of Social Science Data
- Published online:
- 09 November 2023
- Print publication:
- 23 November 2023, pp xv-xvi
-
- Chapter
- Export citation
Chapter 5 - Benford Agreement Analysis of the Sea Around Us Project’s Fish-Landings Data
-
- Book:
- Applying Benford's Law for Assessing the Validity of Social Science Data
- Published online:
- 09 November 2023
- Print publication:
- 23 November 2023, pp 94-130
-
- Chapter
- Export citation
Chapter 4 - Data Characteristics and the Workflow of Benford Agreement Analysis
-
- Book:
- Applying Benford's Law for Assessing the Validity of Social Science Data
- Published online:
- 09 November 2023
- Print publication:
- 23 November 2023, pp 74-93
-
- Chapter
- Export citation
Chapter 7 - Assessing the Impacts of Problematic Benford Validity
-
- Book:
- Applying Benford's Law for Assessing the Validity of Social Science Data
- Published online:
- 09 November 2023
- Print publication:
- 23 November 2023, pp 169-187
-
- Chapter
- Export citation
Index
-
- Book:
- Applying Benford's Law for Assessing the Validity of Social Science Data
- Published online:
- 09 November 2023
- Print publication:
- 23 November 2023, pp 204-208
-
- Chapter
- Export citation
Chapter 3 - Benford’s Law and Assessing Conformity
-
- Book:
- Applying Benford's Law for Assessing the Validity of Social Science Data
- Published online:
- 09 November 2023
- Print publication:
- 23 November 2023, pp 24-73
-
- Chapter
- Export citation
Preface
-
- Book:
- Applying Benford's Law for Assessing the Validity of Social Science Data
- Published online:
- 09 November 2023
- Print publication:
- 23 November 2023, pp xiii-xiv
-
- Chapter
- Export citation
Copyright page
-
- Book:
- Applying Benford's Law for Assessing the Validity of Social Science Data
- Published online:
- 09 November 2023
- Print publication:
- 23 November 2023, pp iv-iv
-
- Chapter
- Export citation
Chapter 6 - Benford Agreement Analysis of US and Global COVID-19 New Cases Data
-
- Book:
- Applying Benford's Law for Assessing the Validity of Social Science Data
- Published online:
- 09 November 2023
- Print publication:
- 23 November 2023, pp 131-168
-
- Chapter
- Export citation
References
-
- Book:
- Applying Benford's Law for Assessing the Validity of Social Science Data
- Published online:
- 09 November 2023
- Print publication:
- 23 November 2023, pp 196-203
-
- Chapter
- Export citation
Tables
-
- Book:
- Applying Benford's Law for Assessing the Validity of Social Science Data
- Published online:
- 09 November 2023
- Print publication:
- 23 November 2023, pp ix-xii
-
- Chapter
- Export citation
Chapter 1 - Introduction
-
- Book:
- Applying Benford's Law for Assessing the Validity of Social Science Data
- Published online:
- 09 November 2023
- Print publication:
- 23 November 2023, pp 1-10
-
- Chapter
-
- You have access
- HTML
- Export citation
Contents
-
- Book:
- Applying Benford's Law for Assessing the Validity of Social Science Data
- Published online:
- 09 November 2023
- Print publication:
- 23 November 2023, pp v-vi
-
- Chapter
- Export citation
Chapter 2 - Validity and Self-Reported Data
-
- Book:
- Applying Benford's Law for Assessing the Validity of Social Science Data
- Published online:
- 09 November 2023
- Print publication:
- 23 November 2023, pp 11-23
-
- Chapter
-
- You have access
- HTML
- Export citation
Chapter 8 - Conclusion
-
- Book:
- Applying Benford's Law for Assessing the Validity of Social Science Data
- Published online:
- 09 November 2023
- Print publication:
- 23 November 2023, pp 188-195
-
- Chapter
- Export citation
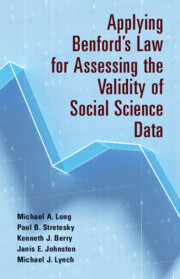
Applying Benford's Law for Assessing the Validity of Social Science Data
-
- Published online:
- 09 November 2023
- Print publication:
- 23 November 2023
Effects of a hard stop for C. difficile testing: Provider uptake and patient outcomes
-
- Journal:
- Antimicrobial Stewardship & Healthcare Epidemiology / Volume 3 / Issue S2 / June 2023
- Published online by Cambridge University Press:
- 29 September 2023, p. s44
-
- Article
-
- You have access
- Open access
- Export citation