Book contents
- Frontmatter
- Contents
- Preface
- Contributors
- 1 Introduction
- 2 Fast ICA by a fixed-point algorithm that maximizes non-Gaussianity
- 3 ICA, graphical models and variational methods
- 4 Nonlinear ICA
- 5 Separation of non-stationary natural signals
- 6 Separation of non-stationary sources: algorithms and performance
- 7 Blind source separation by sparse decomposition in a signal dictionary
- 8 Ensemble Learning for blind source separation
- 9 Image processing methods using ICA mixture models
- 10 Latent class and trait models for data classification and visualisation
- 11 Particle filters for non-stationary ICA
- 12 ICA: model order selection and dynamic source models
- References
- Index
- References
References
Published online by Cambridge University Press: 05 July 2014
- Frontmatter
- Contents
- Preface
- Contributors
- 1 Introduction
- 2 Fast ICA by a fixed-point algorithm that maximizes non-Gaussianity
- 3 ICA, graphical models and variational methods
- 4 Nonlinear ICA
- 5 Separation of non-stationary natural signals
- 6 Separation of non-stationary sources: algorithms and performance
- 7 Blind source separation by sparse decomposition in a signal dictionary
- 8 Ensemble Learning for blind source separation
- 9 Image processing methods using ICA mixture models
- 10 Latent class and trait models for data classification and visualisation
- 11 Particle filters for non-stationary ICA
- 12 ICA: model order selection and dynamic source models
- References
- Index
- References
Summary
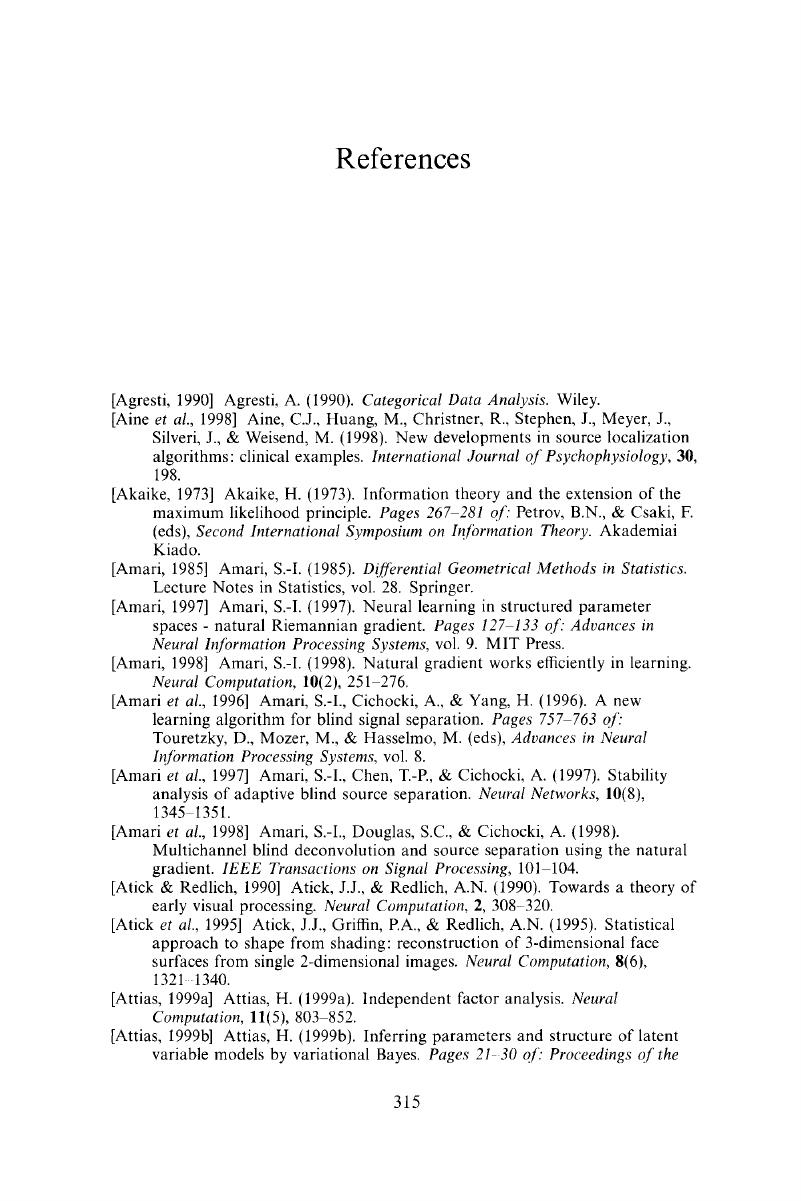
- Type
- Chapter
- Information
- Independent Component AnalysisPrinciples and Practice, pp. 315 - 335Publisher: Cambridge University PressPrint publication year: 2001