Book contents
- Frontmatter
- Contents
- List of contributors
- Preface
- 1 Inference and estimation in probabilistic time series models
- I Monte Carlo
- II Deterministic approximations
- 5 Two problems with variational expectation maximisation for time series models
- 6 Approximate inference for continuous-time Markov processes
- 7 Expectation propagation and generalised EP methods for inference in switching linear dynamical systems
- 8 Approximate inference in switching linear dynamical systems using Gaussian mixtures
- III Switching models
- IV Multi-object models
- V Nonparametric models
- VI Agent-based models
- Index
- Plate section
- References
8 - Approximate inference in switching linear dynamical systems using Gaussian mixtures
from II - Deterministic approximations
Published online by Cambridge University Press: 07 September 2011
- Frontmatter
- Contents
- List of contributors
- Preface
- 1 Inference and estimation in probabilistic time series models
- I Monte Carlo
- II Deterministic approximations
- 5 Two problems with variational expectation maximisation for time series models
- 6 Approximate inference for continuous-time Markov processes
- 7 Expectation propagation and generalised EP methods for inference in switching linear dynamical systems
- 8 Approximate inference in switching linear dynamical systems using Gaussian mixtures
- III Switching models
- IV Multi-object models
- V Nonparametric models
- VI Agent-based models
- Index
- Plate section
- References
Summary
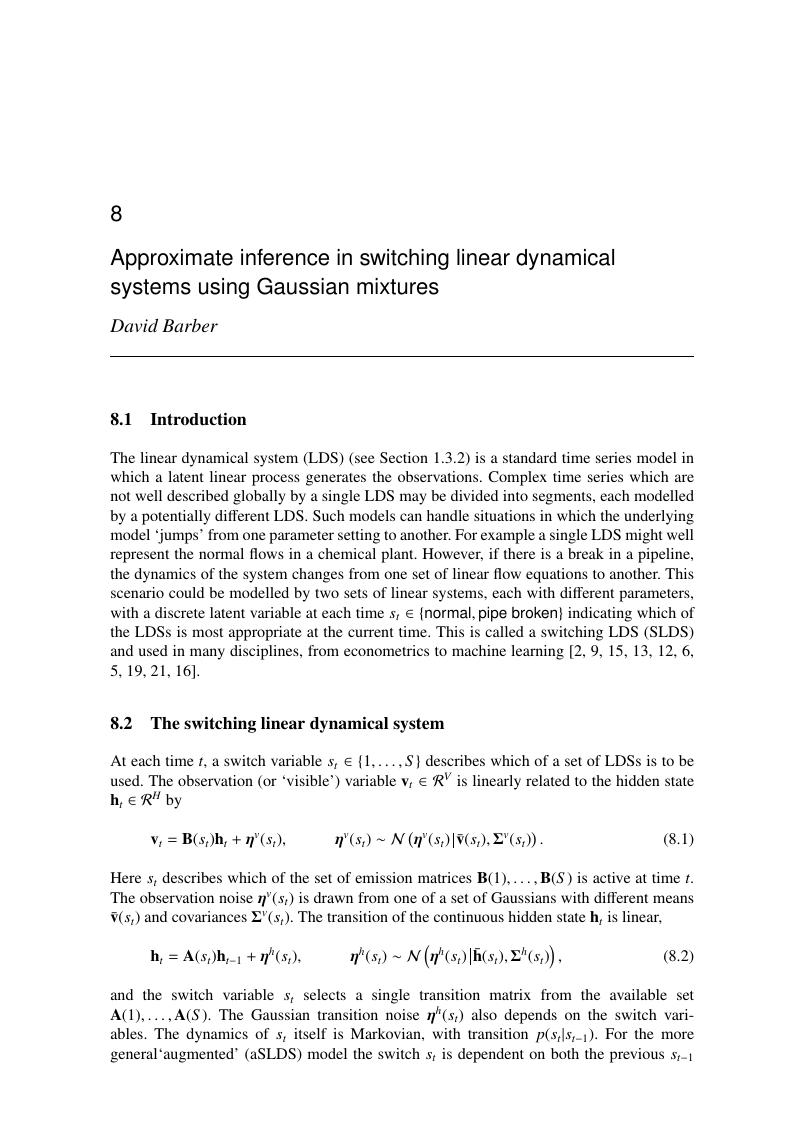
- Type
- Chapter
- Information
- Bayesian Time Series Models , pp. 166 - 181Publisher: Cambridge University PressPrint publication year: 2011