1 Introduction
Novelty is an essential element of invention (Lubart Reference Lubart1994; Sternberg & Lubart Reference Sternberg and Lubart1996; Luo Reference Luo2015). High novelty implies an increase in the variability which can result in both breakthrough and failure (Fleming Reference Fleming2001). Empirical studies on the impact of novel versus conventional design stimuli on creative output have reported mixed results (Chan & Schunn Reference Chan and Schunn2015a ). Despite its clear value to invention, excessive novelty may also harm invention by introducing challenges to its embodiment, product development, manufacture, and user adoption (Luo Reference Luo2015). In the pursuit of invention, inventors are faced with a ‘novelty dilemma’. Prior engineering design research has speculated that a novelty ‘sweet spot’ may exist which delivers the best invention outcome (Fu et al. Reference Fu, Chan, Cagan, Kotovsky, Schunn and Wood2013); there may be such a thing as ‘too much’ or ‘too little’ novelty that will determine an invention’s future value.
If the novelty sweet spot does exist, the next question is where it is – how much novelty is needed for inventions in the sweet spot? In this research, we aim to answer this question empirically by drawing on the combination theory of invention to analyze novelty. Prior studies of creativity considered an invention as the recombination of prior technologies or knowledge, and suggested that uncommon combinations give rise to novelty (Simonton Reference Simonton1999; Fleming Reference Fleming2001; Ward Reference Ward2001; Arthur Reference Arthur2007; Basnet & Magee Reference Basnet and Magee2016). Thus, an invention’s novelty can be measured as the frequency with which the prior technologies the invention recombines had previously been combined (Uzzi et al. Reference Uzzi, Mukherjee, Stringer and Jones2013; Chan & Schunn Reference Chan and Schunn2015a ). Several recent studies have utilized the massive data of patents as the proxy of inventions to analyze technology combinations (Youn et al. Reference Youn, Strumsky, Bettencourt and Lobo2015; Kim et al. Reference Kim, Cerigo, Jeong and Youn2016). We follow these prior works to analyze 3.9 million technical patents from the United States Patent and Trademark Office (USPTO) to explore the novelty sweet spot in the combination space of invention.
Our empirical results show a clear novelty ‘sweet spot’ at which the suitable level of novelty of prior technology combinations favors an invention’s eventual success. We also found that combination of scientific and broader knowledge beyond patentable technologies creates additional value for the patented invention and increases the advantage of the novelty sweet spot. The identification and nuanced understanding of the novelty sweet spot contributes to the design creativity literature and can guide inventors to pursue more valuable inventions in practice. Our methodology may also contribute to the growing literature on the data-driven evaluation of design creativity. Below, we will first review the related literature and introduce our methodology and then report our empirical findings.
2 Literature review
To explore the novelty sweet spot for invention, this research primarily draws on the literature of combination theory and novelty measurement.
2.1 Design combination, novelty and outcome
The prior literature has suggested that novelty arises from uncommon combinations. Simonton (Reference Simonton1999) argued that an invention is the recombination of existing technologies and that the novelty of the invention is the result of unconventional combinations of prior technologies. Arthur (Reference Arthur2007) proposed that invention results from recursive problem solving by combining existing technologies. Youn et al. (Reference Youn, Strumsky, Bettencourt and Lobo2015) used patent classification codes as a proxy of technologies to analyze the multi-classification of US patents and found that the major driver of modern invention has indeed been the combination of existing technologies rather than the introduction of new technologies. Luo & Wood (Reference Luo and Wood2017) found a trend that patented inventions have been combining the knowledge of broader domains over the past three decades. Synthesizing the anecdotal accounts of creative writing and laboratory investigations, Ward (Reference Ward2001) noted that new properties can arise out of conceptual combination. Basnet & Magee (Reference Basnet and Magee2016) focused on the combination of analogical transfers in the cognitive process and argued that new inventive ideas are created by using the combinatorial analogical transfer of existing ideas. Nickerson (Reference Nickerson2015) stressed that creative work can be performed by thousands of people through collective design, i.e., designers modify and combine each other’s work in a process called remixing.
Combinations can involve different sources and lead to different outcomes. With empirical studies and a psychoanalytic interpretation of poem-creation activities, Rothenberg (Reference Rothenberg1980) proposed two combinatory thinking processes that foster creativity, i.e., Janusian thinking which conceives two or more opposite or antithetical entities simultaneously and homospatial thinking which conceives two or more discrete entities occupying the same space. Analyzing scientific publications, Uzzi et al. (Reference Uzzi, Mukherjee, Stringer and Jones2013) found that the scientific papers that have the greatest impact (with an outstanding number of future citations) are grounded in a mass of exceptionally conventional combinations of prior work and a minor insertion of highly novel combinations. Kim et al. (Reference Kim, Cerigo, Jeong and Youn2016) found that patents that add novel combinations of their co-classifications to conventional combinations are most likely to become ‘hits’. Based on US patent citation data, Fleming (Reference Fleming2001, Reference Fleming2007) found that although novel combinations based on rareness in historical occurrences lead to less useful inventions on average, they also give rise to the variability that can result in both breakthrough and failure.
Despite a robust link between distant combinations and the increased novelty of concepts, prior laboratory experiments or empirical studies have reported mixed results on the impact of distant combinations on design outcome (Chan & Schunn Reference Chan and Schunn2015a ). It is naturally difficult for scientists and inventors to retrieve, absorb, and integrate technologies across unfamiliar domains. For instance, Chan & Schunn (Reference Chan and Schunn2015a ) found that the direct effects of far combinations have a mean zero effect on creative concept generation, and iterations are important for converting distant combinations into creative concepts. Kaufman & Baer (Reference Kaufman, Baer, Sternberg, Grigorenko and Singer2004) found that creativity is more domain-specific than general, and it is naturally difficult for people to effectively combine technologies across distant domains. Forbus et al. (Reference Forbus, Gentner and Law1995) found that during knowledge retrieval, superficial reminding is much more frequent than structural reminding, which makes it difficult to achieve design novelty from a distant enough combination. Based on an analysis of 2.8 million inventors’ 3.9 million patents, Alstott et al. (Reference Alstott, Triulzi, Yan and Luo2017a ) found that inventors are far more likely to obtain new patents in new domains that are more related to their previous patents than in more distant domains.
Similarly, studies of design-by-analogy have suggested that novelty may arise when design is conceived by analogy across distant domains (Gick & Holyoak Reference Gick and Holyoak1980; Gentner & Markman Reference Gentner and Markman1997; Ward Reference Ward2001; Chan et al. Reference Chan, Fu, Schunn, Cagan, Wood and Kotovsky2011), but Gick & Holyoak (Reference Gick and Holyoak1980) found that human subjects often fail to notice the relevance of an analogy because of the cognitive distance between the potential solution and the target problem. Chan & Schunn (Reference Chan and Schunn2015b ) conducted an in vivo study and found that distant analogies do not lead directly to creative concepts via large leaps but instead increase the concept generation rate. Chan, Dow and Schunn (Reference Chan, Dow and Schunn2015) found that conceptually closer rather than more distant stimuli appear to be more beneficial to design because of their easier perception and more obvious connection to the design problem. Fu et al. (Reference Fu, Chan, Cagan, Kotovsky, Schunn and Wood2013) found that if stimuli are too distant from the design problem, they can become harmful to the design process and outcome. Accordingly, they argued that there might be such a thing as too ‘near’ and too ‘far’ in design analogies and that the stimuli from the ‘middle ground’ may be desirable for developing creative solutions.
Taken together, the literature has suggested that novel combinations are fundamental for invention and particularly crucial for breakthroughs, but excessively novel combinations may be ineffective and lead to poor results. Meanwhile, conventional combinations, compared with novel ones, are relatively easy and effective. The most desirable design outcome may arise in the middle ground, suggesting a hypothesis that there is a novelty ‘sweet spot’ of prior technology combinations. Our research aims to empirically test this hypothesis and to identify the novelty sweet spot. To do so, the evaluation of novelty is required.
2.2 Evaluation of design novelty
To evaluate novelty, design researchers have proposed various definitions, metrics, and methods. In general, novelty indicates that an invention is new, original, unexpected, and surprising (e.g., Sternberg & Lubart Reference Sternberg and Lubart1999; Simonton Reference Simonton2000; Kaufman & Baer Reference Kaufman, Baer, Sternberg, Grigorenko and Singer2004). Weisberg (Reference Weisberg2006) suggested that novelty is subjective to the experience of the evaluator. Therefore, novelty can be defined with reference to the previous ideas of the individuals concerned or relative to the entirety of human history (Boden Reference Boden1996). For instance, Oman et al. (Reference Oman, Tumer, Wood and Seepersad2013) measured the ‘novelty’ of a new concept as its uniqueness across all functional dimensions relative to a group of comparable ideas. Simonton (Reference Simonton1999) associated the novelty of the invention with the commonality of its combinations of prior technologies. Some researchers have suggested that novelty can be measured by comparing the observed situation with the random one. For example, Uzzi et al. (Reference Uzzi, Mukherjee, Stringer and Jones2013) proposed calculation of the novelty of a combination of scientific fields by comparing the observed frequency of the combination with the random frequency of the combination in the randomized samples. Kim et al. (Reference Kim, Cerigo, Jeong and Youn2016) calculated a relative likelihood that each pair of classification codes is put together at random and a deviation from the empirical observation to assess a patent’s overall novelty.
Traditionally, invention evaluation has been carried out using an expert group approach and based on experts’ subjective opinions, intuitions, or experiences (Amabile Reference Amabile1996). Various procedures and techniques have been proposed to facilitate expert groups and analyze their opinions. Sarkar & Chakrabarti (Reference Sarkar and Chakrabarti2007) introduced the function–behavior–structure (FBS) model and the SAPPhIRE model using product characteristics to measure product novelty. Brown (Reference Brown2015) presented a simple framework for computational design creativity evaluation, which contains agent judging, the set of aspects, knowledge about the designer, etc. Grace et al. (Reference Grace, Maher, Fisher and Brady2015) developed a typology of expectations that, when violated, produce surprise and contribute to creativity.
Evaluation relying on expert opinions is naturally subjective and limited in terms of the data sample size. As a result, it is difficult either to apply rigorous mathematics for evaluation or to test theoretical hypotheses with statistical significance. Meanwhile, there is an increasing call for a computational and data-driven evaluation of design novelty (Brown Reference Brown2015; He & Luo Reference He and Luo2017). Recent studies have developed methods to analyze patent documents to evaluate patented inventions. Patent documents contain rich details, and there are also millions of patents in the public patent databases, which enable a more rigorous and systematical data-driven evaluation of the novelty of patented inventions. For instance, Fleming (Reference Fleming2001) analyzed how frequently the co-classes of a patent were assigned to other patents in the history to indicate the novelty of this patent from a recombination perspective. He & Luo (Reference He and Luo2017) analyzed how frequently a pair of patent classes had appeared together in the references of previous patents to assess its conventionality or novelty.
In this paper, we introduce a data-driven method to measure the novelty of patented inventions, using the extensive data existing in the USPTO patent database, and we then test the hypothesis on the existence of the novelty sweet spot of invention.
3 Data and method
3.1 Data
In this study, we used patents as the proxy of inventions, with awareness of patents’ limitations (e.g., not all inventions are patented). Our analysis involved approximately 3.9 million utility patents granted from 1976 to 2016 contained in the USPTO (United States Patent and Trademark Office) patent databaseFootnote 1 , including the 601,715 patents that were granted in the 1990s with five or more references to prior patents, along with the patents that they cite (i.e., backward references) and the patents that cite them (i.e., forward citations). Our focus on patents in the 1990s ensures that their backward references and forward citations are sufficiently covered, because most citations fall within a time lag of 10 years (Trajtenberg Reference Trajtenberg1990; Hall, Jaffe & Trajtenberg Reference Hall, Jaffe and Trajtenberg2001). The data on patent documents that we used in our analysis are the patent classifications and references (Figure 1). Each patent is assigned to one or more patent classes by the USPTO examiners, indicating which types of technology it embodies. In this study, we used IPC4 (four-digit International Patent Classification) marking 631 patent classes as the proxy of technology fields, as commonly carried out in the innovation literature (Breschi, Lissoni & Malerba Reference Breschi, Lissoni and Malerba2003; Boschma, Heimeriks & Balland Reference Boschma, Heimeriks and Balland2014; Kay et al. Reference Kay, Newman, Youtie, Porter and Rafols2014; Rigby Reference Rigby2015; Alstott et al. Reference Alstott, Triulzi, Yan and Luo2017b ; Yan & Luo Reference Yan and Luo2017).
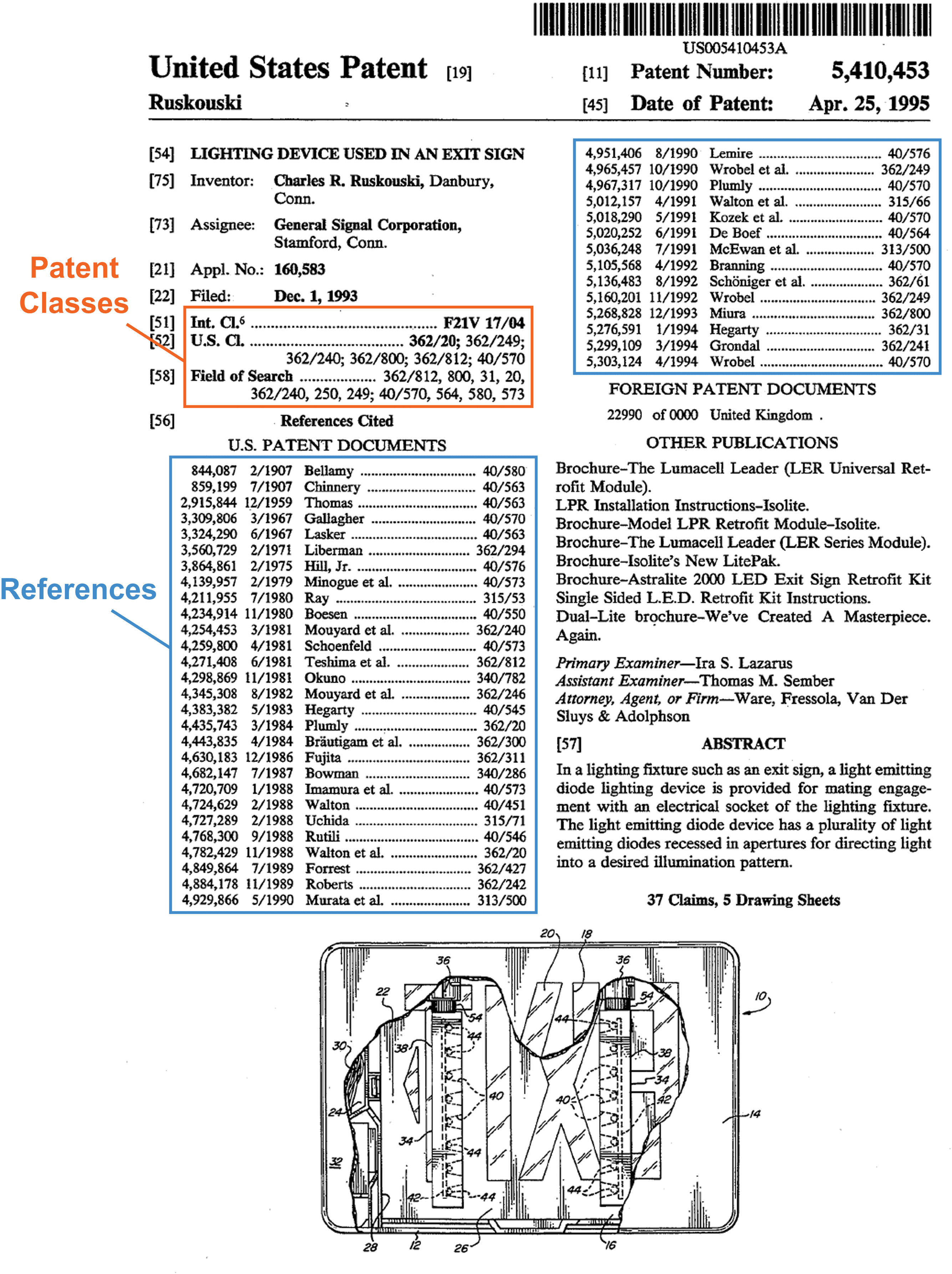
Figure 1. Example of a patent document (US Patent 5410453).
3.2 Method
Figure 2 depicts the structure of our overall research method to statistically compute the novelty profile and the value of a patented invention to identify the novelty sweet spot. The details of each step of the method are described in the following subsections.
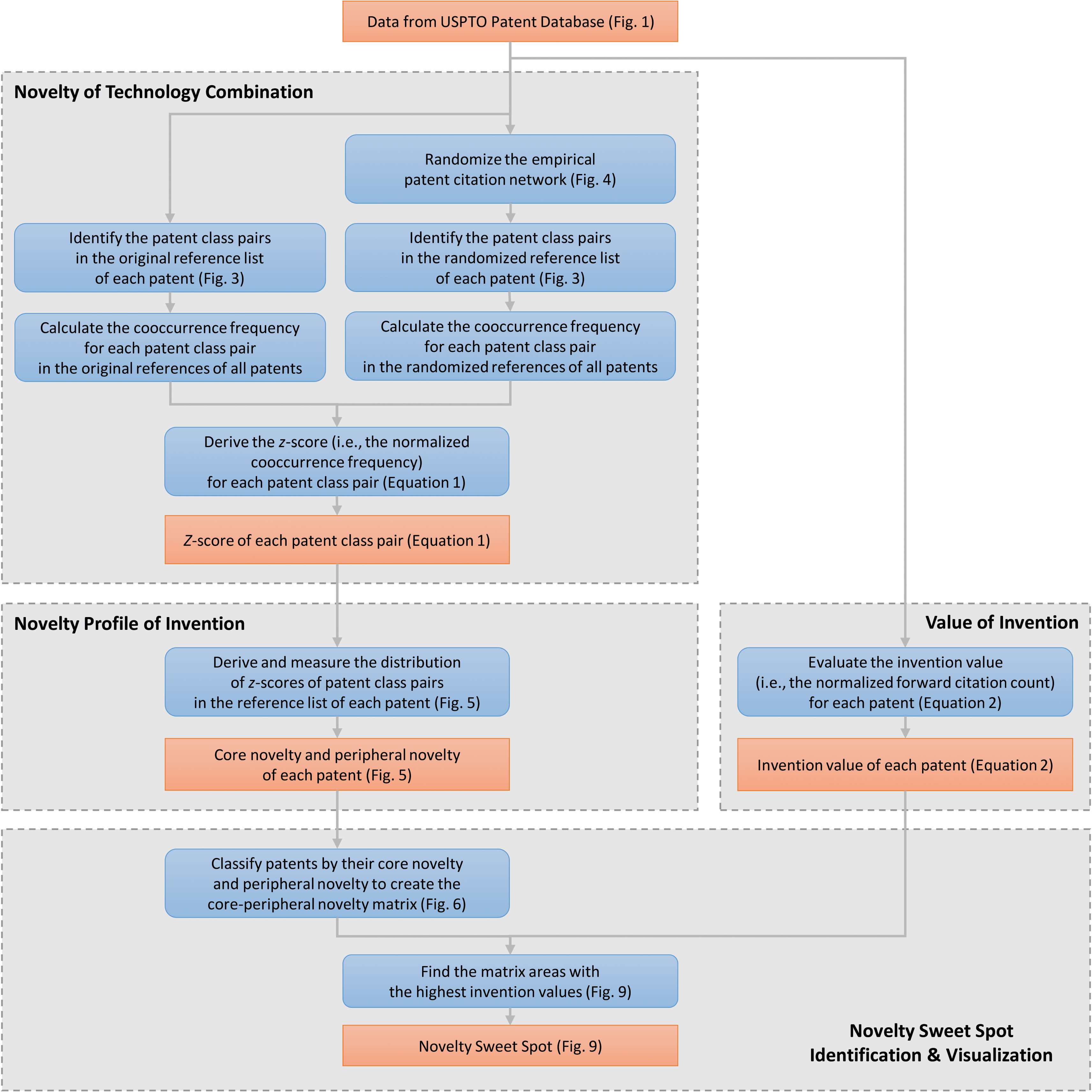
Figure 2. Flow diagram of the research method.
3.2.1 Novelty of technology combination
A pair of patent classes assigned to a patent’s references approximates a recombination of existing technology fields in an invention. With this information, one can calculate the frequency at which a combination of technology fields has occurred in historical inventions’ references to indicate the combination’s novelty. Figure 3 illustrates the procedure to extract all pairs of patent classes assigned to a patent’s references. The first step is to identify the referenced patents of a focal patent (column I). The second step is to identify the classes of these referenced patents (column II). On this basis, the list of all class pairs in the patent’s references is extracted (column III). For example, class A that is assigned to patent 1 (one of the patent references of the focal patent) forms a class pair with class B that is also assigned to patent 1, with class A that is assigned to patent 2, and with classes B, C, and D that are assigned to patent 3, and so on.
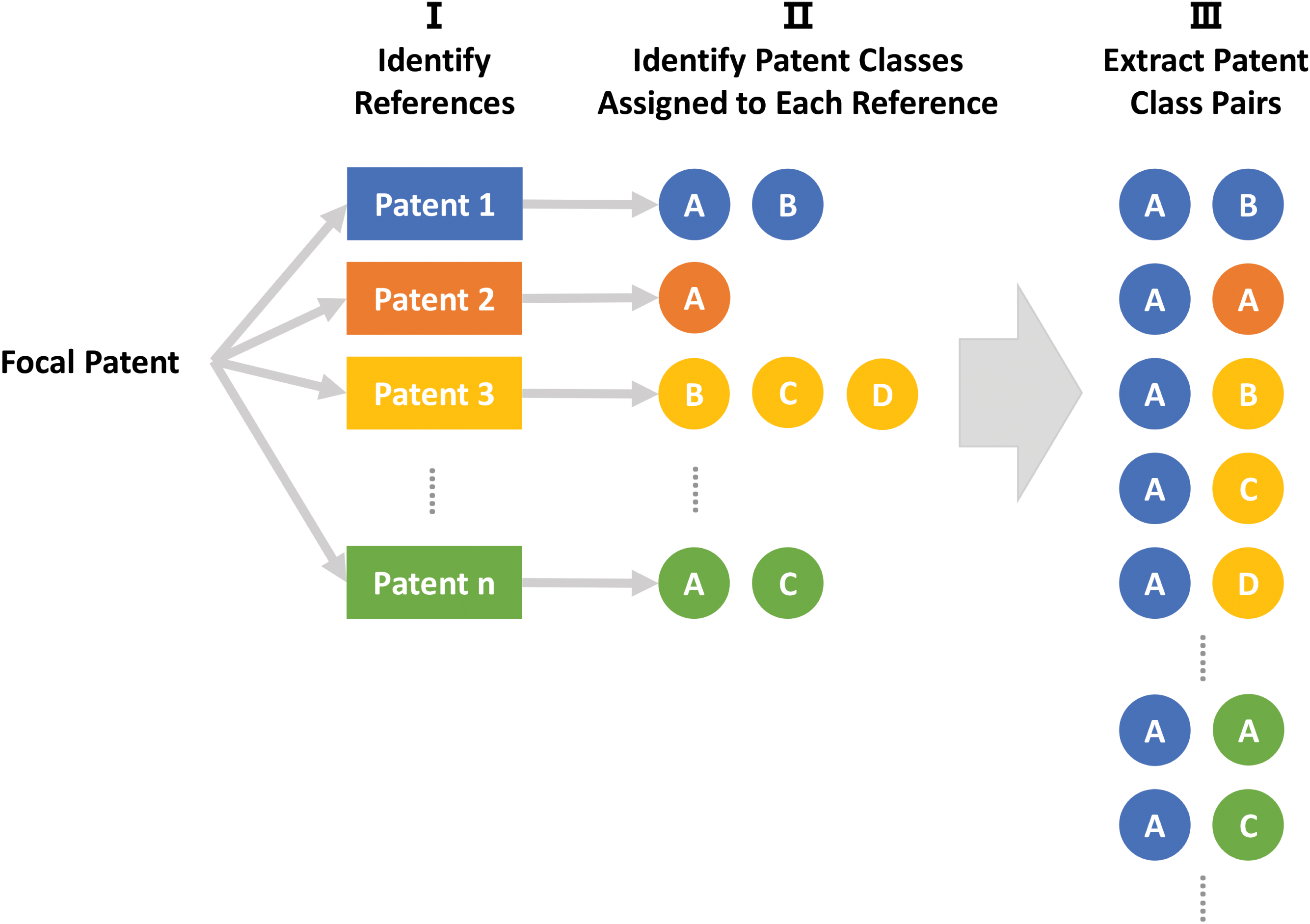
Figure 3. Illustrative procedure of extracting the class pairs in the reference list of a patent.
Following the frequency-based approach to evaluate novelty (Uzzi et al.
Reference Uzzi, Mukherjee, Stringer and Jones2013), we first computed the frequency of a pair of patent classes co-occurring in the references of individual patents in history. Because it is difficult to perceive how large or small a frequency value is, benchmarking is needed. We normalized the empirically observed frequency value by comparing it with the same metric for the comparable randomized citation networks to indicate the novelty of the combination. Such a normalized value relative to comparable random situations is called the ‘
$z$
-score’ in the network science literature, indicating the extent to which the empirical observation deviates from expectations in comparable but randomized settings. The formula for the
$z$
-score is

where
$z_{ij}$
is the relative co-occurrence frequency of the pair of classes
$i$
and
$j$
,
$x$
is the empirically observed co-occurrence frequency of classes
$i$
and
$j$
,
$\unicode[STIX]{x1D707}$
is the average expected co-occurrence frequency of classes
$i$
and
$j$
in comparable randomized citation networks, and
$\unicode[STIX]{x1D70E}$
is its standard deviation. The average expected value and the standard deviation were calculated based on an ensemble of 10 randomized reference lists of the same patents in the randomized citation networks.
Figure 4 illustrates an example of how the empirical citation network was randomized. In the citation network, the nodes are patents and the links are backward references, i.e., an arrow goes from a citing patent to a cited patent. Specifically, we randomly selected a pair of citing-to-cited links with the same citing and cited years (i.e., the years in which the citing and cited patents were granted) and swapped the cited patents. For example, in Figure 4, link
$a$
and link
$b$
can be switched by swapping the cited patents, but link
$a$
and link
$c$
cannot be switched. As a result, the random swapping procedure preserved all of the numbers of forward and backward citations of each patent and the year lags of the citations, which makes the randomized networks comparable with the empirical network.

Figure 4. How the empirical citation network was randomized by swapping the cited patents of randomly selected citing-to-cited links with the same citing and cited years.
We calculated the
$z$
-score of any pair of the 631 IPC4 classes that were assigned to the patents appearing as references of all US patents granted from 1990 to 1999. A lower
$z$
-score, indicating less frequent historical occurrences, suggests higher novelty. Thereafter, we used the additive inverse of the
$z$
-score to measure the novelty of the combination represented by the patent class pair. For example, if the
$z$
-score of a class pair is
$-100$
, its novelty score is 100. If the
$z$
-score of another class pair is 5, its novelty score is
$-5$
. The first pair has a higher novelty score than the latter pair.
3.2.2 Novelty profile of a patented invention
It should be noted that each invention is often composed of multiple combinations of technology fields (i.e., each patent has a set of pairs of classes in the list of its references) and each of the combinations has a different degree of novelty (i.e., each of the class pairs has a
$z$
-score). Thus, the combination space of a patented invention can be viewed as a network of patent classes whose pairings are denoted as weighted links according to the
$z$
-scores of the pairs (see Figure 5(a) for an example). In other words, each invention can be characterized by a spectrum of novelty values given by its combinations, i.e., each patent can be profiled by a spectrum of
$z$
-scores given by the patent class pairs in its references. This spectrum of novelty values can be summarized in a cumulative distribution of the
$z$
-scores (see Figure 5(b) for an example).
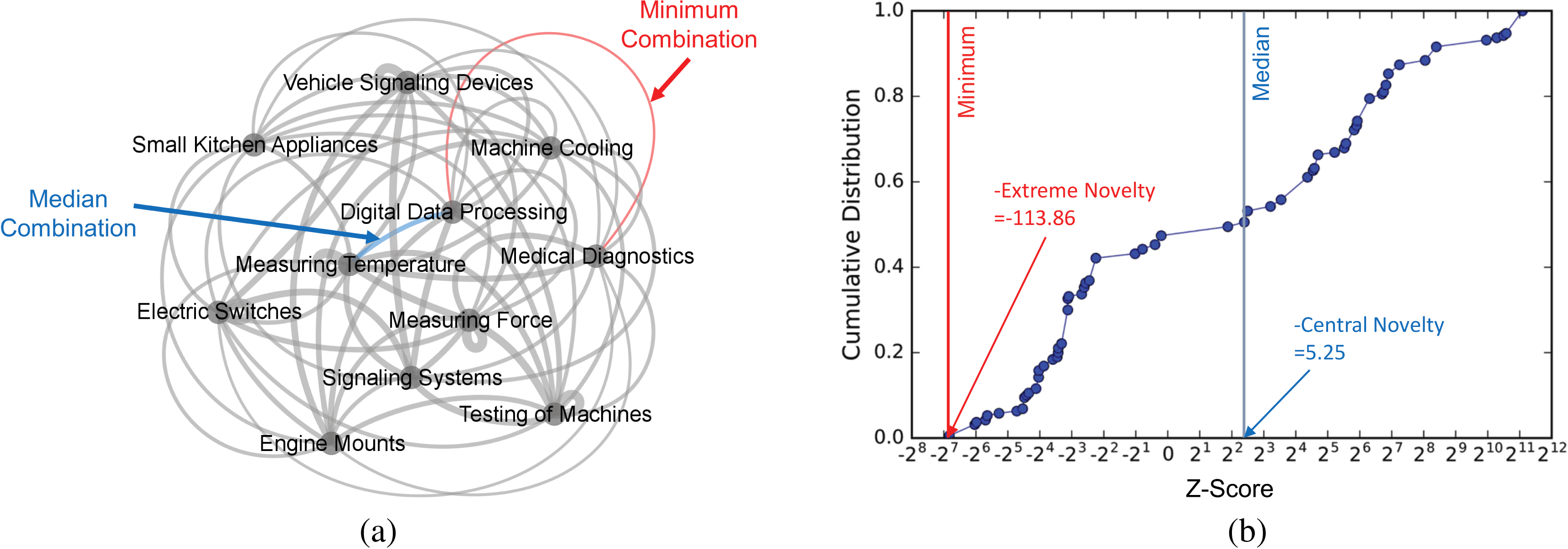
Figure 5. The technology combination space of US patent 5473937 entitled ‘Temperature sensing apparatus’. (a) Network of classes assigned to the references of the patent, connected according to the
$z$
-scores (red link, minimum
$z$
-score; blue link, median
$z$
-score). (b) Cumulative distribution of the class pairs by their
$z$
-scores.
To investigate the spectrum of novelty values, we first considered the median value of the above distribution, which is located at the center of the distribution, thus indicating the novelty in the central area of an invention’s combinations. In the world of invention, the extreme or outlier is as meaningful as the average (Fleming Reference Fleming2007; Girotra, Terwiesch & Ulrich Reference Girotra, Terwiesch and Ulrich2010). Therefore, we are also concerned with the novelty of the most novel combination in the extreme of the spectrum. In brief, to profile the novelty of an invention, we analyzed both the novelty of the center and the novelty of the extreme in the space of its combinations. Specifically, we defined and quantified the central novelty of a patented invention as the additive inverse of the median
$z$
-score in the distribution and its extreme novelty as the additive inverse of the minimum
$z$
-score in the distribution.
It should be noted that the
$z$
-scores in the distribution for a patent were calculated based on the historical data on the co-occurrence of patent class pairs until the granting year of the focal patent, because extreme novelty and central novelty are relative to the past and present artifacts and should change temporarily as newer technologies are developed over time (Weisberg Reference Weisberg2006). As a robustness check (see the Supplementary Appendix available at https://doi.org/10.1017/dsj.2017.23), we also generated the analysis results with the
$z$
-scores calculated based on the co-occurrences of patent class pairs in the granting year of the focal patent. The qualitative patterns in the main text hold.
Figure 6 shows the distributions of all patents in the 1990s according to their median
$z$
-scores and minimum
$z$
-scores. The median
$z$
-score distribution patterns changed little over the two five-year periods in the 1990s. From 1990 to 1994, 6.18% of the patents had a median
$z$
-score below 0, whereas 6.36% of the patents had a negative median
$z$
-score from 1995 to 1999. Moreover, there was no obvious change in the minimum
$z$
-score distribution over time. From 1990 to 1994, 55.58% of the patents had a minimum
$z$
-score below 0, whereas from 1995 to 1999, 59.01% of the patents did so.

Figure 6. Patent distribution according to
$z$
-scores. (a) Cumulative distribution according to median
$z$
-scores (i.e., additive inverse of central novelty). (b) Cumulative distribution according to minimum
$z$
-scores (i.e., additive inverse of extreme novelty).
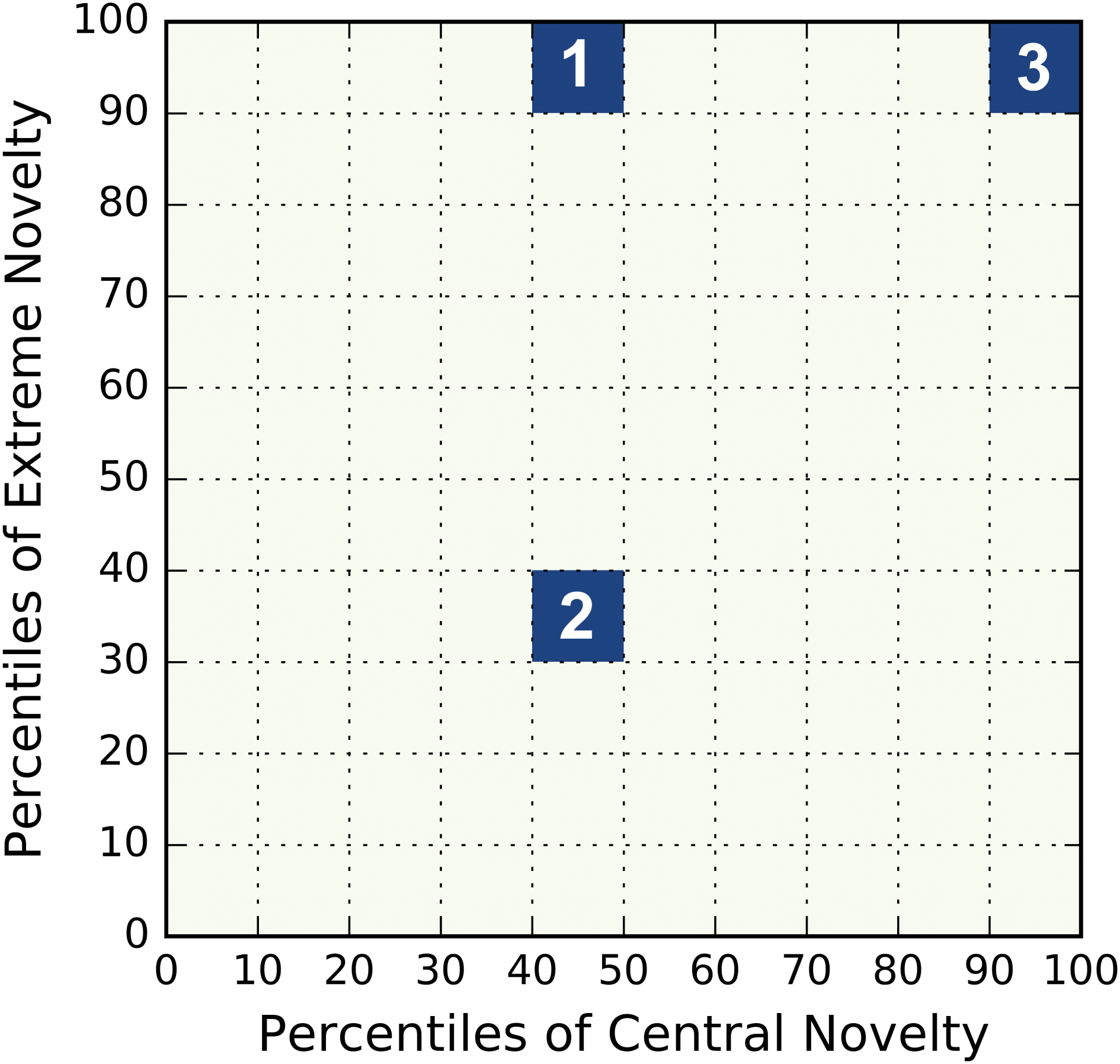
Figure 7. The central–extreme novelty space and the locations of the three patents in Table 1.
With the definition of and the method to compute both the central novelty and the extreme novelty of an invention, we can now assess and position an invention in a two-dimensional space defined by central novelty and extreme novelty (Figure 7). Because the values of central novelty and extreme novelty are highly dispersed, we divided them into 10 (equally sized) categories. A few example patents (Table 1) are located in the respective categories in the 10-by-10 matrix according to their central and extreme novelty values. These patents were all granted in 1995 but differ in their central novelty, extreme novelty, and realized invention values (to be defined below).
Table 1. Example patents of different central novelty, extreme novelty, and invention value

3.2.3 Value of a patented invention
The value of an invention is realized when it is endowed with utility and economic and social significance. Prior empirical studies have shown strong evidence that the number of a patent’s forward citations (i.e., the citations it receives after being granted) is highly correlated with the value it has achieved, as indicated by expert opinions, awards, and market value (Harhoff et al.
Reference Harhoff, Narin, Scherer and Vopel1999; Hall, Jaffe & Trajtenberg Reference Hall, Jaffe and Trajtenberg2000). For example, the patent for crystalline silicoaluminophosphates held by Union Carbide Corporation (patent #4310440) describes an important compound. With its widespread uses as a catalyst in other inventions, the patent created great economic value for its holder and received 229 citations through 1995 as the most cited patent since 1976 granted by USPTO (Hall, Jaffe & Trajtenberg Reference Hall, Jaffe and Trajtenberg2000). Thus, we followed the literature to approximate the value of a patented invention by the count of its forward citations, normalized by the average forward citation count of all of the patents granted in the same patent class and the same year. The normalization allows a comparative analysis across fields and years. The formula for the value (
$v_{i}$
) of a patented invention
$i$
is

where
$a_{i}$
denotes the total count of forward citations received by patent
$i$
and
$\bar{a}$
denotes the average count of forward citations received by all of the patents granted in the same year and in the same IPC4 class as patent
$i$
.
We were also interested in the subset of inventions that achieved outstanding value and are considered to be breakthrough inventions. In this paper, we defined the top 5% of patents in terms of the normalized forward citation count (i.e., the invention value) as ‘hit inventions’. In our analysis, the variable ‘‘hit invention’ of a patent is 1 if the patent is of a top 5% normalized forward citation and 0 otherwise. We also ran robustness tests using top 1%, 3%, and 10% as alternatives to define a hit invention (see the Supplementary Appendix).
3.2.4 Descriptive statistics
Table 2 reports the descriptive statistics for the variables based on our data set of 601,715 utility patents in the USPTO patent database that were granted in the 1990s and have five or more references to prior patents.
4 Results and discussion
We primarily analyzed the patents in our total data set to associate central and extreme novelty with invention value.
Table 2. Descriptive statistics of the key variables

4.1 Novelty sweet spot
The association between central novelty and mean invention value follows a parabola or inverted-U curve (Figure 8a). Invention value increases initially until the 60th percentile of central novelty and declines from the 60th percentile onward. The highest mean invention value appears at a ‘sweet spot’ of the 40th–60th percentiles of central novelty, i.e., a medium level of novelty in the center of their combinations. The association between extreme novelty and mean invention values follows a cubic curve moving upward (Figure 8b). The highest mean invention value appears at the highest level of extreme novelty. The associations between central or extreme novelty and hit invention rates (Figures 8c and d) follow the same patterns. These patterns are further confirmed by multivariable regression analyses (see Table S1 in the Supplementary Appendix).

Figure 8. Mean invention values and hit invention rates of patents of different central and extreme novelty percentiles. (a) Mean invention values with confidence intervals (
$\pm 1.96$
standard errors of the mean) of patents equally distributed over 10 central novelty levels. (b) Mean invention values with confidence intervals (
$\pm 1.96$
standard errors of the mean) of patents equally distributed over 10 extreme novelty levels. (c) Hit invention rates of patents equally distributed over 10 central novelty levels. (d) Hit invention rates of patents equally distributed over 10 extreme novelty levels.
Figure 9(a) shows the distribution of patents in cells of a 10-by-10 matrix by their central and extreme novelty. More patents lie along the diagonal of the central–extreme novelty matrix, implying that, to some extent, the central novelty and extreme novelty of patents are correlated. In the central–extreme novelty matrix, patents are concentrated in the regions in which the central novelty and extreme novelty are simultaneously low or high, i.e., the bottom left and upper right corners. The matrix further enables a two-dimensional comparison of the realized invention values of patents in different regions of the central–extreme novelty space.
Figure 9(b) reports the average invention value of each category of patents in the central–extreme novelty matrix. For interest in the most significant inventions, we also report the probability of achieving the top 5% invention value for patents in each category of the central–extreme novelty matrix (Figure 9c). Figures 9(b) and (c) both exhibit a similar sweet spot, i.e., the regions of medium central novelty (the 30th–60th percentiles) and high extreme novelty (the 90th–100th percentiles) in the central–extreme novelty space, where the highest mean invention values and hit invention rates are located. Notably, the value sweet spot in Figures 9(b) and (c) is away from the popular spots in Figure 9(a) that have the highest concentrations of inventions. Only 2.17% of the patents are located in the sweet spot, despite its high mean value and high rates of hit inventions.

Figure 9. Central–extreme novelty matrix. Each cell represents a category of patents according to their percentiles of central and extreme novelty. Gray indicates a lack of data. (a) Patent distribution across the space. The number in the dashed box is the sweet spot’s share of total patents. (b) Mean invention value, i.e., average normalized forward citation. (c) Hit invention rate, i.e., probability of top 5% invention value.
The realization of a high-value invention requires sufficiently but not excessively novel or conventional combinations in the center. Therefore, in the interest of maximizing invention value, there is such a thing as ‘too novel’ or ‘too conventional’, but only for the combination center of an invention. Meanwhile, a higher extreme novelty monotonically increases the value of an invention regardless of its central novelty. However, for patents with medium central novelty, an increase in extreme novelty increases the invention value more significantly than an increase at the low or high central novelty levels.
4.2 Novelty sweet spot with/without non-patent references
Many patents also cite non-patent references (NPRs) such as scientific papers, technical reports, and books, which may imply a broader scope of the combined knowledge embodied in the inventions. Prior study has shown that patents citing NPRs present a higher average value measured by forward citation counts than those citing only patents, particularly when the patented invention combines technologies from a wider scope of fields (Fleming & Sorenson Reference Fleming and Sorenson2004). Our results (Figure 10) show that patents with NPRs present generally higher invention values than patents without NPRs in every cell of the central–extreme novelty space. More specifically, the value added by NPRs, indicated by the gap between the two surfaces in each panel of Figure 10, is maximized in the sweet spot of the central–extreme novelty matrix, i.e., medium central novelty and high extreme novelty. In brief, the combination of scientific and broader knowledge beyond patentable technologies may create more valuable inventions and enlarge the value advantage of the novelty sweet spot.
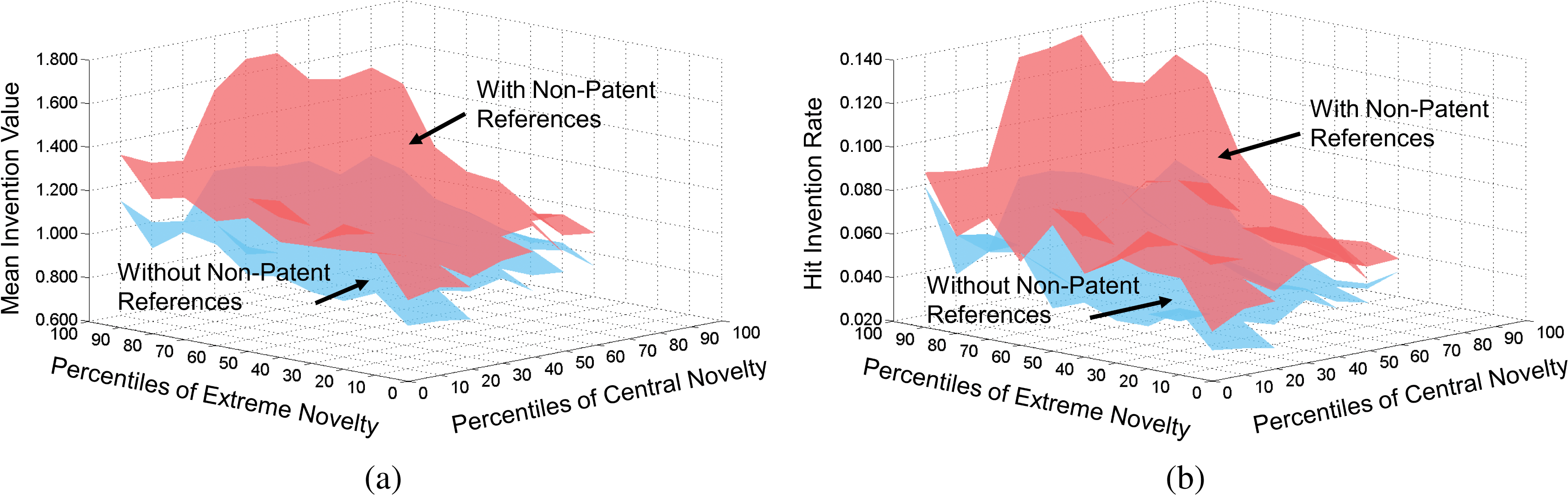
Figure 10. Novelty sweet spot with/without non-patent references (e.g., scientific papers, technical reports, books, etc.) by central and extreme novelty percentiles. Each cell in the base matrix represents a category of patents. (a) Mean invention value, i.e., average normalized forward citation. (b) Hit invention rate, i.e., probability of top 5% invention value.
The foregoing findings hold true when the data sample includes the patents with no fewer than 20, 30, and 50 reference IPC4 pairs (Figures S2–S5 in the Supplementary Appendix), when
$z$
-scores are calculated using the data of co-occurrences of class pairs only in the granting year of the focal patent (Figures S6–S9 in the Supplementary Appendix) rather than the historical data until the granting year, when extreme novelty is alternatively defined as the
$z$
-score of the 3rd, 5th, 8th, or 10th percentile of the
$z$
-score distribution of a patent (Figures S2–S4 in the Supplementary Appendix), and when we change the definition of a hit invention to one that is among the top 10%, 3%, and 1% in terms of normalized forward citation counts (Figure S5 in the Supplementary Appendix). The detailed robustness tests are reported in the Supplementary Appendix.
In brief, we have identified a clear ‘sweet spot’ of invention in the central–extreme novelty space, with a statistical analysis of approximately 600,000 patents in the USPTO database. This finding supports the prior conjecture from engineering design research (Fu et al. Reference Fu, Chan, Cagan, Kotovsky, Schunn and Wood2013). Knowledge of the specified sweet spot may further enable data-driven methods for assessing novelty and profiling inventors. It may also provide some guidance for engineering designers to enhance the value of their potential inventions. Below, we discuss several applications to make sense of this new understanding.
4.3 Potential applications
First, the central–extreme novelty matrix can be used as a data-driven tool to computationally assess the novelty of a new invention. For instance, one can assess the central novelty and extreme novelty of a new invention and locate it in the core–peripheral novelty matrix. Figure 7 has presented a few examples of individual patents positioned in the matrix. For a patented invention, the desirable situation is to lie in the sweet spot. In particular, such data-driven assessment of the novelty of a new patent application may aid in the patent validity examination.
Furthermore, the central–extreme novelty matrix can also be used to profile individual inventors, companies, states, and countries by visualizing the novelty structures of their invention portfolios. The desirable portfolio would have most patents concentrated in the sweet spot of the central–extreme novelty space; in reality, this concentration is gradual. For instance, Figure 11 presents an example that visualizes the patent portfolios of two countries, the USA and China, within the central–extreme novelty space, revealing their differences. To ensure consistency for comparison, only USPTO patents from the USA and China are analyzed here.
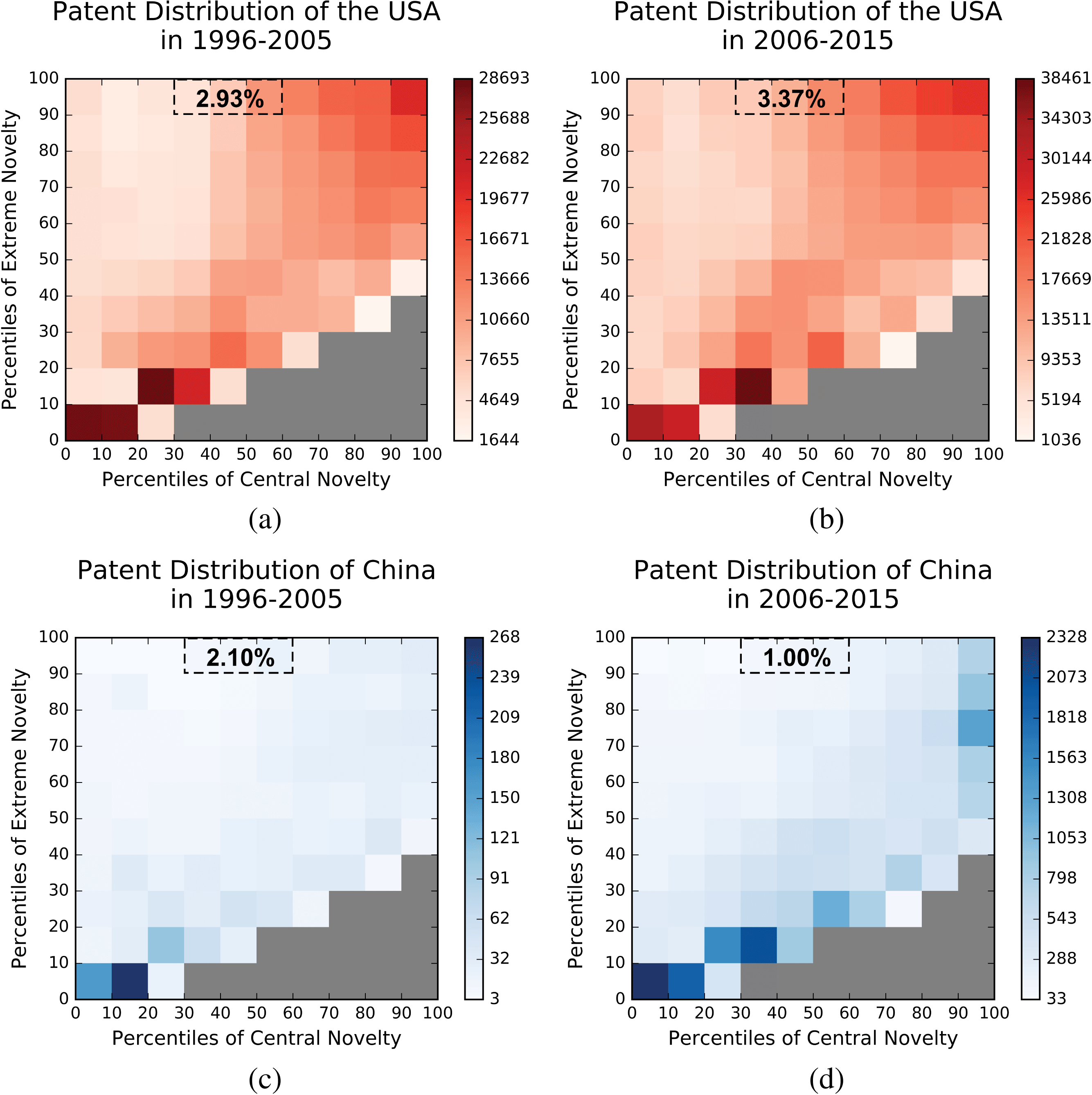
Figure 11. Patent distributions of the USA and China by central and extreme novelty percentiles. Each cell represents a category of patents. Gray indicates a lack of data. (a) Patent distribution of the USA from 1996 to 2005 and (b) from 2006 to 2015; (c) patent distribution of China from 1996 to 2005 and (d) from 2006 to 2015. The numbers in the dashed boxes are the shares of the patents in the sweet spot, i.e., the region with the 30th–60th percentiles of central novelty and the 90th–100th percentiles of extreme novelty.
The USPTO patents of the USA are concentrated around both the high (i.e., upper right) and the low (i.e., bottom left) novelty corners of the matrix and are distributed over the sweet spot. Despite an increase in total patents, this patent distribution profile has changed little over the past two decades (Figures 11a and b), and the percentage of patents within the sweet spot has increased from 2.93% to 3.37%. In contrast, the USPTO patents of China were concentrated at the low novelty corner at first but have exhibited a shift toward the upper right corner over the past two decades (Figures 11c and d), which represents both high central novelty and high extreme novelty. However, the share of the patents in the sweet spot dropped from 2.10% to 1.00%. Such a visual comparison suggests that the USA has a generally more valuable patent portfolio, with an increasing portion of patents in the sweet spot; conversely, China has been producing more novel patents over time, but is losing sight of the potential value of its patented inventions. For interested readers, the novelty profiles of additional countries can be found in the Supplementary Appendix.
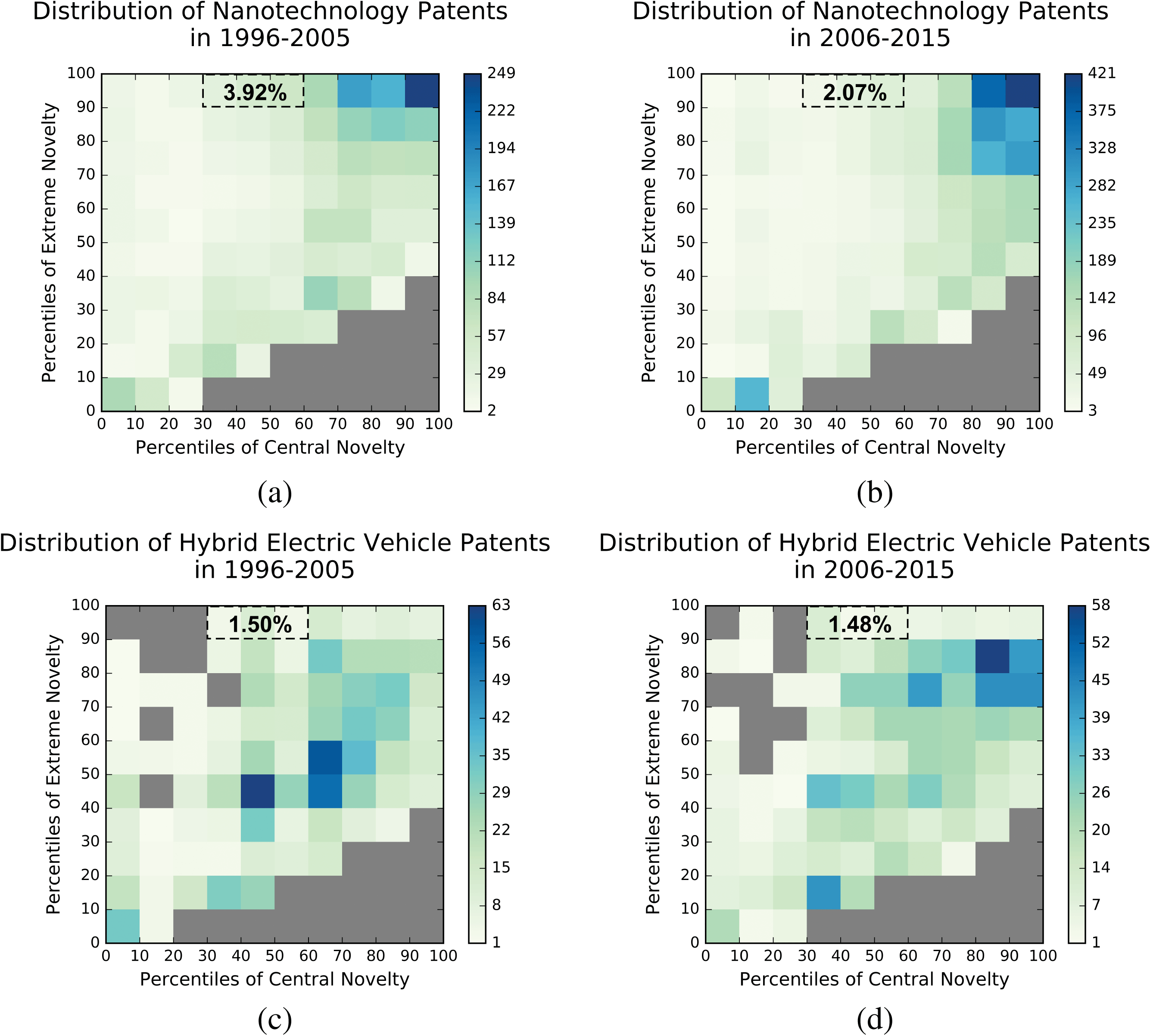
Figure 12. Patent distributions of different technology domains by central and extreme novelty percentiles. Each cell represents a category of patents. Gray indicates a lack of data. (a) Distribution of nanotechnology patents from 1996 to 2005 and (b) from 2006 to 2015; (c) distribution of hybrid electric vehicle patents from 1996 to 2005 and (d) from 2006 to 2015. The patents for the corresponding domains are extracted from the special patent categories ‘903 – Hybrid Electric Vehicles’ and ‘977 – Nanotechnology’ created by the USPTO, among nine art-collection classes whose three-digit IDs start with the number 9. The numbers in the dashed boxes are the shares of the patents in the sweet spot, i.e., the region with the 30th–60th percentiles of central novelty and the 90th–100th percentiles of extreme novelty.
Likewise, the same visual assessment can be applied to technical design domains for comparative and trend analyses. For example, Figure 12 visualizes the distributions of nanotechnology patents and hybrid electrical vehicle patents in the USPTO patent database over two decades. The nanotechnology patents are concentrated at the upper right corner of both high central and extreme novelty, with a tendency to disperse toward a lower periphery novelty over time (Figures 12a and b). The percentage of nanotechnology patents within the sweet spot dropped significantly, from 3.92% to 2.07%. In contrast, the concentration of hybrid electrical vehicle patents shifted obviously from the center of the novelty matrix to the upper right corner over time, suggesting a general increase in the portion of more novel inventions in this domain (Figures 12c and d). However, such an increase in proportion did not take place in the favorable sweet spot for value; the percentage of patents in the sweet spot was almost unchanged (from 1.50% to 1.48%). Nanotechnology inventions were generally more novel and were more present in the valuable sweet spot, whereas hybrid electrical vehicle inventions increased in the more novel categories but not in the sweet spot. Such differences in the visualized novelty profiles of domains may result from or reflect their different technical natures and development stages.
In brief, the central–extreme novelty matrix, together with the knowledge of the sweet spot in the matrix space, may enable more systematic, consistent, and efficient data-driven evaluation (of novelty and value) of inventions or new technologies than traditional approaches using subjective opinions of experts (Hennessey & Amabile Reference Hennessey and Amabile2010). Thus, it will have a broad impact on general inventive practices as well as innovation management and policy.
5 Concluding remarks
Our findings contribute to both creativity theories and inventive practices. The most important finding is a specific ‘sweet spot’ in the central–extreme novelty space. Too much or too little novelty in the center may limit the future value realization of the invention and suppress the positive value contribution of extreme novelty to an invention. To pursue hit inventions or breakthroughs, inventors should be aware of the sweet spot at the beginning of the design process. One can use sufficient but not excessive domain-specific technologies to form a moderately novel center and infuse a small number of technologies from distant domains to form a highly novel extreme in the combination space. This finding about the novelty ‘sweet spot’ is aligned with those of Fu et al. (Reference Fu, Chan, Cagan, Kotovsky, Schunn and Wood2013) and Chan et al. (Reference Chan, Dow and Schunn2015), despite different definitions of novelty, different types of experiments, and different correlation factors. Another important finding is that the combination of scientific and broader knowledge apart from patentable technologies generally creates value for an invention, and it reinforces the added value of the sweet spot over other regions in the central–extreme novelty space. This finding suggests that inventors searching broadly for scientific and non-patentable knowledge in the invention process may find more valuable inventive opportunities.
Our findings favor T-shaped inventors, who are equipped with basic scientific knowledge in various domains and deep design expertise in a specific domain. Such inventors with the T-shaped knowledge structure are less likely to be trapped by the conventional wisdoms of their domains of specialization, and can consistently explore, leverage, and engage technologies from distant domains for invention. This type of domain-crossing exploration is more effective if the inventors engage scientific and broader knowledge to comprehend and integrate technologies across domains. Our results support the movements of engineering education to cultivate holistic inventors with such a T-shaped knowledge structure to promote innovation, and deal with the growing complexity in technological inventions and the invention process (Luo & Wood Reference Luo and Wood2017).
Furthermore, we demonstrate the use of the ‘central–extreme novelty matrix’ to profile the novelty structures of the patent portfolios of different countries and of different technology domains. The visual analysis reveals that the USA had a generally more valuable patent portfolio and an increasing concentration of patents in the sweet spot, while China was losing sight of the value of inventions (i.e., it had a decreasing portion of patents in the sweet spot), despite producing more novel patents over time. We also visually found that nanotechnology inventions were highly novel but had a decreasing portion in the valuable sweet spot, whereas hybrid electrical vehicle inventions shifted their concentration to the more novel categories but not in the sweet spot over the past two decades. The novelty matrix and the knowledge of the sweet spot can be further applied to assess and compare the invention portfolios of individual inventors, companies, states, countries, and industries.
In summary, this paper contributes a scientific understanding of what novelty structure is most likely to give inventions greater value. Such an understanding is valuable for inventive practices in all fields. This paper also contributes a promising novelty evaluation tool, i.e., the central–extreme novelty matrix. It can characterize the two-dimensional novelty structures of inventions and the patent portfolios of inventors at different aggregation levels, including persons, organizations, regions, etc. This new understanding and our methodological contributions are expected to inspire and enhance creativity in design practices, engineering education, innovation management and policy, etc., across fields.
The study has limitations. For example, our method relies on a statistical analysis of the data on patent references; thus, we focused on patents with at least five references. As a result, we may have neglected highly novel patents with few references. In addition, we only analyzed direct references, although indirect references may also have implications for the combination space of invention. For co-occurrences, pair is the simplest and most generic unit of analysis. Other forms of co-occurrences, such as triples or specific topological structures, can be considered for further study to explore additional insights into novelty structures. In addition, the USPTO patent database is just one of many patent databases worldwide. It would be interesting to conduct a similar analysis using other patent databases, such as the patents filed in European, Chinese, and Japanese patent offices, to explore whether our findings in this paper will hold or vary.
This study can move forward in a few directions for future research. First, alternative measures of the novelty spectra can be explored to assess inventions based on patent data. Second, we plan to work with industrial companies and government organizations to apply our work and findings (e.g., the central–extreme novelty matrix and the ‘sweet spot’) for impact on innovation practices. Third, new studies may bring new insights into invention by using the novelty matrix to assess and compare the novelty profiles across different ranges of patents, e.g., system patents versus device patents, singular patents versus grandparent–parent–child families of patents, etc. Fourth, a data-driven invention evaluation tool can be developed to automate the novelty assessment and the visualization functions, as we preliminarily presented in this paper. Thus, laymen (e.g., engineers, managers, patent lawyers, policy makers) can use the tool to quantitatively and visually evaluate inventions and patent portfolios at different scales. Furthermore, we hope that a systematic model to predict the value of new inventions can be developed in future research by incorporating additional factors that affect invention value with central and extreme novelty.
Acknowledgments
This research is funded by SUTD-MIT International Design Centre (IDG31600105) and Singapore Ministry of Education Tier 2 Academic Research Grant (MOE2013-T2-2-167).
Supplementary data
Supplementary data is available at https://doi.org/10.1017/dsj.2017.23.