Book contents
- The Cambridge Handbook of Group Interaction Analysis
- The Cambridge Handbook of Group Interaction Analysis
- Copyright page
- Dedication
- Contents
- Figures
- Tables
- Contributors
- Editors’ Preface
- Organization of This Handbook
- How to Work with This Handbook
- Part I Background and Theory
- Part II Application Areas of Interaction Analysis
- Part III Methodology and Procedures of Interaction Analysis
- Part IV Data Analysis and Data Presentation
- Part V Coding Schemes for Interaction Research
- Appendix
- Index
- References
Part IV - Data Analysis and Data Presentation
Published online by Cambridge University Press: 19 July 2018
- The Cambridge Handbook of Group Interaction Analysis
- The Cambridge Handbook of Group Interaction Analysis
- Copyright page
- Dedication
- Contents
- Figures
- Tables
- Contributors
- Editors’ Preface
- Organization of This Handbook
- How to Work with This Handbook
- Part I Background and Theory
- Part II Application Areas of Interaction Analysis
- Part III Methodology and Procedures of Interaction Analysis
- Part IV Data Analysis and Data Presentation
- Part V Coding Schemes for Interaction Research
- Appendix
- Index
- References
Summary
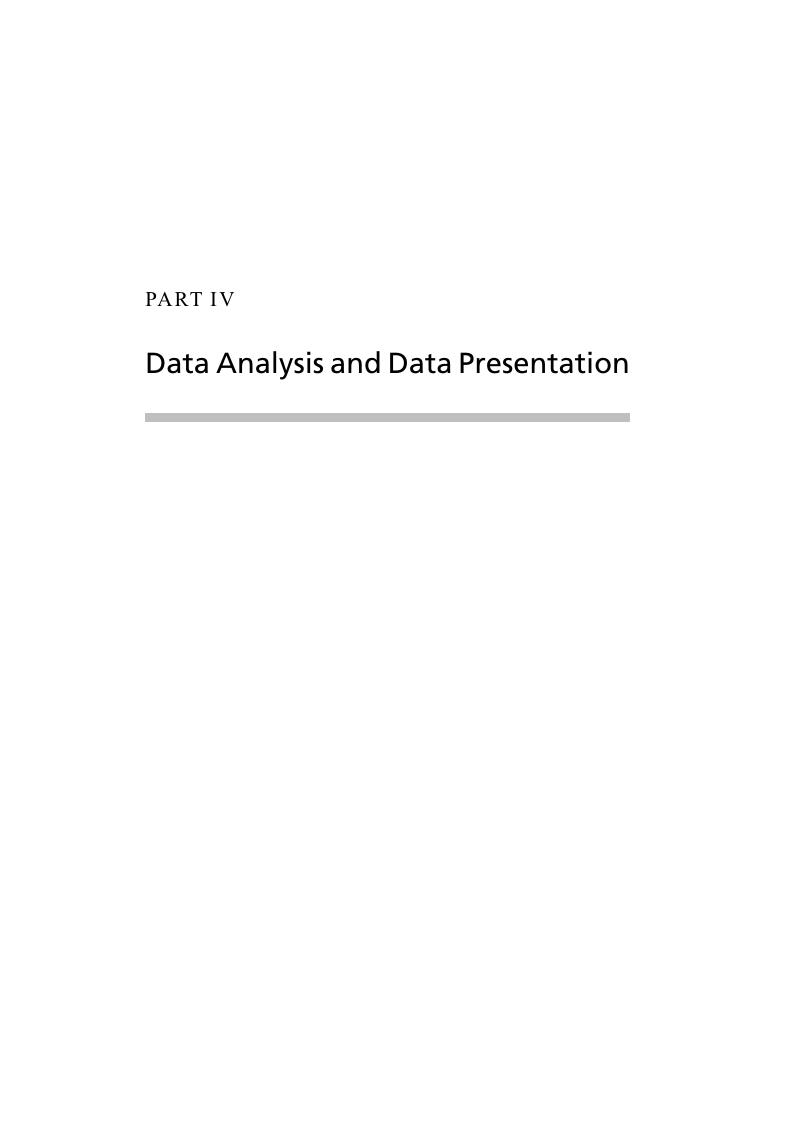
- Type
- Chapter
- Information
- The Cambridge Handbook of Group Interaction Analysis , pp. 275 - 418Publisher: Cambridge University PressPrint publication year: 2018