Article contents
Natural Sentences as Valid Units for Coded Political Texts
Published online by Cambridge University Press: 03 May 2012
Abstract
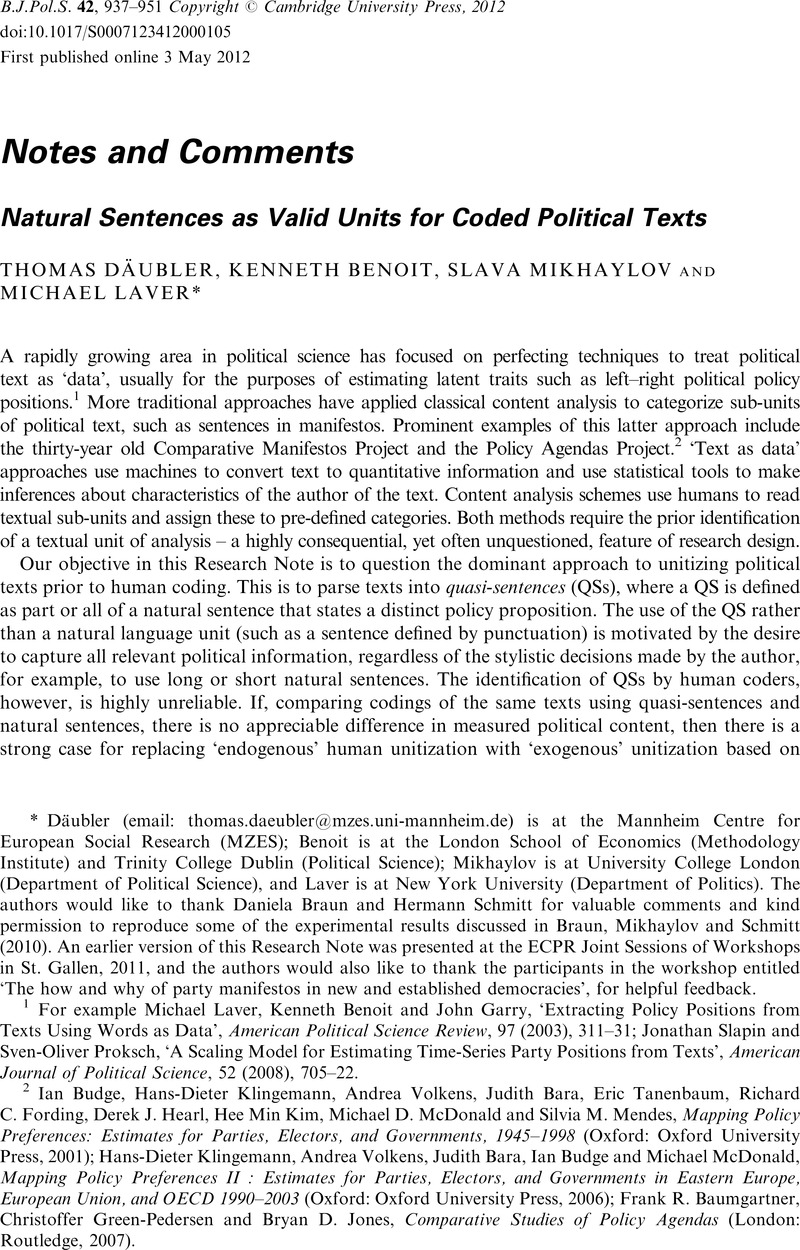
- Type
- Notes and Comments
- Information
- Copyright
- Copyright © Cambridge University Press 2012
Footnotes
Däubler (email: thomas.daeubler@mzes.uni-mannheim.de) is at the Mannheim Centre for European Social Research (MZES); Benoit is at the London School of Economics (Methodology Institute) and Trinity College Dublin (Political Science); Mikhaylov is at University College London (Department of Political Science), and Laver is at New York University (Department of Politics). The authors would like to thank Daniela Braun and Hermann Schmitt for valuable comments and kind permission to reproduce some of the experimental results discussed in Braun, Mikhaylov and Schmitt (2010). An earlier version of this Research Note was presented at the ECPR Joint Sessions of Workshops in St. Gallen, 2011, and the authors would also like to thank the participants in the workshop entitled ‘The how and why of party manifestos in new and established democracies’, for helpful feedback.
References
1 Laver, Michael, Benoit, Kenneth and Garry, John, ‘Extracting Policy Positions from Texts Using Words as Data’, American Political Science Review, 97 (2003), 311–331CrossRefGoogle Scholar
Slapin, Jonathan and Proksch, Sven-Oliver, ‘A Scaling Model for Estimating Time-Series Party Positions from Texts’, American Journal of Political Science, 52 (2008), 705–722CrossRefGoogle Scholar
2 Budge, Ian, Klingemann, Hans-Dieter, Volkens, Andrea, Bara, Judith, Tanenbaum, Eric, Fording, Richard C., Hearl, Derek J., Kim, Hee Min, McDonald, Michael D. and Mendes, Silvia M., Mapping Policy Preferences: Estimates for Parties, Electors, and Governments, 1945–1998 (Oxford: Oxford University Press, 2001)CrossRefGoogle Scholar
Klingemann, Hans-Dieter, Volkens, Andrea, Bara, Judith, Budge, Ian and McDonald, Michael, Mapping Policy Preferences II : Estimates for Parties, Electors, and Governments in Eastern Europe, European Union, and OECD 1990–2003 (Oxford: Oxford University Press, 2006)CrossRefGoogle Scholar
Baumgartner, Frank R., Green-Pedersen, Christoffer and Jones, Bryan D., Comparative Studies of Policy Agendas (London: Routledge, 2007)Google Scholar
3 Krippendorff, Klaus, Content Analysis: An Introduction to its Methodology (Thousand Oaks, Calif.: Sage, 2004)Google Scholar
4 By contrast, the second basic data-generating step, in which each text unit is coded by assigning to it a category from the coding scheme, is always endogenous to the text, and indeed forms the core part of the content analysis exercise.
5 Monroe, Burt L. and Maeda, Ko, Rhetorical Ideal Point Estimation: Mapping Legislative Speech (Palo Alto, Calif.: Stanford University: Society for Political Methodology, 2004)Google Scholar
Monroe, Burt, Colaresi, Michael and Quinn, Kevin M., ‘Fightin’ Words: Lexical Feature Selection and Evaluation for Identifying the Content of Political Conflict’, Political Analysis, 16 (2008), 372–403CrossRefGoogle Scholar
Hopkins, Daniel J. and King, Gary, ‘A Method of Automated Nonparametric Content Analysis for Social Science’, American Journal of Political Science, 54 (2009), 229–247CrossRefGoogle Scholar
6 Details for those unfamiliar with the Jeopardy game show format, or with Watson and DeepQA, can be found at http://www-03.ibm.com/innovation/us/watson/.
7 Krippendorff, Content Analysis: An Introduction to its Methodology, p. 212.
8 Laver, Michael and Garry, John, ‘Estimating Policy Positions from Political Texts’, American Journal of Political Science, 44 (2000), 619–634CrossRefGoogle Scholar
9 See, for instance, Klingemann et al., Mapping Policy Preferences II, chaps 4–6.
10 Budge et al., Mapping Policy Preferences; Baumgartner, Green-Pedersen and Jones, Comparative Studies of Policy Agendas.
11 Andrea Volkens, Manifesto Coding Instructions (2nd revised edn), Wissenschaftszentrum Berlin, Discussion Paper FS III 02-201 (2001), p. 96.
12 Krippendorff, Content Analysis.
13 To return to the Australian 2001 National party example cited earlier, we also observe the following natural sentence: ‘There is no argument about the need for production sustainability and its matching twin, environmental sustainability.’ In this case, the coder deemed this a single QS and coded it as 501 (Environmental Protection: Positive), even though it could plausibly have been seen as comprising two QSs, divided by the ‘and’, with the first coded to 410 (Productivity: Positive) and the second to 501.
14 In the test results, coders with especially bad first round results had these corrected, and were asked to repeat the experiment. Here we report only the second-round unitization results for coders asked to repeat the test. While these results are not a decisive experiment, given that it is part of a training process of new coders, they are the single largest test of multiple unitizations of a manifesto text available. We thank Andrea Volkens for sharing this data with us.
15 Krippendorff, Content Analysis.
16 Laver, Michaeled., Estimating the Policy Positions of Political Actors (New York: Routledge, 2001), pp. 149–161Google Scholar
17 We are assuming here that differences at the unit level are not the quantity of interest, and that the objective of any unit-based coding exercise is to yield aggregate measures of political content.
18 To make the identification of natural sentences as unambiguous as possible, with a view to eventually automating this stage completely, we developed a very explicit set of guidelines as to how to identify a natural sentence. A natural sentence delimiter was defined as the following characters: ‘.’, ‘?’, ‘!’, and ‘;’. Bullet-pointed sentence fragments were also defined to be ‘natural’ sentences, even if not ending in one of the five previously declared delimiters. A full set of the coding instructions we issued to coders (ourselves) is available upon request.
19 Mikhaylov, Slava, Laver, Michael and Benoit, Kenneth, ‘Coder Reliability and Misclassification in the Human Coding of Party Manifestos’, Political Analysis, 20 (2012), 78–91CrossRefGoogle Scholar
20 To be precise, the number of categories is 57 since it includes ‘uncoded’ as a further category, as is the case in the published CMP data. We did not use the four-digit codes that apply to post-communist countries but aggregated them to their respective three-digit category. However, this affected only 7 out of the total 8,481 (0.08%) QSs. In addition, 24 QS codes (0.28%) could not be identified from the documents since they were not legible.
21 The emphasis here is on ‘reconstructed’: we did not ensure that every category percentage from the QS we recorded perfectly matched those reported in the CMP's dataset. An exact replication is not possible, for instance because it appears (not that rarely) that the number of codes on the margins does not correspond to the number of units separated by tick marks (if they are used at all). While we did check that we matched the published figures to a very high degree, a perfect matching is unnecessary since our comparison focuses on units within texts.
22 Lowe, Will, Benoit, Kenneth, Mikhaylov, Slava and Laver, Michael, ‘Scaling Policy Preferences from Coded Political Texts’, Legislative Studies Quarterly, 36 (2011), 123–155CrossRefGoogle Scholar
23 Daniela Braun, Slava Mikhaylov and Hermann Schmitt, ‘Computer-Assisted Human Coding: Experimental Tests of Reliability’ (paper presented at ‘Political Parties and Comparative Policy Agendas’, an ESF Workshop on Political Parties and their Positions, and Policy Agendas, University of Manchester, 2010).
24 Mikhaylov, Slava, Laver, Michael and Benoit, Kenneth, ‘Coder Reliability and Misclassification in the Human Coding of Party Manifestos’, Political Analysis, 20 (2012), 78–91CrossRefGoogle Scholar
25 The excerpt of the 1999 British Liberal Democrats Euromanifesto used in the experiment consists of 83 natural sentences. The Euromanifestos Project previously used this excerpt as the training document and declared it to consist of 112 QSs.
26 Budge et al., Mapping Policy Preferences.
27 As part of the coding procedure, coders coded policy domains (the seven categories defined by the first digit of the CMP code) and coding categories sequentially.
28 Braun, Mikhaylov and Schmitt, ‘Computer-Assisted Human Coding’.
29 Fleiss, Joseph L., ‘Measuring Nominal Scale Agreement among Many Raters’, Psychological Bulletin, 76 (1971), 378–383CrossRefGoogle Scholar
Fleiss, Joseph L., Levin, Bruce A. and Paik, Myunghee Cho, Statistical Methods for Rates and Proportions (Hoboken, N.J.: Wiley, 2003)CrossRefGoogle Scholar
30 Roberts, Chris, ‘Modelling Patterns of Agreement for Nominal Scales’, Statistics in Medicine, 27 (2008), 810–830CrossRefGoogle ScholarPubMed
31 Jacob Cohen (‘A Coefficient of Agreement for Nominal Scales’, Educational and Psychological Measurement, 20 (1960), 37–46)CrossRefGoogle Scholar
Hayes, Andrew F. and Krippendorff, Klaus, ‘Answering the Call for a Standard Reliability Measure for Coding Data’, Communication Methods and Measures, 1 (2007), 77–89CrossRefGoogle Scholar
32 Landis, J. Richard and Koch, Gary G., ‘The Measurement of Observer Agreement for Categorical Data’, Biometrics, 33 (1977), 159–174CrossRefGoogle ScholarPubMed
33 Mikhaylov, Laver and Benoit, ‘Coder Reliability and Misclassification in the Human Coding of Party Manifestos’.
- 39
- Cited by