INTRODUCTION
Evaluation of vaccine safety is an important aspect of any vaccination programme [Reference Farrington1]. Although vaccines are tested extensively for relatively common adverse events in clinical trials before they are licensed for use, not enough people are usually included in such trials to detect adverse reactions that occur only rarely. Post-licensure studies also enable the evaluation of vaccine safety within groups such as the elderly, those with chronic medical conditions, and pregnant women, who might be deliberately excluded from clinical vaccine trials. Finally, by providing accumulating evidence of safety, they can help to maintain the public confidence needed to keep vaccination uptake high enough to prevent disease outbreaks.
Cohort and case-control study designs are commonly used to investigate vaccine safety, but confounding by variables related both to avoidance of vaccination and to the outcome of interest is a potential problem [Reference Farrington, Nash and Miller2]. An alternative study design, the self-controlled case-series (SCCS) method, often combines the power and simplicity of the cohort design and the economy of the case-control method, while eliminating confounding by all time-independent variables [Reference Farrington3, Reference Farrington and Whitaker4]. This method, which uses only information from cases, i.e. individuals with an adverse event, was developed specifically for use in vaccine safety studies, but has since been applied in non-vaccine pharmacoepidemiology and in other areas of epidemiology [Reference Whitaker5]. The method is briefly described at the end of this section.
The purpose of this paper is to review how the SCCS method has been used in vaccine studies since its publication in 1995. Our aim is to highlight good practice, and, based on experience accumulated over the past 15 years, to attempt to give some clear direction on how the method should be used and reported. We also seek, briefly, to clarify some misconceptions about the SCCS method and how it relates to other case-only study designs. Our aims, however, fall short of developing fully-fledged guidelines on reporting SCCS studies, which require detailed consideration of other applications in pharmacoepidemiology. Nevertheless, we hope that this review will contribute towards the eventual elaboration of such guidelines.
The paper has four sections. In section 2 our review criteria and methods are described. In section 3 we present the results of our review, including specific discussion on: data description and accuracy, choice of observation and risk periods, potential biases, comparison of SCCS with other methods such as cohort and case-control, methodological issues, sensitivity analyses, software and good practice. Where appropriate, we also include general comments about the method and make recommendations. Section 4 is a brief discussion of our findings and areas for further research on the SCCS method. First, we set the scene with a brief description of the SCCS method.
The SCCS method
The SCCS method was originally developed to estimate the relative incidence of an acute event in a pre-defined post-vaccination risk period, compared to other times, which constitute the control period [Reference Farrington3]. It is applied as follows.
An overall study time-window, usually defined by age and calendar time boundaries (but also, sometimes, in terms of vaccination date), is chosen, ideally such that the chance that individuals experience both risk and control periods is maximized. Then, all or a random sample of individuals with at least one event (independent recurrences are permitted) within this study time-window are identified: these are the cases. The study time-window also determines individual observation periods for each case, namely the time spent by each individual within the study time-window (the observation periods generally differ between individuals). Next, the vaccination histories of the cases are collected: as in other epidemiological designs, ascertainment of cases must be independent of vaccination histories. The vaccination dates of each case are used to define one or more risk periods, during which individuals are hypothesized to be at increased (or reduced) risk of the event of interest after (or, for reasons to be discussed later, before) vaccination. All other time within an individual's observation period, that does not fall within a risk period, is included in that individual's control period, which forms the study baseline.
So far, the description of the method is in many ways similar to that of a risk-interval cohort study, i.e. a cohort study in which individuals experience successive ‘at risk’ periods and ‘not at risk’ or control periods over a defined follow-up period. Typically, in such a study, the cohort is recreated retrospectively from event and exposure data stored in an electronic database. The big difference with the SCCS method is that only the cases from this retrospective risk-interval cohort study need be sampled or available. Justification for using only cases stems from the analytical strategy, which treats as fixed the number of events each individual experiences within the observation period: this is called conditioning on the number of events. This may be explained heuristically as follows. Having fixed the total number of events an individual has experienced over the observation period, and the risk and control intervals that each individual has progressed through (both before and after experiencing any events), the only quantity that remains undetermined is the interval or intervals (risk period or control) in which that individual's event or events actually occurred. Thus, in a SCCS analysis, only this information contributes to the estimation of the vaccine effect (whereas in a standard cohort study, the number of events experienced by each individual also contributes to the estimation). If an individual in the cohort happens to have experienced zero events, then there is no information on when events occurred, and so that individual contributes nothing to the estimation. Thus, a consequence of the conditioning is that non-cases contribute no information, and therefore need not be sampled.
A further consequence of the conditioning described above (whereby the number of events experienced by each individual is regarded as fixed) is that the analysis is within-individuals and, as a result, in the SCCS method all fixed confounding factors, known and unknown, are controlled for implicitly. To see this, recall that the only information used in the estimation relates to when events occurred, within each individual's observation period. Since the possible event times are all chosen from that same individual's history, the estimation is unaffected by confounding factors that multiply the event rate by a quantity that remains fixed over time. In this sense, the estimation is within-individuals, and controls for fixed confounders. Time-varying confounding factors, such as age, can be accounted for by subdividing each individual's observation period into age categories, which are modelled explicitly. Alternatively, a semi-parametric approach, for which the age groups do not need to be specified, is available, although it is not suitable for large studies [Reference Farrington and Whitaker4].
Estimation of parameters in the SCCS method is most readily achieved by fitting a conditional Poisson regression model (it is essential that it should be a conditional model, in order to justify sampling only cases). The parameter of interest is the relative incidence, i.e. the incidence in a risk period relative to the control or baseline periods. A tutorial is available with full practical details and worked examples [Reference Whitaker5].
Three key assumptions are made by the SCCS method: these are (1) that events are recurrent and independent, or are unique and uncommon over the observation periods; (2) that the occurrence of an event must not alter the probability of subsequent exposure; and (3) that the occurrence of the event of interest must not censor or affect the observation period [Reference Farrington3–Reference Whitaker, Hocine and Farrington6]. Elaborations of the method to weaken these assumptions are mentioned in the Discussion.
METHODS
We identified SCCS studies which included a vaccine as an exposure, first published (in print or electronically) between 1995, when the SCCS method was first introduced, and end of 2010. We identified papers by searching for those citing references [Reference Farrington1–Reference Musonda, Farrington and Whitaker8] in the following databases: Scopus, JSTOR, Science Direct, British Library and all those within the ISI Web of Knowledge.
We excluded all methodological papers published in statistics journals. We also excluded methodological papers published in epidemiology journals, unless they included a specific application using SCCS not reported elsewhere, and sufficient detail of this application was provided.
Each paper was reviewed against a standard form which was piloted on 13 papers. The form included details on: vaccines and adverse events studied, data collection and description, study population, sample size, observation period, age groups, the allowance for any other temporal confounders, risk periods and their rationale, sensitivity analyses undertaken, statistical features, reporting of results, whether key SCCS assumptions were met, any good, bad or unusual practice, and comparison with other study methods used in addition to SCCS.
RESULTS
We identified 40 studies which met our selection criteria [Reference Ali9–Reference Zinman48]. Four of these [Reference Ali9, Reference Burwen14, Reference Farrington17, Reference Gold20] were papers with a methodological flavour, aimed at validating a surveillance system, but including a specific SCCS application. There were three notable exclusions. The first planned to use the SCCS method to study a possible association between vaccination and acute cerebellar ataxia [Reference van der Maas49]. However, that analysis was not undertaken owing to sparseness of the data, and for this reason we excluded the paper. Two further papers [Reference France50, Reference Klein51] were excluded because, while referencing the SCCS literature, it was not clear that they intended to use it, and instead used a ‘before and after vaccination’ design. As it turns out, this is in fact a special case of the SCCS design; we shall return to this issue later in the paper. The papers were excluded because the authors could not be expected to report the study as if it were a SCCS study.
Figure 1 presents the distribution by year of publication of these 40 studies (references [Reference Andrews10] and [Reference Sardinas38] appeared in 2002, even though the journals are dated 2001; reference [Reference Gwini, Coupland and Siriwardena21] was published electronically in 2010). Thirty-eight of the 40 papers appeared in 2000–2010; Figure 1 suggests a moderate increase over this period. All but three studies were undertaken in high median-income countries; these three studies were undertaken in Vietnam [Reference Ali9], Brazil [Reference Dourado16] and Cuba [Reference Sardinas38].
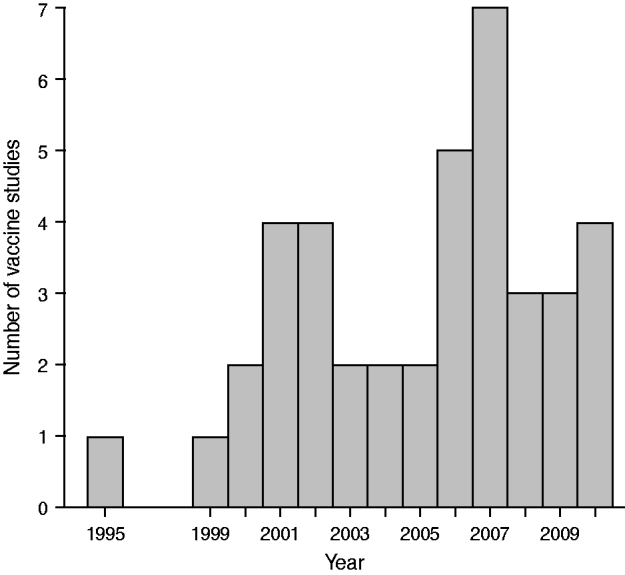
Fig. 1. Distribution of vaccine studies using self-controlled case-series by year of publication.
Vaccines and adverse events studied
Table 1 presents the vaccines and the adverse events studied. For ease of presentation, adverse events have been grouped, as for example purpura (which includes idiopathic thrombocytopenic purpura (ITP), allergic purpura, and other purpura). Similarly, vaccine types (e.g. intranasal and parenteral influenza vaccines) have been listed under the same heading. Measles/mumps/rubella (MMR) and other measles-containing vaccines were the most frequently studied (17 studies), followed by influenza vaccines (13 studies) and vaccines containing pertussis antigens (eight studies). The sample sizes (cases or numbers of events) included in SCCS analyses ranged from the very small (only seven events in one analysis in [Reference Farrington17]) to the very large (8180 cases in [Reference Gwini, Coupland and Siriwardena21], 22 400 in [Reference Smeeth39]).
Table 1. Vaccines and adverse events studied
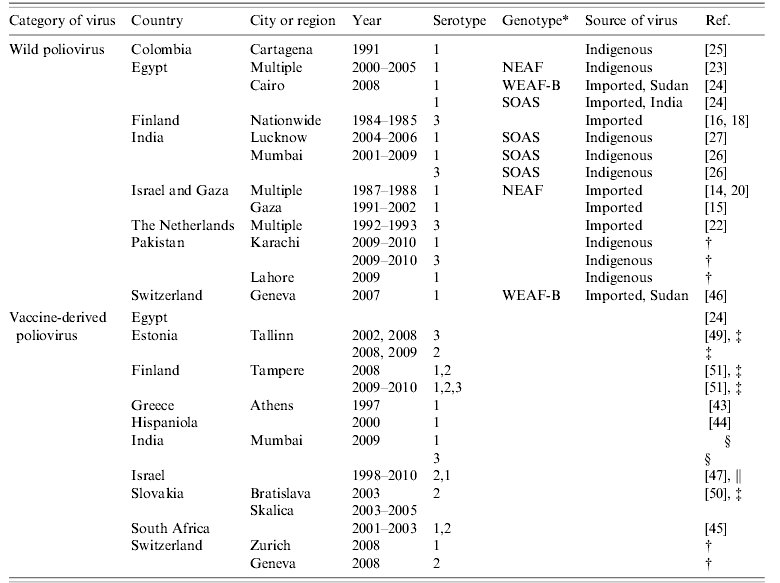
COPD, Chronic obstructive pulmonary disease; DT, diphtheria/tetanus vaccine; DTaP, diphtheria/tetanus/acellular pertussis vaccine; DTP, diphtheria/tetanus/pertussis vaccine; HBV hepatitis B virus vaccine; Hib, Haemophilus influenzae type b vaccine; IPV, inactivated poliovirus vaccine; ITP, idiopathic thrombocytopenic purpura; MCCV, meningococcal group C conjugate vaccine; MMR, measles/mumps/rubella vaccine; OPV, oral poliovirus vaccine; Td, tetanus/diphtheria booster vaccine; UTI, urinary tract infection.
Typically, several vaccines and/or adverse events were studied at the same time. One study [Reference Payne37] investigated concurrency of vaccination (administration of at least two vaccines on the same or adjacent days) as a risk factor. When several vaccines potentially related to the same outcome are administered at similar ages, their effects should be studied within the same model, as was done in [Reference Andrews12]. This also applies to non-vaccine exposures, as with influenza vaccination and influenza-like illness in Guillain–Barré syndrome (GBS) [Reference Stowe42]. In 22 studies, vaccines were given in multiple doses; in 12 of these, dose-specific effects were investigated.
The SCCS method can only cope with a single outcome variable at a time. The most frequently studied events were convulsion (including febrile convulsion and aseptic meningitis) and purpura (six studies each). There were four studies of intussusception, and three each of autism and GBS.
Data description and data accuracy
In common with other epidemiological methods, it is essential that ascertainment of events should be independent of vaccination history; in SCCS studies, this also applies to the timing of events in relation to vaccination. A clear description of how the data were obtained is therefore important in order for the reader to be able to assess any possible dependence. All 40 studies were felt to provide sufficient detail in this respect. Sixteen obtained data on vaccinations and outcomes from a single database [of these, seven studies used the United Kingdom's General Practice Research Database (GPRD) and six the United States' Vaccine Safety Datalink], 14 linked two or more databases, and in 10 data were obtained from other sources.
Case-note reviews were undertaken in 18 studies, and in two of these the review was commendably reported as blinded to vaccine history. In one study [Reference Andrews12], case notes were used to identify vaccinations. This may bias results towards a positive association, in as much as vaccinations prior to the event are more likely to be ascertained by case-note review than vaccinations after the event. However, in this study the association was not significant, and so the ascertainment procedure in this instance leant further weight to the conclusions reached.
Most studies had full information on the day of vaccination and the day of event (studies [Reference Farrington, Miller and Taylor18] and [Reference Taylor45] used month as the time unit for analysis, but with long risk periods). In [Reference Burwen14] and [Reference Dourado16], dates of vaccination were imputed rather than observed exactly. The sensitivity to imputation errors depends on the lengths of the risk periods used, and it would be advisable to study this by sensitivity analyses, although none were reported. In [Reference Andrews13], vaccination dates were known exactly, but the types of vaccines used at different times were derived indirectly.
Observation periods and risk periods
A well-conducted SCCS study requires great rigour in the definition of observation periods and risk periods for each case. The observation period, in particular, must be defined so that, had an event occurred at any point within it, the case would have been ascertained. Often, the observation period is determined by a combination of calendar time and age constraints, defined precisely in the time units of the study. Risk periods are defined in terms of time since vaccination (with, preferably, a stated convention to describe the day of vaccination, for example day 0). A similarly rigorous report of these choices provides confidence that care was taken in the analysis, and enables the reader, in theory at least, to reconstruct the study exactly.
In all 40 studies, observation periods were defined with sufficient detail to reconstruct the study. The idiosyncrasies of specific databases need to be allowed for appropriately in defining observation periods. Thus, some studies excluded day of vaccination (or allocated it a special parameter) owing to the fact that, in some information systems, past events are retrospectively recorded on day of vaccination; left uncorrected, this would induce spurious associations on day of vaccination. This effect is illustrated graphically in marked fashion in [Reference Tata44]. In one study in the GPRD [Reference Andrews13], events on day of vaccination were validated by case-note review.
The risk periods were defined explicitly in all 40 studies. The choice of risk periods should be made a priori and its rationale explained. Typically, the choice will be motivated by reference to previous studies or hypotheses, as in [Reference France19] for example; by biologically plausible mechanisms [Reference Farrington17]; or by expert opinion [Reference Miller30]. Different risk periods may sometimes reflect different scientific questions. For example, in [Reference Farrington17], the 6- to 11-day risk period post-MMR was chosen to capture febrile convulsions associated with the measles component of the vaccine, while the 15- to 35-day risk period was chosen to capture convulsions associated with the mumps component. Inevitably, in some circumstances the risk period is not known, and so the choice is arbitrary; if so this should be stated [Reference Farrington, Miller and Taylor18]. Three studies [Reference Farrington, Miller and Taylor18, Reference Hocine23, Reference Kramarz28] used indefinite post-vaccination risk periods. In several studies (e.g. [Reference Juurlink26]) a sensitivity analysis was undertaken by varying the risk period. A further approach is to use several adjacent risk periods in the same analysis. For example, to investigate seizures and acellular pertussis vaccines, study [Reference Huang24] used the risk periods 0 days (i.e. day of vaccination) and 1–3 days after vaccination. When results are similar across risk periods, or when data are lacking, contiguous risk periods can be combined.
When a relatively long risk period is used, it is advisable to undertake secondary analyses to identify clustering or otherwise of cases within that risk period. Examples include [Reference Miller32], where a clustering of ITP cases was found 15–28 days within the 0- to 42-day risk period studied, and [Reference Farrington, Miller and Taylor18], where no clustering of autism cases was found in adjacent 2-year intervals within the unlimited post-MMR risk period.
Confounders
SCCS studies adjust automatically for time-invariant multiplicative confounders. However, effect modification by fixed covariates can be investigated through interactions with the vaccine effect: for example in [Reference Gwini, Coupland and Siriwardena21] such effects were investigated, for sex and age at start of observation.
The SCCS method, in common with other epidemiological methods, is prone to bias from uncontrolled age- or time-varying confounders. In vaccine studies, particularly those undertaken in children, age (or in some cases season, or both) is likely to be the major confounder, and should, as a rule, be adjusted for in the analysis, unless observation periods are extremely short. Seven studies did not report using any kind of temporal adjustment; in four of these, the observation period was less than a year. Of the remaining 33 studies, 19 adjusted for age only, three for season only, one for calendar time only, six for age and season, one for age and calendar time, and two for age, season and calendar time (e.g. [Reference Huang24]).
Only one study [Reference Hocine23] used the semi-parametric model [Reference Farrington and Whitaker4], in which it is not necessary to specify age classes. If a parametric method of age adjustment is used, it is good practice to check that the age model used is adequate, by varying the number of age classes used. Two studies reported such sensitivity analyses [Reference Hocine23, Reference Smeeth39]. One study [Reference Hughes25] controlled for age as a continuous covariate, although no details of how this was achieved were given; such a method of control is not straightforward owing to the conditioning (see further details under the Software for SCCS analyses subsection).
Control for age-varying or time-varying confounders other than age or season require the confounder to be measured over time. For example, in an analysis of influenza vaccine and GBS [Reference Stowe42], the authors controlled for the possible confounding effect of influenza-like illness. However, it is often impractical to measure time-varying confounders. For example, the healthy vaccine effect is a form of confounding by an unmeasured time-varying factor. This affects SCCS studies as well as other study designs. The potential impact of such bias therefore requires careful discussion.
Discussion of potential biases
The three key assumptions of the the SCCS method listed in the Introduction should be checked, as far as possible, and discussed. We consider these three assumptions in turn.
Assumption (1):
that the events are either recurrent and independent within individuals, or non-recurrent and uncommon, is not usually problematic. For recurrent events, sensitivity to the independence assumption can readily be tested by restricting the analysis to first events, provided these are uncommon in the population considered; see [Reference Farrington and Whitaker4, Reference Whitaker5] for an example with MMR and ITP. More complex approaches to correcting for non-independence of recurrent events are discussed in [Reference Farrington and Hocine52]. Simulations studies in [Reference Farrington53] show that the bias is negligible when the risk that an unvaccinated individual will experience an event over the observation period is under 10%. Most adverse events of interest in post-licensure studies are much less common that this.
Assumption (2):
that the event should not affect the subsequent probability of vaccination, is perhaps the most important for vaccine studies. This assumption fails if the event is a contra-indication for vaccination (as with intussusception and rotavirus vaccination since the publication of [Reference Murphy34]), or if vaccination after the event is more or less likely (as with GBS and influenza vaccination). A third possibility is that vaccination is deferred after (or more rarely, precipitated by) an event, so that the impact of the event on vaccination is short-lived. Nevertheless, an important feature of such biases is that their direction is predictable: if the event reduces the probability of subsequent vaccination, then the relative incidence associated with vaccination will be biased upwards. This is because vaccinations are then less likely to arise after the event.
There are three main ways of coping with such bias: including pre-vaccination ‘risk’ periods to allow for short-term deferral of vaccination (or indeed to investigate the presence of longer term effects); exclusion of all pre-vaccination time (so that the observation period begins with vaccination), which works provided the vaccine can only be given at most once during the projected observation period; and the use of more complex analytic techniques [Reference Farrington, Whitaker and Hocine54]. Of the studies reviewed, 16 used pre-vaccination ‘risk’ periods (see, e.g. [Reference Andrews10] and [Reference Sardinas38]), and three [Reference Juurlink26, Reference Smeeth39, Reference Zinman48] started observation at vaccination for some analyses.
Assumption (3):
that the observation periods are not event-dependent, may be violated, for example, if events increase short-term mortality, or the event of interest is death. This was not an issue in any of the 40 studies reviewed. SCCS methods for dealing with such situations are discussed in [Reference Farrington53–Reference Kuhnert55].
Comparisons with other statistical methods
In addition to implementing the SCCS method, 12 studies used or reported results obtained on the same data using other study designs. These included cohort, case-control, and ecological methods. The different methods should produce the same results, provided that all confounding has been controlled and that the assumptions required are met. Using several methods of analysis is recommended, as it can reinforce conclusions or shed light on possible sources of bias, when these differ for different study designs. Table 2 presents the results obtained using SCCS and other methods, for a selection of analyses.
Table 2. Selected relative incidence (RI) estimates from self-controlled case series method and RI or odds ratio (OR) from other study designs applied to the same case data, and 95% confidence interval (CI)
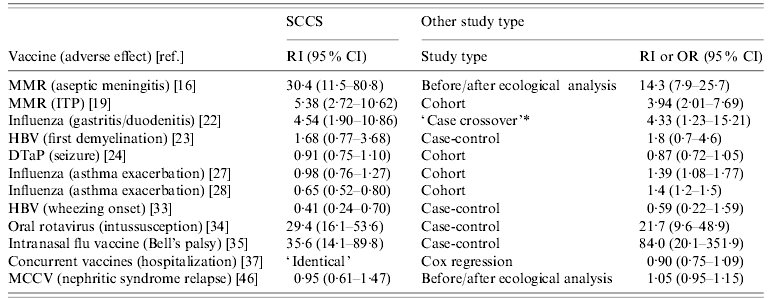
For abbreviations see Table 1 note.
* This description is incorrect: it is actually another SCCS (see text).
The results obtained using SCCS were broadly similar to those obtained by other methods, with the exception of studies of influenza vaccine and asthma exacerbation [Reference Kramarz27, Reference Kramarz28] where the SCCS method found a protective or null effect, but a cohort analysis found a positive association. The most likely explanation for this discrepancy is residual indication bias in the cohort study, children with more severe asthma being more likely to receive influenza vaccine. In the cohort study, underlying asthma severity was quantified using available proxy variables; self-control in the SCCS study was arguably more effective in correcting for indication bias. More generally, the results of a SCCS study should be unaffected by unmeasured or incompletely controlled confounders, and in this sense ought to be more reliable, provided that the assumptions of the method are satisfied.
In a study of hepatitis B vaccine (HBV) and wheezing onset [Reference Mullooly33], the point estimates from SCCS and a case-control study were of the same order, but the greater precision of the SCCS method in this case produced a statistically significant effect. The better precision of the SCCS method was also noted in another study of HBV [Reference Hocine23], where it was pointed out that some cases cannot be used in matched case-control studies owing to lack of matching controls; the SCCS method does not suffer from this problem.
In one study [Reference Hambidge22] the alternative method was incorrectly described as a case-crossover design, when in fact it was another SCCS with a ‘before and after vaccination’ observation period. The distinction between SCCS and case-crossover methods [Reference Delaney and Suissa56] stems from the fact that, as described above, SCCS studies are based on cohort designs, whereas case-crossover studies are based on case-control designs. The use of case-crossover methods for vaccine safety studies is discussed briefly in [Reference Farrington1].
The SCCS method is never exactly as powerful (and therefore, does not yield as precise estimates) as a cohort study with the same cases, unless, as often occurs in practice, there is unexplained between-individual variation in the cohort study which inflates the uncertainty. However, when risk periods are short relative to observation periods, the power of the SCCS method approaches that of a cohort study. However, SCCS studies with long or indefinite risk periods [Reference Farrington, Miller and Taylor18] may have substantially lower power than a cohort study with the same cases (see the discussion of [Reference Farrington and Whitaker4]). A SCCS study is usually more powerful than a case-control study with the same cases and with a single control per case [Reference Farrington, Nash and Miller2]. (As the number of controls increases, the power of the case-control study increases.)
Methodological issues
An unusual feature of the SCCS method is that post-event time is included in the analysis. This is a consequence of the fact that the method works by conditioning, for each individual, on that person's vaccination history over the entire observation period, and on the number of events arising within that period. It follows that observation time should not be censored at the event. One study [Reference Hughes25] did censor observation at the event, in this instance GBS, ostensibly because patients who have had GBS may be advised not to have further immunizations. If GBS patients are less likely to receive immunizations after experiencing the adverse event then, as noted above, a standard SCCS analysis would have resulted in an overestimate of the relative incidence. Censoring at event, however, produces bias of unpredictable direction, and is not recommended.
Several studies of potentially recurrent events, such as convulsions [Reference Huang24], ITP [Reference Miller32] or GBS [Reference Stowe42], considered repeat events to be part of the same episode if separated by less than some minimum time period τ. This presents the methodological problem that, after an event, no other event can then occur for a time interval τ: an instance of immortal time, which, if included in the analysis, may result in bias [Reference Suissa57]. Generally τ is short and repeat events are relatively uncommon, so any such bias is likely to be small. A simple approach is to perform a sensitivity analysis restricted to first events, which also sidesteps the requirement for repeat episodes to be independent. One interesting study [Reference France19] excluded person-time for a period τ after each episode; however, the performance of such a strategy requires further investigation.
Several SCCS studies defined observation periods relative to the day of vaccination, either starting with vaccination and ending a fixed number of days after vaccination [Reference Juurlink26, Reference Zinman48], or starting and ending some fixed number of days before and after vaccination [Reference Ali9, Reference Burwen14]; we refer to such studies as ‘before and after’ designs. For some studies this was done for convenience of data collection. While not invalid, this approach results in short observation periods, which is not optimal, as information from events occurring at other times is not used. In addition, the short control periods may only include time when the risk of temporal bias is high. For example, bias from delayed vaccination following an event may artificially depress the incidence in the period immediately preceding vaccination. This effect is very apparent on the plots of intervals between vaccination and events in [Reference Burwen14], which shows a marked trough of hospitalizations in the week preceding vaccination (this week was, rightly, excluded from the analysis).
As explained in the Introduction, the SCCS method is derived from a cohort model by conditioning on the number of events observed, as well as on vaccination history. Thus, a conditional (Poisson) model is used to estimate the parameters. Fewer than half of the 40 studies indicated that a conditional Poisson regression model was used, either explicitly (e.g. [Reference France19, Reference Gwini, Coupland and Siriwardena21, Reference Hambidge22]) or with words to that effect (as in [Reference Gold20, Reference Zinman48]). In a few studies it was unclear whether a conditional or unconditional model was fitted (e.g. [Reference Payne37]). The only circumstance in which an unconditional Poisson model (i.e. one in which the number of events per individual is not regarded as fixed) may be used in a SCCS analysis is when all individuals have identical observation periods and vaccination histories. In this special case, the conditional and unconditional methods give the same results. In two further instances, the method of analysis appeared somewhat idiosyncratic [Reference Burwen14, Reference Hughes25].
Useful plots
Several studies (e.g. [Reference Dourado16, Reference Stowe43, Reference Tata44]) included plots showing the intervals between events and vaccination; these are useful for visualizing the association between exposure and event (although they are also prone to censoring effects), and for identifying pre-vaccination troughs. Such plots are trickier to draw for multi-dose vaccines, but are useful nonetheless [Reference Murphy34]. Other studies (e.g. [Reference France19, Reference Naleway36]) illustrated the case ascertainment procedure using a flow diagram, which presents clearly the inclusions and exclusions applied to assemble the cases, and hence can help the reader assess any biases that may have arisen in the process. Further useful plots include those illustrating the risk periods used [Reference Gwini, Coupland and Siriwardena21, Reference Smeeth39], those showing estimated age or season effects [Reference Hocine23] and, for complex analyses with many endpoints, graphical representation of the relative incidences [Reference Andrews13].
Power and sample size issues
In studies involving very uncommon events, power and sample size considerations are particularly important [Reference Musonda, Farrington and Whitaker8]. One study [Reference Gwini, Coupland and Siriwardena21] reported checking the sample size required to achieve 90% power to detect at least a doubling of risk. The relevant sample size is the number of events, and if this is too small the estimates and confidence intervals may not be accurate. To aid interpretation, it is important to report the numbers of events in risk and control periods. The larger the imbalance in the expected numbers of events in the risk and control periods, the worse the small sample bias. This is most likely to affect studies with very short risk periods. Simulation studies reported in [Reference Musonda58] suggest that the small sample bias is likely to be small provided at least 2·5 events are expected in the risk period. Note also that a small sample size may adversely affect the ability to control effectively for the effect of age and other time-varying confounders.
Sensitivity analyses
Sensitivity analyses have been mentioned throughout this paper. They provide a simple way of evaluating the robustness of the results; we focus here on where they may be used (other useful sensitivity analyses than those described here can doubtless be performed).
When the SCCS model is used with parametric adjustment for age we recommend checking the sensitivity of exposure risk estimates to choice of age group, by increasing the number of age groups [Reference Hocine23, Reference Smeeth39].
Sensitivity analyses of risk periods should be motivated explicitly (as in [Reference Juurlink26]). Researchers may also wish to consider whether it would be sensible to explore sensitivity of results by adding washout periods to the chosen risk period, removing the day of vaccination or including pre-vaccination risk periods.
If recurrent adverse events occur in episodes, and there is a lack of clarity over whether repeat events are part of the same episode, sensitivity to the choice of definition of episodes can be checked. Note that analyses of first events only can be carried out to avoid any issue of lack of independence between adverse events.
If exact dates or timings of exposures or events are unknown and have to be imputed, sensitivity to how these timings are imputed should be explored.
When sensitivity analyses are performed, they should be reported, with full details when they relate to risk periods, washout periods and pre-vaccination periods. It is important to distinguish between them and the pre-planned primary analyses. If sensitivity analyses suggest possible departures from the assumptions of the method, this should be stated explicitly. If it is thought that departure from assumptions might affect the results, then, where possible, alternative methods of analysis should be used in conjunction with SCCS.
Software for SCCS analyses
Twelve studies reported which statistical package was used to undertake the SCCS analysis. Six used Stata (StataCorp, USA), five used SAS (SAS Institute Inc., USA) and one used GLIM (NAG, UK). Further information about fitting SCCS models using these packages and other standard software may be found in [Reference Whitaker5] and on the associated website (http://statistics.open.ac.uk/sccs).
The SCCS model is most conveniently fitted using software designed for Poisson regression models with fixed effects (in this case, the levels of the fixed effects represent distinct individuals). This method of fitting the models exploits a convenient technical fact known as the ‘Poisson trick’, whereby a multinomial likelihood (which applies for the SCCS method, see [Reference Farrington3, Reference Farrington and Whitaker4]) can be maximised using a Poisson model. However, this trick has its limits: for example, fitting age as a continuous variable cannot be done in this way, because it does not allow for the fact that age varies within each risk or control interval.
DISCUSSION
This review was based on papers quoting papers by the second and third author on the case-series method. We are aware of several independent reinventions of the SCCS method in different contexts: the bidirectional case-crossover method applied to fixed observation times [Reference Navidi59] and the time-stratified case-crossover approach [Reference Lumley and Levy60], developed for the analysis of environmental time-series (see [Reference Vines and Farrington61, Reference Whitaker, Hocine and Farrington62] for a discussion of the connections with the SCCS method), and the method of [Reference Becker, Li and Kelman63] applied to venous thromboembolism after long-haul flights. None of these versions of SCCS have so far been used in connection with vaccine safety. Thus, to the best of our knowledge, we have included all applications of SCCS methodology to vaccine studies that appeared by the end of 2010.
We identified and reviewed 40 papers which applied the SCCS method to vaccine studies. In general the method was applied appropriately. All 40 studies provided sufficient detail of how their data were collected, which enabled the reader to make sure that events are identified independently of vaccinations. Moreover, observation and risk periods were generally carefully specified. Most studies adjusted for age and/or season as appropriate.
The following key issues emerge when using the SCCS method. Ascertainment of cases and collection of data on exposure history should be independent, as bias may result if case ascertainment was influenced by knowledge of exposure status. The observation and risk periods should be clearly defined, and the choice of risk period should be justified. Where necessary, age and season effects should be allowed for, and when using the parametric model, sensitivity to the choice of age and seasonal groups should be checked. Other relevant time-varying covariates (such as concurrent vaccinations and other exposures) which may be associated with both the exposure and outcome should be identified and, if possible, taken into account in the analysis. The validity of the assumptions required by the SCCS method should be carefully considered and appropriate supplementary sensitivity analyses undertaken where these come into question.
A few papers suggest there remains a degree of confusion about what a SCCS study entails, in particular how it differs from a ‘before and after vaccination’ analysis or from the case-crossover paradigm. This is wholly unsurprising, owing to the somewhat abstruse and technical, yet fundamental, distinction between conditional and unconditional analyses. A recent methodological paper [Reference Glanz64] also describes a ‘before and after’ design, described as a ‘risk interval method’, which is in fact a special case of a SCCS design. The term ‘case centred’ has also been used to describe such designs [Reference Klein51]. We excluded two papers [Reference France50, Reference Klein51] with ‘before and after’ analyses from our review because they did not describe the design as SCCS; several ‘before and after’ analyses that did were included in the review. In fact, all these studies are special cases of the SCCS design. Nevertheless, the picture that emerges is dominated by the numerous impressive and often imaginative applications of the method.
The SCCS method has witnessed considerable methodological development aimed at weakening the assumptions it requires. Thus, methods have been developed to handle event-dependent exposures and deaths [Reference Kuhnert55], dependent recurrences [Reference Farrington and Hocine52], event-dependent observation periods [Reference Farrington53]. The method has also been extended to the prospective monitoring of vaccine safety [Reference Musonda65, Reference Hocine66]. Current research is underway to fit SCCS models with smooth functions describing age and vaccine effects.
This review has raised some further methodological issues worthy of further study. One such is how best to handle the ‘immortal time’ after an event, during which recurrences are classified as part of the same episode, and whether ignoring this effect has any substantive bearing on the results. Another is to study and quantify the bias that results from censoring observation periods at events. Sensitivity analyses may be indicated in both circumstances. Further, while the SCCS method is only applicable with a single outcome variable at a time, it may be desirable to study several outcomes jointly. A bivariate SCCS method has been suggested for the analysis of antibiotic resistance [Reference Hocine67]; perhaps similar ideas can be used for a multivariate SCCS applied to vaccine safety, in which several possibly dependent outcomes could be studied at the same time.
SCCS analysis is a relatively new statistical methodology, and the issues that require particular emphasis and care in reporting have, therefore, only become apparent over time. The development of suitable guidelines for reporting such studies, in vaccine safety and pharmacoepidemiology more widely, may perhaps now be indicated.
ACKNOWLEDGEMENTS
We thank two anonymous referees for their comments which greatly improved the paper.
DECLARATION OF INTEREST
None.