Introduction
Drug-resistant tuberculosis (TB) has been a serious obstacle for global TB control programmes. TB patients with drug resistance may be induced by exposure to multidrug- and extensively drug-resistant tuberculosis (MDR/XDR-TB) strains or may develop as a result of other clinical factors, including delayed diagnosis, inappropriate treatment or poor compliance [Reference Kerubo1]. Controlling the high prevalence of drug-resistant TB largely depends on a timely laboratory diagnosis. Traditional TB drug susceptibility testing (DST) relies on solid or liquid culture, which may take weeks or months to yield results. The slow growth of Mycobacterium tuberculosis is an impediment to the rapid diagnosis of anti-TB drug resistance, and aggravates the situation by increasing the incidence of MDR and XDR-TB in the world. Some rapid molecular biology-based diagnostic methods have recently been applied in a clinical setting, including Xpert MTB/RIF and GenoType MTBDRplus [Reference Helb2–Reference Hillemann, Rüsch-Gerdes and Richter4]. Although these methods are rapid and simple, their extension to undeveloped areas of the world is limited by prohibitive costs and availability.
Whole-genome sequencing (WGS), as a molecular diagnostic tool, has been greatly developed in TB research since the first complete genome sequence H37Rv was announced in 1998. The sensitivity and specificity reported for predicting single common anti-TB drugs have been over 80% [Reference Faksri5, Reference Papaventsis6], but few studies evaluated the prediction of MDR and XDR based on WGS.
Therefore, in the current study, we performed WGS on drug-resistant M. tuberculosis isolates from China and Russia. One of our goals was to detect MDR and XDR based on limited numbers of mutation sites, and construct some combinations of drug-resistance-associated loci for predicting MDR/XDR with higher sensitivity and specificity at the same time. Another goal was to characterise the underlying drug-resistance-conferring mutations and higher-frequency novel mutations, promoting a comprehensive understanding of the mechanisms of drug-resistant TB and providing more information for clinical management.
Materials and methods
Sample collection and processing
A total of 105 M. tuberculosis clinical isolates were randomly sampled from among those collected from Shanghai Pulmonary Hospital, the biggest designated TB hospital in Shanghai City, between May 2017 and March 2018. These samples included 35 XDR cases, 35 MDR cases and 35 pan-susceptible cases.
According to the principle of equidistance, we selected the samples on the basis of the strains in the overall unit. MDR-TB indicates TB that is resistant to at least isoniazid (INH) and rifampicin (RIF), whereas XDR-TB indicates TB that is resistant not only to INH and RIF, but also to any fluoroquinolone and at least one of three injectable drugs, amikacin (AMI), kanamycin (KAN) and capreomycin (CAP). Drug susceptibility tests were performed by laboratory technologists from the TB laboratory using a fully automated Mycobacterial Growth Indicator Tube (MGIT) 960 liquid culture system (Becton Dickinson, USA). The critical concentrations of anti-TB drugs in these specific assays were as follows: rifampicin, 1.0 μg/ml (MGIT); isoniazid, 0.1 μg/ml (MGIT); ethambutol, 5.0 μg/ml (MGIT); streptomycin, 1.0 μg/ml (MGIT); ofloxacin, 1.5 μg/ml (MGIT); capreomycin, 2.5 μg/ml (MGIT); and amikacin, 1.0 μg/ml (MGIT) [Reference Katende7]. All experimental methods conformed to standardised clinical laboratory procedures and operating regulations. DNA was extracted using a Mag-MK Bacterial Genomic DNA extraction kit according to the instructions provided. Quality control criteria for WGS included a minimum concentration >50 ng/μl and a minimum OD260/280 ratio (DNA purity) >1.8.
Because some samples failed to meet these criteria owing to failure of seeding, contamination of culture medium or extraction of DNA, only 97 isolates (30 XDR, 32 MDR and 35 pan-susceptible) were included in the study. Other isolates, including three MDR, 16 DR (resistant to any anti-TB drug other than XDR or MDR) and 21 pan-susceptible strains, were from Shanghai Minhang District Central for Disease Control and Prevention and Shanghai Putuo District Center for Disease Control and Prevention. The collection of these latter isolates was community-centred, whereas the original 105 strains were obtained from the designated TB hospital. We also downloaded some genome sequences from the National Center for Biotechnology Information for some foreign strains in fastq file format, including 12 XDR cases, 39 MDR cases and 27 pan-susceptible cases. These strains were mainly derived from Francis Coll's [Reference Coll8] study of TB patients in Russia between 2008 and 2010. By inquiring the phenotypic drug sensitivity results of these strains and matching the corresponding original sequencing sequences, we finally included the sequences of 78 foreign strains in total.
Sequencing
WGS was performed on an Illumina HiSeq 2000 platform. Sickle (http://github.com/ucdavis-bioinformatics/sickle) was used for trimming reads, with a minimum base quality of Q20. Bowtie 2 [Reference Langmead and Salzberg9], version 2.3.3, was used for mapping to the reference genome, M. tuberculosis H37RV, (NC_000962.3), with a minimum mapping quality of Q30. SAMtools [Reference Li10], version 1.6, was used for calling single-nucleotide polymorphisms (SNPs), and VarScan (version 2.3.9) [Reference Koboldt11] was used for calling mutation variants. Sequencing errors and false positives were excluded based on a series of thresholds, including reference coverage >95% and an average read depth >20. Mutation frequencies in cases where alternative alleles were based on fewer than 25% of reads were filtered using VarScan. Finally, SNPs located in Pro-Glu (PE) and Pro-Pro-Glu (PPE) regions, insertion elements, or repetitive regions were excluded.
Identification of drug-resistance-conferring mutations
A total of 215 M. tuberculosis isolates were obtained for genome sequencing, of which 97 were from Shanghai pulmonary hospital, 40 were from Shanghai Minhang District Central Hospital and Shanghai Putuo District Center for Disease Control and Prevention, and 78 were from Russia. Common drug-resistance-conferring mutant genes and their mutation frequencies may differ between domestic isolates and foreign isolates. We first selected 18 candidate genes for our drug-resistance mutation analysis process, taking into account those that were reported more than once in the scientific literature [Reference Walker12]. We found resistance mutations in only eight of these genes, possibly owing to the limited numbers of samples and/or lower mutation frequency. We presumed that synonymous and lineage-defining mutations were attributable to benign mutations that do not lead to a resistant phenotype. Since some mutations are only present in phenotypically susceptible strains or susceptibility is retained despite the presence of a mutation, mutations in all strains that were phenotypically susceptible were considered benign mutations. For all candidate genes, a mutation that occurred in at least one phenotypically resistant isolate was defined as resistance determining. Sometimes a resistant phenotype was not accounted for by a single resistance-conferring mutation in a candidate gene for a given drug as a result of synergy or co-occurring mechanisms among mutations [Reference Walker12]. In such cases, genome sequences were manually examined. An uncharacterised mutation corresponds to a mutation that occurred in a single phenotypically resistant isolate, and thus may not be a resistance-conferring mutation. The sensitivity of prediction was calculated by dividing the number of strains with drug phenotypic resistance mutations found by WGS in phenotypic resistance strains by the total number of phenotypic resistance strains. Fisher's exact test used to determine statistical difference of resistance mutations for each drug between different groups was performed in R (v4.0.2).
Bootstrap analysis
First, Perl scripts were used to combine SNP sites of all strains. Second, high-frequency resistance sites for each drug were filtered and combined in R (v4.0.2). Finally, a Bootstrap analysis (1000 times) was performed in R (v4.0.2) on select combinations to predict MDR and XDR isolates, calculating sensitivity and specificity and 95% confidence intervals (CI).
Results
Characteristics of the samples
We selected a total of 215 isolates for our genetic mutation analysis; 137 strains were collected from Shanghai, including 35 MDR, 30 XDR, 16 DR and 56 pan-susceptible strains. An additional 78 strains were from Russia isolates, including 39 MDR, 12 XDR and 27 pan-susceptible strains. Online Supplementary Table S1 details drug-resistance information for patients. Four first-line drugs, rifampicin (RIF), isoniazid (INH), streptomycin (STR) and ethambutol (EMB), and three second-line drugs, amikacin (AMK), capreomycin (CAP) and ofloxacin (OFL), were considered in our analysis. Of these 215 isolates, 120 (55.8%), 124 (57.7%), 67 (31.2%), 90 (41.9%), 42 (19.5%), 42 (19.5%) and 42 (19.5%) were resistant to RIF, INH, EMB, STR, OFX, AMK and CAP, respectively. A phylogenetic tree was constructed from a total of 215 M. tuberculosis strains using a maximum likelihood phylogenetic method in RAxML software (1000 bootstrap samples), yielding 162 935 high-quality SNPs (Fig. 1). Alternative alleles identified in fewer than 25% of reads were filtered out, and SNPs in PE/PPE regions, insertion elements and repeat regions were excluded from the analysis [Reference Stamatakis, Hoover and Rougemont13]. SNPs with a pairwise genetic distance of 12 or fewer SNPs were defined as recent transmissions [Reference Yang14]. No recent transmission events were present in the tree as finally constructed. We defined the lineage of strains using regions of difference [Reference Faksri15]. A total of 191 strains (88.8%), including 41 XDR, 62 MDR, 13 DR and 75 pan-susceptible isolates, belonged to lineage 2.2 (‘ancient’ Beijing); 24 strains (11.2%), containing one XDR, 12 MDR, three DR and eight pan-susceptible isolates, belonged to four sublineages, L4.2, L4.4, L4.5 and L4.8, designated ‘Euro-American’ lineages [Reference Liu16]. We found 30 drug-resistance markers in eight candidate genes among our 215 M. tuberculosis strains, as summarised in Table 1.
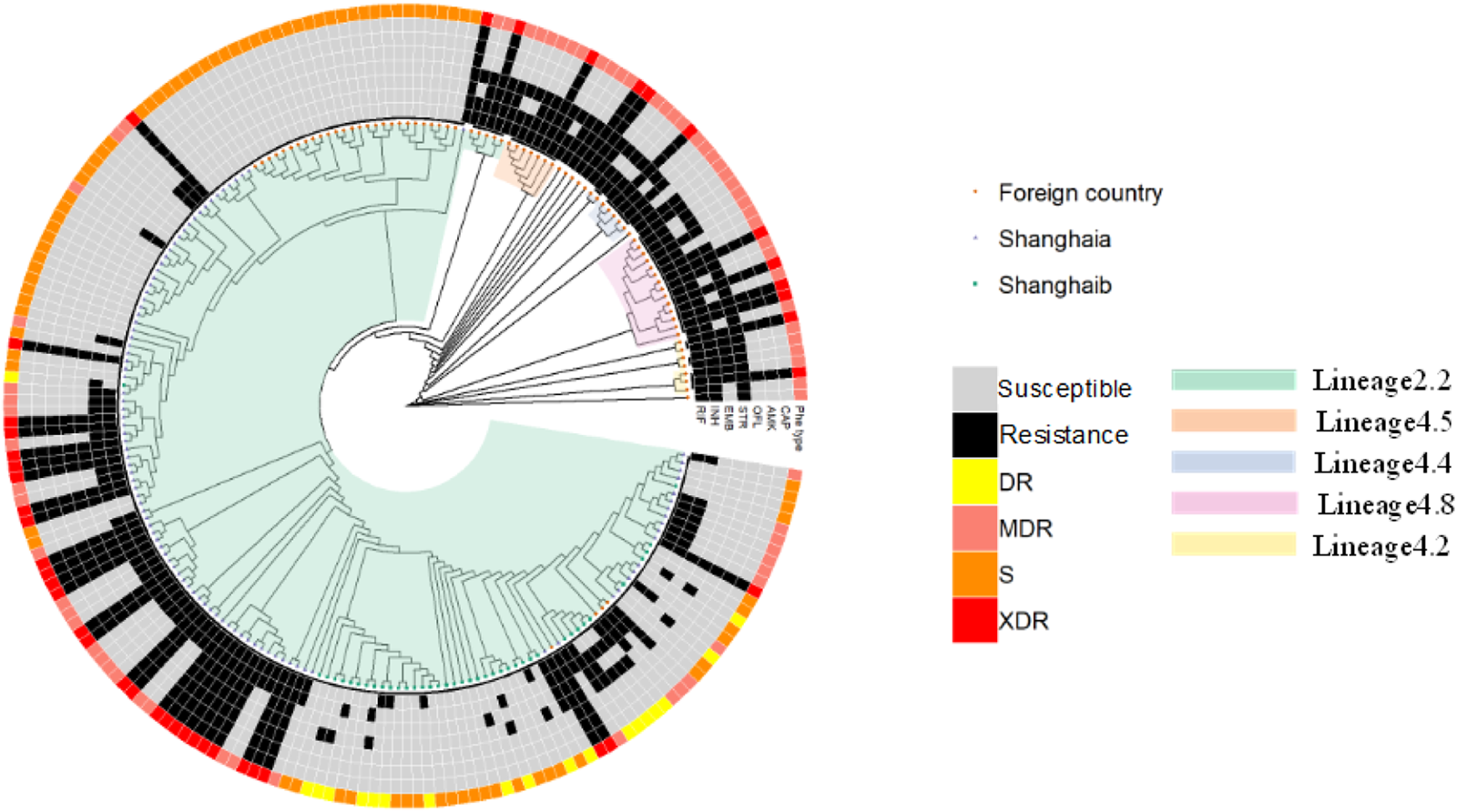
Fig. 1. Whole-genome phylogeny of the 215 M. tuberculosis isolates. Maximum likelihood phylogenetic tree (bootstrap = 1000 times) constructed using 162 936 SNPs spanning the whole genome and rooted on Mycobacterium canetti (not shown). Drug-resistance type is indicated by different colours in the outer ring of the circle; lineage definitions are indicated in the inner part of the circle; and drug-resistance profiles are shown in the middle portion of the circle. Russia isolates were randomly selected from among strains downloaded from GenBank. ‘S’ denotes isolates that were susceptible to all anti-TB drugs tested. ‘DR’ refers to isolates other than MDR or XDR strains that were resistant to any TB drugs. Shanghaia indicates that isolates are from Shanghai Pulmonary Hospital, Shanghaib indicates that isolates are from Shanghai Putuo and Minghang District Center for Disease Control and Prevention.
Table 1. Mutations identified within loci associated with resistance to anti-TB drugs in 215 clinical isolates

RIF, rifampicin; INH, isoniazid; EMB, ethambutol; STR, streptomycin; AMK, amikacin; CAP, capreomycin; OFL, ofloxacin.
a S isolate indicates pan-susceptible isolate (i.e. sensitive to four first-line anti-TB drugs).
Resistance to the first-line anti-TB medicines
Mycobacterium tuberculosis strains that showed resistance to RIF generally exhibited mutations in the 81-bp rpoB RIF-resistance-determining region [Reference Heep17]. The most prevalent drug-resistance-conferring mutation was rpoB Ser450Leu. Among domestic isolates, 69 strains were resistant to RIF, of which 42 strains, including 22 XDR, 17 MDR and three DR strains, possessed rpoB Ser450Leu missense mutation. Among 56 pan-susceptible isolates, none resistance mutations were characterised. The sensitivity and specificity of rpoB Ser450Leu in predicting phenotypic resistance to RIF were 60.87% and 100%, respectively. Other strains lacking this mutation exhibited a lower frequency of other missense mutations. Among domestic 30 XDR strains, there were two isolates with an rpoB Asp435Val mutation, one isolate with an rpoB Gln172Arg mutation, one isolate with an rpoB Gln432Lys mutation, one isolate with an rpoB Gln432Pro mutation, and three phenotypically resistant strains without any mutation in the rpoB gene. Among 35 MDR strains, there were three isolates with an rpoB His445Pro mutation, two isolates with an rpoB Asp435Val mutation, one isolate with an rpoB Gln432Pro mutation, one isolate with an rpoB His445Asp mutation, one isolate with co-occurrence of rpoB His445Asp and His445Pro mutations, as well as phenotypically resistant strains without any mutations in the rpoB gene. Among 16 DR strains, there were four phenotypically resistant strains without any mutations. Among Russia isolates, 47 drug-resistant strains carried an rpoB Ser450Leu mutation. The sensitivity and specificity of rpoBSer450Leu in predicting phenotypic resistance to RIF were 92.16% and 100%, respectively. There was also one strain with an rpoB His445Asp mutant, and three phenotypically resistant strains without any mutations. Totally, WGS was capable of accounting for 79.71% (55/69) of phenotypically RIF-resistant domestic strains and 94.12% (48/51) of phenotypically RIF-resistant Russia strains.
INH is a prodrug that is activated by the catalase-peroxidase enzyme katG, encoded by the katG gene [Reference Cade18]. Among our 215 M. tuberculosis strains, 124 exhibited resistance to INH. The most common drug-resistance-conferring mutation was katG Ser315Thr, in which the codon change may be G-C. Among domestic isolates, 52 strains carried a katG Ser315Thr mutation, including 26 MDR, 21 XDR and five DR strains. The sensitivity and specificity of katG Ser315Thr in predicting phenotypic resistance to INH were 71.23% and 100%, respectively. Among 30 XDR strains, there were 21 resistant strains with a katG Ser315Thr mutation, two strains with an inhA Ile21Thr mutation, one strain with an inhA Ser94Ala mutation, one strain with an ndh Ala352Ser mutation, one strain with a katG Ala144Val mutation, one strain with a katG His97Arg mutation, and three strains that were phenotypically resistant to INH that had no mutations in our candidate genes. Among 35 MDR strains, there were 26 strains with a katG Ser315Thr mutation, two strains with a katG Glu607Lys mutation, one strain with an inhA Ile21Thr mutation, one strain with a katG Ala144Valmutation, one strain with a katG Ser17Gly mutation, and one strain that carried no mutations. Among eight DR strains, there were five strains that carried a katG Ser315Thr mutation. Among Russia isolates, 10 XDR and 37 MDR strains carried a katG Ser315Thr mutation. The frequency of the katG Arg463Leu mutation, used as a phylogenetic marker, was higher than that of any other mutation among our selected genes, but because it does not confer INH resistance, it was excluded. WGS was capable of predicting 86.30% (63/73) of phenotypically INH-resistant domestic strains and 92.16% (47/51) of phenotypically INH-resistant Russia strains.
Mutations in the rpsL gene, encoding a 30S ribosomal protein associated with the first step of RNA translation, have been reported to account for approximately 80% of STR resistance [Reference Chakraborty19]. Thus, the rpsL gene was selected as a candidate gene for assessing STR resistance in different strains. The most frequently detected mutation was rpsL Lys43Arg, which was present in 41 phenotypically STR-resistant strains. The sensitivity and specificity of the rpsL Lys43Arg mutation in predicting phenotypic STR resistance were 45.56% and 100%, respectively. Among domestic isolates, there were 17 XDR strains with the rpsL Lys43Arg mutation, five XDR strains with the rpsL Lys88Arg mutation, eight DR strains with the rpsL Lys43Arg mutation, and two DR strains with the rpsL Lys88Arg mutation. Among Russia isolates, there were eight MDR and six XDR strains with the rpsL Lys43Arg mutation and one MDR strain with the rpsL Lys88Arg mutation. There were also detected rrs C517T mutation in four XDR domestic isolates and three XDR Russia isolates. WGS was capable of predicting 88.37% (38/43) of phenotypically STR-resistant domestic strains and 38.30% (18/47) of phenotypically STR-resistant Russia strains.
Drug-resistance-conferring mutations associated with EMB primarily occur in the embB gene [Reference Jagielski20]. The most common mutation in our phenotypically EMB-resistant strains was embB Met306Val; among 67 phenotypically resistant isolates, 22 strains carried this mutation. Among domestic isolates, there were 12 XDR strains with an embB Met306Val mutation, five XDR strains with an embB Met306Ile mutation, two XDR strains with an embB Gln497Arg mutation, three XDR strains with an embB Gly406Ala mutation, and two XDR strains with an embB Gly406Ser mutation. Among two DR strains that were phenotypically resistant to EMB, one strain carried an embB Met306Val mutation and one strain carried an embB Met306Leu mutation. Among Russia isolates, six MDR and three XDR strains carried an embB Met306Val mutation, 16 MDR and three XDR strains carried an embB Asp354Ala mutation, and one XDR strain carried an embB Gln497Arg mutation. WGS was capable of predicting 76.47% (26/34) of phenotypically EMB-resistant domestic strains and 87.88% (29/33) of phenotypically EMB-resistant Russia strains.
Mutations related to the second-line drugs
Mutations in the rrs gene, encoding 16S rRNA, are markers associated with AMK and CAP resistance, especially mutations occurring at nucleotide positions 1401, 1402 and 1484 [Reference Bhembe21]. Among 30 XDR domestic strains, the most prevalent AMK/CAP-resistance-conferring mutation was rrs A1401G, which was found in 19 phenotypically resistant strains. The sensitivity and specificity of the rrs A1401G mutation in predicting phenotypic resistance to AMK/CAP were 63.33% and 100%, respectively. Other mutations detected among XDR domestic strains were rrs C1402T and rrs G1484T, found in one strain each. Among 12 Russia XDR isolates, there were three with an rrs A1401G mutation, one with an rrs C1402T mutation. WGS was capable of predicting 70.00% (21/30) of phenotypically AMK/CAP-resistant domestic strains and 33.33% (4/12) of phenotypically AMK/CAP-resistant Russia strains.
Resistance to OFL is associated with mutations in the genes, gyrA and gyrB, encoding subunits that constitute the heterotetrameric protein, DNA gyrase; these mutations occur predominantly at codons 88–94 [Reference Disratthakit22]. Among 30 domestic XDR strains, the most common drug-resistance-conferring mutation was gyrA Asp94Gly, which was present in 10 phenotypically OFL-resistant strains. The sensitivity and specificity of the gyrA Asp94Gly mutation in predicting phenotypic resistance to OFL were 33.33% and 100%, respectively. Other mutations included gyrA Ala90Val, found in nine phenotypically resistant strains; gyrA Asp94Ala, found in three strains; and gyrA Asp94Asn, found in three strains. Among 12 Russia XDR strains, two strains carried a gyrA Asp94Gly mutation and one strain carried a gyrA Asp94Asn mutation. WGS was capable of predicting 83.33% (25/30) of phenotypically OFL-resistant domestic strains and 25.00% (3/12) of phenotypically OFL-resistant Russia strains.
Prediction value of loci combination by bootstrap
Finally, we used a bootstrap approach (1000 times) to create combinations of highly prevalent drug-resistance locations to predict the XDR/MDR-resistant phenotype. The sensitivity and specificity of our combinations are summarised in Table 2. The highest sensitivity with respect to the prediction of MDR against RIF and INH was 92.17% (0.8615, 0.9646), which was provided by the combination of rpoB S450L + rpoB H445A + rpoB H445P + katG S315T + inhA I21T + inhA S94A mutations. The highest sensitivity with respect to the prediction of XDR against RIF, INH, AMK/CAP and OFL was 92.86% (0.8158, 0.9796), which was provided by the combination of rpoB S450L + katG S315T + gyrA D94G + rrs A1401G mutations. We also tested the inclusion of additional mutations in combinations, but none of these additions improved the predictive effect.
Table 2. Bootstrap approach for validating MDR/XDR-TB with combinations of drug-resistance loci

Discussion
We found that WGS was capable of predicting about 82.07% and 72.33% of phenotypic drug resistance for China and Russia strains, respectively. For domestic strains, the predictive sensitivity from the highest to the lowest was STR (88.37%), INH (86.30%), OFL (83.33%), RIF (79.71%), EMB (76.47%) and AMK/CAP (70%). For Russia strains, the sequence was RIF (94.12%), INH (92.16%), EMB (87.88%), STR (38.30%), AMK/CAP (33.33%) and OFL (25.00%). First, geographic variation may explain the differences of mutant frequency in predictive values. Second, the predictive sensitivity of STR resistance was the best in China using the WGS method, which could be due to the fact that STR resistance-conferring mutations were largely found in China. Finally, the sensitivity of WGS in detecting resistance to second-line drugs were unsatisfactory, possibly because of the limited numbers of isolates, preventing us from discovering the full variety of mutations.
The phylogenetic tree showed that lineage 2 and lineage 4 spread in both Russia and China, and L2.2 constituted the predominant strain, accounting for 97.4% (76/78) in Russia and 83.9% (115/137) in China. The fact that M. tuberculosis lineage 2.2, also called Beijing family, is equipped with higher adaptability, resistance and virulence may be responsible for its widely prevalence. Actually, human-adapted M. tuberculosis complex comprises seven lineages of various geography distributions. Lineage 2 and lineage 4 distributed in worldwide, while other lineages exhibit a geographical restriction. Moreover, susceptible, drug-resistant and MDR strains existed in lineage 2 and lineage 4, but XDR strains almost exclusively existed in lineage 2.2, which demonstrates that different lineages may play a different role in the outcome of drug resistance.
Only eight resistance genes among 18 candidate genes, and a total of 30 mutations of these eight resistance genes were identified, possibly attributing to a lack of sufficient samples and low rate of mutation. Limited numbers of samples lack the ability to identify comprehensive mutations. Among 30 detected mutations, 26 mutations have been reported in previous studies and four mutations were novel mutations (rpoB Q172R, katG A144V, katG H97R, katG S17G), but whether it could be regarded as markers of drug resistance remains to be further studied. In addition, we identified katG Arg463Leu, gyrA Glu21Gln, gyrA Ser95Thr and gyrA Gly668Asp as lineage-defining mutations. These mutations, which have been reported to be natural polymorphisms that are useful for evolutionary characterisation of the genome [Reference Lau23], were present at elevated frequencies in both resistant and sensitive strains, and thus did not represent drug-resistance-conferring mutations.
Although the specificity of representative genes in predicting phenotype was 100%, it could be overestimated because we assumed that strains without detecting corresponding mutations were susceptible. The rates of dominant mutations of seven drugs in this study were INH (71.23%), RIF (60.87%), STR (62.79%), EMB (38.24%), AMK/CAP (63.33%) and OFL (33.33%). About other mutations of each drug are discussed in the following. For rifampicin resistance, in addition to those phenotypic resistance without any mutation, the rest RIF-resistance-conferring mutations occurred exclusively in the rpoB gene region in this study, including rpoB Ser450Leu, rpoB Asp435Val, rpoB His445Asp/Pro and rpoB Gln432Pro/Lys which have been reported in previous studies [Reference Donnabella24–Reference Sajduda26]. We also found a novel mutation, rpoB Gln172Arg, but it was only detected in a single resistant isolate. The katG gene is thought to have a high association with INH resistance and the most frequently reported katG mutation, Ser315Thr, was found in this study. Other previously known mutations, including inhA Ile21Thr and inhA Ser94Ala, were also identified [Reference Leung27, Reference Morlock28]. We also identified three novel mutations, katG Ala144Val, katG His97Arg and katG Ser17Gly, but further studies are needed to elucidate whether these mutations really confer resistance to INH or not. The most commonly reported mutation of EMB resistance, embB Met306Val, was identified in the current study and other previously reported mutations [Reference Plinke29, Reference Ramaswamy30], embB Gln497Arg, embB Gly406Ala and embB Asp354Ala, also were identified. Nevertheless, identifying embB Met306Val mutation to predict phenotype was not an effective option since the predictive sensitivity was quite lower than expected, which reminds us that embB Met306Val mutation may be just one small part of all main EMB resistance-conferring mutations. Other candidate genes and mutation sites of EMB resistance need to be further explored. STR resistance-conferring mutations mainly happen in the rpsL and rrs genes [Reference Hlaing31]. However, some related studies have indicated that the association of mutations in the rrs gene with resistance is not obvious [Reference Sun32], instead reporting that mutations of lysine to arginine in the rpsL gene are highly correlated with the development of STR resistance. In our results, the most frequent mutations in rpsL gene were rpsL Lys43Arg and rpsL Lys88Arg, similar to the results of Spies et al. [Reference Spies33]. For OFL-resistant strains, two mutations with high frequency, gyrA Ala90Val and gyrA Asp94Gly, that were reported previously [Reference Chen, Zhang and Zhang34] were also found in this study, additional mutations including gyrA Asp94Ala and gyrA Asp94Asn were also found in this study, and both of them have been reported [Reference Takiff35]. Cross-resistance to AMK and CAP attributes to mutations in the rss gene, with the mutations rrs A1401G, rrs C1402T and rrs G1484T being the most common [Reference Maus, Plikaytis and Shinnick36]. In our study, we found that rrs A1401G, detected in 63.3% of AMK/CAP-resistant isolates, was the most prevalent. The frequency of other mutations was lower than expected.
In the attempt to improve the predictive power of WGS, we applied a bootstrap approach to identify combinations of high-frequency mutations that might prove more sensitive. For predicting the MDR phenotype, we found that the lowest predictive sensitivity combination was rpoB S450L + katG S315T. And the predictive sensitivity increased with including more mutation sites, with the highest predictive sensitivity combination of rpoB S450L + rpoB H445A/P + katG S315T + inhA I21T + inhA S94A. No obvious improvement on MDR predictive sensitivity was observed when rpoB A435V and KatG G607L mutations were added. For predicting XDR phenotype, the mutation combination with highest sensitivity (92.86%) was rpoB S450L + katG S315T + gyrA D94G + rrs A1401G. Similarly, no significant improvement was observed when adding more mutations. WGS, in recent years, has been increasingly used to predict drug resistance and guide drug-susceptibility testing patterns. Several automated software tools have been developed for this, such as CASTB, KvarQ, Mykrobe Predictor TB, PhyResSE and TB Profiler [Reference Schleusener37]. Most studies indicated that WGS has a high sensitivity to predict isoniazid and rifampicin resistance prediction, but a variable performance for resistance prediction of other drugs. This might be due to the incompleteness of drug-resistance profiles in local. While most resistance prediction focused on first-line drugs or single drug, hence, the combinations of resistance prediction in present study might provide useful references for MDR and XDR prediction [Reference Allix-Béguec38].
For resistance rates of seven drugs in Russia and China, the proportion of resistance to RIF and INH accounted for the vast majority while the percentage of resistance to second-line drug (OFL) and injectable drugs (AMK/CAP) were quite low, which are consistent with most previous studies. In fact, resistance rates reflect the selective pressure of anti-TB drugs on the evolution of M. tuberculosis and the long-term anti-TB treatment leads to the continuous emergence and expansion of resistant strains. For resistance phenotype, the proportion of MDR-TB in Russia (50.0%) was much higher than in domestic (23.4%), probably because of the history of high level of MDR-TB in Russian federation. The difference in resistance rates and phenotype implied that resistance-conferring mutations are highly associated with geographic location.
WGS, on the one hand, offers a number of advantages compared with traditional DST [Reference Hang39, Reference Takii40], which reduce diagnostic time to 4–8 days compared with traditional laboratory culture methods. The current cost of diagnosing TB with WGS is about $80 per case, which is actually slightly less than the cost of DST. On the other hand, WGS certainly has some limitations. For example, it could not show the minimum inhibitory concentration of drug resistance compared with DST and there are some phenotypically drug-resistant isolates without any gene mutation. One of the limitations in this study is the selection bias of strains and insufficient resistance candidate genes, which reflects the relatively low predictive sensitivity of WGS for some drug. In addition, the definition of XDR-TB has changed in January 2021 [41], which deserves further observation and adjustment in DST prediction.
Conclusions
We found that WGS has a higher sensitivity and specificity in predicting resistance to first-line anti-TB drugs, compared with second-line drugs. We also identified some novel mutations in katG and rpoB genes, but additional insight into drug-resistance mechanisms is needed. These novel mutations, together with frequent mutations, can provide a reference for clinical microbiology laboratory diagnostic methods for identifying drug-resistant TB. The valuable mutation combination, identified using the bootstrap method, for predicting MDR-TB phenotype was rpoB S450L + rpoB H445A/P + katG S315T + inhA I21T + inhA S94A. For second-line drugs, and for predicting XDR-TB phenotype, it was rpoB S450L + katG S315T + gyrA D94G + rrs A1401G.
Supplementary material
The supplementary material for this article can be found at https://doi.org/10.1017/S095026882100279X
Author contributions
H. X., W. W. and F. Y. designed this study. L. W. and J. Y. collected the M. tuberculosis isolates applied in this study. L. W., J. Y., L. C., W. W. and H. X. discussed the outcome of genome sequencing. L. W. and J. Y. performed the statistical analysis and wrote this manuscript. W. W. and H. X. revised the structure and language of this paper. All authors reviewed the manuscript and agreed to the published version of this manuscript.
Financial support
This study was granted by the National Key Scientific and Technological Project against Major Infectious Diseases (Grant nos. 2017ZX10201302-007-003 and 2018ZX10722302-005), and the Shanghai New Three-year Action Plan for Public Health (Grant no. GWV-10.1-XK16).
Conflict of interest
None.
Author statement
We declare that the work described was original research that has not been published previously, and not under consideration for publication elsewhere, in whole or in part. All the authors listed have approved the manuscript that is enclosed.
Data availability statement
Available on reasonable request.