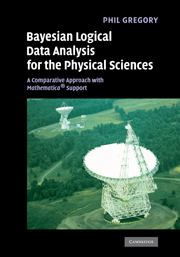
Book contents
- Frontmatter
- Contents
- Preface
- Acknowledgements
- 1 Role of probability theory in science
- 2 Probability theory as extended logic
- 3 The how-to of Bayesian inference
- 4 Assigning probabilities
- 5 Frequentist statistical inference
- 6 What is a statistic?
- 7 Frequentist hypothesis testing
- 8 Maximum entropy probabilities
- 9 Bayesian inference with Gaussian errors
- 10 Linear model fitting (Gaussian errors)
- 11 Nonlinear model fitting
- 12 Markov chain Monte Carlo
- 13 Bayesian revolution in spectral analysis
- 14 Bayesian inference with Poisson sampling
- Appendix A Singular value decomposition
- Appendix B Discrete Fourier Transforms
- Appendix C Difference in two samples
- Appendix D Poisson ON/OFF details
- Appendix E Multivariate Gaussian from maximum entropy
- References
- Index
8 - Maximum entropy probabilities
Published online by Cambridge University Press: 05 September 2012
- Frontmatter
- Contents
- Preface
- Acknowledgements
- 1 Role of probability theory in science
- 2 Probability theory as extended logic
- 3 The how-to of Bayesian inference
- 4 Assigning probabilities
- 5 Frequentist statistical inference
- 6 What is a statistic?
- 7 Frequentist hypothesis testing
- 8 Maximum entropy probabilities
- 9 Bayesian inference with Gaussian errors
- 10 Linear model fitting (Gaussian errors)
- 11 Nonlinear model fitting
- 12 Markov chain Monte Carlo
- 13 Bayesian revolution in spectral analysis
- 14 Bayesian inference with Poisson sampling
- Appendix A Singular value decomposition
- Appendix B Discrete Fourier Transforms
- Appendix C Difference in two samples
- Appendix D Poisson ON/OFF details
- Appendix E Multivariate Gaussian from maximum entropy
- References
- Index
Summary
Overview
This chapter can be thought of as an extension of the material covered in Chapter 4 which was concerned with how to encode a given state of knowledge into a probability distribution suitable for use in Bayes' theorem. However, sometimes the information is of a form that does not simply enable us to evaluate a unique probability distribution p(Y|I). For example, suppose our prior information expresses the following constraint:
I ≡ “the mean value of cos y = 0.6.”
This information alone does not determine a unique p(Y|I), but we can use I to test whether any proposed probability distribution is acceptable. For this reason, we call this type of constraint information testable information. In contrast, consider the following prior information:
I1 ≡ “the mean value of cos y is probably > 0.6.”
This latter information, although clearly relevant to inference about Y, is too vague to be testable because of the qualifier “probably.”
Jaynes (1957) demonstrated how to combine testable information with Claude Shannon's entropy measure of the uncertainty of a probability distribution to arrive at a unique probability distribution. This principle has become known as the maximum entropy principle or simply MaxEnt.
We will first investigate how to measure the uncertainty of a probability distribution and then find how it is related to the entropy of the distribution. We will then examine three simple constraint problems and derive their corresponding probability distributions.
- Type
- Chapter
- Information
- Bayesian Logical Data Analysis for the Physical SciencesA Comparative Approach with Mathematica® Support, pp. 184 - 211Publisher: Cambridge University PressPrint publication year: 2005