Book contents
- Frontmatter
- Contents
- Preface
- 1 Random variables
- 2 Statistical models and inference
- 3 R
- 4 Theory of maximum likelihood estimation
- 5 Numerical maximum likelihood estimation
- 6 Bayesian computation
- 7 Linear models
- Appendix A Some distributions
- Appendix B Matrix computation
- Appendix C Random number generation
- References
- Index
- References
References
Published online by Cambridge University Press: 05 April 2015
- Frontmatter
- Contents
- Preface
- 1 Random variables
- 2 Statistical models and inference
- 3 R
- 4 Theory of maximum likelihood estimation
- 5 Numerical maximum likelihood estimation
- 6 Bayesian computation
- 7 Linear models
- Appendix A Some distributions
- Appendix B Matrix computation
- Appendix C Random number generation
- References
- Index
- References
Summary
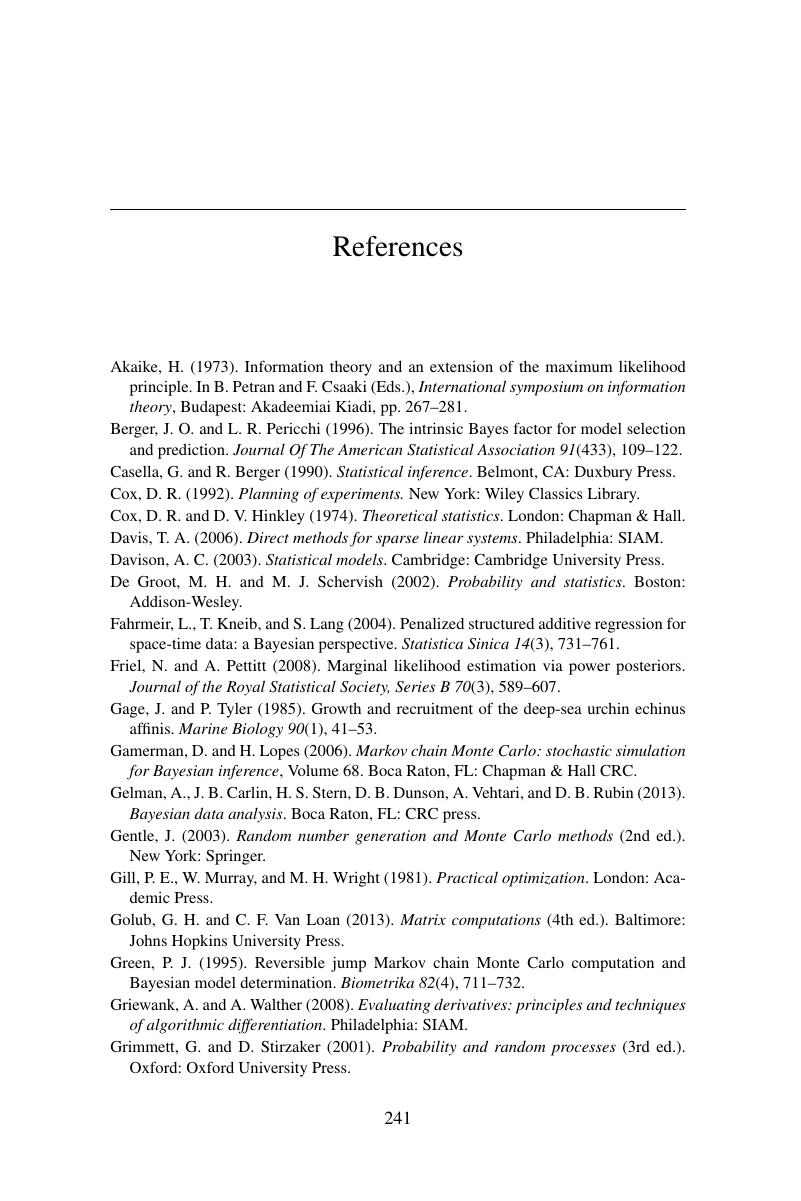
- Type
- Chapter
- Information
- Core Statistics , pp. 241 - 244Publisher: Cambridge University PressPrint publication year: 2015