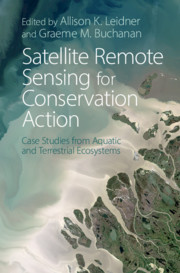
Book contents
- Satellite Remote Sensing for Conservation Action
- Satellite Remote Sensing for Conservation Action
- Copyright page
- Contents
- Contributors
- Preface
- Acknowledgements
- 1 A Brief Introduction to Conservation and Conservation Remote Sensing
- 2 Introduction to Remote Sensing for Conservation Practitioners
- 3 Satellite Remote Sensing for the Conservation of East Asia’s Coastal Wetlands
- 4 Global Forest Maps in Support of Conservation Monitoring
- 5 Wildfire Monitoring with Satellite Remote Sensing in Support of Conservation
- 6 Ecosystem Functioning Observations for Assessing Conservation in the Doñana National Park, Spain
- 7 Predicting Mule Deer Harvests in Real Time
- 8 Lessons Learned from WhaleWatch
- 9 The Evolution of Remote Sensing Applications Vital to Effective Biodiversity Conservation and Sustainable Development
- 10 Operational Conservation Remote Sensing
- Index
- Plate Section
- References
7 - Predicting Mule Deer Harvests in Real Time
Integrating Satellite Remote Sensing Measures of Forage Quality and Climate in Idaho, United States
Published online by Cambridge University Press: 23 July 2018
- Satellite Remote Sensing for Conservation Action
- Satellite Remote Sensing for Conservation Action
- Copyright page
- Contents
- Contributors
- Preface
- Acknowledgements
- 1 A Brief Introduction to Conservation and Conservation Remote Sensing
- 2 Introduction to Remote Sensing for Conservation Practitioners
- 3 Satellite Remote Sensing for the Conservation of East Asia’s Coastal Wetlands
- 4 Global Forest Maps in Support of Conservation Monitoring
- 5 Wildfire Monitoring with Satellite Remote Sensing in Support of Conservation
- 6 Ecosystem Functioning Observations for Assessing Conservation in the Doñana National Park, Spain
- 7 Predicting Mule Deer Harvests in Real Time
- 8 Lessons Learned from WhaleWatch
- 9 The Evolution of Remote Sensing Applications Vital to Effective Biodiversity Conservation and Sustainable Development
- 10 Operational Conservation Remote Sensing
- Index
- Plate Section
- References
Summary
- Type
- Chapter
- Information
- Satellite Remote Sensing for Conservation ActionCase Studies from Aquatic and Terrestrial Ecosystems, pp. 194 - 228Publisher: Cambridge University PressPrint publication year: 2018