Book contents
- Frontmatter
- Contents
- Preface
- Acknowledgements
- Introduction
- Part A The context for spatial data analysis
- Part B Spatial data: obtaining data and quality issues
- Part C The exploratory analysis of spatial data
- Part D Hypothesis testing and spatial autocorrelation
- Part E Modelling spatial data
- Appendix I Software
- Appendix II Cambridgeshire lung cancer data
- Appendix III Sheffield burglary data
- Appendix IV Children excluded from school: Sheffield
- References
- Index
- References
References
Published online by Cambridge University Press: 06 July 2010
- Frontmatter
- Contents
- Preface
- Acknowledgements
- Introduction
- Part A The context for spatial data analysis
- Part B Spatial data: obtaining data and quality issues
- Part C The exploratory analysis of spatial data
- Part D Hypothesis testing and spatial autocorrelation
- Part E Modelling spatial data
- Appendix I Software
- Appendix II Cambridgeshire lung cancer data
- Appendix III Sheffield burglary data
- Appendix IV Children excluded from school: Sheffield
- References
- Index
- References
Summary
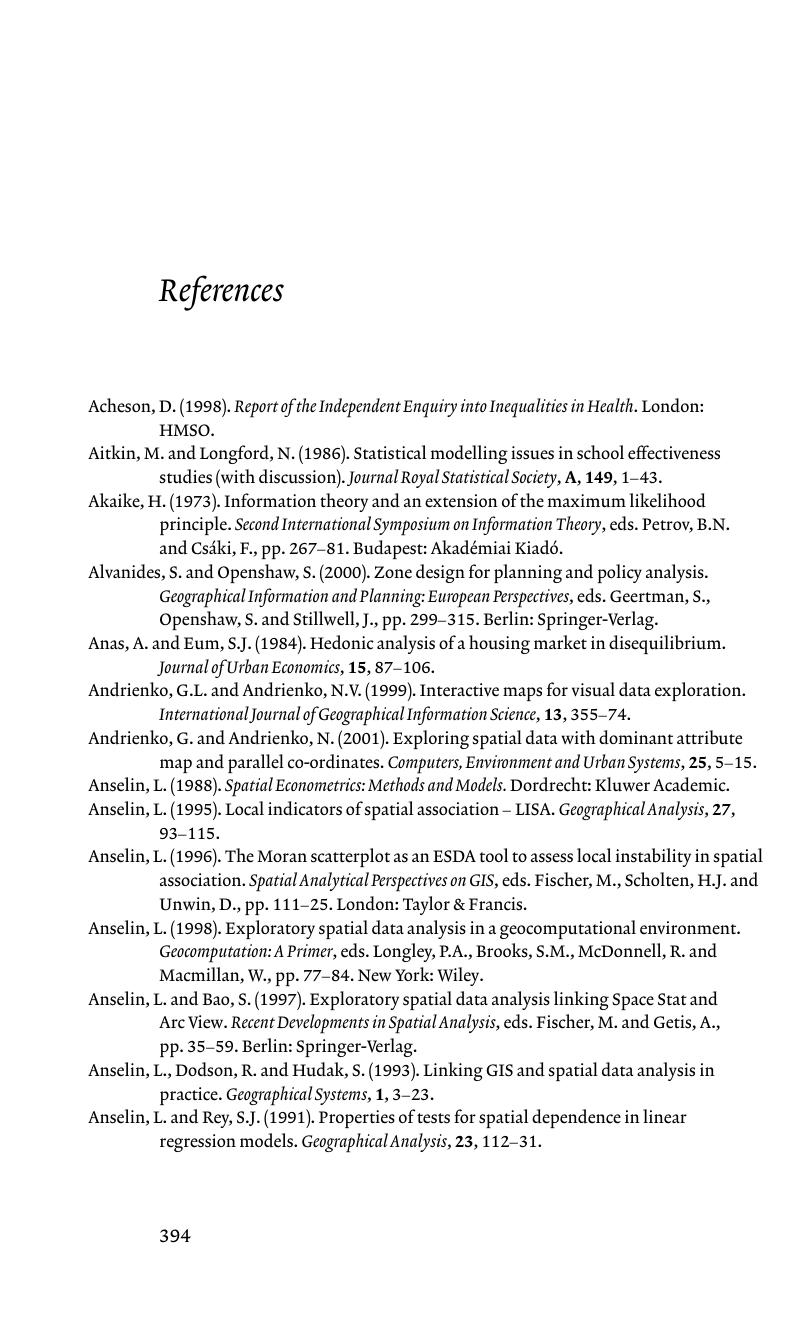
- Type
- Chapter
- Information
- Spatial Data AnalysisTheory and Practice, pp. 394 - 423Publisher: Cambridge University PressPrint publication year: 2003