Book contents
- Frontmatter
- Contents
- Preface
- Nomenclature
- Part I Formulation
- Part II Algorithm
- Part III Nonasymptotic Theory
- Part IV Asymptotic Theory
- Appendix A James–Stein Estimator
- Appendix B Metric in Parameter Space
- Appendix C Detailed Description of Overlap Method
- Appendix D Optimality of Bayesian Learning
- Bibliography
- Index
- References
Bibliography
Published online by Cambridge University Press: 24 June 2019
- Frontmatter
- Contents
- Preface
- Nomenclature
- Part I Formulation
- Part II Algorithm
- Part III Nonasymptotic Theory
- Part IV Asymptotic Theory
- Appendix A James–Stein Estimator
- Appendix B Metric in Parameter Space
- Appendix C Detailed Description of Overlap Method
- Appendix D Optimality of Bayesian Learning
- Bibliography
- Index
- References
Summary
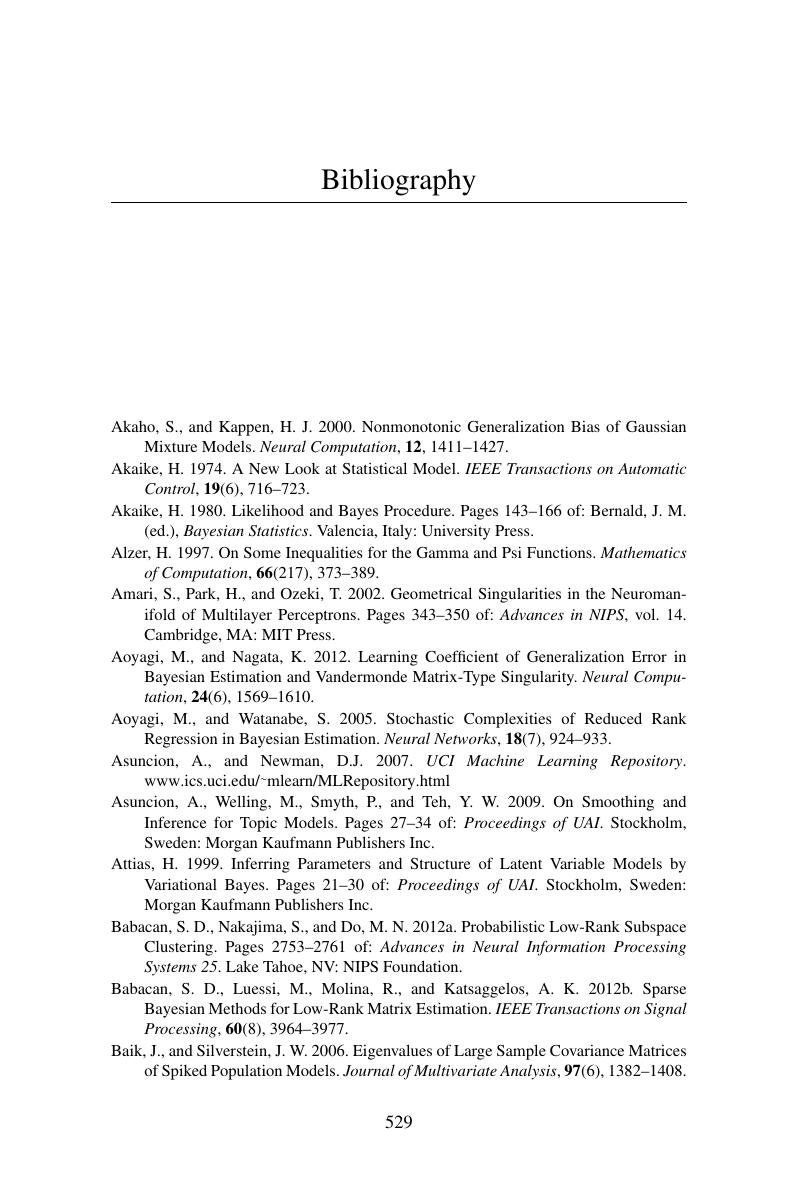
- Type
- Chapter
- Information
- Variational Bayesian Learning Theory , pp. 529 - 539Publisher: Cambridge University PressPrint publication year: 2019