Introduction
For decades, researchers have examined how the bilingual brain supports the fluent and contextually-appropriate use of two languages (i.e., “bilingual language control”). Early studies identified the dorsolateral prefrontal cortex (DLPFC) as a key brain region involved in switching between languages (Hernandez, Reference Hernandez2009; Hernandez et al., Reference Hernandez, Dapretto, Mazziotta and Bookheimer2001). Around the same time, evidence was building with regard to a “bilingual advantage” in cognitive control tasks (i.e., tasks that require executive functions such as conflict monitoring, inhibition, and task-switching), such that bilinguals demonstrated better performance on these tasks than monolinguals (Bialystok, Reference Bialystok2007; Bialystok et al., Reference Bialystok, Craik, Klein and Viswanathan2004; Bialystok & Martin, Reference Bialystok and Martin2004). These lines of research converged in an early theoretical model focused on the overlapping brain networks involved in both bilingual language control and cognitive control (Abutalebi & Green, Reference Abutalebi and Green2007). This model proposed that the prefrontal cortex was a core aspect of the brain network involved in both cognitive control and bilingual language control, which also included the anterior cingulate cortex, inferior parietal lobule, and basal ganglia.
As research on the brain networks connecting bilingual language control to cognitive control has advanced, it has become more nuanced. Many studies now treat bilingualism not as a categorical variable (i.e., bilingual or monolingual), but as a continuum of experience (e.g., considering the duration of bilingual exposure or age of second language acquisition; proficiency in each language; daily use of each language; etc.) (Dash et al., Reference Dash, Joanette and Ansaldo2022; DeLuca et al., Reference DeLuca, Rothman, Bialystok and Pliatsikas2019; Sulpizio et al., Reference Sulpizio, Del Maschio, Del Mauro, Fedeli and Abutalebi2020). More recent studies also make specific hypotheses about the types of cognitive control that may be connected to bilingual experiences (Gosselin & Sabourin, Reference Gosselin and Sabourin2023; Han et al., Reference Han, Wei and Filippi2023; Kałamała et al., Reference Kałamała, Szewczyk, Chuderski, Senderecka and Wodniecka2020; Ng & Yang, Reference Ng and Yang2022). This nuanced approach to understanding the relationship between bilingual experience and cognitive control is based on updated neurocognitive models, including the Adaptive Control Hypothesis (Green & Abutalebi, Reference Green and Abutalebi2013). This model expanded the network of brain regions thought to be involved in both language control and cognitive control to include not only the prefrontal cortex, anterior cingulate cortex, inferior parietal lobule, and basal ganglia (Abutalebi & Green, Reference Abutalebi and Green2007), but also the pre-supplemental motor area, inferior frontal cortex, thalamus, and cerebellum (Green & Abutalebi, Reference Green and Abutalebi2013). Importantly, this model is more specific in connecting regions of this brain network to bilingual experience and cognitive control. In the example above, bilinguals who spend most of their time in monolingual environments may have enhanced inhibition skills, which would be reflected in the left prefrontal and inferior frontal cortex. In a more recent review, the authors expand on the role of each region within the overlapping bilingual language control and cognitive control network, highlighting the role of the right prefrontal cortex in response inhibition and the left prefrontal cortex in response selection (Calabria et al., Reference Calabria, Costa, Green and Abutalebi2018).
Still, theories focused on the neural connection between bilingual experience and cognitive control have not been thoroughly tested (see Tao et al., Reference Tao, Wang, Zhu and Cai2021 for review). A few studies have identified overlap in brain activity in specific regions for bilingual language switching and domain-general task-switching (Anderson et al., Reference Anderson, Chung-Fat-Yim, Bellana, Luk and Bialystok2018; De Baene et al., Reference De Baene, Duyck, Brass and Carreiras2015; Weissberger et al., Reference Weissberger, Gollan, Bondi, Clark and Wierenga2015). The findings of these studies align with the models above: that frontal, parietal, and subcortical regions are involved in both types of switching. A limitation of these previous fMRI studies is that they examine brain activity in terms of relatively large clusters of voxels that co-activate, which averages across subjects and blurs the voxel-wise patterns of brain activity that may distinguish between task conditions (e.g., switching compared to staying) within each subject, but are idiosyncratic to the individual subject's anatomy, their function-structure correspondence, and the registration of the voxel sampling matrix to their brain structure. In other words, these studies reflect regions of the brain that are associated with both language control and cognitive control on average across a group of participants; they are not able to fully capture individual differences in patterns of brain activity at the voxel-level within regions of interest. Presumably, if there is a mechanism connecting bilingual language control and cognitive control, it may be reflected within an individual bilingual's brain at fine scale, even if it is not observed in comparisons of task-switching and language-switching that involve blurring voxels and averaging across participants’ differing function-structure correspondence and differing anatomy. To detect such fine-scale patterns using fMRI, it is necessary to turn to analysis methods that examine patterns of activation at the scale of individual voxels rather than large regions.
As the field has moved towards a more nuanced approach to understanding the bilingual brain, an important next step is to consider within-subject patterns of activity related to both language switching and task switching. If within-subject patterns of activity are similar for bilingual language switching and task switching, this could strengthen theories about the neural mechanisms connecting these two skills, which would ultimately support theories like the Adaptive Control Hypothesis (Green & Abutalebi, Reference Green and Abutalebi2013). Alternatively, if within-subject patterns of activity are not similar for bilingual language switching and task-switching, this could lend support to theories that suggest that bilingual language use may as a process be distinct from non-linguistic cognitive control (see Calabria et al., Reference Calabria, Baus and Costa2019 for review).
Current study
The current study used multi-voxel pattern analysis (MVPA) of fMRI activity during a language switching task and a non-linguistic switching task. This approach has two advantages over traditional fMRI analyses. First, it captures patterns of activity within each participant, and therefore it does not assume that groups of participants will have similar patterns of activity for switching. Second, it focuses on patterns of activity within a region of interest, rather than local clusters of activity, associated with a specific task condition. Therefore, identified patterns of activity can be widespread, but need to be consistent within an individual at an above-chance level. To simplify our analyses and interpretation, we focused our analyses on the DLPFC because it is one of the central regions of the brain that is proposed to be recruited for both language switching and task switching by models such as the Adaptive Control Hypothesis (Green & Abutalebi, Reference Green and Abutalebi2013).
First, we were interested in whether language switching was associated with identifiable patterns of activity in the DLPFC for individual bilinguals. In other words, is the brain activity for language switching compared to staying in a single language distinct enough within the DLPFC for an algorithm to reliably identify the task condition from the pattern of DLPFC activity? This could be true even if the patterns of activity for language switching within the DLPFC differed significantly from person to person: as long as, within each person, there were consistent, identifiable voxel-scale patterns of brain activity within the DLPFC for language switching compared to using a single language.
Second, we asked whether task-switching was associated with similar patterns of activity in the DLPFC. Again, this could be true even if the patterns of brain activity differed substantially between individuals, as long as there were consistent, identifiable patterns of brain activity in the DLPFC for task-switching compared to staying within an individual.
Finally, we assessed whether patterns of DLPFC activity associated with non-linguistic switching were more identifiable for monolinguals than for bilinguals. This was the only bilingual/monolingual comparison we performed in order to connect the findings of this study to previous research and theories, which suggest that reduced differences between switching and not switching task conditions for bilinguals may reflect more efficient task-switching (Abutalebi et al., Reference Abutalebi, Della Rosa, Green, Hernandez, Scifo, Keim, Cappa and Costa2012; Berroir et al., Reference Berroir, Ghazi-Saidi, Dash, Adrover-Roig, Benali and Ansaldo2017). If language switching and task-switching are associated with similar patterns of neural activity for bilinguals, and this pattern of activity differs from monolinguals, these results could add to the body of evidence to support the idea that the DLPFC is a region of the brain that may facilitate transfer between language control and cognitive control, possibly leading to a bilingual advantage.
Method
Participants
Forty-nine right-handed Spanish–English bilingual college students and forty-five right-handed English monolingual college students participated in the fMRI study. These students were recruited through psychology classes and flyers placed around a university campus. All Spanish–English bilinguals learned Spanish as a native language and learned English simultaneously as a native language or later in life as a second language. English monolinguals were not exposed to any language other than English in their homes during childhood, did not travel extensively (i.e., more than one month) to a non-English-speaking country, and did not rate their proficiency in any non-English language higher than 2 on a 7-point scale where 1 = no proficiency and 7 = better than a native speaker. Participants were compensated with either course credit or a gift card. Demographic and language background information for participants is presented in Table 1.
Table 1. Participant Demographics

Note. Socioeconomic status was defined as parental education on a scale of 1-6, where 1 = Some elementary school or less, 2 = Some high school or less, 3 = High school graduate, 4 = Some college, 5 = College graduate, 6 = Advanced degree. English and Spanish picture vocabulary and passage comprehension were measured using the Woodcock-Muñoz Language Survey – Revised and are presented here as the percentage of correct responses out of the total number of items on each measure.
fMRI tasks
Picture-naming task
The picture-naming task, as the name implies, involved verbally producing the name of pictures presented on the screen in a cued language. The two language cues were “diga” and “say” indicating Spanish and English responses, respectively. This task had three different conditions: English only, Spanish only, and mixed (see Figure 1). In the English only condition, every trial was preceded by the “say” cue and every response was the English name for the picture. In the Spanish only condition, every trial was preceded by the “diga” cue and every response was the Spanish name for the picture. In the mixed condition, the cues alternated on every trial (“diga” then “say” then “diga” then “say”, etc.). Each of these conditions was presented in a block lasting one minute and 20 seconds. Participants completed 2 runs of the task, where each run included 1 English-only block, 1 Spanish-only block, and 2 mixed blocks, presented in a randomized order.

Figure 1. Picture-naming task design. Each run contained 2 mixed blocks, 1 Spanish block (“diga” cues), and 1 English block (“say” cues). Bilingual participants completed 2 runs of this task.
Shape-color task
The shape-color task presented participants with red/blue circles/squares and asked them to respond to either the color or the shape of the image they saw on the screen. If the response rule was color, participants pressed one button for red shapes and one button for blue shapes. If the response rule was shape, participants used the same two buttons, but pressed one if the image was a circle and one if it was a square. The response rule was written on the screen at the beginning of the task, but could change or stay the same depending on cues presented throughout the task every 8-12 trials (see Figure 2). These cues were a horizontal and vertical dollar sign. A horizontal dollar sign indicated that participants should continue following the same rule (Shape-Shape or Color-Color). A vertical dollar sign indicated that participants should switch rules (Shape-Color or Color-Shape). Trials were presented for 500ms each and cues were presented for 1000ms each. Before and after each trial, a blank screen appeared for 1000ms, during which participants would respond. Each run of this task involved 14 cues (7 switch and 7 non-switch) and lasted 4.5 minutes. Participants completed a practice run of the task outside of the scanner to ensure they understood the instructions, and then completed 5 runs of this task in the fMRI scanner.

Figure 2. Shape-Color task design. Each run contained 7 switch cues and 7 non-switch cues separated by 8-12 trials. Bilingual and monolingual participants completed 5 runs of this task.
Procedure
The authors assert that all procedures contributing to this work comply with the ethical standards of the relevant national and institutional committees on human experimentation and with the Helsinki Declaration of 1975, as revised in 2008. Participation involved a lab session to screen participants, followed by two MRI sessions for bilingual participants who qualified, and one MRI session for monolingual participants who qualified. Participants qualified if they were Spanish–English bilinguals or English monolinguals, right-handed, had no uncorrected vision or hearing problems, reported no psychological diagnoses, were not taking psychoactive medications, and reported no safety concerns on the MRI screening form.
Bilingual participants who qualified completed the picture-naming task during the first MRI session. MRI data for this study were collected on a 3.0 Tesla (3T) Seimens Magnetom Trio scanner. During this session, the researchers collected a localizer scan to ensure proper placement of the head in the scanner, one T1-weighted MPRAGE anatomical scan (field of view = 245 mm, voxel size = 1.0 x 1.0 x 1.0 mm3, 192 slices, sequential ascending acquisition in the transversal orientation, TR = 1200ms, TE = 2.66 ms, flip angle = 12 deg), and two sequences of BOLD echo-planar imaging (EPI) scans during the two runs of the picture-naming task (field of view = 220 mm, voxel size = 3.4 x 3.4 x 4.0 mm3, 34 slices, interleaved descending acquisition in the transversal orientation, TR = 2500 ms, TE = 30 ms, flip angle = 90 deg). The participants were asked to produce the names of pictures aloud. The order of the conditions (English-only, Spanish-only, and mixed) within each run was counterbalanced across participants.
Both bilinguals and monolinguals completed the cognitive control MRI session. During this session, researchers explained the tasks to participants at a computer outside of the scanner and allowed them to practice to ensure they understood the directions. Then, while participants were in the scanner, the researchers collected another localizer scan, one T1-weighted MPRAGE anatomical scan from bilingual participants and two anatomical scans from monolingual participants with the same parameters as the T1-weighted MPRAGE anatomical scan described above. Researchers also collected a series of BOLD EPI scans during each block of the shape-color task (field of view = 220 mm, voxel size = 3.4 x 3.4 x 4.0 mm3, 34 slices, interleaved descending acquisition in the transversal orientation, TR = 2500 ms, TE = 30 ms, flip angle = 90 deg), as well as the Simon task, which will not be analyzed for the purposes of this study. Participants completed five blocks of the shape-color task lasting about four and a half minutes each. Participants responded using buttons on an MRI-compatible button box that they held during the scanning. Task order, block order, and response-button assignment were all counterbalanced across participants. Participants were compensated for their completion of the MRI sessions.
Analyses
Response times and accuracies
To help contextualize this study within the broader literature, we calculated shape-color switch costs in terms of response time and accuracy. Switch costs were defined as the difference in response time and accuracy for the first trial following the switch cue compared to the first trial following the non-switch cue. We do not have behavioral data for the picture-naming task, as we were unable to record voices and voice onset times within the context of scanner noise with the available equipment.
Preprocessing
First, the data for each participant were preprocessed using Statistical Parametric Mapping software (SPM12, https://www.fil.ion.ucl.ac.uk/spm/software/spm12/), which involved slice-timing correction, motion correction, co-registration to anatomical scans, segmentation, and normalization to place each participant's data in MNI space. We observed a striping/banding artifact in some of the images. To remove this artifact, the data were despiked using a 17-tap high pass filter (robust for noisy images) from the ArtRepair toolbox in SPM (Mazaika et al., Reference Mazaika, Whitfield and Cooper2005). For persistent artifacts, we applied an independent component analysis (ICA) GIFT toolbox (v3.0b) in SPM (Calhoun et al., Reference Calhoun, Liu and Adalı2009) to isolate the component that best represented the artifact. Additional details are provided in Appendix S1 and Figure S1. After both despiking and ICA were applied to remove the striping/banding artifact, the sample of participants with usable data for the shape-color task consisted of 45 bilinguals and 40 monolinguals and for the picture naming task consisted of 44 bilinguals. The number of bilinguals who had usable data for both tasks was 41.
Calculating betas
After preprocessing, the images were used to calculate beta-values representing the neural activity in each voxel of the brain associated with each task condition in each run. For the shape-color task, participants saw a cue that told them to switch from one sorting rule to another or a cue that told them to continue sorting by the same rule. These cues appeared after every 8-12 trials in an event-related design so that the activity associated with switching and not switching could be deconvolved. The picture-naming task had a mixed/switch block in which participants switch languages on every trial and two single-language/non-switch blocks (English and Spanish) in which participants use only a single-language to name the pictures. This task used a blocked design. Each of these task conditions was associated with stimulus onset times that were entered into SPM as vectors for each subject (see Table 2).
Table 2. All regressors for fMRI analyses

Note. The MVPA classifier was trained to distinguish the conditions of each task.
Regions of interest
For both tasks, neural activity in the form of beta vectors were extracted from the left and right DLPFC (see Figure 3), which we identified as parcels from the frontoparitetal community and the dorsal attentional community (Gordon et al., Reference Gordon, Laumann, Adeyemo, Huckins, Kelley and Petersen2016) that were located within the middle frontal gyrus (based on the AAL atlas in SPM) (Tzourio-Mazoyer et al., Reference Tzourio-Mazoyer, Landeau, Papathanassiou, Crivello, Etard, Delcroix, Mazoyer and Joliot2002). See Table S1 for a list of included functional parcellations from Gordon et al. (Reference Gordon, Laumann, Adeyemo, Huckins, Kelley and Petersen2016). These parcels were projected onto each subject's brain for analyses. The beta values associated with each task condition, separately for each individual voxel in the parcels/ROIs, were used as input to the MVPA algorithm.
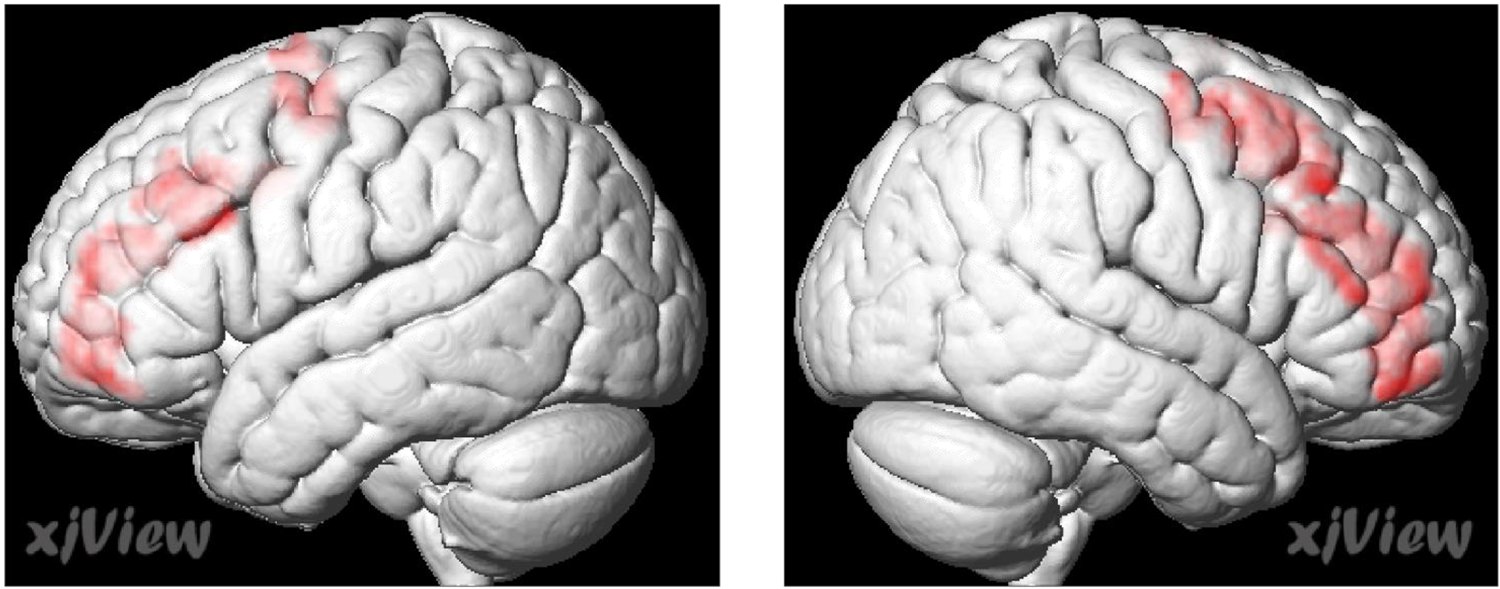
Figure 3. Dorsolateral prefrontal cortex mask in each hemisphere (in red). The mask was created based on the regions that exist within the frontoparietal and dorsal attentional communities developed by Gordon and colleagues (Reference Gordon, Laumann, Adeyemo, Huckins, Kelley and Petersen2016) that overlap with the middle frontal gyrus in SPM's AAL atlas. Image created using the xjview toolbox (http://www.alivelearn.net/xjview).
MVPA
MVPA is a multivariate method for analyzing fMRI data, meaning that it takes into consideration the correlations between different voxels in the brain (Davis & Poldrack, Reference Davis and Poldrack2013). Typical (univariate) approaches to analyzing fMRI data assume that activity in each voxel of the brain is independent from activity in each other voxel, which may ignore some of the more complex patterns of activation elicited by a stimulus (Davis & Poldrack, Reference Davis and Poldrack2013). MVPA involves a machine learning technique in which neural activity from all the voxels within the region(s) of interest – in this case, the bilateral DLPFC – are given to a classifier, along with labels indicating the task condition associated with that pattern of neural activity (known as a “beta vector”) for each subject. When this information is entered for multiple experimental runs (known as “training the classifier”), the classifier will develop a function that distinguishes between the different task conditions. Then, in the testing phase, the classifier is given new neural activity data and uses the function it created to label these data as one of the task conditions (Haxby et al., Reference Haxby, Connolly and Guntupalli2014). The accuracy with which the classifier can label the new data is dependent on the consistency within the training data and between the training data and the testing data. If there are very clear differences between the patterns of activity for each task condition within each subject, and these differences are evident in both the training and testing data, then the classifier will be very accurate.
We used a leave-one-run-out analysis within each task. The training data consisted of a subset of the data from a single task for a single participant (e.g., 4/5 blocks of the shape-color task), and the testing data consisted of the rest of the data from the same task for the same participant (e.g., the remaining block of the shape-color task). This was repeated for both tasks, and classifier accuracy was calculated across all participants to determine how distinct the patterns of neural activity were for each condition within each task.
To evaluate classifier accuracy, we used permutation testing. During permutation testing, the classifier was trained and tested multiple times in order to create a distribution of classifier accuracy that could be used as a basis for calculating p-values. There are multiple approaches for permutation testing; for the proposed study, a “data-wise” scheme, which involves relabeling the beta vectors, was used. The complete dataset that had beta vectors for each participant was randomly relabeled so that the task condition labels were assigned to random beta vectors within the set. The classifier was then trained and tested on this relabeled dataset in the same manner as it was trained and tested on the correctly labeled dataset and classifier accuracy was calculated. This was repeated 1000 times in order to create a distribution. The mean of the classifier accuracy for the correctly labeled data for each subject was compared to the mean of the classifier accuracy from the 1000 simulations for each subject in a paired t-test using R studio (Team, 2015) in order to determine whether the classifier was significantly better at distinguishing between the task conditions when they were correctly labeled compared to when they were randomly labeled.
Bilingual language switching analyses
To evaluate the patterns of activity for language-mixing compared to single-language use, neural activity in the ROIs for these tasks was extracted from the bilingual sample. As explained above, the classifier received the beta vectors for the language-mixing and single-language conditions of one run of the picture naming task along with the correct labels for these beta vectors (language-mixing or single-language). The classifier developed an algorithm to distinguish between the two conditions and was then presented with the language-mixing and single-language beta vectors from the other run of the picture-naming task and made judgments about whether each beta vector was associated with the language-mixing or single-language condition. Permutation testing was then conducted to create a distribution of classifier accuracies based on scrambled-label data. If the classifier associated with the correctly labeled data was significantly higher than the classifier accuracies based on scrambled-label data, this would mean that there were distinguishable patterns of neural activity for language-mixing compared to single-language use for bilinguals.
Bilingual and monolingual task-switching analyses
Next, the MVPA classifier accuracy for the fMRI activity associated with the shape-color task was compared between bilinguals and monolinguals. Thus, the classifier was trained to distinguish between the neural activity associated with switching and non-switching on 4 of the 5 runs of the task within each individual and tested on the remaining run. This was repeated until each of the 5 runs was used as the test data. Then, an accuracy measure for each person was computed as the mean of the accuracies of each fold of the leave-one-run-out analysis. Classifier accuracy for bilinguals and monolinguals was then compared using an ANOVA to test the hypothesis that the classifier would be more accurate at distinguishing between switch and non-switch activity (compared to scrambled-label data classifier accuracies) for monolinguals than for bilinguals. Task switching compared with non-switching should result in very different patterns of neural activity in the DLPFC, but if those patterns are more similar in bilinguals, this could be used as support for the notion that bilingualism changes the way the brain handles switching, even in non-linguistic contexts.
Cross-task analyses
The question of whether language switching and non-linguistic switching rely on the same neural substrates within bilinguals was tested by training the classifier on the shape-color task and testing the classifier on the picture-naming task, as well as the reverse: training the classifier on the picture-naming task and testing the classifier on the shape-color task. Permutation testing was then conducted to create a distribution of classifier accuracies based on scrambled-label data. If the classifier associated with the correctly labeled data was significantly higher than the classifier accuracies based on scrambled-label data, this would mean that language switching and non-linguistic switching involved overlapping patterns of neural activity for bilinguals.
Results
Task performance for the shape-color task was assessed in terms of accuracy and responses times for trials immediately following a switch cue compared to trials immediately following a non-switch cue. This difference resulted in measures of accuracy costs and response time costs for each participant. There were no significant differences in accuracy costs for bilinguals (M = 7.21%; SD = 10.58%) compared to monolinguals (M = 6.39%; SD = 9.17%); (t(68.91) = −0.38, p = 0.70). There was a non-significant trend towards larger response time costs for bilinguals (M = 51.03ms; SD = 45.79ms) compared to monolinguals (M = 29.50ms; SD = 58.41ms); (t(68.91) = −1.86, p = 0.07). Further exploration of the relationship between accuracy and response time costs and patterns of DLPFC activity is included in the “Bilingual and Monolingual Task Switching” section below.
All MVPA analyses were conducted first without mean subtraction and then with mean subtraction. Mean subtraction removes univariate differences from the results. For example, it is possible that within the sub-regions of the DLPFC, fMRI activity patterns for the task conditions may be similar, but there may be more activity overall for one condition than the other. These types of differences would appear in a univariate comparison of task conditions and would be erased using mean subtraction. On the other hand, it is possible that the task conditions are related to different patterns of activity rather than mean differences in the level of fMRI activity. These differences would only appear in a multivariate comparison (i.e., MVPA) and would persist after mean subtraction. The results with and without mean subtraction were not different in any meaningful ways, so they will be discussed together and only the results with mean subtraction are presented in the tables.
Bilingual language switching
The classifier accuracy for distinguishing between language-mixing and single-language activity during the picture naming task was measured within the left and right DLPFC. Classifier accuracy for correctly-labeled data and simulated data (based on scrambled labels) was compared using a paired t-test. A Bonferroni correction was applied to correct for multiple comparisons (Bonferroni corrected alpha = 0.025). The classifier was not better able to predict whether the participant was mixing languages or using a single language within the DLPFC (Left DLPFC: t(43) = 0.91, p = 0.37, Cohen's d = 0.14; Right DLPFC: t(43) = −0.90, p = 0.36, Cohen's d = −0.14). This leads to the conclusion that language-mixing and using a single language were related to indistinguishable patterns of fMRI activity for bilinguals.
Bilingual and monolingual task-switching
The classifier accuracy for the bilinguals was compared to the classifier accuracy for the monolinguals in order to determine whether the patterns of fMRI activity associated with seeing the switch cue were more or less dissociable from the patterns of fMRI activity associated with seeing the non-switch cue for the monolingual group. The hypothesis was that accuracy would be higher for monolinguals than bilinguals. These results were analyzed using a 2-way mixed ANOVA, with language group as a between-subjects variable and data type (real or scrambled data) as a within-subjects variable. The outcome variable was classifier accuracy and a Bonferroni correction was applied (Bonferroni corrected alpha = 0.025). For both bilinguals and monolinguals, the classifier was able to distinguish between a switch and non-switch cue in the left DLPFC (Main effect of data type: F(1,82) = 10.22, p = 0.002; Main effect of language group: F(1,82) = 0.41, p = 0.52; Interaction: F(1,82) = 0.36, p = 0.55; see Figure 4). There was an interaction between datatype (real vs. scrambled) and language group in the right DLPFC (Main effect of datatype: F(1,82) = 0.98, p = 0.33; Main effect of language group: F(1,82) = 4.28, p = 0.04; Interaction: F(1,82) = 3.96, p = 0.05), which did not survive Bonferroni correction. This interaction was in the direction of greater accuracy for real vs. scrambled data for the bilinguals than for the monolinguals.

Figure 4. Classifier accuracy for real and scrambled shape-color task data in the left DLPFC for the shape-color task. Both bilinguals and monolinguals had greater-than-chance classifier accuracy in the left DLPFC. This means that for both bilinguals and monolinguals, the patterns of fMRI activity in the left DLPFC for switching tasks was distinct from the fMRI activity for staying with one task.
To explore some of the variability across participants, we conducted follow-up multiple linear regressions with classifier accuracy as the outcome variable and language group, switch cost, and the interaction between language group and switch cost as the predictors. Four models were conducted to reflect classifier accuracy for both hemispheres and both types of switch cost (response time and accuracy). The interaction between language group and switch cost was not significant in any model, indicating that the relationship between classifier accuracy and switch cost did not differ significantly between bilinguals and monolinguals. Instead, the models suggest that the trend reported above – that the classifier was more accurate at identifying patterns of right DLPFC activity associated with switching for bilinguals than for monolinguals – remained when controlling for task performance. No other relationships were significant (see Table 3).
Table 3. Models of classifier accuracy for bilingual and monolingual non-linguistic switching controlling for task performance

Cross-task results
The classifier was not successful at decoding switch and non-switch fMRI activity when it was trained on one task and tested on the other task (see Table 4). These results suggest that the patterns of neural activity within the DLPFC for bilingual language switching are not similar to the patterns of neural activity for bilingual task-switching.
Table 4. Cross-Task Real & Scrambled Data Differences Bilinguals

Discussion
This study aimed to test the hypothesis that bilingual language switching is related to non-linguistic task-switching through overlapping patterns of activity within the DLPFC. If this were true, it would support the Adaptive Control Hypothesis (Green & Abutalebi, Reference Green and Abutalebi2013), which states that experience with bilingual language control shapes the brain networks involved in non-linguistic or domain-general cognitive control. The results of this MVPA study did not overwhelmingly support the hypotheses nor the theory that bilingual experiences with switching languages can transfer to non-linguistic switching via the action of the DLPFC. The classifier was not able to distinguish between language-mixing and single-language use based on patterns of activity within the DLPFC. The lack of classifier accuracy for the picture-naming task could be interpreted as similar patterns of activity in the DLPFC for mixing languages and using a single language. Multiple studies on bilingual and monolingual cognitive control have interpreted less neural activity in response to a task as increased efficiency, which suggests easier neural processing of the task information (Abutalebi et al., Reference Abutalebi, Della Rosa, Green, Hernandez, Scifo, Keim, Cappa and Costa2012; Berroir et al., Reference Berroir, Ghazi-Saidi, Dash, Adrover-Roig, Benali and Ansaldo2017). From this perspective, results of the current study suggest that, at least when considering patterns of DLPFC activity, the bilinguals have become efficient at language switching, but not task-switching, resulting in a decreased amount of control-related fMRI activity for the picture-naming task only.
Next, we aimed to determine whether there were significant differences in classifier accuracy between bilinguals and monolinguals for task-switching. In the left DLPFC, the classifier was able to distinguish between switch and non-switch fMRI activity for both bilinguals and monolinguals. In the right DLPFC, bilinguals appeared to have better classifier accuracy than for monolinguals, which did not survive Bonferroni correction, but remained when controlling for task performance (i.e., switch costs). This finding runs counter to the hypothesis that the classifier would be less able to distinguish between switch and non-switch activity for the bilinguals because they would be more efficient at switching. When paired with the previous finding that the classifier was unable to distinguish switch and non-switch activity for bilinguals during the picture-naming task, our results do not suggest that bilingual language switching experience trains the DLPFC in a way that transfers to non-linguistic tasks.
Finally, we examined whether there were consistent cross-task patterns of activity within the DLFPC for bilingual language switching and task-switching. The classifier was not able to distinguish between switch and non-switch activity within either the left or right DLPFC when trained on one task and tested on the other task. This indicates that the patterns of DLPFC activity for language switching and task-switching are not overlapping, at least for the picture-naming and shape-color tasks included in this study. While some theories and previous research suggest that the “bilingual advantage” may be explained by an overlap between the brain regions involved in language control and cognitive control (Abutalebi & Green, Reference Abutalebi and Green2007; Anderson et al., Reference Anderson, Chung-Fat-Yim, Bellana, Luk and Bialystok2018; Coderre et al., Reference Coderre, Smith, Van Heuven and Horwitz2016), there is limited evidence that the patterns of activity for these types of tasks overlap within those brain regions. If similar brain regions are involved in both language control and cognitive control, but the patterns of activity within these brain regions differ, it is still difficult to conclude that overlapping brain activity is the mechanism that connects bilingual language control and cognitive control.
Other research continues to raise questions about the relationship between language switching and task-switching (Calabria et al., Reference Calabria, Baus and Costa2019; Paap et al., Reference Paap, Mason and Anders-Jefferson2021). In 2015, Weissberger and colleagues found widespread differences in fMRI activity for the non-switch conditions of a picture-naming task and a shape-color task – claiming that bilinguals were staying, not switching experts. Differences in non-switch activity, as Weissberger and colleagues (Reference Weissberger, Gollan, Bondi, Clark and Wierenga2015) observed, could explain why our classifier was unable to distinguish between switch and non-switch activity across tasks and within the language switching task. Behavioral research also calls into question the relationship between language switching and task-switching (Branzi et al., Reference Branzi, Calabria, Boscarino and Costa2016), including findings of enhanced performance for bilingual language switching associated with poorer performance on shape-color task switching (Segal et al., Reference Segal, Stasenko and Gollan2019).
In a recent study, we applied anodal (i.e., excitatory) transcranial direct current stimulation (tDCS) to the left and right DLPFC before language switching task and task-switching (Vaughn et al., Reference Vaughn, Watlington, Linares Abrego, Tamber-Rosenau and Hernandez2020). Results indicated that excitatory stimulation to the left DLPFC improved language switching, but worsened performance for task-switching. Further, left DLPFC stimulation impacted task-switching performance for bilingual and monolingual participants similarly. These previous findings confirm that the DLPFC is involved in language switching, but run counter to the argument that bilingual experience strengthens the DLPFC in a way that transfers to improved task-switching performance.
The results of the current study align well with the findings of the tDCS study described above. In the current study, we found that patterns of activity within the DLPFC did not differ between language-mixing and single-language use, but they did differ between the switch and non-switch conditions of a non-linguistic switching task. Within the left DLPFC, there were identifiable patterns of switch and non-switch activity for both bilinguals and monolinguals. In sum, these findings suggest that the DLPFC does not facilitate transfer from language switching to task-switching for bilinguals, at least at the level of spatial resolution we were able to detect in the current study. As mentioned in the introduction, the MVPA approach employed here was ideal for capturing voxel-level differences in activity for switching versus staying within individual bilingual and monolingual participants. We focused on the DLPFC rather than conducting a whole-brain analysis because the DLPFC is an essential region in models that connect bilingual experience to cognitive control (Green & Abutalebi, Reference Green and Abutalebi2013), which includes task switching. Future research could use ultra-high-field fMRI to enhance the spatial resolution of the DLFPC imaging. Future research should also continue to explore patterns of activity in other candidate regions or networks, such as the basal ganglia, anterior cingulate cortex, and inferior parietal lobule (Abutalebi & Green, Reference Abutalebi and Green2007) in order to identify a neural mechanism that may connect bilingual language switching to task-switching. Identification of such a neural mechanism would greatly strengthen theories connecting bilingual language control to a “bilingual advantage” in task-switching.
In addition to research on the bilingual advantage, these results have implications for theories about bilingual language control. There are competing theories about the cognitive and neural mechanisms associated with bilingual language control (Calabria et al., Reference Calabria, Baus and Costa2019; Costa et al., Reference Costa, Santesteban and Ivanova2006; Kroll et al., Reference Kroll, Bobb, Misra and Guo2008). On the one hand, language-specific selection models suggest that selecting the context-appropriate words in either language rely on the same neurocognitive mechanisms that a monolingual would use to select among words within a single language. These models suggest that knowing two languages does not create conflict or competition, but simply leads to a larger set of possible words to select from when producing language. On the other hand, language non-specific models assume that knowing two languages creates conflict or competition among translation equivalents, and inhibiting one language is necessary to select the appropriate words. Our hypotheses here aligned with those of language non-specific models: we expected that the DLPFC would be similarly involved in language switching and non-linguistic task switching because of its role in a domain-general cognitive control network that is necessary for both types of switching. However, the results of this study instead may support language-specific models, as we did not identify a clear relationship between language switching and non-linguistic switching. Because distinguishing between these types of models was not an aim of the current study, this interpretation is limited, but future research on the relationship between language control and cognitive control may also strengthen evidence for or against language-specific selection theories and language non-specific selection theories.
Limitations
The fMRI tasks used in this study to assess language switching and task-switching differ in task design, which may have contributed to a lack of classifier accuracy for the picture-naming task compared to the shape-color task. The shape-color task consisted of five runs, whereas the picture-naming task consisted of only two runs. A larger number of runs for the shape-color task may have allowed the classifier to filter out more of the noise from the data than was possible for the two runs of the picture-naming task. It is also notable that the fMRI data from the picture-naming task included more artifacts than the fMRI data from the shape-color task, likely because of head motion when participants were naming the pictures in the scanner. In addition, we were unable to collect behavioral data for the picture-naming task because the scanner noise made it impossible to collect voice recordings and voice onset times. In all studies of bilingual cognitive control, the conclusions are limited by the population of bilinguals and monolinguals studied. In the current manuscript, Spanish–English bilingual young adults were compared to English monolingual young adults. In addition to having different language experiences, our sample of bilinguals had lower socioeconomic status than our sample of monolinguals. This is common in comparisons of Spanish–English bilinguals and English monolinguals in the U.S., but is not representative of all groups of bilinguals and monolinguals, so it is important for future research to replicate these findings with different bilingual and monolingual populations (e.g., Spanish–Catalan bilinguals, French–English bilinguals, etc.).
Acknowledgements
We would like to thank Maya Greene, Aurora Ramos-Nuñez, and Brandin Munson for their role in collecting the data described in this manuscript. Research reported in this publication was supported by the National Institutes of Health to the National Institute on Aging under Award Number R21AG063537 as well as to the National Institutes of Child Health and Human Development under the Award Number P50HD052117. The content is solely the responsibility of the authors and does not necessarily represent the official views of the National Institutes of Health.
Supplementary Material
For supplementary material accompanying this paper, visit https://doi.org/10.1017/S1366728923000834
Supplementary Files
Appendix S1. Description of artifact removal procedure.
Figure S1. Example of component representing the striping/banding artifact that was removed from fMRI data
Table S1. Functional Parcellations Selected from Gordon et al. (Reference Gordon, Laumann, Adeyemo, Huckins, Kelley and Petersen2016)