Negative political communication has been increasingly utilized by US presidential campaigns. Most notably, this approach has been deployed in attack advertisements on television, and a great deal of literature exists debating its effectiveness (Lau, Sigelman, and Rovner Reference Lau, Sigelman and Rovner2007). Given the billion-dollar industry it has now become (Fowler and Ridout Reference Fowler and Ridout2013; Fowler, Ridout, and Franz Reference Fowler, Ridout and Franz2016), televised advertising continues to be the focus of this debate (see, e.g., Lipsitz and Geer Reference Lipsitz and Geer2017; Malloy and Pearson-Merkowitz Reference Malloy and Pearson-Merkowitz2016; Mattes and Redlawsk Reference Mattes and Redlawsk2014). However, presidential campaigns have more recently expanded into new platforms, such as social media, to reach, persuade, and mobilize the mass audiences these platforms house (Conway, Kenski, and Wang Reference Conway, Kenski and Wang2013; Filimonov, Russmann, and Svensson Reference Filimonov, Russmann and Svensson2016; Wattal et al. Reference Wattal, Schuff, Mandviwalla and Williams2010). To enrich the field of analysis and provide contemporary evidence, research on the effectiveness of negative campaigning should similarly expand into these new settings.
Research into the effectiveness of negative campaigns would also benefit from more closely examining voter gender as a determinant. Certainly, a number of potential gender differentials have been investigated in relation to campaign negativity, but many of these focus on the gender of political candidates—not the gender of voters themselves—as independent variables. Research on gender at the candidate level, for example, has assessed female candidate proclivity for negative statements (e.g., Kahn Reference Kahn1993; Trent and Sabourin Reference Trent and Sabourin1993), the relationship between gender stereotypes and candidate negativity (e.g., Kahn Reference Kahn1996; Krupnikov and Bauer Reference Krupnikov and Bauer2014), the types of issues raised in female candidate attacks (e.g., Dolan Reference Dolan2014), female candidate views of negativity (e.g., Herrnson and Lucas Reference Herrnson and Lucas2006), and the (lowered) likelihood of male candidates attacking female opponents (e.g., Fox Reference Fox1997). In contrast to the substantial focus that gender receives at the candidate level, voter gender rarely features prominently in investigations on the effects of negativity (see Brooks Reference Brooks2010). Overlooking such a variable is not an issue if men and women respond similarly to negativity. If, however, men and women respond differently to negativity, then the effect of negativity (e.g., depressed voter turnout) depends on voter gender—a conditional relationship. However, only a handful of studies have appropriately controlled for a conditional relationship of this nature.
There are reasons to expect that men and women do indeed differ in their receptiveness to negative campaigning (i.e., a gender gap). Related academic fields, for example, document women's comparative aversion to direct forms of aggression (Archer Reference Archer2004), such as verbal conflict (Björkqvist Reference Björkqvist1994; Kikas Reference Kikas, Peets, Tropp and Hinn2009), and their lower interest in consuming media depictions of it (Weaver Reference Weaver2011). Consistent with these findings, a gender gap has been observed in the limited research investigating the responses of men and women to negative campaigns to date (e.g., Brooks Reference Brooks2010; Fridkin and Kenney Reference Fridkin and Kenney2019; Kern and Just Reference Kern, Just and Norris1997; King and McConnell Reference King and McConnell2003). However, much of this literature offers divergent results and competing theoretical explanations for the composition of the gender gap.
Beyond this concern, none of these studies has assessed the effect of gender in the context of new campaign platforms such as social media. This limitation is important given the increasing utilization of social media by political campaigns and the emerging evidence demonstrating that the social media environment may actually exacerbate differences in the way men and women engage with political content (Bode Reference Bode2017). The existing theoretical divide, absence of investigation of the phenomenon in new mediums, and the potential for a magnification of the effect on said platforms provide strong justification for examining men's and women's differing receptiveness to candidate negativity on social media.
This article contributes to research in these areas by exploring whether a gender gap exists on the world's most prominent social media platform: Facebook. The Facebook posts of 10 presidential candidates covering two general elections (2012 and 2016) and two primaries (2016 Democratic and Republican) were tracked in this study. User reactions to these posts were also collected; reactions were defined as a Facebook feature permitting users to publicly ‘like’ or otherwise react (e.g., via an emoticon representing ‘love,’ ‘laughter,’ ‘amazement,’ etc.) to a Facebook post. The resulting dataset contains the reactions of nearly 40 million unique users and 20,000 posts from the candidates.
Across nearly 400 million individual reactions, women were comparatively less receptive to candidate negativity than men, showing a clear gender gap. This gap existed for all 10 candidates across all election cycles, regardless of party, election type, or incumbency status. Two potential explanatory factors are investigated here: varied receptiveness to attacks on a female candidate and differing gender preferences for language. However, neither fully explains the gender gap exhibited or resolves competing explanations for the differences in previous research findings. Conceptualizing the gap in a new manner, via a theory of differential receptivity, accomplishes this task and reveals a more complex relationship between candidate negativity and gender response than previously thought.
LITERATURE REVIEW
The theoretical foundations for this study draw from an arena of multidisciplinary past research. In this section, I first outline the debate surrounding the effectiveness of negative political communication, noting the limited attention to voter gender in these studies and the subsequent potential model specification issues present in the literature as a result. Next, I identify the few studies to date that have investigated men's and women's differing receptiveness to negative political communication, along with the conflicting findings produced from such research. I then offer the theoretical foundations for expecting gender differentials, complemented with more recent research on social media behavior that indicates how the online context may widen the gender gap.
The effectiveness of negative political communication has been the subject of continuing disagreement, primarily explored in studies of televised attack advertisements. One side, the dominant position in the debate, finds that negative campaigning does not benefit the intended candidate, and where an effect is observed, that it typically produces backlash from voters (Lau and Rovner Reference Lau and Rovner2009; Lau, Sigelman, and Rovner Reference Lau, Sigelman and Rovner2007; Malloy and Pearson-Merkowitz Reference Malloy and Pearson-Merkowitz2016). In the opposing school of thought, attack ads can work as a successful campaign strategy, but only under certain circumstances. To name just a few, these conditions traditionally include the quality of an attack ad (see Mike Murphy's response in Craig and Hill Reference Craig and Hill2011, 136–137), the tone of an attack (Brooks Reference Brooks2010; Brooks and Geer Reference Brooks and Geer2007; Fridkin and Kenney Reference Fridkin and Kenney2011), whether an attack is policy based or personal (Fridkin and Kenney Reference Fridkin and Kenney2004), and whether it is contrasting or purely negative (Jamieson Reference Jamieson2000). More recent research has expanded these conditions to include the source of the attack (i.e., outside group vs. candidate endorsed) (see, e.g., Brooks and Murov Reference Brooks and Murov2012; Dowling and Wichowsky Reference Dowling and Wichowsky2015; Weber, Dunaway, and Johnson Reference Weber, Dunaway and Johnson2012); the timing of attacks within the election cycle (Krupnikov Reference Krupnikov2014); the relative status of incumbents and challengers in political races (Blackwell Reference Blackwell2013); and the perceived credibility of the attacker (Lipsitz and Geer Reference Lipsitz and Geer2017; Mattes and Redlawsk Reference Mattes and Redlawsk2014).
Some studies on the effectiveness of negative political communication have focused more specifically on certain subgroups of voters. For example, many of these studies on voter subgroups have assessed the potential mobilizing or demobilizing influence of negativity on partisans and independents (e.g., Ansolabehere and Gerber Reference Ansolabehere and Gerber1994; Ansolabehere and Iyengar Reference Ansolabehere and Iyengar1995; Ansolabehere, Iyengar, and Simon Reference Ansolabehere, Iyengar and Simon1999; Finkel and Geer Reference Finkel and Geer1998; Goldstein and Freedman Reference Goldstein and Freedman2002; Wattenberg and Brians Reference Wattenberg and Brians1999). Differentiating between subgroups of voters is a critical task, given that the effects of negativity may differ substantially across a number of voter subgroups. Yet voter gender receives considerably less attention in research on negative campaigns. Failing to account for these differences in other voter subgroups, such as men and women, may therefore produce underspecified models from which erroneous conclusions are drawn (see Brooks Reference Brooks2006, 694).
If the effects of negativity are conditional on gender, then properly specified models accounting for this conditional effect must either employ an interaction between voter gender and negativity or must estimate the same model via separate subsamples (i.e., run separate regressions for samples of women and then men; see Kam and Franzese Reference Kam and Franzese2007). To be clear, it is not enough to merely include a control variable for voter gender in models without taking either of these approaches. In such a linear-additive model, any change in an independent variable (e.g., increase in negativity) exerts an unconditional influence (i.e., holding all else including gender constant) on the dependent variable (e.g., voter turnout). The consequences of omitting this type of conditional relationship, should it exist, are discussed more extensively elsewhere (see, e.g., Franzese Reference Franzese2003), but the prevailing advice is to account for such a relationship where possible or risk model specification error (Friedrich Reference Friedrich1982). As Brambor, Clark, and Golder (Reference Brambor, Clark and Golder2006) show, there is no other way to appropriately model such a conditional hypothesis, including within nonlinear methods.
To demonstrate the scale of this potential specification issue more clearly, I surveyed the existing body of published research on the effects of negative campaigning on voters for appropriate model specification of the conditional relationship described in the previous section (see Table 5 in the Supplementary Material online). For context, 80 published studies were examined in Lau, Sigelman, and Rovner's well-known Reference Lau, Sigelman and Rovner2007 meta-analytic reassessment of the field, showing that the discipline's published work has nearly doubled over the past decade. Of the 148 publications examined in the current survey, fewer than half (n = 63) specify empirical models that include a voter gender variable; thus, the variable is not yet considered a necessity in this field. Many of these studies control for voter gender as a mere demographic feature, not one with a conditional relationship on the effects of negativity. In total, only 19 of the published studies on the effects of negative campaigns specify an interaction between voter gender and negativity (or take the separate subsample approach), and only six present a voter-gender conditional hypothesis in text (i.e., test whether a gender gap exists). Although it is not new, specific research into men's and women's differing receptiveness to negativity is quite rare.
Further complicating matters, little consensus can be gleaned from this small collection of (potentially) appropriately specified work. Some, for example, offer evidence that casts doubt on whether men and women differ at all in their receptiveness to negativity (e.g., Garramone Reference Garramone1984; Goldstein and Freedman Reference Goldstein and Freedman2002), including when such voters share the gender of the attacker or not (e.g., Craig and Rippere Reference Craig and Rippere2016). Others demonstrate that women are repulsed by negativity (e.g., Kern and Just Reference Kern, Just and Norris1997), a finding which also tends to coincide with evidence that men are indifferent to attacks. For example, King and McConnell (Reference King and McConnell2003) find that the likelihood of women voting for the attacker is reduced when women are overexposed to candidate endorsed attacks, but men are unaffected. Similarly, Kahn and Kenney (Reference Kahn and Kenney2004) show that women, but not men, are demobilized by negativity. Conversely, Brooks (Reference Brooks2010) argues precisely the opposite: according to her research, men are mobilized by negativity, which has an insignificant influence on women.
This limited body of evidence on voter gender and negativity indicates that a gender gap exists, albeit without a definitive agreement on the direction or nature of the gap itself. Regardless, observing such a gender gap would fall well in line with theories developed in a number of other academic fields. Differences in behavioral responses to emotional stimuli, particularly anger, represent one such area. Although a comprehensive body of psychology literature shows no self-reported difference in the rate at which men and women experience anger generally, the genders differ significantly in aggressive behavioral responses to the emotion (Archer Reference Archer2004). Men are more likely to engage in physical and verbal aggression (direct) when angered, whereas women display a preference for indirect forms of aggressive behavior (e.g., social exclusion; see Archer and Coyne Reference Archer and Coyne2005; Crick and Grotpeter Reference Crick and Grotpeter1995).
The trigger—anger—is particularly relevant to the current study, given that other negative emotional cues in attack ads, like sadness and fear, often provoke anger in subjects (Weber Reference Weber2012) as emotional reactions of similar tone (i.e., negative) often co-occur (Diener and Iran-Nejad Reference Diener and Iran-Nejad1986). Meaning, attack ads without the specific anger cue are still likely to trigger anger concurrently with other negative emotions. Anger cues in attack ads are also unique in their ability to alter behavior in subjects ranging from volunteering to voting (Weber Reference Weber2012); thus, anger provoked in response to negative campaigns may trigger divergent aggressive behavioral responses in men and women.
Further differences exist between men's and women's preference for direct aggression represented in media. For example, men exhibit greater preference for violent media programs (Atkin et al. Reference Atkin, Greenberg, Korzenny and McDermott1979; Weaver Reference Weaver2011), and women show greater levels of empathy and aversion when viewing violence (Schienle et al. Reference Schienle, SchÌfer, Stark, Walter and Vaitl2005). Women's aversion to moderately negative stimuli manifests in other critically related areas, including a lowered preference for consuming negative news (Kamhawi and Grabe Reference Kamhawi and Grabe2008). On this basis, women should be comparatively less tolerant of negative political communication, and nearly two decades of research on political incivility shows just this (e.g., Fridkin and Kenney Reference Fridkin and Kenney2011; Fridkin and Kenney Reference Fridkin and Kenney2019; Kahn and Kenney Reference Kahn and Kenney2004; Stryker, Danielson, and Conway Reference Stryker, Taylor Danielson and Conway2015).
We should therefore expect men and women to respond quite differently to negative campaigns. However, this comparative aversion to mediated forms of direct aggression needs further consideration given the interactive social media context, where supporters can actively seek to consume—or avoid—negative political communication to a greater degree than in traditional media, such as television, where the only option for the viewer is to watch the message or change the channel. New evidence, for example, suggests that men consume more political information on social media than women (Gray, Gainous, and Wagner Reference Gray, Gainous and Wagner2017), magnifying gender differences in an environment with greater user choice. By design, Facebook also identifies and offers content most likely to prolong engagement with the platform based on the user's previous behavior (Lipsman et al. Reference Lipsman, Mudd, Rich and Bruich2012). Users are therefore given more choice, and their choices may incentivize the platform to give them more of their preferred content. Such an environment differs substantially from studies of televised attack ads. If men are more receptive to negativity, social media platforms are likely to give them more of it, magnifying the gender gap. The feed also differs in another critical manner from television: it publicizes a user's support for negativity among their following.
The bulk of social media users are deeply cautious about publicizing their activities online, often self-regulating behavior to be consistent with their online persona and the expectations of their following (Mehdizadeh Reference Mehdizadeh2010; van Dijck Reference Dijck2013). In a political context, this manifests when individuals support a political candidate offline but fear publicizing their support on social media (Marder et al. Reference Marder, Slade, Houghton and Archer-Brown2016). Critically, gender behavioral expectations appear to figure prominently in the self-regulatory calculus of these users. For example, women on social media, in contrast to men, seek to avoid offending their social network by limiting their visible political engagement (Bode Reference Bode2017). The directly aggressive nature of negative political communication may further magnify this self-regulatory behavior in a public forum, like social media, given that women show reduced aggressive tendencies in experiments when their gender is publicized (Lightdale and Prentice Reference Lightdale and Prentice1994).
Gender differences also extend to language preferences (Mulac Reference Mulac, Canary and Dindia2006; Newman et al. Reference Newman, Groom, Handelman and Pennebaker2008). From a conflict standpoint, as Brooks notes (Reference Brooks2010), female-preferred language is slightly, but measurably, more amicable, warmer, and less aggressive than male-preferred language (Holmes Reference Holmes1995), including in discourse on social media (Park et al. Reference Park, Yaden, Andrew Schwartz, Kern, Eichstaedt and Kosinski2016). Such differences are significant enough in large datasets to extrapolate the gender of subjects purely from their language deployment alone (Schwartz et al. Reference Schwartz, Eichstaedt, Kern, Dziurzynski, Ramones and Agrawal2013). Women's response to negative campaigns might therefore depend to some degree on the severity of the language used. In testing just such a condition, Brooks (Reference Brooks2010) found that uncivil (more aggressive) language magnifies the gender gap, thereby showing language to be a potential determinant worth consideration in any study of men's and women's response to negative campaigns.
Finally, the increasing importance of social media for candidates and voters alike is notable. In the current environment, campaigns of all sizes seek to persuade and mobilize voters via new media (Miller Reference Miller2013). Among many potential outlets, Facebook has become the social media focal point for elections, and rightfully so. Seven of 10 Americans possessed a Facebook account by the end of the 2016 presidential election (Pew Research Center 2017). By some estimates, nearly half of US adults acquire news from Facebook (Matsa Reference Matsa and Shearer2018), and roughly one-tenth of voters in the most recent presidential campaign used it as their primary source for election news (Gottfried, Barthel, and Mitchell Reference Gottfried, Barthel and Mitchell2018). This mass adoption of social media has revolutionized the way candidates and voters communicate about politics, transforming the political environment now and well into the foreseeable future (Gainous, Marlowe, and Wagner Reference Gainous, Marlowe and Wagner2013).
Negative political communication therefore has the potential to reach millions of voters on Facebook during presidential campaigns. Although a large body of literature debates the effectiveness of candidate endorsed attacks on television, work is still to be done in the social media environment, which offers researchers opportunities to contribute by investigating the effects of negativity in new and unique ways. Understanding gender differentials is one such area that needs attention. As the discussion in the previous section outlines, there are a number of reasons to expect such a difference in receptiveness to campaign negativity to exist, and the limited evidence from research into this gap tends to agree that men and women respond differently to attacks, at least in a televised environment. These studies, however, disagree on the nature of the gap itself. Further investigation into the gender gap in response to negative political communication on social media is worthy of exploration for three reasons: First, the environment of social media may magnify the gap, due to platform algorithms encouraging users to engage with negativity in line with exhibited personal preference, gender behavioral expectations, and political self-censorship on these platforms. Second, the large-scale adoption of social media by Americans permits the expansion of observations into the millions, thereby offering the prospect of more robust empirical findings from a larger dataset and contributing to the sparse amount of knowledge in this area. Finally, a study of this size may be able to offer greater clarity on the divergent theoretical explanations for the gender gap that currently exists.
DATA COLLECTION AND METHODOLOGY
In the present study, I investigated the active participation of Facebook users during two presidential general elections in 2012 and 2016 and two presidential primaries in 2016. Data were collected using Facebook's publicly available Graph API: Facebook's required data collection method. For the general elections, data were gathered from the Monday following the final party nomination convention to the evening before the election. The collection period for the 2016 presidential primary elections spanned the first official day of Hillary Clinton's presidential campaign, on April 12, 2015, to Clinton's acceptance speech on the last day of the Democratic National Convention, on July 28, 2016. During this time, all posts, including status updates, links, images, videos, offers, and events, were collected from selected candidate pages. Only Facebook Live posts were removed from this dataset due to their late implementation (August 2016) and the inability to code them accurately.
Ten candidates were selected for data collection. In the 2012 and 2016 general elections, the Democratic and Republican nominees were included: Barack Obama, Mitt Romney, Hillary Clinton, and Donald Trump. The candidates compared in the 2016 primaries were the Republicans Ted Cruz, John Kasich, Marco Rubio, and Donald Trump, and the Democratic contestants Hillary Clinton and Bernie Sanders, as each had won at least one state during the primary contests, thereby indicating some measure of success as candidates. For candidate posts, all names and Facebook numeric identifiers were collected for ‘likes’ and ‘reactions,’ which are both defined as reactions in this study. The collected dataset contains nearly 400 million reactions, 40 million unique users, and 20 thousand posts from the candidates.
To identify gender, the entire dataset of users needed to be categorized as male or female. First names (Liu and Ruths Reference Liu and Ruths2013) and surnames (Fiscella and Fremont Reference Fiscella and Fremont2006) have been used by academics, corporations, and governing institutions to accurately identify the gender and ethnicity of individuals in large datasets. To gender-code observations, data from the Social Security Administration (SSA) were enlisted. The SSA database used (2017) contains a list of approximately 80,000 unique first names and the number of births and gender of individuals assigned each name in the period 1945–2017. Users in this study were categorized as either male or female according their first name, based on whichever gender had the largest total number of births for that name in the SSA database. Unidentified names were dropped from the statistical analysis. Nearly 38 million individuals, approximately 90% of all users, were categorized by gender in this way.
Overall, 20,000 political posts were coded as positive or negative utilizing two common approaches, described as evaluative and directional (Walter and Vliegenthart Reference Walter and Vliegenthart2010). The evaluative method requires a detailed assessment of the legitimacy and fairness of the critique lodged, whereas the directional method merely labels any critique of an opponent as negative. Although the directional approach is far easier to implement given the size of the dataset, it is too simplistic for use in assessing social media posts given their high degree of rhetorical nuance. This can be seen, for example, in the use of ironic or sarcastic devices. It is also possible that such posts are produced with the intention of allowing message decoding by a narrower target audience, one with the ability to “fill in the gaps” intentionally left out of subtle communications.
In this study, I adopted a combined approach to coding the posts using the following criteria. First, candidate Facebook posts that identified opposing candidates (directional) were isolated, following Geer's (Reference Geer2006) directional definition of negativity as “any criticism leveled by one candidate against another during a campaign.” Whether the opponent was criticized within the first 150 words of a post was then checked, within any associated image, or within the first 5 seconds of a video. This limit was imposed because Facebook often only displays the first portion of text for posts with long messages, so attacks at the end may not be visible to users. On videos, Facebook's (2015) own data suggest that the first 3 seconds of videos are the most memorable to users when scrolling through their newsfeed. The imposed limit therefore reflects a reasonable time period for stimulating user response. In the Facebook Live video section, a user's view cannot be identified; thus, these videos were impossible to code and were discarded from the dataset. Finally, if a candidate was not explicitly criticized, the evaluative method was applied to determine whether the post could be interpreted by supporters as implicitly criticizing an opponent, due to the presence of verbal and visual cues such as sarcasm, satire, irony, or a backhanded compliment within the same post restrictions. Included in this consideration were links to external articles that critiqued opponents, unflattering images of opponents, and attacks on an opponent's spouse. Krippendorff interrater reliability tests were conducted on approximately 300 observations (an adequate sample size; see Lacy and Riffe Reference Lacy and Riffe1997) between the two researchers coding the data, producing acceptable results (∝ =0.902).
The resulting coded dataset represents an ecosystem of hundreds of millions of reactions from men and women to candidate endorsed attacks. The rate at which candidates attacked varied both between the candidates themselves and across election types (i.e., the primaries vs. general elections). For example, 28% of general election candidate posts were negative; conversely, primary candidates attacked at a rate of approximately 13%. In this way supporters of some candidates were exposed to more negativity than others, leaving them fewer opportunities to prefer positive messages. As a result, investigating men's and women's reactions to candidate negativity must account for this difference in statistical tests either by interaction or testing on separate candidate subsamples. More detail on this variation between the candidates can be found in the Supplementary Material section online, where tabulation data and time series figures are provided.
The data were assessed both at the candidate (macro) level according to reactions to the candidate's posts and at the user (micro) level according to individual user preference for negativity. At the macro level, tests were conducted to determine whether a candidate received more reactions for negative statements than positive ones. Notably, separating the tests by candidate in this manner satisfies the separate subsamples requirement outlined previously. Statistically assessing noisy, nonnormal data of this nature typically requires population tests that are free of distributional assumptions. The single-tailed, directional, Wilcoxon-Mann-Whitney method used in this study is a nonparametric test that can be used to determine whether samples are drawn from populations with the same distribution. The null hypothesis for this test stipulates that there is no difference in location between the distribution of reactions for negative posts (R n) and the distribution of reactions for positive posts (R p). The alternative hypotheses stipulate that the distribution of the reactions for negative posts are greater than (i.e., shifted to the right of) the distribution of reactions of positive posts (H A1 : R n > R p) with test statistic Z ≥ 1.64, or less than (H A2 : R n < R p) with test statistic Z ≤ −1.64. The population test results therefore illustrate whether the candidate benefits (Z ≥ +1.64), suffers (Z ≤ −1.64), or receives no significant difference (−1.64 < Z< + 1.64) in reactions from users when the candidate makes attacks in comparison to the reactions the candidate receives when he or she remains positive.
The advantage of using Z-scores in this manner is that they are standardized, which permits comparisons across tests on observations with different scales. This is particularly useful given men may be more likely to participate online (i.e., to react to more posts) than women, so the standardized results provide the basis for testing for the differential receptiveness between men's and women's reactions to negativity. This difference between men's (Z m) and women's (Z w) reactions at this macro level can be calculated via a single Z-score differential ($Z_\delta = (Z_m-Z_w)/\sqrt 2 $; see Rosenthal and Rubin Reference Rosenthal and Rubin1979). Results of this differential Z-score (Z δ) above or below the ±1.64 threshold reflect a statistically significant difference (i.e., gender gap) in how men and women respond to a specific candidate's attacks. To assess collective gender differences across all 10 candidates, the Stouffer method was used, which is also sometimes referred to as inverse normal (
$Z_{s\delta} = \mathop \sum \limits_{i = 1}^n Z_{\delta i}/\; \sqrt n $). The Stouffer method is used to ascertain whether the sample of differential Z-scores (Z δ) collectively diverges from a normal distribution. If the divergence is statistically significant, the alternative hypotheses outlined above continue to be supported. A collective result (Z sδ) above or below the ±1.64 threshold reveals a significant gender gap across all 10 candidates.
Differing language preference was explored as a potential explanatory factor for gender imbalance. Because the setting in which language is used is relevant for textual analyses (Hamilton et al. Reference Hamilton, Clark, Leskovec and Jurafsky2016), posts were coded using the Facebook user language preference dataset from the University of Pennsylvania's World Well Being Project (see Sap et al. Reference Sap, Park, Eichstaedt, Kern, Stillwell and Kosinski2014; WWBP 2014). The WWBP project collected 300 million words posted by 75,000 men and women on Facebook, and a value (x) was assigned along a spectrum based on that gender's comparative usage of the word (Park et al. Reference Park, Yaden, Andrew Schwartz, Kern, Eichstaedt and Kosinski2016; Sap et al. Reference Sap, Park, Eichstaedt, Kern, Stillwell and Kosinski2014; Schwartz et al. Reference Schwartz, Eichstaedt, Kern, Dziurzynski, Ramones and Agrawal2013). Posts in the present study were coded according to its location along the WWBP defined gender language spectrum, based on the sum of words used indicating preference by women $\left( {\mathop \sum \limits_{i = 1}^n x_i \ge 0} \right)$ or men
$\left(\mathop \sum \limits_{i = 1}^n x_i < 0\right)$.
The population tests outlined above were reapplied to posts coded for language as preferred by women or men. Differences between the reactions from men and women to male-preferred language posts $(Z_{\delta m} = (Z_m-Z_w)/\sqrt 2 $) and female-preferred language posts (Z δf) for each candidate were assessed for collective statistical significance using the Stouffer method (Z sδm; Z sδf). To assess the explanatory power of language preference, the difference test was applied to the collective Stouffer Z-scores for male and female preferred language (
$Z_{s\delta m}-Z_{s\delta f}/\sqrt 2 )$, which, if positive and significant, reflect that differing language preferences drive the gender gap.
Attacks on female candidates were also considered for their potential explanatory power, given the debate over how voters react to candidate negativity when female candidates are attacked (and go on the attack). A final macro-level population test was applied to discern whether attacks on the lone female candidate in these races (Hillary Clinton) magnify or diminish the gender gap in comparison to attacks on male candidates. Results above or below ±1.64 reveal statistically significant differences between men and women when a single candidate attacks Clinton (Z δ), and the collective significance of such attacks across all of Clinton's opponents via the Stouffer test (Z sδ).
At the micro level, tests of individual user behavior are required for diagnostic purposes in two ways. First, a small cluster of individuals might disproportionately react to negativity, potentially distorting the macro-level results. For example, the 2012 general election candidates collectively had more than 11 million unique supporters, yet only a few thousand reactions represent the difference between average reactions for positive and negative posts from men and women. Micro-level tests of the sample size used here—nearly 40 million observations—are sensitive enough to discern whether a few thousand men or women dedicated to supporting negativity from their preferred candidate are disproportionately influencing the macro-level results. Second, as outlined previously, differing candidate proclivity for negativity is another critical factor worth further exploration. Contestants that frequently post negative messages leave fewer opportunities for supporters to react to positive posts than candidates who attack less often. The macro-level population tests were thus constructed to account for this conditional relationship via separate subsamples. The micro-level assessments of individual user preference for negativity accounted for this relationship via interaction. In doing so, they may further illustrate that the gender gap grows (or shrinks) as a result of increased negativity from the candidates. These micro tests, therefore, provide a fuller account of the gender gap, while also operating as an important diagnostic.
Logistic regression was used to test for gender differences at this micro (individual) level. Individuals in the models were identified by their gender (1 for female, 0 for male); the rate of attack from their supported candidate (percentage of contestant's negative posts multiplied by 10); the election type (1 for general election, 0 for primary); and candidate incumbency. The dependent variable represents a user's preference for negativity (1 for a reaction to a negative post, 0 for a reaction to a positive post). If users randomly liked posts from a candidate, then men's and women's preference for negativity would not diverge significantly (leading to an insignificant coefficient), regardless of candidate proclivity for going negative. In contrast, a significant gender coefficient indicates that a gender gap exists at the individual level, and a negative gender coefficient shows that women are less receptive to negativity than men. For the reasons discussed in the previous section, the interaction between gender and candidate attack rate was also analyzed, and where negative, it shows that women became increasingly repulsed by negativity as candidates increased their rate of attacks.
Given the theoretical positions described in the previous section and the methodology used to test them, four results are anticipated in the following hypotheses:
H1:
Provided the many reasons to suspect that men will be more receptive to negativity than women, the macro tests will produce statistically significant positive Stouffer Z-scores (Z sδ).
H2:
Attacks using female-preferred language will reduce the gap (Zsδf) and male-preferred language will magnify it (Zsδm).
H3:
The gender of the target is a driver of the gender gap, and attacks on a lone female candidate magnify the gender gap.
H4:
Micro-level tests will produce negative gender coefficients, offering further evidence of a gender gap and ruling out other potential explanations not discernible from the macro-level tests.
RESULTS
Figure 1 provides a visual example comparing the number of reactions Ted Cruz received for each of his negative and positive posts during the 2016 primary campaign. As the figure visually demonstrates, both men and women were more receptive to Cruz's negative posts (R n) than his positive posts (R p). The Wilcoxon-Mann-Whitney Z scores confirm the statistical significance of this receptiveness to negativity over positivity: Population tests for both men (Z m = 8.84) and women (Z w = 5.75) produced results above the 5% significance threshold (Z ≥ 1.64). More importantly, as the visual representation depicts, men prefer negativity to positivity to a greater degree than women do in this example. A Z-score differential test confirms this gender gap $\left( {Z_\delta = ( {8.84-5.75} ) /\sqrt 2 = 2.19} \right)$. Similar visual examples for the remaining candidates are provided in the Supplementary Material online (Figures 13–21).
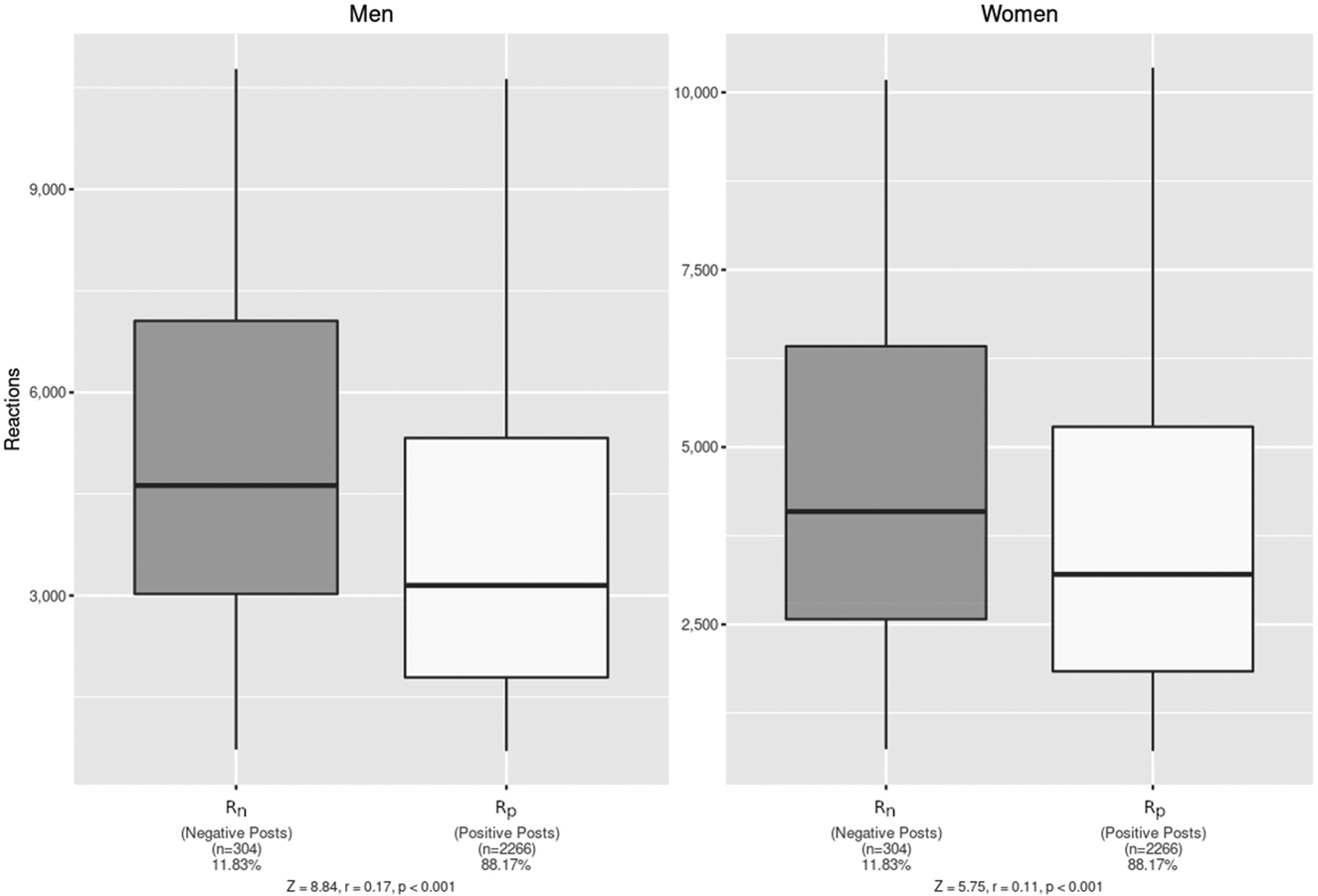
Figure 1. Box plot example of Ted Cruz's negative post reactions and positive post reactions.
Table 1 shows the Wilcoxon-Mann-Whitney Z scores for these macro-level population tests for all 10 candidates. Candidates in this table are considered 2016 primary contestants unless they are explicitly identified as general election candidates. Following the visual example provided in the previous section, Table 1 also displays men's (Z m) and women's (Z w) receptiveness to negativity over positivity, along with the difference between men's and women's preferences for their candidate's negativity reflected in the differential Z-scores (Z δ). The table is split into three separate categories of attacks: all negativity, attacks on Democratic candidates only, and attacks on Republicans only. For example, men (Z m = 0.10) and women (Z w = −0.66) that reacted to President Obama's posts in the 2012 general election exhibited no statistically significant preference for all the candidate's negative posts over his or her positive posts. Although the difference (Z δ = 0.54) between men's and women's preference for negativity (i.e., the gender gap) for President Obama was positively signed, as expected, the result falls below the 5% significance threshold.
Table 1. Wilcoxon-Mann-Whitney Z-scores comparing negative reactions to positive reactions
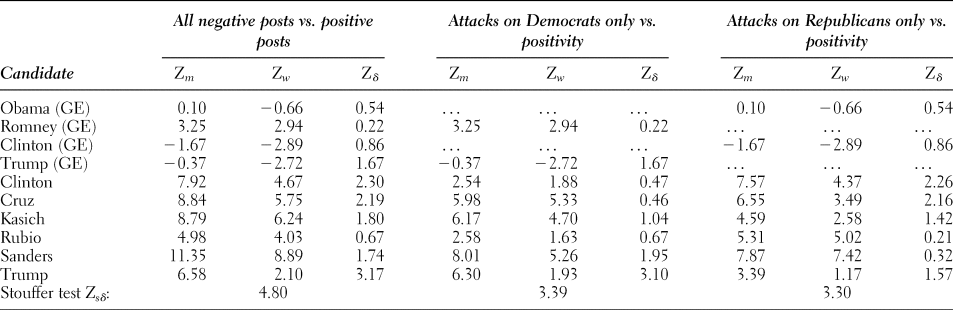
Note. GE, general election. Candidates are considered primary contestants unless otherwise specified via GE. H A1: R n > R p ≡ Z ≥ +1.64; H A2 : R n < R p ≡ Z ≤ −1.64.
Continuing with this assessment of the gender gap for all negativity versus positivity, six of the 10 candidates showed a statistically significant difference between men's and women's receptivity for attacks (Z δ ≥ 1.64; i.e., Trump GE, 1.67; Clinton, 2.30; Cruz, 2.19; Kasich, 1.80; Sanders, 1.74; Trump, 3.17). Like the Obama gender gap, the remaining four statistically insignificant results (Obama GE, 0.54; Romney GE, 0.22; Clinton GE, 0.86; Rubio, 0.67) also yielded a positively signed Z-score differential gender-gap test (0 < Z δ < 1.64). All 10 candidates showed a gender gap in the expected direction, and the Stouffer test confirms the collective statistical significance of these gender-gap results (Z sδ = 4.80). These results confirm H 1.
To discern whether the direction of an attack played any role in this result, attacks were further classified by the target's party, as previously noted (i.e., an attack on Democratic candidate or Republican candidate only). The gender gap (Z δ > 0) persists regardless of the direction of the attack (i.e., both within and between parties) for all 26 differential tests with varying levels of significance for each candidate. The Stouffer tests confirm the collective statistical significance of this gender gap for attacks targeted just at Democratic (Z sδ = 3.39) or Republican candidates (Z sδ = 3.30). Therefore, the results uniformly show that men preferred negativity more than women. This evidence indicates a gender gap, regardless of election type (i.e., general election vs. primary) or direction of the negativity (Democratic target vs. Republican), even when candidates attacked members of their own political party, further confirming H 1.
Table 2 displays the results of my investigation into whether differing language preferences operate as a potential explanatory factor for the gap exhibited in this dataset, which previous literature suggests may be the case. If language preferences magnify the gap, the difference of the Stouffer results for male- and female-preferred language $\left( {Z_{s\delta m}-Z_{s\delta f}/\sqrt 2} \right)$ would yield a significant positive result. However, the results do not reflect a significant difference (Table 2;
$0.45 = Z_{s\delta m}-Z_{s\delta f}/\sqrt 2 )$ between how men and women respond to negativity dependent on language preference. This finding rules out language preference as the major explanatory factor for the gender gap in this dataset, thus refuting H 2.
Table 2. Wilcoxon-Mann-Whitney Z scores comparing negative reactions categorized by language preference to positive reactions
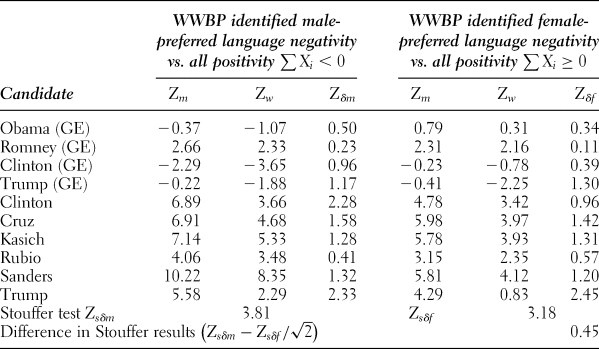
Note. GE, general election; WWBP, World Well Being Project. Candidates are considered are primary contestants unless otherwise specified via GE. H A1 : R n > R p ≡ Z ≥ +1.64; H A2 : R n <R p ≡ Z ≤ −1.64.
Table 3 displays the results of my investigation into whether negativity targeted at female candidates explains the gender gap observed in the present study. The tests compare whether reactions to attacks on Clinton by primary candidates differed from those lodged against other (male) candidates during the primaries. None of these results were statistically significant. Four of Clinton's five primary opponents had insignificant positive gender differentials (Z δ) when attacks were lodged against her versus other targets. In contrast, Cruz's negative differential (−0.69) suggests that his female supporters rewarded him for attacking Clinton, but not significantly. The Stouffer test similarly showed no significant difference between attacks targeted at Clinton versus male targets (Z sδ = 0.92), ruling out the gender of the target as a potential driver for the gender gap observed in the present study. These results thus negate H 3.
Table 3. Reactions to attacks on Clinton (Rc) in the primary campaign versus attacks on male candidates (Ro)

Note. H A1 : R c > R o ≡ Z ≥ +1.64; H A2 : R c < R o ≡ Z ≤ −1.64.
Table 4 displays the results for the logistic regression tests which investigate men's and women's receptiveness to candidate-endorsed attacks at the individual user (micro) level. As expected given the macro-level results, both models show that women were less likely to prefer negative posts than men (negatively signed), ruling out the disproportionality concern noted in the previous section and validating H 4. The interactive term in the second model also shows that the gender gap increased as candidates became more negative (i.e., attacked more). Marginal effects plots (Figure 11, Supplementary Material online) uphold this conclusion, as do analysis of deviance tests (Table 7, Supplementary Material online) in which both logistic regression models deviate significantly from the null, further underscoring the conditional relationship between gender and negativity, and the existence of a gender gap. Put simply, like the macro-level tests, these results uniformly confirm the gender gap across nearly 38 million user observations with a high degree of statistical significance.
Table 4. Individual preference for negativity over positivity logistic regression
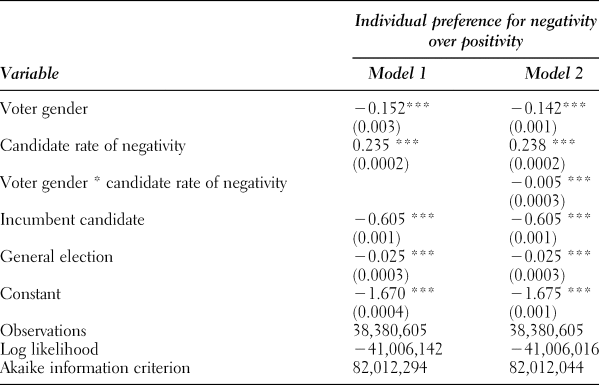
Note: * p < 0.1; ** p < 0.05; *** p < 0.01.
Although both levels of testing point to the presence of a gender gap, the results also reveal some important discrepancies in supporter receptivity to attacks that require greater exploration before proceeding. As the macro- and micro-level tests show, primary supporters rewarded negativity over positivity, yet supporters in the general elections demonstrated a far greater range of responses to candidate attacks (see Table 1). The two candidates assessed in both their primary and general election contests best demonstrate this difference in receptivity. For example, men and women significantly punished Hillary Clinton for being negative in the general election, yet her attacks in the primaries were not similarly punished; they were, in fact, rewarded by her supporters. In addition, women (but not men) were repulsed by Donald Trump's attacks on Clinton during the general election (see Table 1), but like Clinton, his negativity in the primaries was rewarded regardless of the supporter's gender. Although this might merely reflect a difference in the type of supporters primaries and general elections attract (e.g., partisans vs. independents), it nevertheless underscores that a range of responses to negativity from men and women emerge from this dataset (e.g., sometimes rewarding or punishing it). With the gender gap persisting across all the tests, these discrepancies, among others noted in the following section, demand the development of a gender-gap theory which can provide a more accurate explanation for this broad range of men's and women's receptivity to candidate attacks.
DISCUSSION: A THEORY OF DIFFERENTIAL RECEPTIVITY
As outlined previously, the limited existing research on the gender gap offers contradictory evidence on the difference between men's and women's receptiveness to negativity. On one end of the spectrum, women are influenced by negativity, but men are unaffected (Kahn and Kenney Reference Kahn and Kenney2004; King and McConnell Reference King and McConnell2003). On the other, Brooks (Reference Brooks2010) finds that women are not affected by attacks, whereas men are energized by candidate negativity. Previous research also shows little if any difference between men's and women's receptiveness to negative campaigns (e.g., Craig and Rippere Reference Craig and Rippere2016; Garramone Reference Garramone1984; Goldstein and Freedman Reference Goldstein and Freedman2002). The results from the present study provide additional evidence for the presence of a gender gap and offer a foundation for developing an explanatory theory that better incorporates past and present findings: a theory of differential receptivity.
Both men and women are responsive to candidate attacks, and the direction of their responses are, very broadly, the same. More specifically, men and women exhibited similar directional responses to candidate negativity in this study (together rewarding it, statistically indifferent to it, or punishing it), but they differed in their degree of receptiveness to such attacks in this same direction (the magnitude of the response), thereby suggesting differential receptivity to negativity. Across all three categories of macro-level tests (26 total tests), only 2 produced different directional signs for men and women. Therefore, men and women almost uniformly responded to attacks in the same direction (again, rewarding it, indifferent to it, or punishing negativity).
In the rewarding direction, men and women both significantly preferred all negativity over positivity from Romney in 2012 and from almost all the 2016 primary candidates. They offered these same candidates greater public support when they made negative attacks than when they were positive. Men and women, however, also showed indifference in their preference for attacks over positivity from some candidates (Obama in 2012). Finally, men and women were repulsed by attacks from Clinton in the 2016 general election, significantly preferring her positive messages instead. This shared direction between men's and women's response to negativity reveals a more complex response from both genders to candidate attacks than previously thought, making previous explanations for the gender gap inadequate. To clarify, the gender gap does not appear to be driven by one gender being energized or by another being averse, as some previous research has suggested. Instead, the varied degree of receptiveness between the genders is responsible for the gap. Both genders are receptive to negativity in the same direction, but one is more receptive than the other. Therefore, the force driving the gender gap is best described in terms of differential receptivity.
The two macro-level results in which men's and women's reactions straddle the 5% significance threshold best demonstrate why differential receptivity is the most appropriate way to describe the gender gap. Specifically, in Trump's 2016 general election candidacy, women were significantly repulsed when Trump went on the attack, whereas men had no substantial reaction, as King and McConnell (Reference King and McConnell2003) as well as Kahn and Kenney (Reference Kahn and Kenney2004) found. Conversely, when Trump attacked Republican opponents in the primaries, women were statistically indifferent but men significantly rewarded such negativity, as Brooks (Reference Brooks2010) found. In both cases, there is substantial differential receptivity (Z δ), but these two particular gaps stretch across the significance threshold, thereby conforming to both competing results from previous literature. Conceptualizing the gender gap as differential receptivity accommodates these previous studies’ characterizations of the gender gap as well as the present findings.
CONCLUSION
Although the effects of negative political communication have been the subject of much research, often focused on traditional media formats, the field has too often overlooked the potential conditional relationship between supporter gender and response to negativity. Social media data provide a natural source for advancing research into negative campaigns. By extending observations of supporter behavior into the hundreds of millions, social media data can be used to comprehensively investigate whether men and women respond differently when candidates deliver negative messages, thereby testing for the presence of just such a conditional relationship. This study has done precisely that.
Using Facebook data collected from 10 different candidates across four elections, these findings reveal that men were uniformly more receptive to negativity than women. All four elections revealed statistically significant gender effects along these lines at both macro and micro levels. In addition, the empirical results from the elections studied revealed more complexity in the gender gap than was previously thought. In particular, although men and women differed significantly in the magnitude of their response (i.e., a gender gap), the direction of their response was largely similar (e.g., together rewarding or opposing attacks). These results provide strong support for a theory of differential receptivity between men's and women's responses to negative political communication. Differential receptivity captures this shared directional outcome while also accounting for a gap in the magnitude of response. An advantage of this conceptualization of the gender gap is that it can explain earlier (divergent) findings on gender differences as well as those of the current investigation.
In the present study, two potential explanations for the observed gender gap were examined: language preference and attacks on female candidates. Neither were sufficient to explain the gender gap. Regarding language preference, the gender gap dissipated insignificantly when female-preferred language was used in attacks. This slight decline offers some promise that further research may yet unveil more complex language discrepancies. To this end, future research should consider utilizing other language preference databases or techniques to investigate this phenomenon further.
Regarding attacks on female candidates, some limited evidence indicates that the gender of the target may be a partial determinant, but this evidence is insignificant. Given that Clinton is the exclusive focus of these attacks, it is entirely possible that voter opinions of her played a part in their response. For example, female targets with higher favorability ratings might magnify the gap. Further research should therefore seek to ascertain whether the effect on social media is stronger for more popular or less known candidates and would also benefit from considering shared voter and candidate gender in such models (see, e.g., Craig and Rippere Reference Craig and Rippere2016). New evidence suggests female candidates are more likely to adopt social media platforms in congressional races (Wagner, Gainous, and Holman Reference Wagner, Gainous and Holman2017), leaving future researchers with many potential subjects for observation.
Several other opportunities exist for the study of negative political communication in social media data. For example, voters conceptualize negative campaigns very differently than academics (Fridkin and Kenney Reference Fridkin and Kenney2019; Lipsitz and Geer Reference Lipsitz and Geer2017); thus, more closely defining negativity in these terms may yield new insights. Other potential determinants may exist within the content as well, such as the type of attack (e.g., whether issue or character), its nature (e.g., whether fair or unfair), and the complexity, quality, length, and the technical style of the attack (e.g., whether video content is edited or live; see Elder and Phillips Reference Elder and Phillips2017). Users (regardless of gender) react to attacks differently on social media depending on the election type, which is outside the focus of the current study, but more detailed investigation in this area may be helpful, as would examining receptiveness among other important subgroups of interest, such as partisans and independents.
Regardless of the direction of future research, these results demonstrate that gender is a factor well worth accounting for in research on negative political communication. Although television remains the primary method of communication with voters, social media platforms are becoming more prominent in elections. One day, social media, including Facebook, may overtake television's role in campaigning, and the gender gap may be magnified on these platforms. Undoubtedly, research will continue to expand into the realm of social media. However, investigators failing to account for gender, and its conditional relationship with negativity, in their explorations of either medium not only continue to ‘mask’ this differential in their research, as Brooks suggests (Reference Brooks2010), but also may risk producing underspecified models in doing so.
SUPPLEMENTARY MATERIAL
To view supplementary material for this article, please visit https://doi.org/10.1017/S1743923X1900059X.