Introduction
Modern agriculture is in constant development to surpass management challenges and achieve the high yields required to feed the exponentially growing world population. Weed management challenges have been pervasive for the last few decades due to the constant evolution and adaptation of weeds to chemical management practices (Perotti et al. Reference Perotti, Larran, Palmieri, Martinatto and Permingeat2020; Renton et al. Reference Renton, Busi, Neve, Thornby and Vila-Aiub2014).
Herbicides are the primary tool used to control and suppress weeds in broad-acre agriculture; however, herbicide resistance has reduced the effectiveness of many herbicides (Chauhan et al. Reference Chauhan, Matloob, Mahajan, Aslam, Florentine and Jha2017; Powles and Yu Reference Powles and Yu2010). Currently, more than 260 weed species have evolved herbicide resistance (Heap Reference Heap2021). The lack of herbicides with new sites of action (SOAs) and the overuse of the ones currently available have generated repeated selection pressure on weeds, resulting in the frequent occurrence of herbicide resistance (Délye et al. Reference Délye, Jasieniuk and Le Corre2013; Duke Reference Duke2012).
Waterhemp [Amaranthus tuberculatus (Moq.) Sauer] is one of the most troublesome weed species in the midwestern United States. Recent surveys from the Weed Science Society of America ranked A. tuberculatus as the most troublesome weed in U.S. soybean [Glycine max (L.) Merr.] fields and in Iowa, Illinois, and Nebraska cornfields (Zea mays L.) (Sarangi and Jhala Reference Sarangi and Jhala2018; Van Wychen Reference Van Wychen2016, Reference Van Wychen2019). Amaranthus tuberculatus has prolific seed production and can drastically reduce soybean and corn yields by 40% to 70%, respectively (Hager et al. Reference Hager, Wax, Stoller and Bollero2002; Steckel and Sprague Reference Steckel and Sprague2004). This species also can affect future crops due to its persistent soil seedbank (Korres et al. Reference Korres, Norsworthy, Young, Reynolds, Johnson, Conley, Smeda, Mueller, Spaunhorst, Gage, Loux, Kruger and Bagavathiannan2018).
The rapid evolution and selection of herbicide-resistance traits in A. tuberculatus represent a major challenge in managing this species. Amaranthus tuberculatus resistant to acetolactate synthase (ALS) and photosystem II (PSII) inhibitors was first identified in the 1990s; currently, resistance to herbicides from seven SOA groups (Groups 2, 4, 5, 9, 14, 15, 27) has been documented (Heap Reference Heap2021; Tranel Reference Tranel2021).
An A. tuberculatus population, designated CHR, was identified in 2012 in Champaign County, IL, after unsuccessful control with topramezone. CHR was initially characterized as resistant to herbicides from five SOA groups: synthetic auxins (2,4-D) and ALS, protoporphyrinogen oxidase, 4-hydroxyphenylpyruvate dioxygenase, and PSII inhibitors (Evans et al. Reference Evans, Strom, Riechers, Davis, Tranel and Hager2019). Subsequently, CHR was shown to also be resistant to inhibitors of very-long-chain fatty-acid synthesis (Strom et al. Reference Strom, Gonzini, Mitsdarfer, Davis, Riechers and Hager2019). Interestingly, the history of the field from which CHR was collected does not indicate previous usage of 2,4-D (Evans et al. Reference Evans, Strom, Riechers, Davis, Tranel and Hager2019), raising questions about the potential resistance to other auxinic herbicides such as dicamba.
Dicamba is a synthetic auxin herbicide that has been commercially available since the late 1960s to control a wide range of broadleaf weeds (Egan and Mortensen Reference Egan and Mortensen2012). Dicamba is an important herbicide in corn production and also in soybean following the release of dicamba-resistant soybean cultivars (Behrens et al. Reference Behrens, Mutlu, Chakraborty, Dumitru, Jiang, LaVallee, Herman, Clemente and Weeks2007). These cultivars have increased dicamba usage, as previously observed for glyphosate usage following the release of glyphosate-resistant crops (Heap and Duke Reference Duke2018). Increased use of dicamba will also raise selection for dicamba resistance in A. tuberculatus, similar to what occurred in other weed species, such as kochia [Bassia scoparia (L.) A.J. Scott] (LeClere et al. Reference LeClere, Wu, Westra and Sammons2018). Dicamba is also volatile; this could increase resistance selection due to exposure to sublethal rates from drift (Bish et al. Reference Bish, Farrell, Lerch and Bradley2019; Kniss Reference Kniss2018b; Tehranchian et al. Reference Tehranchian, Norsworthy, Powles, Bararpour, Bagavathiannan, Barber and Scott2017).
Evans et al. (Reference Evans, Strom, Riechers, Davis, Tranel and Hager2019) conducted field experiments in 2014 and 2015 with CHR and reported that dicamba, at the recommended field rate, provided 80% control. The lack of a more acceptable level of control prompted further investigation into potential dicamba resistance in this population.
This study presents the characterization and inheritance patterns of dicamba resistance in CHR. The objectives of this experiments were to: (1) quantify dicamba efficiency and compare it with efficiency of other synthetic auxin and alternative herbicides in the field; (2) characterize the current dicamba effectiveness on CHR via dose–response experiments; and (3) conduct a segregation analysis to identify whether dicamba resistance is a heritable trait in CHR.
Material and Methods
Field Experiments
Three separate field trials were conducted at the CHR location in Champaign County. All trials used a randomized complete block design with four replications and a 23.2-m2 plot area. The soil is a Flanagan silt loam (fine, smectitic, mesic Aquic Argiudolls) with a pH ranging from 5.5 to 6.2 and organic matter content of 4.8%. All trials were sprayed using a pressurized-CO2 backpack sprayer with a 3-m boom equipped with six TeeJet® (TeeJet Technologies, P.O. Box 7900, Wheaton, IL) AIXR110025 nozzles spaced 51 cm apart and calibrated to deliver 187 L ha−1. Herbicide efficacy was visually evaluated in all four experiments using a 0% (no control) to 100% (complete control) scale.
The first trial (Trial A) was conducted in 2016 with soybean planted on May 23. The objective of this trial was to evaluate the control with 2,4-D, dicamba, and 2,4-D + glyphosate (Table 1). All treatments were applied when soybean reached the V4 stage, with A. tuberculatus plant heights ranging from 7 to 10 cm. Control evaluations were conducted 14 d after treatment (DAT).
Table 1. Herbicides and application rates for field trials across multiple years.

The second and third trials (Trial B and Trial C) were conducted in 2018 with no crop planted. Trial B compared dicamba with glufosinate and included treatments of dicamba, glufosinate, and their combination, all at recommended field use rates (Table 1). Treatments were applied when A. tuberculatus plant height was 7 to 10 cm. Control was evaluated at 14 DAT.
Trial C consisted of a dicamba dose–response field experiment. Eight dicamba rates were applied when A. tuberculatus plants reached 7 to 10 cm height (Table 1). Control evaluations were conducted at 14, 21, and 30 DAT.
CHR-derived Populations
The population development of CHR for characterizing dicamba resistance followed a standard procedure for the generation of parental lines (R and S), F1 lines (F1), backcross (BC) lines, and a pseudo-F2 line (F2) (Figure 1). CHR seeds were collected in 2018 from plants in the field Trial C that survived postemergence-applied dicamba (560 g ae ha–1) and brought to the lab to generate populations.

Figure 1. Dicamba resistance population design. Initial field screening was conducted, and the most resistant plants were selected. Parental lines were developed, and reciprocal crosses were conducted to produce F1 lines. The most uniform F1 population was selected to further generate an F2 (pseudo-F2) and backcross (BC) populations.
To enhance germination, all seeds were subjected to a 50% commercial bleach treatment for 10 min and rinsed twice with water for 10 min each rinse. Seeds were suspended in a 0.1 g L−1 agarose solution and placed in a 4 C refrigerator for 4 wk (Bell et al. Reference Bell, Hager and Tranel2013). After stratification, seeds were germinated in petri dishes containing blotting paper with 2.0 ml of water. Petri dishes were closed with sealing film to avoid water loss and placed in a growth chamber for 48 h set for 12/12 h day/night and 32/15 C temperature regimes. After germination, seedlings were transplanted into pots and placed in the greenhouse. All laboratory-derived populations followed this germination process.
CHR plants with 6 to 7 leaves and 7 to 10 cm in height were treated with dicamba (560 g ha−1) to select the most resistant (R) plants for generating parental lines. Six male and six female plants demonstrating moderate to no auxinic damage at 21 DAT were selected for pairwise crossing to produce an R parental line. Seeds from the six R×R crosses were pooled and used as the R parental line. The Washington University Sensitive (WUS) population (Wu et al. Reference Wu, Davis and Tranel2018) was used as the herbicide-sensitive (S) line.
Reciprocal crosses were made between R and S parental lines to produce four F1 populations designated F1-1 and F1-2 (R female × S male) and F1-3 and F1-4 (S female × R male). F1 populations were treated with dicamba (560 g ae ha−1) at the 7- to 8-leaf stage and 7- to 10-cm height. Because waterhemp is dioecious, selfing F1 plants was impossible, so F1 plants were intermated to produce a pseudo-F2 (hereafter designated as F2). A preliminary screening of the F1 populations was conducted to select the F1 population with the most uniform phenotype for F2 and BC generation (data not shown). A female and a male from F1-1 were used to generate an F2 population. A backcross population (designated BC-1) was made using the same male F1-1 plant crossed with an S female. A second backcross (designated BC-2) was generated by crossing an F1-1 female and an S male.
Greenhouse Experiments
Two greenhouse experiments were conducted using the derived populations, and experiments were repeated. The first experiment was a whole-plant dicamba dose response, while the second consisted of a segregation analysis. Seeds for both experiments were germinated as previously described, and seedlings were transplanted into 164-cm3 Cone-tainers (Ray Leach SC10 “Cone-tainer,” 31933 Rolland Drive, Tangent, OR). Plants were kept under a mist bench programmed to water plants twice a day.
All Cone-tainers were filled with a custom sandy loam growth medium containing 1:1:1 (soil:peat:sand) and 3.3% organic matter, 6.8 pH. About 0.45 kg of a slow-release complete fertilizer (Osmocote® 13–13–13 slow-release fertilizer, Scotts, 14111 Scottslawn Road, Marysville, OH) was mixed into 200 kg of medium before planting. A supplement of 80 mg of additional Osmocote® fertilizer was added to the top of the growth medium in each Cone-tainer 1 wk after transplanting. The greenhouse was kept in a temperature and light regime of 28/22 C and 12/8 h, respectively.
Uniform plants were selected and sprayed at the 7- to 8-leaf stage and 8- to 10-cm height. All treatments in both experiments were sprayed using a compressed-air research sprayer (DeVries Manufacturing, 86956 State Highway 251, Hollandale, MN) using a TeeJet® 80015 EVS nozzle set to deliver 187 L ha−1 at 275 kPa. The nozzle was spaced 46 cm above the plant canopy.
A whole-plant dose–response experiment was conducted to characterize the level and dominance degree of dicamba resistance. The experiment used a randomized complete block design with six replications per treatment. Two dose–response runs were conducted as experimental replicates. The first dose–response (DR-1) run was conducted using the R, S, F1-1, F1-2, and F1-4 lines. Plants were treated with nine dicamba rates of 0, 1.18, 3.92, 11.8, 39.2, 118, 392, 1,180, and 2,350 g ha−1 (XtendiMax® with VaporGrip® Technology, Bayer CropScience, St Louis, MO). Dry weight biomass and visual survival estimation were obtained at 21 DAT. The second dose–response (DR-2) run was conducted using the same methodology as DR-1, but with the addition of the F1-3 population.
Segregation analysis was conducted to quantify the inheritance of dicamba resistance. Based on the dose–response trial, a rate of 500 g ha−1 of dicamba was selected as a delimiting rate. The R (148 plants), S (131 plants), F1-1 (100 plants), F1-2 (99 plants), F1-3 (80 plants), F1-4 (85 plants), BC-1 (147 plants), BC-2 (110 plants), and F2 (431 plants) lines were included in this experiment. Dry weight biomass, visual survival estimation, and plant area were recorded at 21 DAT.
Plant area was estimated using a previously described image analysis method (Bobadilla et al. Reference Bobadilla, Hulting, Berry, Moretti and Mallory-Smith2021). Briefly, multiple images at different angles were taken of each plant using a Fujifilm Xpro-2 camera with a 23-mm 2.0 Fujifilm lens (Fujifilm Manufacturing U.S.A., 211 Pucketts Ferry Road, Greenwood, SC) set on a tripod with the lens 60 cm from the plant. Images were analyzed on ImageJ software (Figure 2) to obtain plant area, as previously described by Bobadilla et al. (Reference Bobadilla, Hulting, Berry, Moretti and Mallory-Smith2021), and the average value between all angles was used as plant area (cm−2).

Figure 2. Dicamba damage evaluation methodology. Three measurements were used to estimate dicamba damage: visual estimation, plant area, and biomass. (A) The correlation between the three measurements; (B) the application of a Naïve Bayes classification to separate samples into two categories for chi-square analysis; (C) examples of the visual damage estimation; and (D) an example of the image analysis with ImageJ to capture plant area.
Data Analysis
All data analysis was conducted using R (R Core Team 2018), and plots were generated using the package Tidyverse (Wickham et al. Reference Wickham, Averick, Bryan, Chang, McGowan, François, Grolemund, Hayes, Henry and Hester2019). Field Trial A and B data were fit into linear regression models and subjected to an ANOVA and an HSD Tukey test (P-value < 0.05) using the package Agricolae (de Mendiburu Reference de Mendiburu2017). For Trial A, each application and evaluation timing were analyzed separately. Field Trial C data were analyzed using a three-parameter log-logistic model (Ritz et al. Reference Ritz, Baty, Streibig and Gerhard2015) comparing control efficacy among the three evaluation timings and different rates. Model assumptions for normality and heteroskedasticity were assessed using diagnostic plots and Shapiro-Wilk’s and Levene’s tests. Box-Cox transformation was conducted (Box and Cox Reference Box and Cox1964) when normality and/or heteroskedasticity assumptions were not met.
Whole-plant greenhouse dose–response data were analyzed using the drc package (Ritz et al. Reference Ritz, Baty, Streibig and Gerhard2015) to fit a log-logistic model for biomass, plant area, and survival estimates (Equation 1):

where y corresponds to the response, c the lower limit, d the upper limit, b the slope in which x refers to the dose, and ED50 the dose required to reduce the response to halfway between d and c. Biomass and plant area were normalized to the control treatment. Degree of dominance (Equation 2) was calculated for the F1 populations as proposed by Stone (Reference Stone1968):

where W 1, W 2, and W 3 are the log (ED50) of the sensitive parent, resistant parent, and F1 population, respectively, calculated from the log-logistic models. Because both biomass and plant area are representing the effects of dicamba on the tested populations, the degree of dominance was calculated for each variable, and the average from each was used as the final value for degree of dominance. DR-1 and DR-2 were analyzed separately.
For the segregation analysis, a chi-square goodness-of-fit test (χ2) was used to compare the observed and expected plant damage frequencies and determine whether the trait conforms to a single-gene model. Corrections to F2 and BC expected frequencies were applied based on observations of F1 and parental populations, assuming a single-gene model (Busi et al. Reference Busi, Neve and Powles2013; Han et al. Reference Han, Yu, Vila-Aiub and Powles2014; Huffman et al. Reference Huffman, Hausman, Hager, Riechers and Tranel2015). Levene’s test of homogeneity was used to check whether data from the two experimental replicates could be pooled. Due to the difficulty in estimating auxinic herbicide damage, plant damage was estimated using the three described measures: visible damage (severe, partial, and no damage), plant biomass, and plant area (Figure 2). Visible damage was estimated based on the observed severity of auxinic herbicide symptoms: plants with “severe damage” typically displayed severe chlorosis, stunting of growth, and/or epinasty; those with “partial damage” displayed moderate growth reduction and epinasty; and plants classified as “no damage” exhibited few, if any, symptoms.
The three variables were combined to achieve a binomial damage classification (no/partial damage and severe damage) with a Naïve Bayes classifier using the package e1071 (Meyer et al. Reference Meyer, Dimitriadou, Hornik, Weingessel, Leisch, Chang and Lin2019) to apply the data into the chi-square goodness-of-fit test. Using 25% of the data as a training set, the Naïve Bayes model achieved 84% classification accuracy, with sensitivity, specificity, and balanced accuracy each over 80%. Broad-sense heritability was calculated for the F2 population based on the variances of biomass and plant area (Equation 3):

where V g refers to the genetic variance, V p refers to the phenotypic variance, and V e refers to the environmental variance. V e was estimated based on the observed parental and F1 biomass and plant area variances. Homogeneity of variances across replications was uniform and combined for heritability estimation.
Results and Discussion
Field Trials
Field Trial A compared the effects of 2,4-D, dicamba, and 2,4-D combined with glyphosate at multiple rates (Figure 3A). CHR was previously characterized as 2,4-D resistant (Evans et al. Reference Evans, Strom, Riechers, Davis, Tranel and Hager2019) and 2,4-D control at 1X never exceeded 30%, while a 2X rate provided 45% control. When glyphosate was included with 2,4-D, A. tuberculatus control increased to 90%, indicating CHR remains susceptible to glyphosate. The response of CHR to dicamba was similar to response to 2,4-D. Control of CHR was less than 50% with 1X dicamba (560 g ai ha−1) and only 60% with 2X dicamba (1,200 g ai ha−1).

Figure 3. Field trial results comparing dicamba and alternative herbicides. (A) Field Trial A: Amaranthus tuberculatus control comparison between dicamba, 2,4-D, and, 2,4-D + glyphosate at multiple rates 14 d after treatment (DAT). Herbicide rates were 0, the recommended field rate (1X), or double the recommended field rate (2X). (B) Field Trial B: dicamba, glufosinate, and their combination (at 1X rates) compared with untreated control plots. Error bars indicate the estimated standard errors. (C) Dose–response of the CHR A. tuberculatus population to dicamba in the field. Curves represent three evaluations made at 14, 21, and 30 DAT. Blue dashed line represents the dicamba recommended field rate; solid lines represent the effective dose for 50% control estimated from each evaluation time, with the 50% control level represented by a dashed red line.
Field Trial B compared CHR control from dicamba, glufosinate, or their combination (Figure 3B). Glufosinate + dicamba provided the greatest control, with an average of 95%, while glufosinate alone controlled CHR 92%. In contrast, control with dicamba was less than 75%. Field Trial C was a field dose response to quantify the resistance level of CHR to dicamba (Figure 3C). Dose–response results show that dicamba provided less than 65% control at the 1X rate. The ED50 values at 14 and 21 DAT were approximately 0.36 kg ha−1, while at 30 DAT, ED50 was 0.63 kg ha−1 (Table 2), greater than the 1X rate. The effective dose needed to achieve 90% control for all evaluation times exceeded 3 kg ha−1, indicating that control is not feasible at the current labeled field rate.
Table 2. Parameter estimates from a three-parameter log-logistic regression model fit for dose–response field trial data collected 14, 21, and 30 d after treatment (DAT).

a ED50 is the dose necessary to achieve 50% control.
In 2015, dicamba controlled CHR 80% to 94% (Evans et al. Reference Evans, Strom, Riechers, Davis, Tranel and Hager2019). All field trials conducted after 2015 indicate a decrease in dicamba’s efficacy to an average of 65%. Experimental and environmental variability should also be considered as potential sources of variation from previous trials conducted at this site; however, current results showed a pattern of dicamba resistance evolution in CHR.
The CHR field has no recent history of dicamba application, leading to some potential hypotheses about the evolution of dicamba resistance in this population. CHR was previously characterized as resistant to herbicides from six different SOAs, including 2,4-D, suggesting the possibility of cross-resistance with other synthetic auxin herbicides such as dicamba (Evans et al. Reference Evans, Strom, Riechers, Davis, Tranel and Hager2019; Strom et al. Reference Strom, Gonzini, Mitsdarfer, Davis, Riechers and Hager2019). Cross-resistance between synthetic auxin herbicides is confirmed for other weed species such as B. scoparia (LeClere et al. Reference LeClere, Wu, Westra and Sammons2018). Non–target site resistance is a potential mechanism that can reduce the efficacy of multiple herbicides (Jugulam and Shyam Reference Jugulam and Shyam2019; Yuan et al. Reference Yuan, Tranel and Stewart2007), and because metabolic resistance to S-metolachlor, atrazine, and mesotrione was previously identified in CHR (Ma et al. Reference Ma, Kaundun, Tranel, Riggins, McGinness, Hager, Hawkes, McIndoe and Riechers2013; Strom et al. Reference Strom, Hager, Seiter, Davis and Riechers2020), this could be a potential explanation for dicamba resistance.
Interestingly, the decline in dicamba effectiveness against CHR overlaps with the increased usage of dicamba-resistant cultivars (Spaunhorst et al. Reference Spaunhorst, Siefert-Higgins and Bradley2014; Werle et al. Reference Werle, Oliveira, Jhala, Proctor, Rees and Klein2018). Even though none of the field trials used dicamba-resistant soybean cultivars, these cultivars are grown in fields surrounding the CHR field, which could contribute to resistance evolution from sublethal drift events (Bish et al. Reference Bish, Farrell, Lerch and Bradley2019; Kniss Reference Kniss2018b; Tehranchian et al. Reference Tehranchian, Norsworthy, Powles, Bararpour, Bagavathiannan, Barber and Scott2017). The increased usage of herbicide-resistant crops such as glyphosate-resistant crops already showed a correlation with increased selection pressure for herbicide resistance in weeds (Duke Reference Duke2018; Heap and Duke Reference Duke2018; Kniss Reference Kniss2018a).
Another potential explanation for this evolved dicamba resistance is gene flow from another A. tuberculatus population. Cross-pollinated weed species are commonly known to exchange herbicide-resistance traits via gene flow (Beckie et al. Reference Beckie, Busi, Bagavathiannan and Martin2019; Jhala et al. Reference Jhala, Norsworthy, Ganie, Sosnoskie, Beckie, Mallory-Smith, Liu, Wei, Wang and Stoltenberg2020). Examples of gene flow in A. tuberculatus, within the species and between other Amaranthus species, have been documented (Franssen et al. Reference Franssen, Skinner, Al-Khatib, Horak and Kulakow2001; Jhala et al. Reference Jhala, Norsworthy, Ganie, Sosnoskie, Beckie, Mallory-Smith, Liu, Wei, Wang and Stoltenberg2020; Liu et al. Reference Liu, Davis and Tranel2012; Sarangi et al. Reference Sarangi, Tyre, Patterson, Gaines, Irmak, Knezevic, Lindquist and Jhala2017). However, this is the first confirmed case in Illinois of dicamba resistance in A. tuberculatus, making this scenario less likely. New studies and surveys in the area assessing the current overall effectiveness of dicamba are needed to test this hypothesis.
Greenhouse Trials
To estimate the dicamba resistance level and inheritance, a greenhouse study was conducted that included two dose–response experiments (Table 3; Figure 4). Multiple F1 populations from reciprocal crosses were tested along with the R and S parents. Both experiments were consistent in indicating that the R population is less sensitive to dicamba compared with the WUS sensitive control. Resistance indexes were calculated for biomass and plant area, and then the average between the two measurements was calculated. Resistance indexes of 5.6 and 10.6 were obtained for the R parental line relative to WUS in the two runs, respectively. The resistance index differences are potentially an artifact of environmental factors leading to an increase in sensitivity in the WUS population (Table 3), which previously have been shown to have an effect on the resistance level for synthetic auxin herbicides (Johnston et al. Reference Johnston, Vencill, Grey, Culpepper, Henry and Czarnota2019).
Table 3. Greenhouse dose–response trial ED50 estimates. a

a Results are from two dose–response runs (DR-1 and DR-2). ED50 refers to the dose necessary to reduce 50% of biomass, survival, or plant area.
b CHR is the resistant parental line, while WUS is the sensitive parental line.

Figure 4. Dicamba dose–response curves from greenhouse trials. (A and B) Biomass reduction for each dose–response experiment: (A) dose–response run 1 (DR-1); and (B) dose–response run 2 (DR-2). Ribbons refer to lower and upper limits estimated by log-logistic models for each population. Each F1 population was obtained from a pairwise cross using plants from the dicamba-resistant (CHR) and dicamba-sensitive (WUS) parental populations. CHR plants were used as females for generating F1-1 and F1-2, and as males for generating F1-3 and F1-4 populations.
All F1 population curves were between those of the parental lines, indicating an intermediate resistance level (Figure 4). There was a consistent trend of the F1 populations derived from a maternal resistant plant (F1-1 and F1-2) showing a larger resistance level compared with the F1 crosses derived from a resistant male parent (F1-3 and F1-4; Table 3). This would indicate some maternal inheritance, consistent with a previous study demonstrating that some auxin responses in Arabidopsis thaliana are partitioned between nuclear and cytoplasmatic loci (Powers et al. Reference Powers, Holehouse, Korasick, Schreiber, Clark, Jing, Emenecker, Han, Tycksen, Hwang, Sozzani, Jez, Pappu and Strader2019). However, the difference observed between reciprocal F1s was not significant (ED50 t-test P-value = 0.18). Consequently, we conclude that dicamba resistance in CHR is primarily nuclear inherited, although a minor contribution of maternal inheritance cannot be completely ruled out. Differences between F1 lines also could result from the use of heterogenous parental lines (a challenge when working with a dioecious species), particularly in this study, in which the R parental line was a pool of multiple R×R plant crosses.
The degree of dominance (D) was calculated for each F1 population individually (Table 4). For three of the F1 lines, across both dose–response experiments, D ranged from 0.13 to 0.50, indicating an incompletely dominant trait. The F1-3 population, which was included in only the second dose–response experiment, yielded a D of −0.15, indicating an incompletely recessive trait. Again, the lack of homogenous parental lines makes accurate determination of D for a trait of interest difficult. If a resistant parent is not homozygous at all resistance loci, the degree of dominance based on F1 progeny would likely be underestimated. Incomplete dominance was already documented for other non–target site resistance traits in A. tuberculatus populations from Illinois (Huffman et al. Reference Huffman, Hausman, Hager, Riechers and Tranel2015). Other studies also showed that herbicide resistance in A. tuberculatus could be additive, incompletely dominant, or even incompletely recessive (Oliveira et al. Reference Oliveira, Gaines, Jhala and Knezevic2018).
Table 4. Degree-of-dominance values based on biomass and plant area log-ED50 for each F1 population compared with the parental lines (CHR and WUS). a

a Results are from two dose–response runs (DR-1 and DR-2). ED50 refers to the dose necessary to reduce 50% of biomass, survival, or plant area.
Segregation analysis was conducted to characterize the inheritance pattern of dicamba resistance. Results suggest that dicamba resistance does not follow a single nuclear gene inheritance pattern (i.e., expected R:S ratios of 3:1 and 1:1 for F2 and BC populations, respectively). Both F2 and BC populations significantly deviated from expected ratios (Table 5). Interestingly, F2 and BC lines deviated in opposite directions: whereas the F2 population contained fewer resistant plants than expected, the BC populations had more resistant plants than expected. This scenario could be due to epistatic gene interactions, nonuniformity of the parental populations used for the crosses, or misplacement by the classification method (84% classification accuracy). Nevertheless, dicamba resistance in neither the F2 nor the BC populations followed a single gene model. Dicamba resistance heritability, based on both biomass and plant area, was quantified as fair to moderate (H 2 = 0.27). These results indicate that dicamba resistance in CHR is heritable and likely a multigenic trait.
Table 5. Chi-square results from the pooled segregation analysis experiments. a

a Plants were classified into two groups (no/partial damage and severe damage) to be used in the chi-square analysis.
b F1-R♀×S♂ refers to the F1-1 and F1-2 populations, while F1-S♀×R♂ refers to the F1-3 and F1-4 populations.
c Expected ratios were corrected based on parental populations and on the F1-1 population, which was used to generate both backcross (BC) and F2 populations.
d Chi-square analysis was based on the model for a single, dominant gene.
Although the F2 population showed clear segregation for dicamba resistance (Figure 5), the distributions of phenotypes for both biomass and plant area were continuous (Figure 6). Similarly, there was a lack of distinct phenotypic classes within the BC lines, further evidence that dicamba resistance in CHR is a multigenic trait. It is important to note that parental and F1 lines also had relatively broad distributions, which can be attributed to lack of homogeneity within each parental line, environmental variation in dicamba response (Flasiński and Hąc-Wydro Reference Flasiński and Hąc-Wydro2014; Johnston et al. Reference Johnston, Vencill, Grey, Culpepper, Henry and Czarnota2019), and difficulty in quantifying responses to growth-regulator herbicides. The relatively low level of dicamba resistance, coupled with the heretofore mentioned challenges, limits the conclusions that can be drawn about the inheritance of the trait. Nevertheless, the greenhouse results collectively confirm that dicamba resistance is present in CHR and is heritable. Dicamba resistance is relatively low level (about 5- to 10-fold) compared with the other resistance traits present in CHR. Our results suggest fair to moderate heritability of the trait; however, heritability values tend to be higher in controlled environments (Young et al. Reference Young, Stanton, Ellstrand and Clegg1994). This potential lower heritability coupled with environmental factors at the field level may suggest why this trait was not yet identified in other populations. However, it should also be noted that the lack of genetic uniformity within the parental and F1 lines would lead to an overestimation of environmental variation and, consequently, to an underestimation in the heritability of the trait.
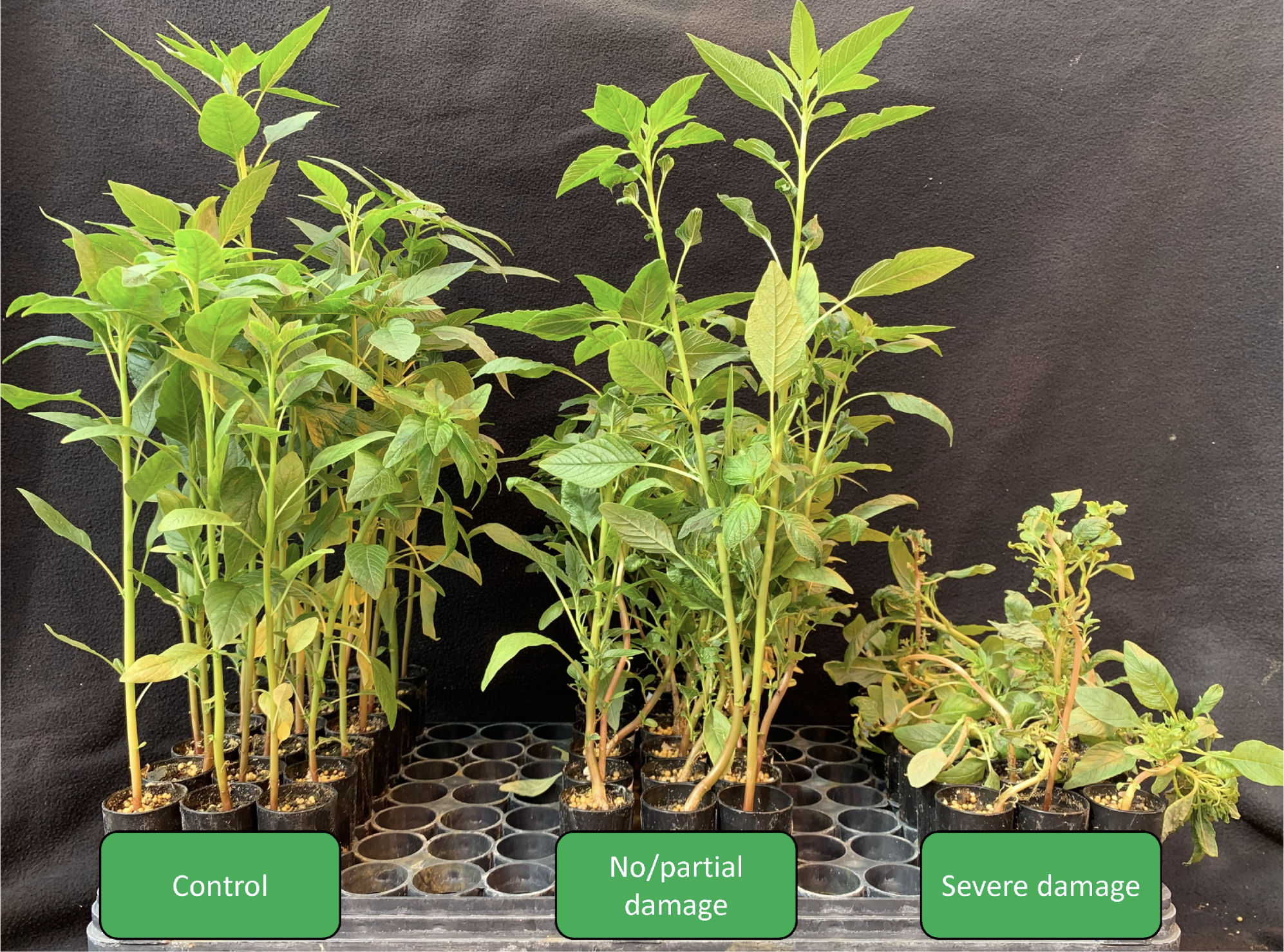
Figure 5. F2 plant phenotypes at 21 days after 560 g ae ha−1 of dicamba compared with untreated control plants.

Figure 6. Segregation analysis using plant area (A) and biomass (B) distributions. Histograms (bin = 30) show the number of individuals distributed according to their plant area and biomass measurements. F1 populations within reciprocal crosses were pooled.
Dicamba Resistance and Next Steps
Field trials over multiple years and greenhouse trial results show that dicamba resistance is present in CHR, making this one of the first cases of dicamba resistance within the Amaranthus genus (Dellaferrera et al. Reference Dellaferrera, Cortés, Panigo, De Prado, Christoffoleti and Perreta2018).
These results add a new challenge for growers in the Midwest region to overcome (Bish et al. Reference Bish, Farrell, Lerch and Bradley2019; Werle et al. Reference Werle, Oliveira, Jhala, Proctor, Rees and Klein2018). Due to the ability of herbicide-resistant A. tuberculatus to rapidly spread, the addition of dicamba resistance will require growers to reevaluate their weed management strategies to mitigate the spread of dicamba resistance (Murphy et al. Reference Murphy, Larran, Ackley, Loux and Tranel2019; Tranel Reference Tranel2021). Our results show that other postemergence herbicides such as glufosinate and glyphosate still provide good control of CHR. Although not present in the CHR population, glyphosate resistance is common in A. tuberculatus populations, whereas glufosinate resistance has not yet been confirmed (Tranel Reference Tranel2021). CHR can rapidly metabolize chloroacetamide herbicides, indicating a necessity to identify optimal preemergence herbicide options (Strom et al. Reference Strom, Hager, Seiter, Davis and Riechers2020).
Dicamba usage has increased with the introduction of resistant crops, which may contribute to other resistant populations over time. Conducting future surveys and quantifying the overall response to dicamba in multiple populations would provide useful data regarding dicamba resistance. This constant evolution and adaptation of A. tuberculatus to different herbicides shows the evolutionary potential of this species, highlighting the need for integrating nonchemical tactics and for new herbicides.
CHR has evolved resistance to herbicides from six SOAs (Evans et al. Reference Evans, Strom, Riechers, Davis, Tranel and Hager2019; Strom et al. Reference Strom, Gonzini, Mitsdarfer, Davis, Riechers and Hager2019). Previous research using physiology and transcriptomics approaches reveals this population has metabolic resistance to S-metolachlor, 2,4-D, atrazine, mesotrione, and tembotrione, with both cytochrome P450 and glutathione-S-transferase genes implicated (Giacomini et al. Reference Giacomini, Patterson, Küpper, Beffa, Gaines and Tranel2020; Strom et al. Reference Strom, Hager, Seiter, Davis and Riechers2020). Our results point to a possible dicamba cross-resistance scenario caused by a previously existing non–target resistance mechanism in CHR.
The phenotypes observed after dicamba treatment show that some auxinic damage, such as epinasty and leaf curling, still occur, suggesting that dicamba is still binding to auxin binding sites. Dicamba resistance is possibly mediated by limiting production of reactive oxygen species (ROS) or by reducing dicamba efflux and uptake, which would also reduce ROS production (Busi et al. Reference Busi, Goggin, Heap, Horak, Jugulam, Masters, Napier, Riar, Satchivi, Torra, Westra and Wright2018; Todd et al. Reference Todd, Figueiredo, Morran, Soni, Preston, Kubeš, Napier and Gaines2020). Dicamba resistance also could be associated with reduced abscisic acid synthesis and accumulation, leading to less production of ROS (Gaines Reference Gaines2020).
RNA-seq experiments will be conducted to quantify differential gene expression patterns and identify putative genes related to the trait. It will be interesting to determine whether the previously identified genes implicated in herbicide resistance in CHR are also playing a role in dicamba resistance (Giacomini et al. Reference Giacomini, Gaines, Beffa and Tranel2018, 2020).
Acknowledgments
This research was partially supported by funds from Bayer CropScience. No other conflicts of interest have been declared.