Introduction
Over a third of the world's population is either overweight or obese today (Stevens et al., Reference Stevens, Singh, Lu, Danaei, Lin, Finucane, Bahalim, McIntire, Gutierrez, Cowan, Paciorek, Farzadfar, Riley and Ezzati2012; Ng et al., Reference Ng, Fleming, Robinson, Thomson, Graetz, Margono and Gakidou2014). By 2030, an estimated 58% of the world's adult population will be overweight or obese (Kelly et al., Reference Kelly, Yang, Chen, Reynolds and He2008). Obesity greatly increases the risks of getting many chronic noncommunicable diseases, including Type 2 diabetes, cardiovascular disease, hypertension, kidney disease, and some types of cancer. Because the increasing prevalence of obesity will lead to substantial disease burdens on many societies, governments are increasingly looking for innovative approaches to combat obesity at the community level. Thus, interventions that can be scaled up to promote weight loss for large numbers of people are of particular interest to policymakers and organizations that run weight loss programs.
Weight loss programs that have the capacity to reach a large number of overweight individuals must be fundamentally self-directed (i.e., involve little or no healthcare provider participation). The challenge, therefore, is to motivate actual weight reduction in the context of a self-help program. Governments, not-for-profit organizations, and companies have explored the use of financial incentives to motivate weight loss in such programs. For example, commercial programs such as Dietbet and Fatbet motivate their clients by getting them to bet on meeting certain weight loss goals; the clients get their money back and make additional money if they achieve their goals, but lose their money if they fail. Government agencies that target at nationwide populations diverse in financial status and ethnicity tend to use simple positive incentives that contain no betting elements. For example, in the ‘Weigh and Win’ program run by Kaiser Permanente, the ‘Million KG Challenge’ by the Singapore Health Promotion Board, and the ‘Pounds for Pounds’ pilot program funded by the UK National Health Service, cash rewards are tied directly to each individual participant's weight loss result (e.g., win $X upon meeting a specific weight loss target during a specific period).
The use of weight loss incentives has gained traction in public health settings partly because weight loss at any age could lead to cost savings; even going from obese to overweight can result in lower medical costs and lower productivity loss (Fallah-Fini et al., Reference Fallah-Fini, Adam, Cheskin, Bartsch and Lee2017). An important consideration concerning such uses of financial incentives is whether the incentives work equally for everyone, specifically between genders. Having an empirical answer to this question is important: It will push policy makers to reconsider the provision of incentives for weight loss as an effective policy for all (see Al-Ubaydli et al., Reference Al-Ubaydli, Lee, List, Mackevicius and Suskind2019a, Reference Al-Ubaydli, List and Suskind2019b; Ho et al., Reference Ho, Leong and Yeung2021 for a discussion of the voltage drop problem) and motivate behavioral scientists to design gender-specific interventions for solving the obesity problem. In this report, we provide evidence that financial incentives work differently for the two genders.
Our RCT and past-related research
We conducted a two-arm randomized controlled trial (RCT) stratified by gender to examine how overweight individuals respond to a positive incentive for weight loss. The treatment arm, IW (incentive for weight loss), was a cash award of US$150 given for achieving 5% weight loss over 12 weeks. We compared the effectiveness of IW to control in promoting weight loss in overweight individuals and investigated whether incentives work equally well for men and women. We also evaluated IW for its potential to be implemented at a scale by testing it in the context of a large-scale self-directed weight loss program.
Our research built on behavioral economics theories that explain why weight loss attempts often fail and how financial incentives might promote weight loss. Accordingly, people tend to discount future benefits of weight loss and demonstrate time-inconsistent preferences (Downs & Loewenstein, Reference Downs, Loewenstein and Cawley2011). When people are present-biased, the immediate costs of undertaking a weight loss regime (increasing exercise, switching to a healthy diet) and the immediate enjoyment of eating unhealthy food are more salient, in comparison with the future benefits of weight loss (e.g., reduced risks of chronic diseases such as heart disease and Type 2 diabetes) and the future costs of being overweight (e.g., reduced quality of life and medical expenses). Consequently, there is a tendency to assign too little weight to future payoffs relative to immediate payoffs in decision making; this tendency is often referred to as ‘hyperbolic discounting.’ (For evidence, see Loewenstein & Thaler, Reference Loewenstein and Thaler1989; Ainslie Reference Ainslie1991, Reference Ainslie1992; Akerlof, Reference Akerlof1991; Loewenstein & Prelec, Reference Loewenstein and Prelec1992; Ainslie & Haslam Reference Ainslie, Haslam, Loewenstein and Elster1992a, Reference Ainslie, Haslam, Loewenstein and Elster1992b; Laibson, Reference Laibson1997; O'Donoghue & Rabin, Reference O'Donoghue and Rabin1999). Financial incentives serve to add an immediate reward for weight loss, thereby tipping the cost-benefit tradeoff toward behavioral change.
The notion that financial incentives could motivate weight loss has been supported by a body of evidence acquired through proof-of-concept studies (i.e., ‘Wave 1’ studies; List Reference List2020) (Finkelstein et al., Reference Finkelstein, Linnan, Tate and Birken2007, Reference Finkelstein, Tham, Haaland and Sahasranaman2017; Volpp et al., Reference Volpp, John, Troxel, Norton, Fassbender and Loewenstein2008; John et al., Reference John, Loewenstein, Troxel, Norton, Fassbender and Volpp2011; Kullgren et al., Reference Kullgren, Troxel, Loewenstein, Asch, Norton, Wesby, Tao, Zhu and Volpp2013). These studies, like many other proof-of-concept studies, used relatively homogeneous participant samples and restricted experimental settings; therefore, the generalizability of their findings remains unclear. In terms of participant samples, these RCTs have focused on either predominantly (i.e., >75%) male (Volpp et al., Reference Volpp, John, Troxel, Norton, Fassbender and Loewenstein2008; John et al., Reference John, Loewenstein, Troxel, Norton, Fassbender and Volpp2011) or female participants (Finkelstein et al., Reference Finkelstein, Linnan, Tate and Birken2007; Kullgren et al., Reference Kullgren, Troxel, Loewenstein, Asch, Norton, Wesby, Tao, Zhu and Volpp2013). Moreover, the sample sizes of these studies were not large enough for a statistical evaluation of gender difference (sample sizes of these studies ranged from 57 to 207; all employed a three-arm design). In terms of experimental setting, many of these RCTs were conducted in clinical contexts (e.g., medical centers and hospitals in Volpp et al., Reference Volpp, John, Troxel, Norton, Fassbender and Loewenstein2008, John et al., Reference John, Loewenstein, Troxel, Norton, Fassbender and Volpp2011, Kullgren et al., Reference Kullgren, Troxel, Loewenstein, Asch, Norton, Wesby, Tao, Zhu and Volpp2013, and Finkelstein et al., Reference Finkelstein, Tham, Haaland and Sahasranaman2017). These contexts were distinct from large-scale rollouts in which healthcare providers’ involvement is minimal.
Our RCT was a ‘Wave 2’ study (List Reference List2020) that built on the foundations of the previous Wave 1 studies. Our primary goal was to evaluate the effects of weight loss incentives (IW) in a context that more resembled a community rollout. Our RCT met two important criteria that defined it as a Wave 2 study: First, it involved a heterogeneous group of participants recruited from all over Singapore through publicly accessible media (nationwide newspaper advertisements). Moreover, it had a sample size of 472, making it possible to examine gender difference in the effect of incentives on weight loss. Second, we examined the impact of IW in an experimental setting that is much more scalable, specifically, in the context of an online self-directed weight loss program.
The literature has documented evidence from other Wave 2 studies conducted based on workplace wellness programs with larger sample sizes (e.g., Cawley & Price, Reference Cawley and Price2013; Misra-Hebert et al., Reference Misra-Hebert, Hu, Taksler, Zimmerman and Rothberg2016). Notably, Cawley and Price (Reference Cawley and Price2013) found only modest effect of financial incentives on weight loss, which contrasts with the better outcomes reported in previous proof-of-concept studies and suggests a potential ‘voltage drop’ problem. Nevertheless, these findings must be interpreted with the caveat that randomized designs were not used in these studies. Other weight loss studies that did not employ a randomized design or did not use an intent-to-treat (ITT) analysis are not discussed further (see Ananthapavan et al., Reference Ananthapavan, Peterson and Sacks2018).
Research design
The online weight loss program
A 12-week, self-administered online weight loss was a program based on the University of Pittsburg's Lifestyle Balance Program (The DPP Research Group, 2002). The following topics were covered in the program, in sequence: (1) The risks of being overweight; (2) Be a smart eater; (3) Healthy eating; (4) Move those muscles; (5) Tip the calorie balance; (6) Take charge of what's around you; (7) Problem solving; (8) Healthy eating while out; (9) The slippery slope of lifestyle change; (10) Jump start your physical activity plan; (11) Eating and exercising while away; and (12) Preparing for self-management. The RCT was conducted in Singapore; therefore, the content was adapted for the local culture, diet, and lifestyle; it was also modified to suit bite-size e-learning. The material was developed by the research team and verified by a clinical team consisting of an endocrinologist, a psychologist, a physiotherapist, and two dieticians.
The online program was delivered through 12 weekly sessions. All participants were enrolled in the same online program, had access to the videos, and were given a weight loss goal of 5% of their baseline body weight by the end of the program.
RCT design and timeline
There were two arms in this RCT: (1) Control arm with no financial incentive for weight loss and (2) IW arm with a US$150 (S$200) incentive for losing at least 5% of baseline weight by the end of the program. In other words, although all participants were given the goal of losing 5% of weight and had access to the online program, only those in the IW arm were incentivized to meet the 5% weight loss goal.Footnote 2
The RCT consisted of two periods: a 12-week intervention and a 12-week post-intervention (Figure 1). Participant weight was measured by trained research assistants at three points: right before the intervention (at Week 0), at the end of the intervention (at Week 13), and at the end of the post-intervention period (Week 25). All participants received US$65 (S$88) as a participation fee for attending all three weigh-ins. This amount was split into two payments: US$15 was paid at the Week 13 weigh-in and US$50, at the Week 25 weigh-in. The IW incentive was tied to attendance at all three weigh-ins and was paid at the Week 25 weigh-in.

Figure 1. Timeline of the RCT.
Participants: selection criteria, recruitment, and randomization
The weight loss program was a nationwide program conducted in Singapore. Participants had to meet the following eligibility criteria: age between 40 and 60; body mass index (BMI) between 23 and 33 kg/m2; not pregnant or planning to get pregnant; free from chronic diseases that require medical attention, including diabetes, cardiovascular disease, high blood pressure, and lung disease. A BMI of 23 kg/m2 was chosen as Asian individuals above this BMI are considered to be overweight and at a greater risk for cardiometabolic complications (WHO Expert Consultation, 2004). The BMI upper limit of 33 kg/m2 was chosen to minimize the influence of outliers on the main result of weight loss, as less than 5% of the Singapore population has a BMI of 34 kg/m2 and higher according to a National Health Survey conducted by the Singapore Ministry of Health in 2004. The exclusion criteria concerning chronic diseases were imposed to ensure that participants were medically suited to undertake a self-administered weight loss program. All selection criteria were set before subject recruitment commenced.
Participants were recruited from the public through newspaper advertisements. The newspaper advertisement provided the general public with the following key information: (1) the program was an online weight loss program with the objective of helping participants lose 5% of their body weight; (2) the participants could earn a participation fee of S$88 (US$65); and (3) participants must be 40–60 years old with a BMI between 23 and 33. The recruitment advertisement is shown in Appendix A. Potential participants were invited to visit a website for an initial screening. Those who passed the screening were invited to attend a weigh-in session at Week 0, where they had their weight and height measured, age verified, and other baseline measures collected. They were advised to log in to the program's website after the weigh-in.
Randomization took place when the participants first logged on to the website. The randomization sequence followed a stratified block randomization scheme with 8 gender-ethnicity strata [2 (Male, Female) × 4 (Chinese, Malay, Indian, Others)Footnote 3]. In other words, the participants were first sorted by gender and ethnicity, and then randomly assigned to one of two arms. After randomization, information on the additional financial incentives offered in the IW arm was shown to participants via an automated message shown onscreen. A total of 472 participants (of which 171 were males) were randomly assigned to one of the two armsFootnote 4.
Outcome measures
The first outcome of interest was weight loss after 12 weeks (measured at Week 13), which revealed whether IW led to a greater weight loss. To provide an unbiased comparison of weight loss between genders, we chose percentage weight loss rather than absolute weight loss as the unit of analysis because the former accounted for baseline weight differences between the two genders. Percentage weight loss was defined as the difference in weight between Week 13 and Week 0, divided by weight at Week 0.
Weight loss outcomes were analyzed using both the ITT approach and the per-protocol (PP) approach as a strategy to handle the potential effects of non-compliance on our empirical tests. In the ITT analysis, participants who did not attend a weigh-in were still included in the analysis and were treated as having the same weight at Week 0. The PP approach included only the participants who attended all three weigh-ins. The ITT approach relies solely on an exogenous source of variation (i.e., randomization procedure) and is free from other endogenous sources of influence (e.g., selection bias introduced by dropout); therefore, it provides the cleanest possible evaluation of treatment effects from a methodological standpoint (for further discussion of why the ITT approach is the standard approach in the analysis of any RCT, see Glennerster & Takavarasha, Reference Glennerster and Takavarasha2013). Nevertheless, the PP approach informs us the effect of a treatment conditional on compliance to experimental requirements. Hence, the two approaches together constitute a sensitivity analysis to verify that our results hold, irrespective of (non-) compliance issues. Throughout our article, we discuss our results based on the ITT approach, but we present the PP analysis results alongside the ITT results in tables.
To control the family-wise error rate, we performed multiple hypothesis testing (MHT) and reported MHT-adjusted p-values for statistically significant results. MHT was conducted based on the resampling-based stepdown method developed by Romano and Wolf (Reference Romano and Wolf2005a, Reference Romano and Wolf2005b, Reference Romano and Wolf2016) with 1000 bootstrap replications.Footnote 5 We also collected data on weekly exercise level and diet quality; such responses were self-reported on a voluntary basis. Readers who are interested in these measurements can refer to Appendix B.
Estimation strategy
To evaluate the effect of the incentive on the weight loss and its differential effect by gender, we estimate the following ordinary least squares (OLS) regression:

where y i is the main outcome: the percentage of weight loss at Week 13 or at Week 25, IWi, is the dummy variable for the treatment group, Malei is the indicator for male participants, and IW*Malei is the interaction term between the treatment status and the gender dummy. X i includes the covariates: the baseline BMI, age, and educational level. Throughout we run OLS regressions with heteroscedasticity-consistent standard errors.
Results
Participant characteristics and attrition
A total of 472 participants were randomly assigned to one of the two arms (males: Control = 85, IW = 86; females: Control = 150, IW = 151). Of the 472 participants we recruited, 171 were male. Our male participants were 47.8 years old on average (SD = 4.8), with an average BMI of 27.0 kg/m2 (SD = 2.5). Our female participants were 48.9 years old on average (SD = 5.4), with an average BMI of 26.6 kg/m2 (SD = 2.5). Other participant characteristics are shown in Table 1. Statistical tests were conducted separately for each baseline measure. In terms of age, BMI, and education levels, males had a slightly higher BMI than females (F(1, 468) = 3.54, p = 0.06), were on average one year younger than females (F(1, 468) = 4.63, p = 0.03), and had higher levels of education (Mantel–Haenszel test: χ 2(1) = 16.40, p < 0.001). Males also had higher levels of income than females (Mantel–Haenszel test: χ 2(1) = 33.73, p < 0.001; this result remains the same if the ‘no income’ category was excluded: χ 2(1) = 31.36, p < 0.001).
Table 1. Baseline measures by gender and treatment arm.

Notes:
1 Includes Indian, Malay, Indonesian, Filipino, Thai, Arab, Sri Lankan, and Burmese.
2 Participants were asked to predict their weight (in kg) in 3 months, upon program completion. Expected weight loss in % of initial weight was computed as weight loss divided by baseline weight.
Within each gender, there were no differences in age, baseline BMI, income levels, and educational levels between the two arms. We also asked the participants to predict their weight in 3 months. There were no differences in expected weight loss across arms for each gender. There were no other differences in baseline measures. To ensure the robustness of our findings, we report models with and without baseline BMI, age, and educational level as controls.
Attrition is defined as no-shows for the Week 13 or Week 25 weigh-in. Among the males, the Week 13 attrition rates for the Control and IW conditions were 22.35% and 8.14%, respectively (χ 2 = 6.89, df = 1, p = .01). Among the females, the Week 13 attrition rates for these conditions were 18.67% and 17.22%, respectively (χ 2 = 0.11, df = 1, p = 0.74). At Week 25, the overall attrition rate was 15.7%; there were no differences across the two conditions for males (Control: 16.47%, IW: 10.47%; χ 2 = 1.32, df = 1, p = 0.25) and females (Control: 17.33%, IW: 16.56%; χ 2 = 0.03, df = 1, p = 0.86). To handle attrition, we report results using the ITT approach by assuming that the participants who did not show up at the weigh-ins remained at their baseline weights.
Weight loss at Week 13
We first evaluated the effects of incentive on male and female weight loss at Week 13. In Table 2, we show the OLS regression results: columns (1) and (2) show those using the ITT approach, and columns (3) and (4), the PP sample. An OLS regression (model (1), Table 2) yielded a nonsignificant main effect of gender (p = 0.11) and a significant incentive × gender interaction (p < 0.001; MHT-adjusted p = 0.002).
Table 2. Regression results of treatment and gender effects on percentage weight loss at Week 13.

Note. This table presents the OLS regression coefficient estimates, with p-values in parentheses. MHT-adjusted p-values are shown in [brackets]. Dependent variables are Week 13 weight loss [−(Week 13 weight − Week 0 weight)/Week 0 weight] * 100. Control and Female are used as the reference categories. Regression models (1) and (2) are constructed using the ITT approach, whereas models (3) and (4), the PP approach.
*, **, ***indicate statistical significance at the 5%, 1%, and 0.5% levels, respectively.
In Figure 2, the left panel shows the average percentage weight loss at Week 13. For males, the average weight loss percentage was higher in the IW (2.40%) than in the Control (0.87%) (F(1, 468) = 11.79, p < 0.001; MHT-adjusted p = 0.006), indicating that the financial incentive promoted greater weight loss among males. For females, we did not detect any difference in weight loss between the IW arm (1.03%) and the Control arm (1.44%) (p = 0.16). Next, we compared weight loss across gender. In the IW arm, the weight loss percentage was higher for males than for females (2.40% for males vs. 1.03% for females) (F(1, 468) = 12.01, p < 0.001; MHT-adjusted p = 0.002). In the Control arm, there was no difference in weight loss between genders (0.87% for males and 1.44% for females; p = 0.11).
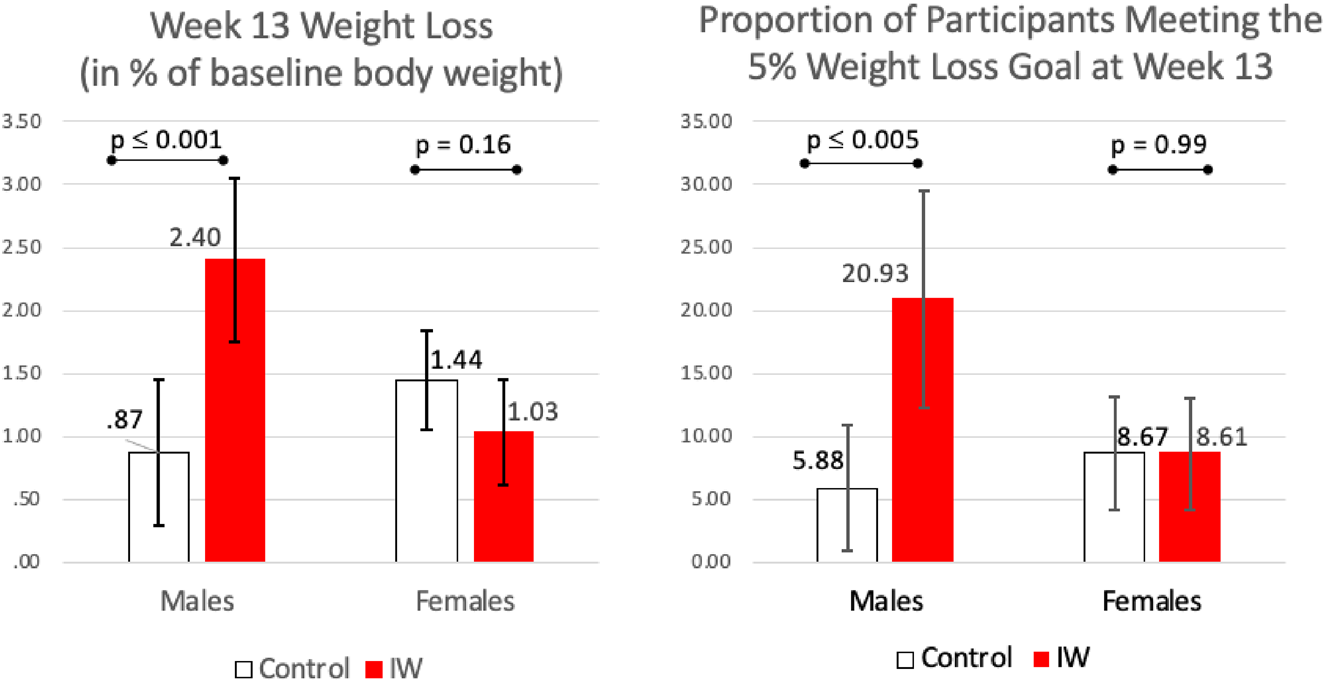
Figure 2. Weight loss at Week 13. Error bars show 95% CI. n = 472.
For robustness, we ran regression models with and without control variables. The odd-numbered columns of Table 2 show the regression results without the control variables, whereas the even-numbered columns show those with the controls (baseline BMI, age, and educational level). Qualitatively, the addition of the control variables has little impact on treatment effect point estimates. Importantly, these results show that the gender difference is robust using both the ITT and PP approaches, and independent of participants’ BMI, age, and education level.
Weight loss target met at Week 13
In Figure 2, the right panel displays the proportion of participants in each arm who met the 5% weight loss target. We fitted a binary logistic regression on success in meeting the target and conducted pairwise comparisons based on the model. We found that 20.93% of males in the IW arm achieved the weight loss target, higher than the 5.88% in the Control; the difference was statistically significant (IW vs. Control: χ 2(1) = 8.72, p < 0.01; MHT-adjusted p = 0.01). For females, there were no differences across the two arms, with 8.67% and 8.61% of participants meeting the target in the Control and IW arms, respectively.
We next examined whether the greater weight loss among males in the IW arm was driven only by those who met the weight loss target. See Figure 3, which shows the proportion of participants by various weight loss outcomes in each arm. The proportion of males who recorded weight loss of 1% or less (this includes some participants who had gained weight) was lower in the IW arm (43.0%) than the Control (63.5%) (diff = 20.5%, χ 2(1) = 7.45, p < 0.01). Moreover, an OLS regression conducted based on the subsample of participants who did not meet the 5% target (148 males, 275 females) using Week 13 weight loss as the dependent variable showed that the incentive × gender interaction was still significant (p < 0.01; MHT-adjusted p = 0.01), and percentage weight loss was still higher among males in the IW (1.09%) than males in the Control (0.44%) arm, F(1, 419) = 4.45, p = 0.04; MHT-adjusted p = 0.07). Hence, the greater weight loss in the IW arm achieved by males was driven not only by participants who met the weight loss target, but also those who did not; in contrast, no such effect of IW was observed in females.

Figure 3. Proportion of participants by weight loss outcomes, Week 13.
Weight loss at Week 25 (post-intervention)
Figure 4 shows weight loss at Week 25. Table 3 displays the OLS regression results. Similar to the results for Week 13 weight loss, the OLS regression (model (1), Table 3) shows a nonsignificant main effect of gender (p = 0.13), with a significant incentive type × gender interaction (p = 0.02; MHT-adjusted p = 0.03). Among males, weight loss in the IW arm (2.53%) remained higher than in the Control arm (0.88%) (F(1, 468) = 9.68; p < 0.005; MHT-adjusted p = 0.01). In other words, for males, the effect of IW sustained for at least 3 months after the incentive was removed. For females, as in Week 13, there remained no differences in weight loss at Week 25 between the IW (1.66%) and Control (1.55%) arms (p = 0.79).

Figure 4. Weight loss at Week 25 by treatment and gender. Error bars show 95% CI. n = 472.
Table 3. Regression results of treatment and gender effects on percentage weight loss at Week 25.

Note. This table presents the OLS regression coefficient estimates, with p-values in parentheses. MHT-adjusted p-values are shown in [brackets]. Dependent variables are Week 25 weight loss [−(Week 25 weight − Week 0 weight)/Week 0 weight] * 100. Control and Female are used as the reference categories. Regression models (1) and (2) are constructed using the ITT approach; models (3) and (4) are constructed using the per-protocol approach.
*, **, *** indicate statistical significance at the 5%, 1%, and 0.5% levels, respectively.
Next, we compared weight loss across gender. In the IW arm, the weight loss percentage was higher for males (2.53%) than for females (1.66%), but the difference was only marginally significant (p = 0.08). In the Control arm, the weight loss percentage in males (0.88%) was not different than that in females (1.55%) (p = 0.23).
General discussion
This article shows that offering overweight males and females a significant financial incentive to meet a weight loss target (IW) works only for males. Our findings refute the commonly held assumption that financial incentives for weight loss work equally well for the two genders. To the best of our knowledge, none of the weight loss incentives currently in use assume a gender difference in response to such incentives. This is perhaps unsurprising since research so far has not provided any evidence of such a gender difference (see our literature review). Notably, however, the lack of evidence is due to the lack of testing, rather than observing supportive evidence of no difference. Our findings fill this gap by showing strong evidence of gender difference and provide implications for both theoretical research and behaviorally informed weight loss policies.
SANS conditions and generalizability of findings
To help policy makers assess the generalizability of our findings, we follow List (Reference List2020)'s recommendation and report the four transparency conditions (SANS conditions) – selection, attrition, naturalness, and scaling.
Selection
We compared our sample to the national population between the age of 40 and 60 in terms of educational level, income, and race (see Appendix C). Our participants were largely comparable to the population in these aspects, which suggests that our recruitment reached a considerably heterogeneous group of people from the general public.
Since we did not have a ‘nonparticipant’ group for comparison in our RCT, we are unable to evaluate the extent of volunteering bias. In general, we expect people who volunteer to participate in a weight loss program to be more motivated to lose weight than those who do not volunteer to join. Hence, we expect our findings to be generalizable to community-level weight loss programs, where participation is usually voluntary.
Attrition
We present an attrition analysis in the section ‘Participant characteristics and attrition’ and participant flow in Figure 5. There could be several reasons for participant no-shows. First, some participants were simply too busy or forgot to attend. Second, participants who had not qualified for the IW reward (i.e., either not in the IW arm or failed to meet the weight loss target) had a lower incentive to attend the weigh-ins than those who did (i.e., those in the IW arm and met the 5% weight loss target). Third, participants who were disengaged from the program might be less motivated to attend the weigh-ins than those who had been actively engaged, despite not having met the weight loss target. The second and third reasons could potentially introduce selection bias to our RCT. The standard approach to handle attrition in weight loss study is to report results using the ITT approach by assuming that the participants who did not show up at the weigh-ins remained at their baseline weights (see, e.g., Volpp et al., Reference Volpp, John, Troxel, Norton, Fassbender and Loewenstein2008). We used the same approach in our data analysis. A supplementary analysis was also conducted using the PP approach, which included only the participants who attended all three weigh-ins.

Figure 5. Diagram of participant flow.
Naturalness
The RCT was a real-world intervention conducted in a highly natural setting. The weight loss program was fully adapted to the context of self-directed weight loss. The weight loss program was hosted online with no mandatory requirements for participants to follow any of the health recommendations. Along the same lines, we refrained from arranging any online meetings with healthcare providers for the participants, nor did we give the participants personalized dietary feedback, even though such features may be effective in promoting weight loss (Tate et al., Reference Tate, Wing and Winett2001; Gold et al., Reference Gold, Burke, Pintauro, Buzzell and Harvey-Berino2007). Therefore, our setting highly resembles large-scale rollouts of self-directed weight loss programs.
Scaling
Our findings show that the ‘voltage drop’ problem could happen for large-scale weight loss programs that employ financial incentives to motivate weight loss – if financial incentives for weight loss work only for one gender, we would expect a significant voltage drop when incentives for weight loss are offered to the general population.
Potential explanations for the gender difference in financial incentives for weight loss
Why were our male participants more driven by IW to lose weight? Our RCT did not allow us to identify the mechanism behind the gender difference observed; nevertheless, we discuss some possible explanations for our findings.
One possibility would be that the male participants were more driven by IW because they had less income than the female participants. However, this was unlikely to be the case because in our study, the male participants earned higher income than the female participants.
Another possibility would be that the female participants were less responsive to financial incentives for weight loss, because there is a stronger pairing between weight management and social rewards in females. Indeed, there is an extensive literature that documents differences between men and women in the type of rewards they seek from weight management.
In many societies, there is a stronger emphasis on women's physical appearance than on men's. Women are more vulnerable to weight discrimination than men: While men do not experience notable weight discrimination until their BMI reaches 35, women feel discriminated at the much lower BMI level of 27 (Puhl et al., Reference Puhl, Andreyeva and Brownell2008). Judgment of physical appearance comes along with implications to romantic relationships, popularity, and employment, making the social implications of physical appearance a lot more significant for women than men (Feingold, Reference Feingold1990, Reference Feingold1992). Finally, in a weight loss program, women were more likely than men to report improving personal esteem (e.g., improve appearance, feel better about oneself) as a motivator than men (Crane et al., Reference Crane, Jeffery and Sherwood2017). Overall, these streams of research suggest that social rewards may have a stronger motivational significance for women than men in the domain of weight management, so that the financial incentives for weight loss may be relatively less attractive to the former. In this regard, women may benefit more from programs that motivate weight loss through social incentives (e.g., face-to-face guided programs, group weight loss programs).
Implications for behaviorally informed policies
Recently, behavioral scientists and economists have raised their attention to the role of demographics for their critical relevance to the success of scaled implementation of behavioral interventions. Specifically, the assumption that an intervention would work equally well for all demographic groups is a major cause of ‘voltage drop’ – the phenomenon that the effect size of an intervention drops significantly relative to that reported in the original research when it is implemented at a large scale in the society (Banerjee et al., Reference Banerjee, Barnhardt and Duflo2018; Al-Ubaydli et al., Reference Al-Ubaydli, Lee, List, Mackevicius and Suskind2019a, Reference Al-Ubaydli, List and Suskind2019b; Ho et al., Reference Ho, Leong and Yeung2021). The same could occur for large-scale weight loss programs that use financial incentives to motivate weight loss, because financial incentives for weight loss work only for one gender. Future research could experiment combining financial incentives with other gender-specific behavioral interventions (e.g., online discussion forum, which works well to promote women weight loss; Johnson & Wardle, Reference Johnson and Wardle2011) to produce more promising and gender-balanced overall results. In this respect, behavioral scientists who run commercial weight loss programs would be in a favorable position to analyze their data to examine gender difference in their incentive programs, and customize weight loss options to achieve greater effectiveness. Notably, our findings do not suggest a policy that offers money for men to lose weight but not to women; rather, they suggest that policy makers may consider offering different incentive options and letting the respondents pick the one that will best motivate them.
Potential limitations
We conclude with three limitations. First, we provided a cash incentive of $150 for achieving 5% weight loss. People's responses to the $150 weight loss incentive depend on how much they value the benefit of an incremental $150 cash reward, in addition to the benefit of losing weight. For example, severely overweight people may not require the extra $150 for them to weight loss, so we may observe no effect if the $150 were offered to them. For a similar reason, it is also unclear if one would obtain the same pattern of results with an incentive of a substantially different monetary value. Second, we had only one post-intervention weigh-in (12 weeks after the end of the program) and are thus unable to conclude if the greater weight loss among males in the IW arm persisted beyond the 24-week timeframe. Also, we did not draw blood for analysis, so we were unable to evaluate any health benefits beyond weight loss (e.g., reducing cardiometabolic risk). Third, since RCT relied on participants’ voluntary signup, there was a potential for volunteer bias in our recruitment. Nevertheless, considering that most community-level weight loss programs are organized on a volunteer basis, we would expect our findings to be generalizable to such contexts.
Funding
This work was supported by the Global Asia Institute, National University of Singapore (NIHA-2013-1-001) and the Singapore National Research Foundation's Returning Singaporean Scientists Scheme (grant no. NRFRSS2014-001). The funding sources had no involvement in the conduct of the research or preparation of the manuscript.
Conflict of interest
None.
Appendix A. Recruitment advertisement

Appendix B. Diet and exercise: measurements and results
Self-reporting of diet and exercise
Each week, the website prompted participants to report the number of minutes they exercised over the previous week. These self-reports were voluntary; a total of 203 participants reported their exercise for each of the 12 weeks, while 18 participants did not provide any reports at all. We reported analysis results of exercise in Week 1 and average weekly exercise for the intervention period in this Appendix (see below). Average weekly exercise was computed for a participant only when three or more self-reports were logged. Because no baseline measure for exercise duration was collected, an ITT analysis of the exercise duration data was not possible.
Diet quality was also self-reported. During the first two weigh-ins, participants were given a food intake questionnaire that asked about portion sizes and frequency of consumption of 25 different food items over the previous week. A DASH (Dietary Approaches to Stop Hypertension) score was then computed for each participant using the DASH diet index developed by Fung et al. (Reference Fung, Chiuve, McCullough, Rexrode, Logroscino and Hu2008). The score is computed based on consumption of items in seven food groups: whole grains, vegetables, fruit, low-fat dairy, nuts and legumes, red and processed meat, and sugar-sweetened beverages. The scoring method is based on quintiles. We first divided participants into quintiles according to their intake ranking for each of the components. For beneficial foods, 5 points are given for intake in the highest quintile, 4 for intake in the fourth quintile, etc. For unhealthy items, 5 points are given for intake in the lowest quintile, 4 points for intake the second quintile, etc. The set of quintile cut-offs identified at Week 0 was used to compute component scores for food consumption measured at Week 13. Analysis of the DASH score was conducted using the ITT approach and reported below; participants with missing measurements were treated as having their diet reverted to baseline.
Findings on exercise duration and diet
The table below (upper panel) shows exercise duration (in minutes) at Week 1 of the intervention and average exercise duration across the 12-week intervention. For males, exercise duration in Week 1 was higher in the IW arm (M = 223.84) than in the Control (M = 105.70) (F(1, 344) = 7.72, p < 0.01). The average weekly exercise duration was also higher in the IW (M = 204.05) than in the Control (M = 141.63) (F(1, 425) = 3.74, p = 0.05). A bootstrapping mediation analysis using 5000 samples (Hayes & Preacher, Reference Hayes and Preacher2014) revealed that the 12-week average exercise duration partially mediated the relationship between the IW arm and weight loss (95% CI of indirect effect: [0.0002, 0.0059]). In other words, one reason why there was greater weight loss in the IW arm was because males in that arm exercised more than those in the Control arm. For females, there were no differences in exercise duration across the two arms across the intervention period.
The bottom panel of the table shows the Week 0 and Week 13 diet quality scores, and their differences across those weeks. The diet quality scores reported by participants at Week 13 were higher for male participants in both the IW and Control arms, and for females in the Control arm. However, females in the IW arm did not report higher diet quality scores, and there were no gender differences across arms. These results suggest that the positive effect of monetary incentives on weight loss for males cannot be attributed to dietary improvements.
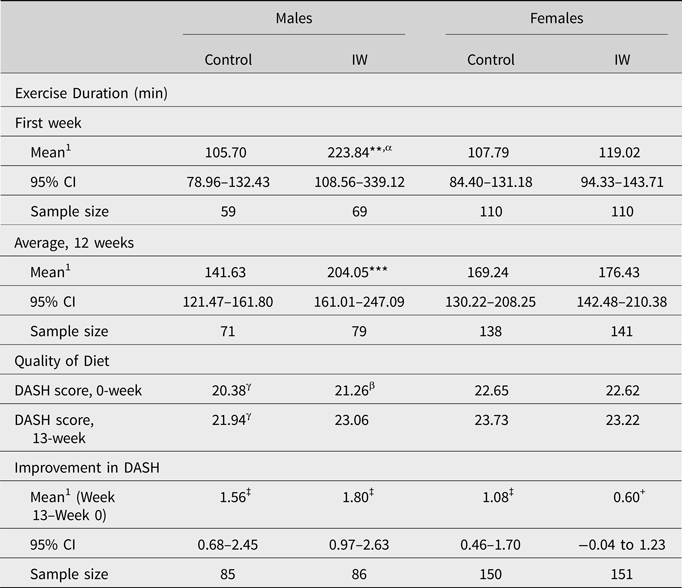
Appendix C. Key demographics of national sample age 40–59, Singapore
