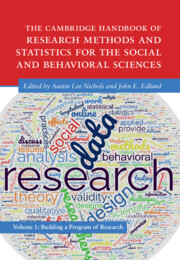
Book contents
- The Cambridge Handbook of Research Methods and Statistics for the Social and Behavioral Sciences
- Cambridge Handbooks in Psychology
- The Cambridge Handbook of Research Methods and Statistics for the Social and Behavioral Sciences
- Copyright page
- Dedication
- Contents
- Figures
- Tables
- Contributors
- Preface
- Part I From Idea to Reality: The Basics of Research
- Part II The Building Blocks of a Study
- Part III Data Collection
- Part IV Statistical Approaches
- 21 Data Cleaning
- 22 Descriptive and Inferential Statistics
- 23 Testing Theories with Bayes Factors
- 24 Introduction to Exploratory Factor Analysis: An Applied Approach
- 25 Structural Equation Modeling
- 26 Multilevel Modeling
- 27 Meta-Analysis
- 28 Qualitative Analysis
- Part V Tips for a Successful Research Career
- Index
- References
26 - Multilevel Modeling
from Part IV - Statistical Approaches
Published online by Cambridge University Press: 25 May 2023
- The Cambridge Handbook of Research Methods and Statistics for the Social and Behavioral Sciences
- Cambridge Handbooks in Psychology
- The Cambridge Handbook of Research Methods and Statistics for the Social and Behavioral Sciences
- Copyright page
- Dedication
- Contents
- Figures
- Tables
- Contributors
- Preface
- Part I From Idea to Reality: The Basics of Research
- Part II The Building Blocks of a Study
- Part III Data Collection
- Part IV Statistical Approaches
- 21 Data Cleaning
- 22 Descriptive and Inferential Statistics
- 23 Testing Theories with Bayes Factors
- 24 Introduction to Exploratory Factor Analysis: An Applied Approach
- 25 Structural Equation Modeling
- 26 Multilevel Modeling
- 27 Meta-Analysis
- 28 Qualitative Analysis
- Part V Tips for a Successful Research Career
- Index
- References
Summary
This chapter provides a brief introduction to multilevel models, specifically organizational models, and should be accessible to researchers who are familiar with ordinary least-squares (OLS) regression (i.e., multiple regression models). OLS regression assumes independence of observations; however, the responses of people clustered within organizational units (e.g., schools, classrooms, hospitals, companies) are likely to exhibit some degree of relatedness. In such scenarios, violating the assumption of independence produces incorrect standard errors that are smaller than they should be – multilevel modeling can alleviate this concern. However, the advantages of multilevel modeling are not purely statistical. Substantively, researchers may seek to understand the degree to which people from the same cluster are similar to each other and identify variables that predict variability within and across clusters. Multilevel analyses allow us to exploit the information in clustered samples and partition variance in the outcome variable into between-cluster and within-cluster variability. We can also use predictors at both the individual (level 1) and group (level 2) levels to explain this between- and within-cluster outcome variance.
Keywords
- Type
- Chapter
- Information
- The Cambridge Handbook of Research Methods and Statistics for the Social and Behavioral SciencesVolume 1: Building a Program of Research, pp. 559 - 582Publisher: Cambridge University PressPrint publication year: 2023