References
Published online by Cambridge University Press: 05 August 2014
Summary
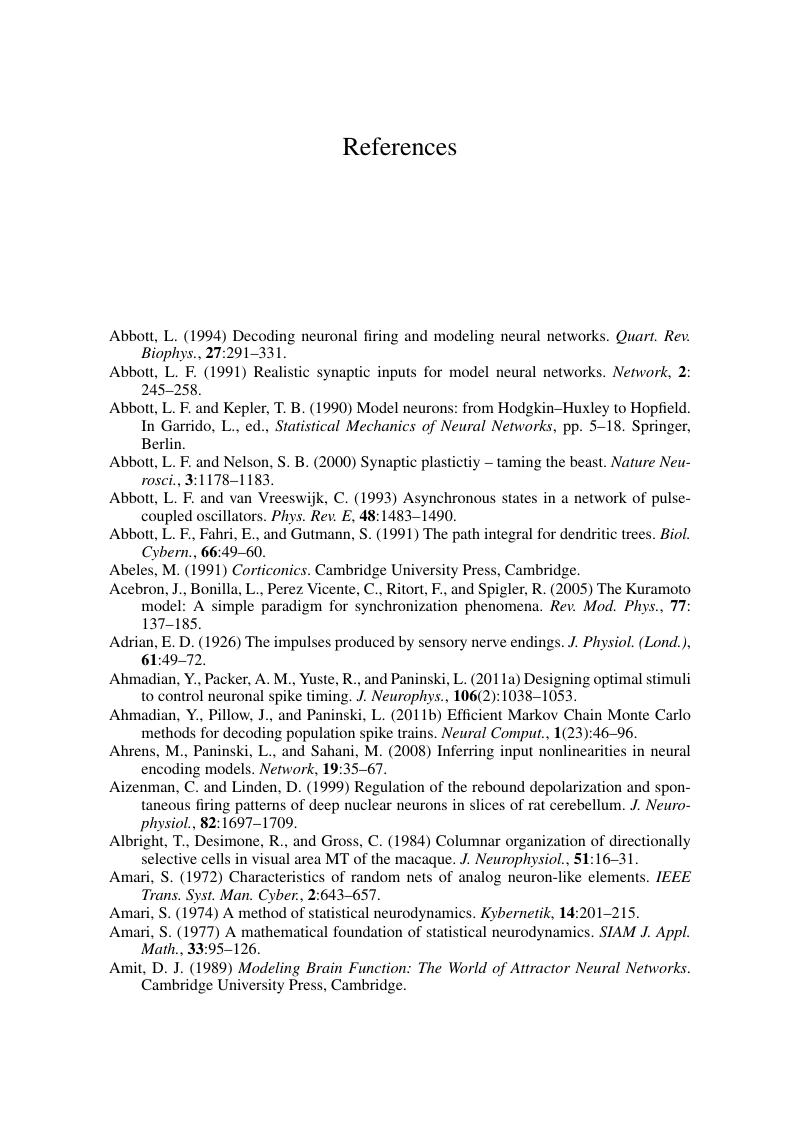
- Type
- Chapter
- Information
- Neuronal DynamicsFrom Single Neurons to Networks and Models of Cognition, pp. 547 - 572Publisher: Cambridge University PressPrint publication year: 2014