Book contents
- Frontmatter
- Contents
- Preface
- Acknowledgements
- 1 Introduction
- 2 Study design
- 3 Continuous outcome variables
- 4 Continuous outcome variables – relationships with other variables
- 5 The modeling of time
- 6 Other possibilities for modeling longitudinal data
- 7 Dichotomous outcome variables
- 8 Categorical and “count” outcome variables
- 9 Analysis of experimental studies
- 10 Missing data in longitudinal studies
- 11 Sample size calculations
- 12 Software for longitudinal data analysis
- 13 One step further
- References
- Index
- References
References
Published online by Cambridge University Press: 05 May 2013
- Frontmatter
- Contents
- Preface
- Acknowledgements
- 1 Introduction
- 2 Study design
- 3 Continuous outcome variables
- 4 Continuous outcome variables – relationships with other variables
- 5 The modeling of time
- 6 Other possibilities for modeling longitudinal data
- 7 Dichotomous outcome variables
- 8 Categorical and “count” outcome variables
- 9 Analysis of experimental studies
- 10 Missing data in longitudinal studies
- 11 Sample size calculations
- 12 Software for longitudinal data analysis
- 13 One step further
- References
- Index
- References
Summary
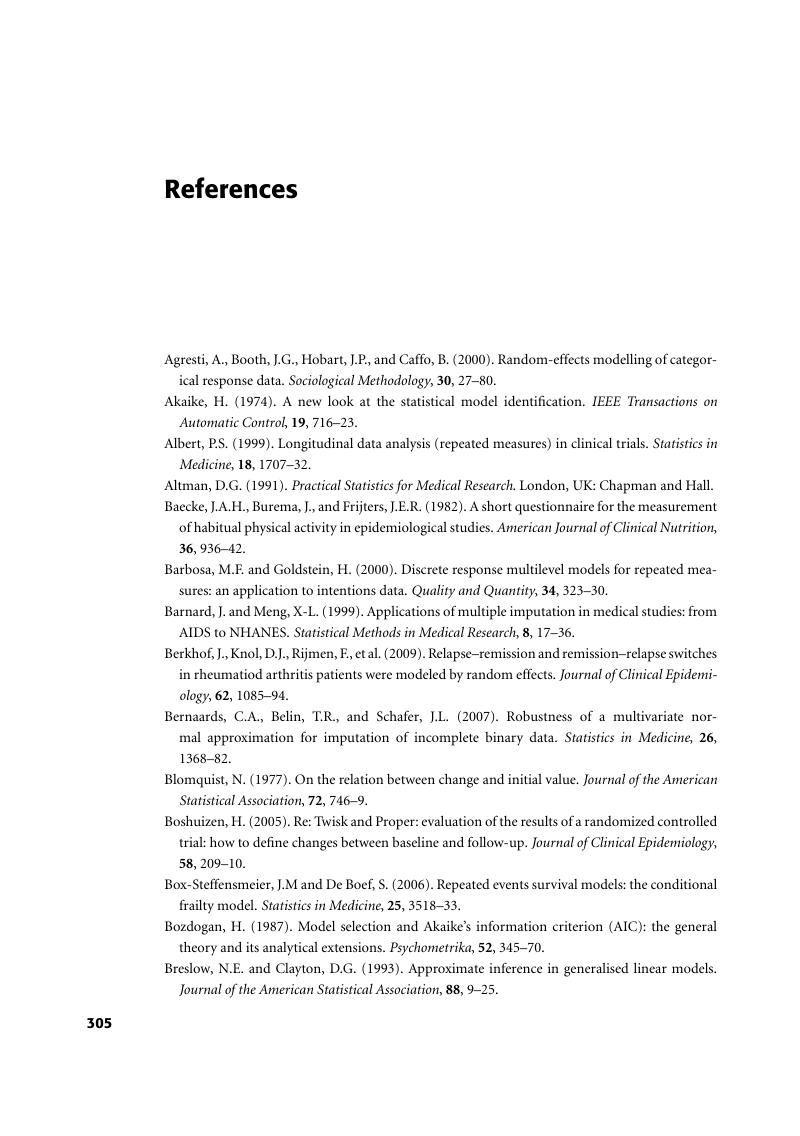
- Type
- Chapter
- Information
- Applied Longitudinal Data Analysis for EpidemiologyA Practical Guide, pp. 305 - 315Publisher: Cambridge University PressPrint publication year: 2013