Unipolar depression affects more than 151 million people worldwide, and it is expected to become the world’s second most common disease, after CVD, by 2020( 1 ). Results from the Global Burden of Disease Study indicate that mental health and substance use disorders are the leading global cause for all non-fatal conditions contributing to the burden of disease, as measured by years lived with disability, with depressive disorders contributing the most to this burden of disease( Reference Whiteford, Degenhardt and Rehm 2 ). Women are more likely to be diagnosed with depression compared with men( Reference Ford and Erlinger 3 , Reference Albert 4 ). Metabolic and inflammatory processes, such as reduced insulin sensitivity, elevations in plasma homocysteine levels, increased production of pro-inflammatory cytokines, such as C-reactive protein, increased levels of stress hormones and endothelial dysfunction, appear to be the major factors responsible for depression( Reference Hood, Lawrence and Anderson 5 – Reference Halaris 8 ).
Various dietary components are known to exert different effects on inflammation( Reference de Mello, Schwab and Kolehmainen 9 – Reference Luciano, Mottus and Starr 11 ). A prudent dietary pattern high in fish, yogurt, pulses, rice, fruits, vegetables, pasta and wine has been shown to be associated with lower concentrations of intermediary inflammatory markers( Reference Wood, Strachan and Thies 12 ).
Various studies have been conducted to evaluate dietary exposures in relation to depression( Reference Guo, Park and Freedman 13 – Reference Mihrshahi, Dobson and Mishra 17 ). In general, prospective cohort studies have shown that dietary patterns rich in components such as fruits, vegetables, olive oil and legumes may be protective against depression( Reference Rienks, Dobson and Mishra 18 , Reference Sanchez-Villegas, Delgado-Rodriguez and Alonso 19 ). Previous results from the Australian Longitudinal Study on Women’s Health (ALSWH) study showed that increased consumption of fruits reduced both the prevalence and the incidence of depressive symptoms, although no association was observed with vegetable intake( Reference Mihrshahi, Dobson and Mishra 17 ). In contrast, increased risk has been observed with diets rich in SFA, n-6 fatty acids and refined carbohydrates( Reference Akbaraly, Brunner and Ferrie 20 , Reference Le Port, Gueguen and Kesse-Guyot 21 ). However, a few studies have explored the association between inflammatory potential of diet and depression.
A literature-derived, population-based dietary inflammatory index (DII) was developed to assess the inflammatory potential of an individual’s diet( Reference Shivappa, Steck and Hurley 22 ). Previously, higher DII scores have been shown to be associated with increased risk of depression in a Spanish study( Reference Sanchez-Villegas, Ruiz-Canela and de la Fuente-Arrillaga 23 ). Our aim in this study was to assess the association between the inflammatory potential of dietary patterns of middle-aged Australian women measured through DII scores and the risk of depression.
Methods
Study design and participants
ALSWH is an ongoing, population-based cohort study examining factors associated with health and well-being of Australian women. Full details on study design, recruitment methods and response rates have been published previously( Reference Brown, Bryson and Byles 24 , Reference Lee, Dobson and Brown 25 ). Women were randomly selected from the National Medicare Health Insurance database, including all Australian citizens and permanent residents. Women from rural and remote areas were intentionally oversampled. Informed consent was obtained from all participants at each survey, with ethics clearance obtained from the Human Research Ethics Committees of the University of Newcastle and the University of Queensland, Australia.
For this study, we used data from the middle-aged cohort of women born between 1946 and 1951. A total of 13 715 women aged 45–50 years responded to the first survey in 1996. This sample was found to be broadly representative of the general Australian population of women of similar age based on the national census( Reference Brown, Bryson and Byles 24 ). Self-administered questionnaires were sent to participants every 2–3 years, until 2013 (survey 7). Response rates for surveys 2, 3, 4, 5, 6 and 7 were 90·0 % (n 12 338), 81·8 % (n 11 221), 79·5 % (n 10 905), 77·6 % (n 10 638), 73·0 % (n 10 011) and 66·7 % (n 9151) of baseline, respectively. Information on dietary intake was first collected at survey 3 in 2001, when women were aged 50–55 years; this survey was therefore used as baseline for this study. Women were excluded if they reported a history of depressive symptoms (Center for Epidemiologic Studies Depression (CESD-10) score of ≥10) from survey 1 (1996) until survey 3 (2001) (n 3459), had missing data on baseline dietary intake or depressive symptoms during follow-up (n 695) or had missing data on relevant confounders (n 634). Our analyses included 6438 women (online Supplementary Fig. S1).
Dietary assessment
Diet was assessed using the Dietary Questionnaire for Epidemiological Studies (DQES) version 2. The development of this 101-item FFQ has been described elsewhere( Reference Ireland, Jolley and Giles 26 ). The FFQ was validated against 7-d weighed food records in sixty-three women with a mean age of 33·3 (sd 9·5) years. Mean intakes from the weighed food records and FFQ were within ±20 % for twenty-one of twenty-seven nutrients( Reference Hodge, Patterson and Brown 27 ). Energy-adjusted correlation coefficients for nutrient intake ranged between 0·28 for vitamin A and 0·78 for carbohydrates, indicating that the FFQ performed reasonably well for assessing habitual intake. Participants were asked to report their usual frequency of consumption of seventy-four food items and six alcoholic beverages over the previous 12 months using a ten-point scale ranging from ‘never’ to ‘three or more times per day’. Portion size photographs were used to adjust the serving sizes for vegetables, meat and casseroles. The FFQ included twenty-one additional items on the number of servings of milk, bread, sugar and eggs and the type of milk, bread, fat spreads and cheese consumed. Consumption of 101 food items (g/d) and nutrient intakes were computed from the national government food composition database of Australian foods – the NUTTAB95( Reference Lewis and Hunt 28 ).
The dietary inflammatory index
The development and validation of the DII are described in detail elsewhere( Reference Shivappa, Steck and Hurley 22 , Reference Shivappa, Steck and Hurley 29 ). Developing the DII involved reviewing and scoring nearly 2000 scientific articles representing cell culture and laboratory animal experiments, as well as a variety of human studies on diet and six inflammatory markers (i.e., C-reactive protein, IL-1b, IL-4, IL-6, IL-10, TNF-α). One of three possible values was assigned to each article on the basis of the effect of the particular food or constituent on inflammation: +1 if pro-inflammatory, −1 if anti-inflammatory and 0 if it produced no change in the inflammatory marker. In addition, these articles were weighed on the basis of the study design, with experimental cell studies receiving the lowest weight of 3 and human trials the highest weight of 10( Reference Shivappa, Steck and Hurley 22 , Reference Shivappa, Steck and Hurley 29 ). These procedures also entailed the creation of a world standard database that involved obtaining eleven data sets from around the world (Japan, Korea, USA, Canada, Mexico, Taiwan, Australia, New Zealand, Bahrain, Denmark and India) to which individuals’ intakes of forty-five food parameters (consisting of nutrients, spices and whole foods) on which the DII is based could then be compared.
The steps involved in computing DII scores are described in Fig. 1. To summarise, FFQ-derived dietary data were used to calculate DII scores for all participants. First, they were linked to the world database described previously( Reference Shivappa, Steck and Hurley 22 ). These then became the multipliers to express an individual’s exposure relative to the ‘standard global mean’ as a z-score, which was computed by subtracting the ‘standard global mean’ from the amount reported and dividing this value by the ‘global standard deviation’. To minimise the effect of ‘right skewing’, this value was then converted to a centred percentile score( Reference Shivappa, Steck and Hurley 22 ) obtained by doubling and subtracting 1. Food parameter-specific DII scores were obtained by multiplying this score( Reference Shivappa, Steck and Hurley 22 ). All of the food parameter-specific DII scores were then summed to create the overall DII score for each participant in the study.
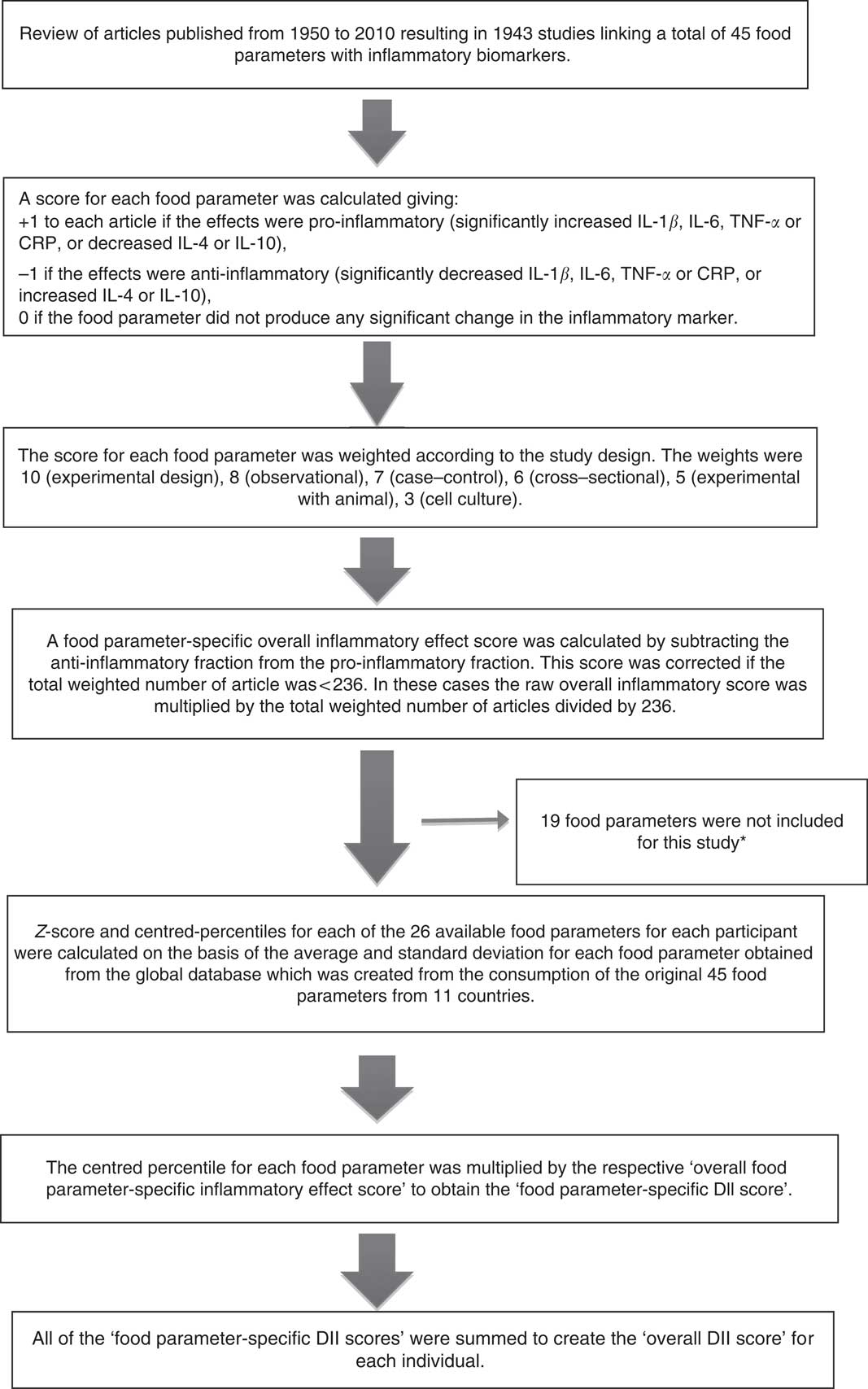
Fig. 1 Sequence of steps in creating the dietary inflammatory index (DII) in the Australian Longitudinal Study on Women’s Health. * The missing food items were anthocyanin, eugenol, flavan-3-ols, flavones, flavonols, flavonones, isoflavones, caffeine, ginger, saffron, turmeric, vitamin D, pepper, thyme and oregano, rosemary and Se. CRP, C-reactive protein.
For the current study, data on twenty-six of the forty-five DII food parameters could be derived from the FFQ, and were thus used for DII calculation. These included pro-inflammatory components (energy, carbohydrate, protein, fat, SFA, Fe, cholesterol) and anti-inflammatory components (alcohol, fibre, MUFA, PUFA, n-3, n-6, niacin, thiamin, riboflavin, Mg, Zn, vitamin A, vitamin C, vitamin E, folic acid, β-carotene, garlic and onions). The DII score can range from the maximum pro-inflammatory score of +7·98 to the minimum anti-inflammatory score of −8·87( Reference Shivappa, Steck and Hurley 22 ). Positive DII scores indicate a pro-inflammatory diet and negative scores an anti-inflammatory diet.
The DII method has been validated by comparing the DII scores with various inflammatory markers( Reference Tabung, Steck and Zhang 30 – Reference Wood, Shivappa and Berthon 32 ). In addition, the DII score has been shown to be associated with glucose intolerance and dyslipidaemia components of the metabolic syndrome( Reference Alkerwi, Sauvageot and Malan 33 , Reference Wirth, Burch and Shivappa 34 ), anthropometric measurements in Spain( Reference Ruiz-Canela, Zazpe and Shivappa 35 ), asthma in Australia( Reference Wood, Shivappa and Berthon 32 ), bone mineral density among postmenopausal women in Iran( Reference Ge, Rudolph and Shivappa 36 ), two colorectal cancer case–control studies in Spain and Italy( Reference Zamora-Ros, Shivappa and Steck 37 , Reference Shivappa, Zucchetto and Montella 38 ) and in a cohort study of women in the USA( Reference Zamora-Ros, Shivappa and Steck 37 , Reference Shivappa, Prizment and Blair 39 ), and pancreatic and prostate cancers in Italy( Reference Shivappa, Bosetti and Zucchetto 40 , Reference Shivappa, Bosetti and Zucchetto 41 ).
Depressive symptoms
Depressive symptoms were measured using the CESD-10 scale. This scale is constructed on the basis of self-reported responses to ten questions on whether, over the past week, women experienced symptoms associated with depression, such as worrying, sleeping difficulty and difficulty relaxing. The CESD-10 has a good test–re-test reliability and predictive validity when compared with the original twenty-item version( Reference Andresen, Malmgren and Carter 42 ), and has high sensitivity (97–100 %) and specificity (84–93 %) for the screening of major depression in middle-aged and older adults( Reference Irwin, Artin and Oxman 43 ). Responses to the ten questions were reported using a four-point Likert scale ranging from ‘rarely’ (scored 0) to ‘most of the time’ (scored 3). Possible scores range from 0 to 30, with higher scores indicating more depressive symptoms. A CESD-10 score of ≥10 was used to define the presence of depressive symptoms. Validation of the CESD-10 against the twenty-item version using a cut-off of ≥10 has been shown to minimise false-positive results with little loss of sensitivity( Reference Andresen, Malmgren and Carter 42 ). Incident depression was defined as new onset of depressive symptoms between survey 4 (2004) and survey 7 (2013).
Assessment of covariates
Self-reported information was collected at each survey on a range of socio-demographic variables, symptoms, lifestyle factors and health behaviours. Responses were categorised for the highest qualification obtained as ‘no formal’, ‘(higher) school certificate’, ‘trade/diploma’ or ‘university/higher degree’; for marital status as ‘single/never married’, ‘married or de facto’ or ‘separated/divorced/widowed’; for night sweats as ‘no’ (never) or ‘yes’ (rarely/sometimes/often); for smoking as ‘non-smoker’, ‘ex-smoker’ or ‘current smoker’; and for experience of stressful life events or diagnosis or treatment of depression since the previous survey as ‘no’ or ‘yes’.
BMI was computed as self-reported weight (kg) divided by the square of self-reported height (m) and categorised as ‘normal weight’ (BMI<25 kg/m2), ‘overweight’ (25≤BMI<30 kg/m2) or ‘obese’ (BMI≥30 kg/m2). As only a limited proportion of women (1·5 %) were classified as ‘underweight’ (BMI<18·5 kg/m2), they were combined with the ‘normal weight’ group. Self-reported weight and height were validated in a subsample of 159 women (age 54–59 years). Both self-reported weight and height tended to be underestimated compared with measured values, with a mean difference of 0·95 (95 % CI 0·44, 1·47) kg and 0·67 (95 % CI 0·26, 1·08) cm, respectively. Agreement between BMI categories derived from self-reported and measured data was 84 %( Reference Burton, Brown and Dobson 44 ).
Physical activity scores were derived from validated questions on frequency and duration of walking (for recreation or transport) and from questions on moderate- and vigorous-intensity activity in the last week and categorised as ‘sedentary/low’ (<600 metabolic equivalent (MET)-min/week), ‘moderate’ (600≤MET<1200 min/week) or ‘high’ (≥1200 MET-min/week)( Reference Brown, Burton and Marshall 45 ). Menopause status was determined using questions on hysterectomy, oophorectomy, hormone therapy and menstrual pattern and categorised as ‘hysterectomy and/or oophorectomy’, ‘hormone therapy use’, ‘premenopausal’, ‘perimenopausal’ or ‘postmenopausal’( Reference Berecki-Gisolf, Begum and Dobson 46 ).
Statistical analysis
Baseline characteristics were described for women according to reporting of depressive symptoms at least once during follow-up and according to quartiles of the DII, and were compared using t tests, ANOVA or χ 2 tests. All descriptive statistics were weighted by area of residence to account for oversampling of women from rural and remote areas.
Generalised estimating equations were used to examine the prospective associations between the DII score (computed based on survey 3 data) and the incidence of depressive symptoms (survey 4–7). Log-binomial models or log-Poisson models (when log-binomial models did not converge) were used to estimate relative risks (RR) and 95 % CI. Models were adjusted for the time-varying confounders described above (survey 3–6). Model 1 adjusted for total energy intake, highest qualification completed, marital status, menopause status, hot flushes and experience of major personal illness or injury; model 2 additionally adjusted for lifestyle factors: smoking status, physical activity and BMI; and model 3 adjusted for depression diagnosis or treatment.
Factors including new onset of hot flushes, occupation, time spent socialising, performing active (walking or exercise) and passive (watching TV, reading, relaxing) leisure activities, and stressful life events including break-up of relationships or divorce and death of a close family member or friend were considered as potential confounders but not included in the final models as adjustments did not substantially change the effect estimates.
To test the robustness of our findings, additional analyses were performed. First, characteristics were compared with women included in the analysis and excluded because of missing data on confounders. Second, a multiple imputation analysis was performed to assess the influence of participant exclusions that resulted from missing data( Reference Berglund 47 ). Third, in addition to examining the association between DII score and risk of depression in women with no history of depression before survey 3 based on a CESD-10 score of ≥10, we examined the association in women with no history of doctor-diagnosed or treated depression. All statistical tests were conducted using a two-sided 5 % level of significance.
Results
The mean DII score in this study was 1·11 (sd 1·54), which indicated a slightly pro-inflammatory diet. Table 1 describes the distribution of several characteristics according to depression status. Women who developed depression during follow-up were more likely to have lower education, be obese, be sedentary/have low physical activity, be a current smoker, to have hot flushes, to have experienced major personal illness or injury and be less likely to be postmenopausal. Table 2 describes the distribution of characteristics across quartiles of DII scores. Women in the most pro-inflammatory group (quartile 4) were more likely to have lower education, be separated/divorced/widowed, be sedentary/have low physical activity and be current smokers.
Table 1 Baseline characteristics of middle-aged women according to reporting of depressive symptoms at least once during 12 years of follow-up, Australian Longitudinal Study on Women’s Health, 2001 to 2013Footnote * (Mean value and standard deviation; numbers and percentages, weighted by area of residence; n 6438)

MET, total metabolic equivalent.
* Numbers may vary due to missing baseline values.
† P-values from t test or χ 2 test. All statistical tests were conducted using a two-sided 5 % level of significance.
Table 2 Baseline characteristics of middle-aged women according to quartiles of the dietary inflammatory index (DII)Footnote *, Australian Longitudinal Study on Women’s Health, 2001 to 2013 (Mean value and standard deviation; n"umbers and percentages, weighted by area of residence; n 6438)Footnote †

MET, total metabolic equivalent.
* Higher DII indicates a more pro-inflammatory diet, and lower DII indicates a more anti-inflammatory diet.
† Numbers may vary due to missing baseline values.
‡ P-values from ANOVA or χ 2 test. All statistical tests were conducted using a two-sided 5 % level of significance.
The distribution of consumption of various nutrients and food items per day across quartiles of DII is shown in the online Supplementary Table S1. Most of the anti-inflammatory dietary components such as PUFA, dietary fibre, Zn, fruits, vegetables, fish and high-fibre grain foods decreased linearly across quartiles of DII. Similar trends were observed for intakes of total energy, total meat, and red and processed meat. Pro-inflammatory components whose intakes were higher among women in the fourth quartile were SFA, cholesterol, Ca, high-fat dairy products and added sugar.
Compared with the most pro-inflammatory group (4th quartile), the risk of developing depression was 26 % lower in the most anti-inflammatory group (1st quartile) (RRDII quartile 1 v. 4=0·74; 95 % CI 0·61, 0·89, P trend=0·001) for model 1, 21 % (RRquartile 1 v. 4=0·79; 95 % CI 0·66, 0·95, P trend=0·03) for model 2 and 19 % (RRquartile 1 v. 4=0·81; 95 % CI 0·69, 0·96, P trend=0·03) for model 3 (Table 3). The difference in the incidence of depression over 12 years between quartile 4 and quartile 1 of DII was 1·6 %.
Table 3 Relative risks for associations between the dietary inflammatory index and incidence of depressive symptoms, Australian Longitudinal Study on Women’s Health, 2001 to 2013 (Numbers and percentages; relative risks and 95 % confidence intervals; n 6438)

* Adjusted for total energy intake, highest qualification completed, marital status, menopause status, night sweats and major personal illness or injury.
† Additionally adjusted for lifestyle factors: smoking, physical activity and BMI.
‡ Additionally adjusted for depression diagnosis or treatment.
Compared with women who were included in the analysis (n 6438), the 10 % of women who were excluded because of missing confounding data (n 634) were more likely to have a pro-inflammatory diet, lower education, be separated/divorced/widowed, be postmenopausal (surgical or natural), be obese, smoke tobacco, be sedentary or have low physical activity levels and have a history of major illness or injury. Incidence of depressive symptoms during follow-up did not differ for women who were included in the analysis compared with women who were excluded because of missing data (online Supplementary Table S2). Conclusions on the association between DII and depression remained similar in analyses where missing confounding data were imputed (online Supplementary Table S3). Excluding women with a history of doctor-diagnosed or treated depression instead of a history of depressive symptoms based on a CESD-10 score of ≥10 did not alter our findings (data not shown).
Discussion
The present study, the largest prospective investigation on inflammatory potential of diet and depression to date in an Australian population, shows an inverse association between an anti-inflammatory dietary pattern – characterised by consumption of nutrients such as n-3 and n-6 PUFA, dietary fibre and food groups such as fruits, vegetables, meat, red meat, fish and high-fibre grain foods – and a lower risk of depression in a population-based study of middle-aged women.
In our study, most of the nutrient and food group distributions across quartiles of DII were as expected, but the distributions of total meat, processed meat and red meat were contrary to our expectation (levels increased across quartiles of DII). However, it should be noted that red meat is one among several other food items that influence inflammation. There are other food items such as vegetables, fruits and fish that exert strong anti-inflammatory effects per unit exposure and their distributions are as expected. In addition, red meat is rich in nutrients including Zn, vitamin B12 and n-6 fatty acids, all of which have an anti-inflammatory score with DII( Reference McAfee, McSorley and Cuskelly 48 ). As the DII takes into account diet as a whole, and red meat eaters also consume vegetable components, spices and other anti-inflammatory foods, overall, their diet was more anti-inflammatory.
These results are consistent with a report from the Nurse’s Health Study, where researchers observed a strong association between an inflammatory dietary pattern and depression( Reference Lucas, Chocano-Bedoya and Shulze 16 ). Unlike the DII, which is empirically linked to findings in the literature, the inflammatory dietary pattern in that study was identified using reduced-rank regression, which in turn was related to plasma levels of inflammatory markers. To date, there has been only one other study, conducted in Spain, which has examined the association between the inflammatory potential of diet as determined by DII scores and depression. In that study, the hazards ratio for participants in the highest quintile of the DII (strongly pro-inflammatory) was 1·47 (95 % CI: 1·17, 1·85) compared with those in the lowest quintile, with a significant dose–response relationship (P for trend=0·01)( Reference Sanchez-Villegas, Ruiz-Canela and de la Fuente-Arrillaga 23 ).
A majority of our participants were well educated, with a high percentage of women in all DII quartiles having at least high school certificate education. It is also evident from the distribution of demographic data across quartiles of DII that women in quartile 4 were more likely to be separated/divorced/widowed, report low levels of physical activity and currently smoking. We also observed decreased energy intake among women in quartile 4 compared with women in quartile 1. This is consistent with the fact that observations across all of the many studies we have conducted to date using the DII reveal two countervailing effects( Reference Shivappa, Prizment and Blair 39 , Reference Ruiz-Canela, Zazpe and Shivappa 49 , Reference Shivappa, Harris and Wolk 50 ). The first is a tendency to eat more of everything as one increases energy intake; this results in a positive correlation between energy intake and nutrient intake. The other is the ‘healthy eater’ effect (e.g., due to the intention of careful, health-conscious people to choose nutrient-dense, energy-sparse foods). This results in negative correlations between energy density and nutrient density. Thus far, we have observed that there is considerable variability in how these eating behaviours are distributed across different populations. In this population, it appears that the former effect of eating more of everything as one increases energy intake is stronger; hence, the mean energy intake is low in higher quartiles, which, in turn, means that the intakes of nutrients that are considered anti-inflammatory, such as vitamins and minerals, are also low.
Previously, in the ALSWH, we showed that increasing fruit consumption and adherence to the Mediterranean dietary pattern (garlic, peppers, mushrooms, salad greens, pasta and red wine) are protective against depression( Reference Mihrshahi, Dobson and Mishra 17 , Reference Rienks, Dobson and Mishra 18 ). In a recent publication from the ALSWH, no association was observed between Australian Recommended Food Score and Mediterranean dietary pattern and depression, after adjusting for time-varying covariates( Reference Lai, Oldmeadow and Hure 51 ). In addition, pooled results from two Australian cohorts showed people with lower intakes of Zn to be at higher risk of developing depression( Reference Vashum, McEvoy and Milton 52 ).
Furthermore, a review of the literature on the role of n-3 fatty acids and depression supports an inverse association between the two( Reference Liperoti, Landi and Fusco 15 ). In a large cohort study conducted in the USA, frequent consumption of sweetened beverages, especially diet drinks, was found to increase the risk of depression among older adults, whereas coffee consumption lowered the risk( Reference Guo, Park and Freedman 13 ). Various studies have been conducted examining the association between dietary patterns and depression. A systematic review conducted on twenty-one studies indicated that high intakes of fruit, vegetables, fish and wholegrains may be associated with a reduced depression risk( Reference Lai, Hiles and Bisquera 53 ). In analyses of a cross-sectional study conducted in the USA, which explored the association between various dietary patterns and depression, a healthy dietary pattern characterised by increased fruit and vegetable intakes was inversely associated with depression; increased risk was observed with the sweet dietary pattern characterised by increased intake of cookies, ice cream and chocolates. However, in the same study, no increased risk was observed with an unhealthy pattern characterised by increased intake of fried potatoes, cheese, processed meat and red meat( Reference Dipnall, Pasco and Meyer 54 ). In a US cohort study conducted exclusively among women, no clear association was observed between any dietary patterns (prudent and Western) derived from factor analysis and depression( Reference Chocano-Bedoya, O’Reilly and Lucas 55 ). Several studies have shown dietary factors and dietary patterns not to be associated with depression – for example, in a cross-sectional study in Japan, no statistically significant linear association was observed for the intake of riboflavin, pyridoxine, cobalamin, total n-3 PUFA, α-linolenic acid, EPA or DHA( Reference Murakami, Mizoue and Sasaki 56 ). Nutrients such as n-3 and Zn and food items such as fruits and vegetables, which are rich in components such as flavonoids and vitamins, and garlic, which are anti-inflammatory, produce more negative DII scores( Reference Shivappa, Steck and Hurley 22 ).
Several previous studies have established the link between inflammation and depression( Reference Hood, Lawrence and Anderson 5 , Reference Doyle, de Groot and Harris 6 , Reference Chocano-Bedoya, Mirzaei and O’Reilly 57 , Reference Au, Smith and Gariepy 58 ). Possible mechanisms for this observed association could be through the process of hyperleptinaemia or insulin resistance, which are observed in conditions such as obesity, the metabolic syndrome and type 2 diabetes mellitus and have been linked to inflammation processes, which are also common in depressive disorders( Reference Flehmig, Scholz and Kloting 59 ). Similarly, higher levels of cortisol have been observed among obese people, which in turn has resulted in higher hypothalamic–pituitary–adrenal axis reactivity to psychological stress as well as physiological and pharmacological stimulation( Reference Bjorntorp 60 ). Indeed, the pro-inflammatory dietary components could induce sensitisation with increased activation of the inflammatory response system. It has been shown that in people with depression, inflammation is increased( Reference Hegarty and Parker 14 , Reference Parker, Gibson and Brotchie 61 ) and brain-derived neurotrophic factor is reduced( Reference Belmaker and Agam 62 ).
The strengths of our study include its relatively large sample size and its use of a validated and tested CESD scale to measure depressive symptoms. The prospective nature of the study minimises potential problems resulting from reverse causation.
Limitations include the loss to follow-up (approximately 10 %), which may have introduced selection bias. Results from multiple imputations to assess the impact of missing data from confounding variables did not alter the conclusions on the association between DII and depression. Another limitation of our study is that detailed dietary information was collected just once, and thus if changes in diet occurred from survey 4 to 6 over the 6-year period they could not be accounted for in this analysis. However, previous studies have reported that dietary pattern classification is moderately stable over long periods of time during adulthood( Reference Jain, Howe and Harrison 63 – Reference Thompson, Metzner and Lamphiear 68 ). For the current study, data were available on only twenty-six of the forty-five food parameters, and therefore missing information on the remaining nineteen food parameters could be a limitation. The missing food items were anthocyanin, eugenol, flavan-3-ols, flavones, flavonols, flavonones, isoflavones, caffeine, ginger, saffron, turmeric, vitamin D, pepper, thyme and oregano, rosemary and Se. Several of these items are presumably not consumed frequently in the Australian population. Other food items such as flavonoids are more frequently consumed, and therefore might have influenced the DII scores computed in our study. Although we could not test and confirm this result in our cohort, other studies have observed relatively strong correlations between inflammatory biomarkers and DII when it was computed with fewer than thirty food parameters( Reference Tabung, Steck and Zhang 30 , Reference Shivappa, Hebert and Rietzschel 69 , Reference Shivappa, Steck and Hurley 70 ). Another limitation could be the absence of evidence of DII scores being associated with inflammatory markers in this study, as no data on inflammatory markers were available. However, the DII has been validated previously with a variety of inflammatory markers in various studies( Reference Tabung, Steck and Zhang 30 , Reference Shivappa, Hebert and Rietzschel 69 , Reference Shivappa, Steck and Hurley 70 ). Although the validity of the FFQ has been evaluated( Reference Hodge, Patterson and Brown 71 ), some degree of misclassification may exist in the dietary assessment. However, owing to the prospective nature of the study, the misclassification is unlikely to be differential. As such, the results probably would be biased towards the null. Another limitation of the FFQ is that it was validated on a small group of women of younger age than those included in the present study. Moreover, some of the results obtained could be biased because of residual confounding from measurement errors with factors such as education, marital status, physical activity and smoking status or unmeasured factors including psychosocial factors that might also be associated with depression (e.g., acquiescence)( Reference Edwards 72 ). We think that despite the small difference between quartile 4 and quartile 1, our findings may be relevant at a population level
In conclusion, anti-inflammatory diet as shown by lower DII scores was associated with a lower risk of developing depression in women from a population-based cohort study of middle-aged Australian women. Therefore, promoting diets with increased consumption of anti-inflammatory foods such as vegetables and fruits could protect against developing depression. However, further studies including both cohort and intervention studies, analysing the link between diet, inflammation and depression, are warranted to elucidate the causal role of diet in the development of depression and other mental disorders.
Acknowledgements
The research on which this paper is based was conducted as part of the ALSWH, the University of Newcastle and the University of Queensland. The authors are grateful to the Australian Government Department of Health for funding and to the women who provided the survey data. The authors thank Professor Graham Giles of the Cancer Epidemiology Centre of Cancer Council Victoria for permission to use the DQES (version 2), Melbourne: Cancer Council Victoria, 1996.
N. S. and J. R. H. were supported by grant number R44DK103377 from the United States National Institute of Diabetes and Digestive and Kidney Diseases. G. D. M. is supported by an Australian Research Council Future Fellowship (FT120100812). None of the funding organisations had any role in the design, analysis or writing of this article.
G. D. M. designed and conducted the study; N. S. calculated the DII scores and wrote the first draft of the manuscript; D. A. J. M. S. performed analyses and worked on the methods and results sections of the manuscript. N. S., D. A. J. M. S., J. R. H. and G. D. M. provided suggestions and revised the manuscript. All the authors approved the final version of the manuscript.
J. R. H. owns controlling interest in Connecting Health Innovations LLC (CHI), a company planning to licence the right to his invention of the DII from the University of South Carolina in order to develop computer and smart phone applications for patient counselling and dietary intervention in clinical settings. N. S. is an employee of CHI.
Supplementary material
For supplementary material/s referred to in this article, please visit http://dx.doi.org/doi:10.1017/S0007114516002853