Book contents
- Frontmatter
- Dedication
- Contents
- Preface
- Notation
- 1 Introduction
- 2 Simple examples
- 3 Embedded geometry: first order
- 4 First-order optimization algorithms
- 5 Embedded geometry: second order
- 6 Second-order optimization algorithms
- 7 Embedded submanifolds: examples
- 8 General manifolds
- 9 Quotient manifolds
- 10 Additional tools
- 11 Geodesic convexity
- References
- Index
- References
References
Published online by Cambridge University Press: 09 March 2023
- Frontmatter
- Dedication
- Contents
- Preface
- Notation
- 1 Introduction
- 2 Simple examples
- 3 Embedded geometry: first order
- 4 First-order optimization algorithms
- 5 Embedded geometry: second order
- 6 Second-order optimization algorithms
- 7 Embedded submanifolds: examples
- 8 General manifolds
- 9 Quotient manifolds
- 10 Additional tools
- 11 Geodesic convexity
- References
- Index
- References
Summary
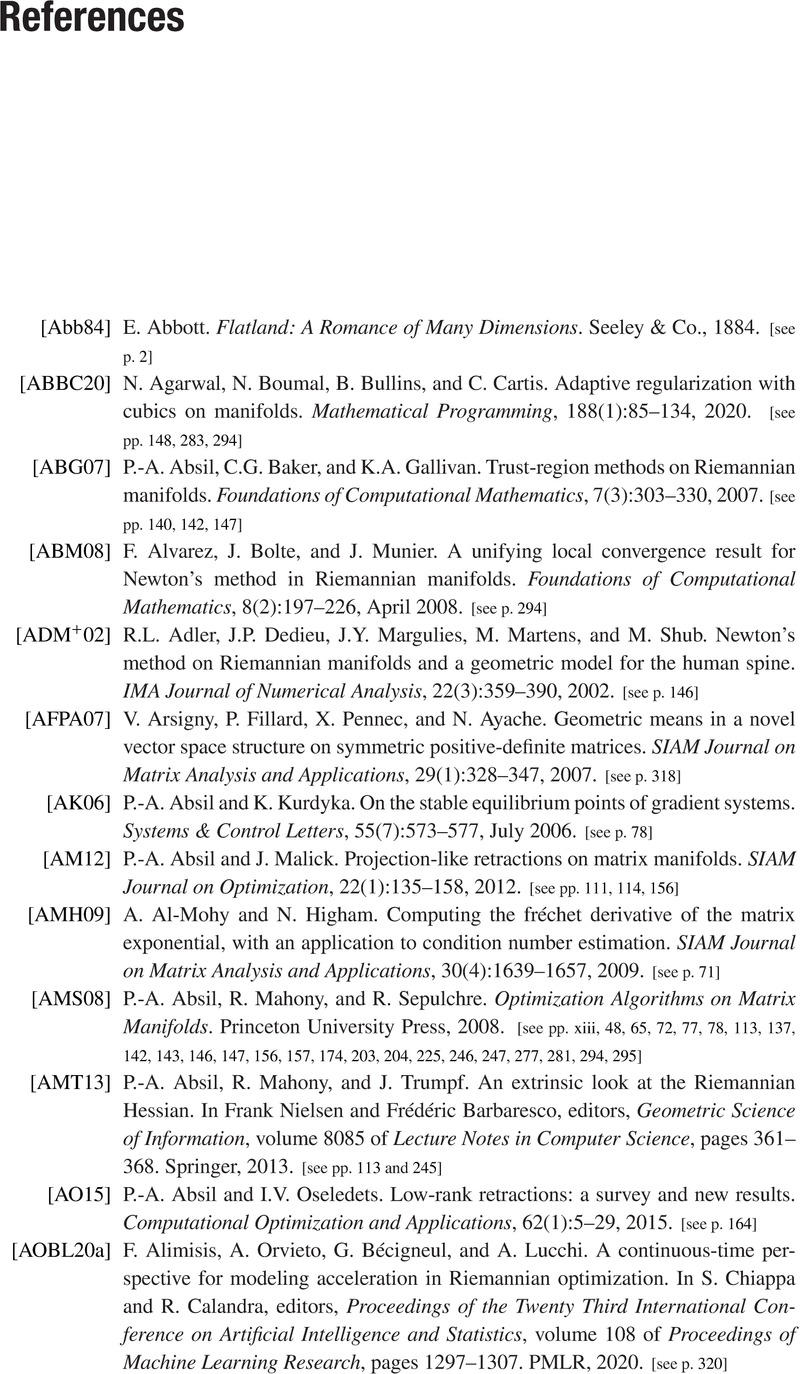
- Type
- Chapter
- Information
- An Introduction to Optimization on Smooth Manifolds , pp. 321 - 332Publisher: Cambridge University PressPrint publication year: 2023